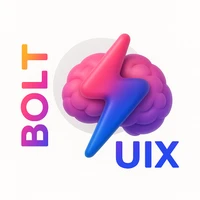
Datasets:
split
stringclasses 3
values | tokens
sequencelengths 1
275
| ner_tags
sequencelengths 1
275
|
---|---|---|
train | [
"Big",
"Managers",
"on",
"Campus"
] | [
"O",
"O",
"O",
"O"
] |
train | [
"In",
"recent",
"years",
",",
"advanced",
"education",
"for",
"professionals",
"has",
"become",
"a",
"hot",
"topic",
"in",
"the",
"business",
"community",
"."
] | [
"O",
"B-DATE",
"I-DATE",
"O",
"O",
"O",
"O",
"O",
"O",
"O",
"O",
"O",
"O",
"O",
"O",
"O",
"O",
"O"
] |
train | [
"With",
"this",
"trend",
",",
"suddenly",
"the",
"mature",
"faces",
"of",
"managers",
"boasting",
"an",
"average",
"of",
"over",
"ten",
"years",
"of",
"professional",
"experience",
"have",
"flooded",
"in",
"among",
"the",
"young",
"people",
"populating",
"university",
"campuses",
"."
] | [
"O",
"O",
"O",
"O",
"O",
"O",
"O",
"O",
"O",
"O",
"O",
"O",
"O",
"O",
"O",
"B-DATE",
"I-DATE",
"O",
"O",
"O",
"O",
"O",
"O",
"O",
"O",
"O",
"O",
"O",
"O",
"O",
"O"
] |
train | [
"In",
"order",
"to",
"attract",
"this",
"group",
"of",
"seasoned",
"adults",
"pulling",
"in",
"over",
"NT$",
"1",
"million",
"a",
"year",
"back",
"to",
"the",
"ivory",
"tower",
",",
"universities",
"have",
"begun",
"to",
"establish",
"executive",
"MBA",
"-LRB-",
"EMBA",
"-RRB-",
"programs",
"."
] | [
"O",
"O",
"O",
"O",
"O",
"O",
"O",
"O",
"O",
"O",
"O",
"O",
"B-MONEY",
"I-MONEY",
"I-MONEY",
"O",
"O",
"O",
"O",
"O",
"O",
"O",
"O",
"O",
"O",
"O",
"O",
"O",
"O",
"B-WORK_OF_ART",
"O",
"B-WORK_OF_ART",
"O",
"O",
"O"
] |
train | [
"In",
"response",
",",
"each",
"year",
"over",
"1000",
"mature",
"professionals",
"looking",
"to",
"recharge",
"their",
"minds",
"and",
"retool",
"their",
"know",
"-",
"how",
"compete",
"for",
"a",
"precious",
"few",
"openings",
"in",
"executive",
"degree",
"programs",
"at",
"top",
"institutions",
"such",
"as",
"National",
"Taiwan",
"University",
"-LRB-",
"NTU",
"-RRB-",
"and",
"National",
"Chengchi",
"University",
"."
] | [
"O",
"O",
"O",
"O",
"O",
"O",
"B-CARDINAL",
"O",
"O",
"O",
"O",
"O",
"O",
"O",
"O",
"O",
"O",
"O",
"O",
"O",
"O",
"O",
"O",
"O",
"O",
"O",
"O",
"O",
"O",
"O",
"O",
"O",
"O",
"O",
"O",
"B-ORG",
"I-ORG",
"I-ORG",
"O",
"B-ORG",
"O",
"O",
"B-ORG",
"I-ORG",
"I-ORG",
"O"
] |
train | [
"What",
"brings",
"these",
"accomplished",
"business",
"professionals",
"back",
"to",
"the",
"classroom",
"?"
] | [
"O",
"O",
"O",
"O",
"O",
"O",
"O",
"O",
"O",
"O",
"O"
] |
train | [
"What",
"knowledge",
"do",
"they",
"seek",
"that",
"can",
"not",
"be",
"found",
"in",
"society",
"at",
"large",
"?"
] | [
"O",
"O",
"O",
"O",
"O",
"O",
"O",
"O",
"O",
"O",
"O",
"O",
"O",
"O",
"O"
] |
train | [
"And",
"what",
"effect",
"does",
"their",
"return",
"have",
"on",
"campus",
"?"
] | [
"O",
"O",
"O",
"O",
"O",
"O",
"O",
"O",
"O",
"O"
] |
train | [
"Morris",
"Chang",
",",
"chairman",
"of",
"TSMC",
"and",
"a",
"leading",
"light",
"of",
"Taiwan",
"'s",
"high",
"-",
"tech",
"industry",
",",
"is",
"the",
"hottest",
"university",
"lecturer",
"around",
"."
] | [
"B-PERSON",
"I-PERSON",
"O",
"O",
"O",
"B-ORG",
"O",
"O",
"O",
"O",
"O",
"B-GPE",
"O",
"O",
"O",
"O",
"O",
"O",
"O",
"O",
"O",
"O",
"O",
"O",
"O"
] |
train | [
"Two",
"years",
"ago",
",",
"National",
"Chiaotung",
"University",
"invited",
"Chang",
",",
"one",
"of",
"the",
"Taiwan",
"IT",
"sector",
"'s",
"most",
"prominent",
"success",
"stories",
",",
"to",
"lecture",
"to",
"students",
"in",
"its",
"MBA",
"program",
"for",
"top",
"managers",
",",
"single",
"-",
"handedly",
"raising",
"awareness",
"of",
"executive",
"MBA",
"-LRB-",
"EMBA",
"-RRB-",
"programs",
"."
] | [
"B-DATE",
"I-DATE",
"I-DATE",
"O",
"B-ORG",
"I-ORG",
"I-ORG",
"O",
"B-PERSON",
"O",
"B-CARDINAL",
"O",
"O",
"B-GPE",
"O",
"O",
"O",
"O",
"O",
"O",
"O",
"O",
"O",
"O",
"O",
"O",
"O",
"O",
"B-WORK_OF_ART",
"O",
"O",
"O",
"O",
"O",
"O",
"O",
"O",
"O",
"O",
"O",
"O",
"B-WORK_OF_ART",
"O",
"B-WORK_OF_ART",
"O",
"O",
"O"
] |
train | [
"This",
"year",
",",
"Acer",
"Group",
"chairman",
"Stan",
"Shih",
"will",
"be",
"a",
"guest",
"lecturer",
"at",
"the",
"university",
"."
] | [
"B-DATE",
"I-DATE",
"O",
"B-ORG",
"I-ORG",
"O",
"B-PERSON",
"I-PERSON",
"O",
"O",
"O",
"O",
"O",
"O",
"O",
"O",
"O"
] |
train | [
"The",
"crush",
"of",
"business",
"professionals",
"fighting",
"for",
"a",
"spot",
"in",
"the",
"MBA",
"program",
"at",
"Taiwan",
"'s",
"most",
"illustrious",
"institution",
"of",
"higher",
"education",
"-",
"National",
"Taiwan",
"University",
"-",
"ensured",
"that",
"the",
"acceptance",
"rate",
"for",
"the",
"executive",
"graduate",
"school",
"was",
"even",
"lower",
"than",
"that",
"for",
"undergraduates",
"taking",
"the",
"national",
"university",
"entrance",
"examinations",
"."
] | [
"O",
"O",
"O",
"O",
"O",
"O",
"O",
"O",
"O",
"O",
"O",
"B-WORK_OF_ART",
"O",
"O",
"B-GPE",
"O",
"O",
"O",
"O",
"O",
"O",
"O",
"B-ORG",
"I-ORG",
"I-ORG",
"I-ORG",
"I-ORG",
"O",
"O",
"O",
"O",
"O",
"O",
"O",
"O",
"O",
"O",
"O",
"O",
"O",
"O",
"O",
"O",
"O",
"O",
"O",
"O",
"O",
"O",
"O",
"O"
] |
train | [
"Various",
"indications",
"demonstrate",
"that",
"the",
"\"",
"back",
"flow",
"education",
"\"",
"promoted",
"by",
"the",
"Ministry",
"of",
"Education",
"has",
"become",
"the",
"hottest",
"investment",
"undertaking",
"among",
"Taiwan",
"'s",
"universities",
"."
] | [
"O",
"O",
"O",
"O",
"O",
"O",
"O",
"O",
"O",
"O",
"O",
"O",
"B-ORG",
"I-ORG",
"I-ORG",
"I-ORG",
"O",
"O",
"O",
"O",
"O",
"O",
"O",
"B-GPE",
"O",
"O",
"O"
] |
train | [
"Three",
"years",
"ago",
",",
"in",
"the",
"effort",
"to",
"establish",
"a",
"greater",
"diversity",
"of",
"higher",
"educational",
"avenues",
",",
"the",
"Ministry",
"of",
"Education",
"-LRB-",
"MOE",
"-RRB-",
"greatly",
"increased",
"the",
"number",
"of",
"MA",
"degrees",
"open",
"to",
"professionals",
"in",
"the",
"workforce",
",",
"steadily",
"reducing",
"the",
"proportion",
"of",
"regular",
"students",
"to",
"professionals",
"from",
"the",
"previous",
"9",
"-",
"to",
"-",
"1",
"ratio",
"."
] | [
"B-DATE",
"I-DATE",
"I-DATE",
"O",
"O",
"O",
"O",
"O",
"O",
"O",
"O",
"O",
"O",
"O",
"O",
"O",
"O",
"B-ORG",
"I-ORG",
"I-ORG",
"I-ORG",
"O",
"B-ORG",
"O",
"O",
"O",
"O",
"O",
"O",
"B-WORK_OF_ART",
"O",
"O",
"O",
"O",
"O",
"O",
"O",
"O",
"O",
"O",
"O",
"O",
"O",
"O",
"O",
"O",
"O",
"O",
"O",
"O",
"B-CARDINAL",
"O",
"O",
"O",
"B-CARDINAL",
"O",
"O"
] |
train | [
"In",
"addition",
",",
"the",
"MOE",
"allowed",
"universities",
"to",
"determine",
"entrance",
"examination",
"topics",
",",
"testing",
"dates",
"and",
"times",
",",
"and",
"fee",
"structures",
"individually",
"to",
"help",
"them",
"find",
"and",
"obtain",
"the",
"best",
"students",
"."
] | [
"O",
"O",
"O",
"O",
"B-ORG",
"O",
"O",
"O",
"O",
"O",
"O",
"O",
"O",
"O",
"O",
"O",
"O",
"O",
"O",
"O",
"O",
"O",
"O",
"O",
"O",
"O",
"O",
"O",
"O",
"O",
"O",
"O"
] |
train | [
"Always",
"in",
"search",
"of",
"revenue",
"sources",
",",
"universities",
"naturally",
"fell",
"right",
"into",
"line",
"."
] | [
"O",
"O",
"O",
"O",
"O",
"O",
"O",
"O",
"O",
"O",
"O",
"O",
"O",
"O"
] |
train | [
"Twelve",
"institutions",
"opened",
"programs",
"in",
"1999",
",",
"with",
"the",
"number",
"of",
"executive",
"degree",
"programs",
"growing",
"to",
"34",
"as",
"of",
"this",
"year",
"."
] | [
"B-CARDINAL",
"O",
"O",
"O",
"O",
"B-DATE",
"O",
"O",
"O",
"O",
"O",
"O",
"O",
"O",
"O",
"O",
"B-CARDINAL",
"O",
"O",
"B-DATE",
"I-DATE",
"O"
] |
train | [
"And",
"as",
"the",
"supply",
"has",
"grown",
",",
"professionals",
"looking",
"to",
"recharge",
"their",
"intellectual",
"batteries",
"in",
"disciplines",
"ranging",
"from",
"Chinese",
",",
"foreign",
"languages",
",",
"and",
"physics",
"to",
"information",
"technology",
"and",
"management",
"now",
"have",
"a",
"greater",
"selection",
"from",
"which",
"to",
"choose",
"."
] | [
"O",
"O",
"O",
"O",
"O",
"O",
"O",
"O",
"O",
"O",
"O",
"O",
"O",
"O",
"O",
"O",
"O",
"O",
"B-NORP",
"O",
"O",
"O",
"O",
"O",
"O",
"O",
"O",
"O",
"O",
"O",
"O",
"O",
"O",
"O",
"O",
"O",
"O",
"O",
"O",
"O"
] |
train | [
"Management",
"white",
"-",
"hot"
] | [
"O",
"O",
"O",
"O"
] |
train | [
"A",
"wide",
"range",
"of",
"programs",
"is",
"available",
",",
"but",
"popularity",
"varies",
"greatly",
"."
] | [
"O",
"O",
"O",
"O",
"O",
"O",
"O",
"O",
"O",
"O",
"O",
"O",
"O"
] |
train | [
"Last",
"year",
",",
"National",
"Taiwan",
"University",
"of",
"Science",
"and",
"Technology",
"-LRB-",
"NTUST",
"-RRB-",
"opened",
"the",
"doors",
"of",
"five",
"of",
"its",
"graduate",
"schools",
"to",
"professionals",
"in",
"the",
"workforce",
"."
] | [
"B-DATE",
"I-DATE",
"O",
"B-ORG",
"I-ORG",
"I-ORG",
"I-ORG",
"I-ORG",
"I-ORG",
"I-ORG",
"O",
"B-ORG",
"O",
"O",
"O",
"O",
"O",
"B-CARDINAL",
"O",
"O",
"O",
"O",
"O",
"O",
"O",
"O",
"O",
"O"
] |
train | [
"Among",
"them",
",",
"the",
"Institute",
"of",
"Fiber",
"planned",
"to",
"accept",
"10",
"students",
",",
"but",
"only",
"20",
"applied",
",",
"resulting",
"in",
"a",
"50",
"%",
"acceptance",
"rate",
";",
"meanwhile",
",",
"the",
"Institute",
"of",
"Mechanical",
"Engineering",
"had",
"only",
"30",
"applicants",
"for",
"10",
"spots",
"."
] | [
"O",
"O",
"O",
"B-ORG",
"I-ORG",
"I-ORG",
"I-ORG",
"O",
"O",
"O",
"B-CARDINAL",
"O",
"O",
"O",
"O",
"B-CARDINAL",
"O",
"O",
"O",
"O",
"O",
"B-PERCENT",
"I-PERCENT",
"O",
"O",
"O",
"O",
"O",
"B-ORG",
"I-ORG",
"I-ORG",
"I-ORG",
"I-ORG",
"O",
"O",
"B-CARDINAL",
"O",
"O",
"B-CARDINAL",
"O",
"O"
] |
train | [
"Similarly",
",",
"nine",
"graduate",
"institutes",
"at",
"NTU",
",",
"including",
"mechanical",
"engineering",
",",
"Three",
"Principles",
"of",
"the",
"People",
",",
"and",
"biology",
",",
"opened",
"degree",
"programs",
"to",
"professionals",
"last",
"year",
"."
] | [
"O",
"O",
"B-CARDINAL",
"O",
"O",
"O",
"B-ORG",
"O",
"O",
"O",
"O",
"O",
"B-CARDINAL",
"O",
"O",
"O",
"O",
"O",
"O",
"O",
"O",
"O",
"O",
"O",
"O",
"O",
"B-DATE",
"I-DATE",
"O"
] |
train | [
"Expecting",
"to",
"accept",
"167",
"students",
",",
"they",
"attracted",
"a",
"mere",
"330",
"-",
"combined",
"applicants",
"."
] | [
"O",
"O",
"O",
"B-CARDINAL",
"O",
"O",
"O",
"O",
"O",
"O",
"B-CARDINAL",
"O",
"O",
"O",
"O"
] |
train | [
"Compared",
"to",
"the",
"lukewarm",
"popularity",
"of",
"other",
"graduate",
"programs",
",",
"management",
"institutes",
"are",
"white",
"hot",
"."
] | [
"O",
"O",
"O",
"O",
"O",
"O",
"O",
"O",
"O",
"O",
"O",
"O",
"O",
"O",
"O",
"O"
] |
train | [
"The",
"NTUST",
"College",
"of",
"Management",
"attracted",
"nearly",
"500",
"applicants",
"with",
"over",
"10",
"years",
"of",
"work",
"experience",
"for",
"20",
"spots",
"in",
"its",
"executive",
"management",
"program",
"beginning",
"in",
"1999",
"."
] | [
"O",
"B-ORG",
"B-ORG",
"I-ORG",
"I-ORG",
"O",
"O",
"B-CARDINAL",
"O",
"O",
"O",
"B-DATE",
"I-DATE",
"O",
"O",
"O",
"O",
"B-CARDINAL",
"O",
"O",
"O",
"O",
"O",
"O",
"O",
"O",
"B-DATE",
"O"
] |
train | [
"NTU",
"'s",
"College",
"of",
"Management",
"opened",
"its",
"doors",
"to",
"44",
"professionals",
"on",
"a",
"trial",
"basis",
"in",
"1996",
",",
"attracting",
"a",
"phenomenal",
"990",
"applicants",
"."
] | [
"B-ORG",
"O",
"B-ORG",
"I-ORG",
"I-ORG",
"O",
"O",
"O",
"O",
"B-CARDINAL",
"O",
"O",
"O",
"O",
"O",
"O",
"B-DATE",
"O",
"O",
"O",
"O",
"B-CARDINAL",
"O",
"O"
] |
train | [
"When",
"95",
"spots",
"were",
"offered",
"the",
"following",
"year",
",",
"the",
"number",
"of",
"applicants",
"rose",
"again",
"to",
"1400",
"."
] | [
"O",
"B-CARDINAL",
"O",
"O",
"O",
"B-DATE",
"I-DATE",
"I-DATE",
"O",
"O",
"O",
"O",
"O",
"O",
"O",
"O",
"B-CARDINAL",
"O"
] |
train | [
"Currently",
",",
"around",
"a",
"dozen",
"public",
"and",
"private",
"universities",
"in",
"Taiwan",
",",
"including",
"NTU",
",",
"National",
"Chiaotung",
"University",
",",
"National",
"Sun",
"Yat",
"-",
"sen",
"University",
",",
"Da",
"-",
"Yeh",
"Institute",
"of",
"Technology",
",",
"and",
"Yuan",
"-",
"Ze",
"Institute",
"of",
"Technology",
",",
"offer",
"advanced",
"degree",
"programs",
"for",
"executive",
"management",
"personnel",
"."
] | [
"O",
"O",
"B-CARDINAL",
"I-CARDINAL",
"I-CARDINAL",
"O",
"O",
"O",
"O",
"O",
"B-GPE",
"O",
"O",
"B-ORG",
"O",
"B-ORG",
"I-ORG",
"I-ORG",
"O",
"B-ORG",
"I-ORG",
"I-ORG",
"I-ORG",
"I-ORG",
"I-ORG",
"O",
"B-ORG",
"I-ORG",
"I-ORG",
"I-ORG",
"I-ORG",
"I-ORG",
"O",
"O",
"B-ORG",
"I-ORG",
"I-ORG",
"I-ORG",
"I-ORG",
"I-ORG",
"O",
"O",
"O",
"O",
"O",
"O",
"O",
"O",
"O",
"O"
] |
train | [
"What",
"is",
"it",
"that",
"makes",
"graduate",
"programs",
"in",
"management",
"so",
"popular",
"among",
"the",
"working",
"population",
"?"
] | [
"O",
"O",
"O",
"O",
"O",
"O",
"O",
"O",
"O",
"O",
"O",
"O",
"O",
"O",
"O",
"O"
] |
train | [
"Liao",
"Ching",
"-",
"jong",
",",
"director",
"of",
"the",
"NTUST",
"College",
"of",
"Management",
",",
"relates",
"that",
"new",
"entrants",
"into",
"the",
"professional",
"ranks",
"concentrate",
"on",
"accumulating",
"professional",
"know",
"-",
"how",
",",
"while",
"seasoned",
"middle",
"-",
"and",
"top",
"-",
"level",
"executives",
"seek",
"an",
"edge",
"in",
"management",
"."
] | [
"B-PERSON",
"I-PERSON",
"I-PERSON",
"I-PERSON",
"O",
"O",
"O",
"O",
"B-ORG",
"B-ORG",
"I-ORG",
"I-ORG",
"O",
"O",
"O",
"O",
"O",
"O",
"O",
"O",
"O",
"O",
"O",
"O",
"O",
"O",
"O",
"O",
"O",
"O",
"O",
"O",
"O",
"O",
"O",
"O",
"O",
"O",
"O",
"O",
"O",
"O",
"O",
"O"
] |
train | [
"And",
"while",
"most",
"recent",
"university",
"graduates",
"are",
"able",
"to",
"apply",
"professional",
"know",
"-",
"how",
"upon",
"entering",
"the",
"work",
"force",
",",
"one",
"is",
"not",
"necessarily",
"taught",
"management",
"in",
"the",
"workplace",
"."
] | [
"O",
"O",
"O",
"O",
"O",
"O",
"O",
"O",
"O",
"O",
"O",
"O",
"O",
"O",
"O",
"O",
"O",
"O",
"O",
"O",
"O",
"O",
"O",
"O",
"O",
"O",
"O",
"O",
"O",
"O"
] |
train | [
"Hence",
"universities",
"are",
"the",
"place",
"for",
"systematic",
"instruction",
"on",
"management",
"."
] | [
"O",
"O",
"O",
"O",
"O",
"O",
"O",
"O",
"O",
"O",
"O"
] |
train | [
"\"",
"Given",
"the",
"speed",
"at",
"which",
"the",
"business",
"environment",
"is",
"changing",
",",
"many",
"new",
"concepts",
"were",
"not",
"previously",
"taught",
",",
"\"",
"says",
"Wu",
"Se",
"-",
"hwa",
",",
"dean",
"of",
"National",
"Chengchi",
"University",
"'s",
"College",
"of",
"Commerce",
"."
] | [
"O",
"O",
"O",
"O",
"O",
"O",
"O",
"O",
"O",
"O",
"O",
"O",
"O",
"O",
"O",
"O",
"O",
"O",
"O",
"O",
"O",
"O",
"B-PERSON",
"I-PERSON",
"I-PERSON",
"I-PERSON",
"O",
"O",
"O",
"B-ORG",
"I-ORG",
"I-ORG",
"I-ORG",
"B-ORG",
"I-ORG",
"I-ORG",
"O"
] |
train | [
"Wu",
"notes",
"that",
"such",
"new",
"fields",
"as",
"venture",
"capital",
",",
"technology",
"management",
",",
"intellectual",
"property",
",",
"and",
"e-commerce",
"are",
"all",
"recent",
"additions",
"to",
"the",
"curriculum",
"over",
"the",
"past",
"few",
"years",
"."
] | [
"B-PERSON",
"O",
"O",
"O",
"O",
"O",
"O",
"O",
"O",
"O",
"O",
"O",
"O",
"O",
"O",
"O",
"O",
"O",
"O",
"O",
"O",
"O",
"O",
"O",
"O",
"O",
"B-DATE",
"I-DATE",
"I-DATE",
"I-DATE",
"O"
] |
train | [
"Professor",
"Tang",
"Ming",
"-",
"je",
"of",
"NTU",
"'s",
"College",
"of",
"Management",
"adds",
":",
"\"",
"With",
"small",
",",
"medium",
"-",
"size",
"and",
"family",
"businesses",
"forming",
"the",
"backbone",
"of",
"Taiwanese",
"industry",
",",
"one",
"could",
"do",
"just",
"fine",
"without",
"professional",
"management",
"."
] | [
"O",
"B-PERSON",
"I-PERSON",
"I-PERSON",
"I-PERSON",
"O",
"B-ORG",
"O",
"B-ORG",
"I-ORG",
"I-ORG",
"O",
"O",
"O",
"O",
"O",
"O",
"O",
"O",
"O",
"O",
"O",
"O",
"O",
"O",
"O",
"O",
"B-NORP",
"O",
"O",
"O",
"O",
"O",
"O",
"O",
"O",
"O",
"O",
"O"
] |
train | [
"But",
"in",
"the",
"age",
"of",
"the",
"global",
"village",
",",
"many",
"bosses",
"are",
"finding",
"that",
"relying",
"on",
"'",
"instinct",
"'",
"to",
"make",
"business",
"decisions",
"is",
"not",
"enough",
",",
"and",
"that",
"they",
"need",
"systematic",
"management",
"concepts",
".",
"\""
] | [
"O",
"O",
"O",
"O",
"O",
"O",
"O",
"O",
"O",
"O",
"O",
"O",
"O",
"O",
"O",
"O",
"O",
"O",
"O",
"O",
"O",
"O",
"O",
"O",
"O",
"O",
"O",
"O",
"O",
"O",
"O",
"O",
"O",
"O",
"O",
"O"
] |
train | [
"Other",
"management",
"experts",
"note",
"that",
"once",
"Taiwan",
"joins",
"the",
"World",
"Trade",
"Organization",
"-LRB-",
"WTO",
"-RRB-",
",",
"foreign",
"universities",
"will",
"be",
"able",
"to",
"recruit",
"students",
"directly",
"in",
"Taiwan",
",",
"and",
"that",
"with",
"the",
"Ministry",
"of",
"Education",
"asking",
"national",
"universities",
"to",
"come",
"up",
"with",
"a",
"portion",
"of",
"their",
"own",
"funding",
",",
"universities",
"are",
"doubly",
"motivated",
"to",
"establish",
"programs",
"of",
"this",
"sort",
"."
] | [
"O",
"O",
"O",
"O",
"O",
"O",
"B-GPE",
"O",
"B-ORG",
"I-ORG",
"I-ORG",
"I-ORG",
"O",
"B-ORG",
"O",
"O",
"O",
"O",
"O",
"O",
"O",
"O",
"O",
"O",
"O",
"O",
"B-GPE",
"O",
"O",
"O",
"O",
"O",
"B-ORG",
"I-ORG",
"I-ORG",
"O",
"O",
"O",
"O",
"O",
"O",
"O",
"O",
"O",
"O",
"O",
"O",
"O",
"O",
"O",
"O",
"O",
"O",
"O",
"O",
"O",
"O",
"O",
"O",
"O"
] |
train | [
"NTU",
",",
"which",
"enjoys",
"the",
"distinction",
"of",
"being",
"Taiwan",
"'s",
"premier",
"institution",
"of",
"higher",
"education",
",",
"\"",
"never",
"lacks",
"for",
"students",
",",
"because",
"there",
"are",
"always",
"people",
"who",
"want",
"to",
"attend",
"NTU",
",",
"\"",
"says",
"Tang",
"Ming",
"-",
"je",
"."
] | [
"B-ORG",
"O",
"O",
"O",
"O",
"O",
"O",
"O",
"B-GPE",
"O",
"O",
"O",
"O",
"O",
"O",
"O",
"O",
"O",
"O",
"O",
"O",
"O",
"O",
"O",
"O",
"O",
"O",
"O",
"O",
"O",
"O",
"B-ORG",
"O",
"O",
"O",
"B-PERSON",
"I-PERSON",
"I-PERSON",
"I-PERSON",
"O"
] |
train | [
"More",
"than",
"a",
"diploma"
] | [
"O",
"O",
"O",
"O"
] |
train | [
"In",
"order",
"to",
"accommodate",
"professionals",
"with",
"day",
"jobs",
",",
"many",
"universities",
"have",
"scheduled",
"their",
"business",
"management",
"courses",
"during",
"evening",
"hours",
"."
] | [
"O",
"O",
"O",
"O",
"O",
"O",
"O",
"O",
"O",
"O",
"O",
"O",
"O",
"O",
"O",
"O",
"O",
"O",
"O",
"O",
"O"
] |
train | [
"The",
"sight",
"of",
"professionals",
"in",
"suits",
"and",
"ties",
"rushing",
"to",
"class",
"at",
"dusk",
"contrasts",
"greatly",
"from",
"the",
"typical",
"jeans",
"and",
"casual",
"attire",
"of",
"fresh",
"-",
"faced",
"undergrads",
"."
] | [
"O",
"O",
"O",
"O",
"O",
"O",
"O",
"O",
"O",
"O",
"O",
"O",
"O",
"O",
"O",
"O",
"O",
"O",
"O",
"O",
"O",
"O",
"O",
"O",
"O",
"O",
"O",
"O"
] |
train | [
"Shuttling",
"between",
"the",
"office",
"and",
"the",
"campus",
",",
"do",
"they",
"ever",
"feel",
"like",
"they",
"'re",
"burning",
"the",
"candle",
"at",
"both",
"ends",
"?"
] | [
"O",
"O",
"O",
"O",
"O",
"O",
"O",
"O",
"O",
"O",
"O",
"O",
"O",
"O",
"O",
"O",
"O",
"O",
"O",
"O",
"O",
"O"
] |
train | [
"At",
"some",
"schools",
",",
"even",
"professionals",
"boasting",
"Ph.D.",
"degrees",
"are",
"coming",
"back",
"to",
"school",
"for",
"Master",
"'s",
"degrees",
"."
] | [
"O",
"O",
"O",
"O",
"O",
"O",
"O",
"B-WORK_OF_ART",
"O",
"O",
"O",
"O",
"O",
"O",
"O",
"B-WORK_OF_ART",
"I-WORK_OF_ART",
"I-WORK_OF_ART",
"O"
] |
train | [
"Is",
"the",
"degree",
"really",
"that",
"important",
"to",
"them",
"?"
] | [
"O",
"O",
"O",
"O",
"O",
"O",
"O",
"O",
"O"
] |
train | [
"Mouton",
"Lee",
",",
"in",
"charge",
"of",
"over",
"80",
"employees",
"and",
"production",
"of",
"key",
"components",
"as",
"president",
"of",
"ABBA",
"Linear",
"Tech",
"Co.",
",",
"Ltd.",
",",
"is",
"a",
"second",
"-",
"year",
"student",
"in",
"the",
"NTUST",
"Graduate",
"School",
"of",
"Management",
"."
] | [
"B-PERSON",
"I-PERSON",
"O",
"O",
"O",
"O",
"O",
"B-CARDINAL",
"O",
"O",
"O",
"O",
"O",
"O",
"O",
"O",
"O",
"B-ORG",
"I-ORG",
"I-ORG",
"I-ORG",
"I-ORG",
"I-ORG",
"O",
"O",
"O",
"B-DATE",
"I-DATE",
"I-DATE",
"O",
"O",
"O",
"B-ORG",
"B-ORG",
"I-ORG",
"I-ORG",
"I-ORG",
"O"
] |
train | [
"Lee",
"relates",
"that",
"as",
"a",
"youth",
"he",
"studied",
"because",
"he",
"was",
"forced",
"to",
",",
"but",
"now",
"his",
"motivations",
"for",
"learning",
"are",
"far",
"greater",
"-",
"so",
"great",
"in",
"fact",
"that",
"he",
"registered",
"for",
"three",
"classes",
"at",
"the",
"start",
"of",
"the",
"semester",
",",
"a",
"decision",
"he",
"has",
"come",
"to",
"regret",
"for",
"lack",
"of",
"time",
"."
] | [
"B-PERSON",
"O",
"O",
"O",
"O",
"O",
"O",
"O",
"O",
"O",
"O",
"O",
"O",
"O",
"O",
"O",
"O",
"O",
"O",
"O",
"O",
"O",
"O",
"O",
"O",
"O",
"O",
"O",
"O",
"O",
"O",
"O",
"B-CARDINAL",
"O",
"O",
"B-DATE",
"I-DATE",
"I-DATE",
"I-DATE",
"I-DATE",
"O",
"O",
"O",
"O",
"O",
"O",
"O",
"O",
"O",
"O",
"O",
"O",
"O"
] |
train | [
"Lee",
",",
"who",
"has",
"also",
"taken",
"short",
"-",
"term",
"management",
"courses",
",",
"says",
",",
"\"",
"Degree",
"programs",
"are",
"better",
",",
"since",
"the",
"pressure",
"makes",
"you",
"grow",
".",
"\""
] | [
"B-PERSON",
"O",
"O",
"O",
"O",
"O",
"O",
"O",
"O",
"O",
"O",
"O",
"O",
"O",
"O",
"O",
"O",
"O",
"O",
"O",
"O",
"O",
"O",
"O",
"O",
"O",
"O",
"O"
] |
train | [
"Mouton",
"Lee",
"would",
"like",
"a",
"Master",
"'s",
"degree",
",",
"yet",
"today",
"he",
"studies",
"for",
"the",
"knowledge",
"it",
"brings",
"."
] | [
"B-PERSON",
"I-PERSON",
"O",
"O",
"B-WORK_OF_ART",
"I-WORK_OF_ART",
"I-WORK_OF_ART",
"I-WORK_OF_ART",
"O",
"O",
"B-DATE",
"O",
"O",
"O",
"O",
"O",
"O",
"O",
"O"
] |
train | [
"Ever",
"since",
"graduating",
"with",
"a",
"degree",
"in",
"mechanical",
"engineering",
"from",
"NTUST",
"and",
"setting",
"out",
"to",
"start",
"his",
"own",
"business",
"over",
"20",
"years",
"ago",
",",
"that",
"degree",
"has",
"sat",
"in",
"a",
"drawer",
"somewhere",
",",
"relegated",
"to",
"mere",
"symbolic",
"significance",
"."
] | [
"O",
"O",
"O",
"O",
"O",
"O",
"O",
"O",
"O",
"O",
"B-ORG",
"O",
"O",
"O",
"O",
"O",
"O",
"O",
"O",
"O",
"B-DATE",
"I-DATE",
"I-DATE",
"O",
"O",
"O",
"O",
"O",
"O",
"O",
"O",
"O",
"O",
"O",
"O",
"O",
"O",
"O",
"O"
] |
train | [
"Hsieh",
"Sun",
"-",
"chi",
",",
"director",
"of",
"the",
"Control",
"Yuan",
"'s",
"Office",
"of",
"Information",
"Management",
"and",
"a",
"25",
"-",
"year",
"veteran",
"of",
"civil",
"service",
",",
"relates",
"that",
"he",
"has",
"already",
"attained",
"the",
"highest",
"rank",
"a",
"general",
"civil",
"servant",
"can",
"achieve",
",",
"yet",
"at",
"the",
"age",
"of",
"50",
"he",
"still",
"has",
"over",
"a",
"decade",
"until",
"retirement",
"."
] | [
"B-PERSON",
"I-PERSON",
"I-PERSON",
"I-PERSON",
"O",
"O",
"O",
"B-ORG",
"I-ORG",
"I-ORG",
"I-ORG",
"B-ORG",
"I-ORG",
"I-ORG",
"I-ORG",
"O",
"O",
"B-DATE",
"I-DATE",
"I-DATE",
"O",
"O",
"O",
"O",
"O",
"O",
"O",
"O",
"O",
"O",
"O",
"O",
"O",
"O",
"O",
"O",
"O",
"O",
"O",
"O",
"O",
"O",
"O",
"O",
"O",
"O",
"B-DATE",
"O",
"O",
"O",
"O",
"O",
"O",
"O",
"O",
"O"
] |
train | [
"Two",
"years",
"ago",
",",
"this",
"led",
"him",
"to",
"consider",
"taking",
"a",
"new",
"direction",
",",
"and",
"he",
"tested",
"into",
"National",
"Chengchi",
"University",
"'s",
"College",
"of",
"Commerce",
"intent",
"on",
"making",
"connections",
"with",
"movers",
"and",
"shakers",
"in",
"different",
"fields",
"."
] | [
"B-DATE",
"I-DATE",
"I-DATE",
"O",
"O",
"O",
"O",
"O",
"O",
"O",
"O",
"O",
"O",
"O",
"O",
"O",
"O",
"O",
"B-ORG",
"I-ORG",
"I-ORG",
"I-ORG",
"B-ORG",
"I-ORG",
"I-ORG",
"O",
"O",
"O",
"O",
"O",
"O",
"O",
"O",
"O",
"O",
"O",
"O"
] |
train | [
"Chang",
"Chin",
"-",
"tsung",
",",
"a",
"researcher",
"at",
"the",
"Chung",
"-",
"Shan",
"Institute",
"of",
"Science",
"&",
"Technology",
"with",
"a",
"Ph.D.",
"in",
"mechanical",
"engineering",
"from",
"a",
"US",
"university",
",",
"shuttles",
"between",
"his",
"office",
"in",
"Taoyuan",
"s",
"Lungtan",
"and",
"the",
"NTU",
"campus",
"three",
"days",
"each",
"week",
"."
] | [
"B-PERSON",
"I-PERSON",
"I-PERSON",
"I-PERSON",
"O",
"O",
"O",
"O",
"B-ORG",
"I-ORG",
"I-ORG",
"I-ORG",
"I-ORG",
"I-ORG",
"I-ORG",
"I-ORG",
"I-ORG",
"O",
"O",
"B-WORK_OF_ART",
"O",
"O",
"O",
"O",
"O",
"B-GPE",
"O",
"O",
"O",
"O",
"O",
"O",
"O",
"B-GPE",
"O",
"B-GPE",
"O",
"O",
"B-ORG",
"O",
"B-DATE",
"I-DATE",
"O",
"O",
"O"
] |
train | [
"Long",
"immersed",
"in",
"the",
"sciences",
"and",
"always",
"focusing",
"on",
"numbers",
"and",
"statistics",
",",
"Chang",
"says",
"he",
"feels",
"like",
"he",
"\"",
"has",
"gotten",
"into",
"a",
"mental",
"rut",
".",
"\""
] | [
"O",
"O",
"O",
"O",
"O",
"O",
"O",
"O",
"O",
"O",
"O",
"O",
"O",
"B-PERSON",
"O",
"O",
"O",
"O",
"O",
"O",
"O",
"O",
"O",
"O",
"O",
"O",
"O",
"O"
] |
train | [
"Although",
"Hsinchu",
"'s",
"National",
"Chiaotung",
"University",
"offers",
"a",
"similar",
"management",
"curriculum",
",",
"he",
"was",
"concerned",
"that",
"the",
"students",
"there",
"would",
"be",
"science",
"park",
"types",
"with",
"similar",
"backgrounds",
"in",
"high",
"tech",
"fields",
"."
] | [
"O",
"B-GPE",
"O",
"B-ORG",
"I-ORG",
"I-ORG",
"O",
"O",
"O",
"O",
"O",
"O",
"O",
"O",
"O",
"O",
"O",
"O",
"O",
"O",
"O",
"O",
"O",
"O",
"O",
"O",
"O",
"O",
"O",
"O",
"O",
"O"
] |
train | [
"Thus",
"he",
"would",
"rather",
"spend",
"the",
"extra",
"time",
"commuting",
"to",
"NTU",
"to",
"be",
"around",
"students",
"with",
"experience",
"in",
"such",
"fields",
"as",
"advertising",
",",
"finance",
",",
"and",
"architecture",
"."
] | [
"O",
"O",
"O",
"O",
"O",
"O",
"O",
"O",
"O",
"O",
"B-ORG",
"O",
"O",
"O",
"O",
"O",
"O",
"O",
"O",
"O",
"O",
"O",
"O",
"O",
"O",
"O",
"O",
"O"
] |
train | [
"\"",
"I",
"'d",
"be",
"lying",
"if",
"I",
"said",
"the",
"degree",
"does",
"n't",
"matter",
"."
] | [
"O",
"O",
"O",
"O",
"O",
"O",
"O",
"O",
"O",
"O",
"O",
"O",
"O",
"O"
] |
train | [
"If",
"it",
"did",
"n't",
",",
"why",
"not",
"just",
"get",
"into",
"a",
"non-degree",
"program",
"?"
] | [
"O",
"O",
"O",
"O",
"O",
"O",
"O",
"O",
"O",
"O",
"O",
"O",
"O",
"O"
] |
train | [
"The",
"truth",
"is",
"that",
"a",
"degree",
"in",
"management",
"does",
"nothing",
"to",
"help",
"me",
"in",
"my",
"current",
"job",
",",
"but",
"it",
"gives",
"me",
"a",
"second",
"skill",
"if",
"I",
"choose",
"another",
"route",
"in",
"the",
"future",
".",
"\""
] | [
"O",
"O",
"O",
"O",
"O",
"O",
"O",
"O",
"O",
"O",
"O",
"O",
"O",
"O",
"O",
"O",
"O",
"O",
"O",
"O",
"O",
"O",
"O",
"B-ORDINAL",
"O",
"O",
"O",
"O",
"O",
"O",
"O",
"O",
"O",
"O",
"O"
] |
train | [
"Advanced",
"training",
"for",
"professionals",
"has",
"become",
"a",
"hot",
"topic",
"among",
"executives",
"over",
"the",
"past",
"two",
"years",
",",
"but",
"continuing",
"education",
"is",
"nothing",
"new",
"among",
"Taiwan",
"'s",
"universities",
"."
] | [
"O",
"O",
"O",
"O",
"O",
"O",
"O",
"O",
"O",
"O",
"O",
"O",
"B-DATE",
"I-DATE",
"I-DATE",
"I-DATE",
"O",
"O",
"O",
"O",
"O",
"O",
"O",
"O",
"B-GPE",
"O",
"O",
"O"
] |
train | [
"For",
"instance",
",",
"National",
"Chengchi",
"University",
"'s",
"Public",
"Administration",
"Center",
"has",
"offered",
"graduate",
"extension",
"courses",
"for",
"executives",
"for",
"over",
"a",
"decade",
",",
"the",
"difference",
"being",
"that",
",",
"upon",
"completion",
"of",
"the",
"curriculum",
"students",
"only",
"receive",
"a",
"certificate",
"of",
"course",
"credit",
"."
] | [
"O",
"O",
"O",
"B-ORG",
"I-ORG",
"I-ORG",
"I-ORG",
"B-ORG",
"I-ORG",
"I-ORG",
"O",
"O",
"O",
"O",
"O",
"O",
"O",
"O",
"O",
"O",
"B-DATE",
"O",
"O",
"O",
"O",
"O",
"O",
"O",
"O",
"O",
"O",
"O",
"O",
"O",
"O",
"O",
"O",
"O",
"O",
"O",
"O"
] |
train | [
"In",
"comparison",
",",
"a",
"Master",
"'s",
"degree",
"is",
"far",
"more",
"appealing",
",",
"given",
"that",
"a",
"degree",
"is",
"awarded",
"upon",
"completion",
"of",
"studies",
"."
] | [
"O",
"O",
"O",
"B-WORK_OF_ART",
"I-WORK_OF_ART",
"I-WORK_OF_ART",
"I-WORK_OF_ART",
"O",
"O",
"O",
"O",
"O",
"O",
"O",
"O",
"O",
"O",
"O",
"O",
"O",
"O",
"O",
"O"
] |
train | [
"Burning",
"the",
"proverbial",
"candle"
] | [
"O",
"O",
"O",
"O"
] |
train | [
"Motivated",
"by",
"the",
"degree",
"or",
"not",
",",
"the",
"enthusiasm",
"of",
"these",
"professionals",
"for",
"their",
"studies",
"is",
"impressive",
"."
] | [
"O",
"O",
"O",
"O",
"O",
"O",
"O",
"O",
"O",
"O",
"O",
"O",
"O",
"O",
"O",
"O",
"O",
"O"
] |
train | [
"Nevertheless",
",",
"they",
"must",
"pay",
"a",
"hefty",
"price",
"to",
"be",
"able",
"to",
"study",
"in",
"the",
"first",
"place",
"."
] | [
"O",
"O",
"O",
"O",
"O",
"O",
"O",
"O",
"O",
"O",
"O",
"O",
"O",
"O",
"O",
"B-ORDINAL",
"O",
"O"
] |
train | [
"First",
",",
"working",
"during",
"the",
"day",
"and",
"attending",
"classes",
"at",
"night",
"is",
"often",
"a",
"war",
"of",
"energy",
"and",
"will",
"."
] | [
"B-ORDINAL",
"O",
"O",
"O",
"O",
"O",
"O",
"O",
"O",
"O",
"O",
"O",
"O",
"O",
"O",
"O",
"O",
"O",
"O",
"O"
] |
train | [
"Next",
",",
"they",
"must",
"shuffle",
"their",
"work",
"schedules",
"to",
"accommodate",
"their",
"studies",
"."
] | [
"O",
"O",
"O",
"O",
"O",
"O",
"O",
"O",
"O",
"O",
"O",
"O",
"O"
] |
train | [
"Many",
"of",
"them",
"have",
"to",
"reschedule",
"business",
"trips",
"."
] | [
"O",
"O",
"O",
"O",
"O",
"O",
"O",
"O",
"O"
] |
train | [
"Meanwhile",
",",
"some",
"become",
"highway",
"speeders",
",",
"rushing",
"from",
"Taoyuan",
"or",
"Hsinchu",
"to",
"attend",
"class",
"."
] | [
"O",
"O",
"O",
"O",
"O",
"O",
"O",
"O",
"O",
"B-GPE",
"O",
"B-GPE",
"O",
"O",
"O",
"O"
] |
train | [
"And",
"female",
"managers",
",",
"ordinarily",
"responsible",
"for",
"household",
"chores",
"as",
"well",
",",
"really",
"burn",
"the",
"proverbial",
"candle",
"at",
"both",
"ends",
"."
] | [
"O",
"O",
"O",
"O",
"O",
"O",
"O",
"O",
"O",
"O",
"O",
"O",
"O",
"O",
"O",
"O",
"O",
"O",
"O",
"O",
"O"
] |
train | [
"\"",
"I",
"have",
"my",
"time",
"perfectly",
"allotted",
",",
"\"",
"says",
"Morna",
"Lee",
",",
"Taiwan",
"regional",
"business",
"manager",
"for",
"Kellogg",
"'s",
"."
] | [
"O",
"O",
"O",
"O",
"O",
"O",
"O",
"O",
"O",
"O",
"B-PERSON",
"I-PERSON",
"O",
"B-GPE",
"O",
"O",
"O",
"O",
"B-ORG",
"I-ORG",
"O"
] |
train | [
"She",
"divides",
"her",
"schedule",
"into",
"three",
"-",
"month",
"cycles",
",",
"and",
"with",
"one",
"class",
"on",
"Thursday",
"afternoons",
",",
"her",
"company",
"does",
"its",
"best",
"to",
"accommodate",
"her",
"by",
"not",
"holding",
"meetings",
"that",
"involve",
"her",
"on",
"that",
"day",
"of",
"the",
"week",
"."
] | [
"O",
"O",
"O",
"O",
"O",
"B-DATE",
"I-DATE",
"I-DATE",
"O",
"O",
"O",
"O",
"B-CARDINAL",
"O",
"O",
"B-DATE",
"O",
"O",
"O",
"O",
"O",
"O",
"O",
"O",
"O",
"O",
"O",
"O",
"O",
"O",
"O",
"O",
"O",
"O",
"B-DATE",
"I-DATE",
"I-DATE",
"I-DATE",
"I-DATE",
"O"
] |
train | [
"Still",
",",
"sometimes",
"nothing",
"can",
"be",
"done",
",",
"such",
"as",
"the",
"time",
"that",
"she",
"had",
"a",
"meeting",
"in",
"Hong",
"Kong",
"early",
"one",
"Friday",
"morning",
",",
"forcing",
"her",
"to",
"miss",
"class",
"on",
"Thursday",
"while",
"she",
"flew",
"to",
"Hong",
"Kong",
"."
] | [
"O",
"O",
"O",
"O",
"O",
"O",
"O",
"O",
"O",
"O",
"O",
"O",
"O",
"O",
"O",
"O",
"O",
"O",
"B-GPE",
"I-GPE",
"B-TIME",
"I-TIME",
"I-TIME",
"I-TIME",
"O",
"O",
"O",
"O",
"O",
"O",
"O",
"B-DATE",
"O",
"O",
"O",
"O",
"B-GPE",
"I-GPE",
"O"
] |
train | [
"She",
"had",
"to",
"fly",
"quickly",
"back",
"to",
"Taipei",
"to",
"make",
"class",
"on",
"Saturday",
"afternoon",
"."
] | [
"O",
"O",
"O",
"O",
"O",
"O",
"O",
"B-GPE",
"O",
"O",
"O",
"O",
"B-TIME",
"I-TIME",
"O"
] |
train | [
"Despite",
"often",
"staying",
"up",
"until",
"2:00",
"or",
"3:00",
"AM",
"to",
"study",
"or",
"write",
"reports",
",",
"Lee",
"likes",
"mingling",
"in",
"the",
"executive",
"management",
"class",
"with",
"a",
"diverse",
"group",
"of",
"students",
"from",
"different",
"fields",
",",
"and",
"feels",
"that",
"the",
"new",
"ideas",
"the",
"professors",
"feed",
"them",
"help",
"keep",
"her",
"brain",
"supple",
"."
] | [
"O",
"O",
"O",
"O",
"O",
"B-TIME",
"I-TIME",
"I-TIME",
"I-TIME",
"O",
"O",
"O",
"O",
"O",
"O",
"B-PERSON",
"O",
"O",
"O",
"O",
"O",
"O",
"O",
"O",
"O",
"O",
"O",
"O",
"O",
"O",
"O",
"O",
"O",
"O",
"O",
"O",
"O",
"O",
"O",
"O",
"O",
"O",
"O",
"O",
"O",
"O",
"O",
"O",
"O"
] |
train | [
"Moreover",
",",
"she",
"muses",
",",
"\"",
"We",
"managers",
"are",
"a",
"strange",
"breed",
"."
] | [
"O",
"O",
"O",
"O",
"O",
"O",
"O",
"O",
"O",
"O",
"O",
"O",
"O"
] |
train | [
"If",
"we",
"do",
"n't",
"exhaust",
"ourselves",
",",
"we",
"end",
"up",
"sick",
".",
"\""
] | [
"O",
"O",
"O",
"O",
"O",
"O",
"O",
"O",
"O",
"O",
"O",
"O",
"O"
] |
train | [
"Female",
"professionals",
"like",
"Morna",
"Lee",
"represent",
"a",
"minority",
"among",
"executive",
"degree",
"students",
",",
"accounting",
"for",
"just",
"about",
"five",
"percent",
"."
] | [
"O",
"O",
"O",
"B-PERSON",
"I-PERSON",
"O",
"O",
"O",
"O",
"O",
"O",
"O",
"O",
"O",
"O",
"O",
"B-PERCENT",
"I-PERCENT",
"I-PERCENT",
"O"
] |
train | [
"\"",
"If",
"you",
"asked",
"me",
"whether",
"work",
"or",
"family",
"is",
"more",
"important",
",",
"I",
"would",
"say",
"that",
"since",
"entering",
"society",
"20",
"years",
"ago",
"work",
"has",
"always",
"come",
"first",
",",
"\"",
"states",
"Donna",
"Sun",
",",
"vice",
"president",
"of",
"the",
"Human",
"Resource",
"Division",
"at",
"Schmidt",
"Scientific",
"Taiwan",
"."
] | [
"O",
"O",
"O",
"O",
"O",
"O",
"O",
"O",
"O",
"O",
"O",
"O",
"O",
"O",
"O",
"O",
"O",
"O",
"O",
"O",
"B-DATE",
"I-DATE",
"I-DATE",
"O",
"O",
"O",
"O",
"B-ORDINAL",
"O",
"O",
"O",
"B-PERSON",
"I-PERSON",
"O",
"O",
"O",
"O",
"B-ORG",
"I-ORG",
"I-ORG",
"I-ORG",
"O",
"B-ORG",
"I-ORG",
"I-ORG",
"O"
] |
train | [
"Even",
"if",
"she",
"has",
"no",
"classes",
"to",
"attend",
",",
"leaving",
"the",
"office",
"at",
"8:00",
"PM",
"is",
"no",
"different",
"from",
"the",
"perspective",
"of",
"her",
"family",
"life",
"than",
"the",
"extra",
"hour",
"she",
"spends",
"attending",
"classes",
"until",
"9:00",
"PM",
"."
] | [
"O",
"O",
"O",
"O",
"O",
"O",
"O",
"O",
"O",
"O",
"O",
"O",
"O",
"B-TIME",
"I-TIME",
"O",
"O",
"O",
"O",
"O",
"O",
"O",
"O",
"O",
"O",
"O",
"O",
"O",
"O",
"O",
"O",
"O",
"O",
"O",
"B-TIME",
"I-TIME",
"O"
] |
train | [
"She",
"is",
"thankful",
"that",
"her",
"son",
",",
"a",
"university",
"student",
",",
"and",
"her",
"daughter",
",",
"a",
"third",
"-",
"year",
"junior",
"high",
"school",
"student",
",",
"have",
"always",
"been",
"very",
"independent",
"and",
"self",
"-",
"reliant",
"."
] | [
"O",
"O",
"O",
"O",
"O",
"O",
"O",
"O",
"O",
"O",
"O",
"O",
"O",
"O",
"O",
"O",
"B-DATE",
"I-DATE",
"I-DATE",
"O",
"O",
"O",
"O",
"O",
"O",
"O",
"O",
"O",
"O",
"O",
"O",
"O",
"O",
"O"
] |
train | [
"Hiring",
"someone",
"to",
"help",
"with",
"housework",
",",
"Lee",
"is",
"a",
"model",
"for",
"the",
"children",
"when",
"it",
"comes",
"to",
"studying",
",",
"even",
"taking",
"them",
"to",
"the",
"library",
"on",
"breaks",
"to",
"conduct",
"research",
"."
] | [
"O",
"O",
"O",
"O",
"O",
"O",
"O",
"B-PERSON",
"O",
"O",
"O",
"O",
"O",
"O",
"O",
"O",
"O",
"O",
"O",
"O",
"O",
"O",
"O",
"O",
"O",
"O",
"O",
"O",
"O",
"O",
"O",
"O"
] |
train | [
"No",
"magic",
"bullet"
] | [
"O",
"O",
"O"
] |
train | [
"Compared",
"to",
"young",
"students",
"focused",
"on",
"getting",
"a",
"degree",
",",
"the",
"motivations",
"of",
"professionals",
"studying",
"for",
"advanced",
"degrees",
"\"",
"are",
"not",
"that",
"simple",
".",
"\""
] | [
"O",
"O",
"O",
"O",
"O",
"O",
"O",
"O",
"O",
"O",
"O",
"O",
"O",
"O",
"O",
"O",
"O",
"O",
"O",
"O",
"O",
"O",
"O",
"O",
"O"
] |
train | [
"They",
"might",
"be",
"after",
"new",
"knowledge",
",",
"keen",
"to",
"sport",
"a",
"fancy",
"degree",
"from",
"a",
"graduate",
"school",
"like",
"NTU",
"or",
"Chengchi",
"University",
",",
"out",
"to",
"network",
"with",
"others",
",",
"or",
"looking",
"out",
"for",
"the",
"survival",
"of",
"business",
"and",
"to",
"raise",
"competitiveness",
"by",
"searching",
"for",
"answers",
"to",
"difficult",
"work",
"or",
"business",
"issues",
"."
] | [
"O",
"O",
"O",
"O",
"O",
"O",
"O",
"O",
"O",
"O",
"O",
"O",
"O",
"O",
"O",
"O",
"O",
"O",
"B-ORG",
"O",
"B-ORG",
"I-ORG",
"O",
"O",
"O",
"O",
"O",
"O",
"O",
"O",
"O",
"O",
"O",
"O",
"O",
"O",
"O",
"O",
"O",
"O",
"O",
"O",
"O",
"O",
"O",
"O",
"O",
"O",
"O",
"O",
"O",
"O"
] |
train | [
"\"",
"Looking",
"for",
"quick",
"answers",
"from",
"professors",
"is",
"a",
"misguided",
"concept",
",",
"\"",
"says",
"Tang",
"Ming",
"-",
"je",
",",
"professor",
"of",
"strategic",
"management",
"at",
"NTU",
"'s",
"Department",
"of",
"International",
"Business",
"."
] | [
"O",
"O",
"O",
"O",
"O",
"O",
"O",
"O",
"O",
"O",
"O",
"O",
"O",
"O",
"B-PERSON",
"I-PERSON",
"I-PERSON",
"I-PERSON",
"O",
"O",
"O",
"O",
"O",
"O",
"B-ORG",
"O",
"B-ORG",
"I-ORG",
"I-ORG",
"I-ORG",
"O"
] |
train | [
"Before",
"commencing",
"a",
"course",
",",
"Tang",
"tells",
"his",
"students",
",",
"\"",
"I",
"am",
"not",
"in",
"the",
"consulting",
"business",
"."
] | [
"O",
"O",
"O",
"O",
"O",
"B-PERSON",
"O",
"O",
"O",
"O",
"O",
"O",
"O",
"O",
"O",
"O",
"O",
"O",
"O"
] |
train | [
"There",
"are",
"no",
"magic",
"bullets",
",",
"for",
"if",
"I",
"had",
"any",
"I",
"'d",
"have",
"gotten",
"rich",
"long",
"ago",
".",
"\""
] | [
"O",
"O",
"O",
"O",
"O",
"O",
"O",
"O",
"O",
"O",
"O",
"O",
"O",
"O",
"O",
"O",
"O",
"O",
"O",
"O"
] |
train | [
"Wu",
"Se",
"-",
"hwa",
",",
"dean",
"of",
"National",
"Chengchi",
"University",
"'s",
"College",
"of",
"Commerce",
",",
"relates",
",",
"\"",
"Academic",
"institutions",
"provide",
"systematic",
"thinking",
",",
"not",
"the",
"answers",
"to",
"specific",
"questions",
".",
"\""
] | [
"B-PERSON",
"I-PERSON",
"I-PERSON",
"I-PERSON",
"O",
"O",
"O",
"B-ORG",
"I-ORG",
"I-ORG",
"I-ORG",
"B-ORG",
"I-ORG",
"I-ORG",
"O",
"O",
"O",
"O",
"O",
"O",
"O",
"O",
"O",
"O",
"O",
"O",
"O",
"O",
"O",
"O",
"O",
"O"
] |
train | [
"Wu",
"tells",
"his",
"students",
"that",
"if",
"they",
"put",
"other",
"things",
"aside",
"and",
"concentrate",
"on",
"their",
"studies",
"for",
"three",
"weeks",
",",
"maybe",
"they",
"'ll",
"find",
"the",
"answer",
"themselves",
"."
] | [
"B-PERSON",
"O",
"O",
"O",
"O",
"O",
"O",
"O",
"O",
"O",
"O",
"O",
"O",
"O",
"O",
"O",
"O",
"B-DATE",
"I-DATE",
"O",
"O",
"O",
"O",
"O",
"O",
"O",
"O",
"O"
] |
train | [
"Wu",
"notes",
"that",
"adult",
"students",
"bring",
"rich",
"practical",
"experience",
"to",
"the",
"table",
",",
"and",
"lack",
"only",
"the",
"knowledge",
"to",
"combine",
"and",
"integrate",
"scattered",
"experience",
"."
] | [
"B-PERSON",
"O",
"O",
"O",
"O",
"O",
"O",
"O",
"O",
"O",
"O",
"O",
"O",
"O",
"O",
"O",
"O",
"O",
"O",
"O",
"O",
"O",
"O",
"O",
"O"
] |
train | [
"For",
"them",
",",
"returning",
"to",
"school",
"is",
"valuable",
"for",
"establishing",
"their",
"own",
"modes",
"of",
"thinking",
"with",
"the",
"knowledge",
"they",
"pick",
"up",
"."
] | [
"O",
"O",
"O",
"O",
"O",
"O",
"O",
"O",
"O",
"O",
"O",
"O",
"O",
"O",
"O",
"O",
"O",
"O",
"O",
"O",
"O",
"O"
] |
train | [
"Unlike",
"the",
"20th",
"century",
",",
"where",
"the",
"amount",
"of",
"capital",
"was",
"the",
"greatest",
"factor",
"determining",
"success",
",",
"Wu",
"Se",
"-",
"hwa",
"believes",
"that",
"the",
"next",
"century",
"will",
"be",
"about",
"\"",
"knowledge",
"as",
"the",
"main",
"competitive",
"force",
".",
"\""
] | [
"O",
"B-DATE",
"I-DATE",
"I-DATE",
"O",
"O",
"O",
"O",
"O",
"O",
"O",
"O",
"O",
"O",
"O",
"O",
"O",
"B-PERSON",
"I-PERSON",
"I-PERSON",
"I-PERSON",
"O",
"O",
"B-DATE",
"I-DATE",
"I-DATE",
"O",
"O",
"O",
"O",
"O",
"O",
"O",
"O",
"O",
"O",
"O",
"O"
] |
train | [
"The",
"book",
"The",
"500",
"-",
"year",
"Delta",
"notes",
"that",
"in",
"the",
"1960s",
"each",
"executive",
"might",
"only",
"have",
"to",
"learn",
"one",
"new",
"skill",
"each",
"year",
",",
"such",
"as",
"how",
"to",
"use",
"a",
"computer",
"or",
"send",
"e-mail",
"."
] | [
"O",
"O",
"B-WORK_OF_ART",
"I-WORK_OF_ART",
"I-WORK_OF_ART",
"I-WORK_OF_ART",
"I-WORK_OF_ART",
"O",
"O",
"O",
"B-DATE",
"I-DATE",
"O",
"O",
"O",
"O",
"O",
"O",
"O",
"B-CARDINAL",
"O",
"O",
"O",
"O",
"O",
"O",
"O",
"O",
"O",
"O",
"O",
"O",
"O",
"O",
"O",
"O"
] |
train | [
"By",
"the",
"1990s",
",",
"accumulation",
"of",
"know",
"-",
"how",
"accelerated",
"many",
"times",
"over",
",",
"so",
"that",
"executives",
"needed",
"to",
"learn",
"something",
"new",
"each",
"day",
"."
] | [
"O",
"B-DATE",
"I-DATE",
"O",
"O",
"O",
"O",
"O",
"O",
"O",
"O",
"O",
"O",
"O",
"O",
"O",
"O",
"O",
"O",
"O",
"O",
"O",
"O",
"O",
"O"
] |
train | [
"Now",
",",
"in",
"the",
"21st",
"century",
",",
"each",
"manager",
"might",
"have",
"to",
"learn",
"something",
"new",
"every",
"single",
"hour",
"of",
"the",
"working",
"day",
"."
] | [
"O",
"O",
"O",
"B-DATE",
"I-DATE",
"I-DATE",
"O",
"O",
"O",
"O",
"O",
"O",
"O",
"O",
"O",
"B-TIME",
"I-TIME",
"I-TIME",
"I-TIME",
"I-TIME",
"I-TIME",
"I-TIME",
"O"
] |
train | [
"Curriculums",
"need",
"selling",
"points"
] | [
"O",
"O",
"O",
"O"
] |
train | [
"In",
"order",
"to",
"satisfy",
"students",
"with",
"diverse",
"expectations",
"and",
"needs",
"for",
"basic",
"knowledge",
",",
"what",
"innovative",
"means",
"must",
"a",
"professor",
"resort",
"to",
"?"
] | [
"O",
"O",
"O",
"O",
"O",
"O",
"O",
"O",
"O",
"O",
"O",
"O",
"O",
"O",
"O",
"O",
"O",
"O",
"O",
"O",
"O",
"O",
"O"
] |
train | [
"And",
"what",
"challenges",
"does",
"this",
"pose",
"for",
"the",
"institution",
"itself",
"?"
] | [
"O",
"O",
"O",
"O",
"O",
"O",
"O",
"O",
"O",
"O",
"O"
] |
train | [
"People",
"are",
"going",
"to",
"fall",
"asleep",
"no",
"matter",
"what",
",",
"so",
"you",
"have",
"to",
"think",
"on",
"your",
"feet",
"and",
"use",
"different",
"techniques",
"to",
"stimulate",
"their",
"attention",
",",
"\"",
"remarks",
"Mark",
"Gia",
"-",
"khy",
"Tang",
",",
"professor",
"in",
"the",
"Department",
"of",
"Risk",
"Management",
"and",
"Insurance",
"at",
"National",
"Chengchi",
"University",
"."
] | [
"O",
"O",
"O",
"O",
"O",
"O",
"O",
"O",
"O",
"O",
"O",
"O",
"O",
"O",
"O",
"O",
"O",
"O",
"O",
"O",
"O",
"O",
"O",
"O",
"O",
"O",
"O",
"O",
"O",
"B-PERSON",
"I-PERSON",
"I-PERSON",
"I-PERSON",
"I-PERSON",
"O",
"O",
"O",
"B-ORG",
"I-ORG",
"I-ORG",
"I-ORG",
"I-ORG",
"I-ORG",
"I-ORG",
"O",
"B-ORG",
"I-ORG",
"I-ORG",
"O"
] |
π CoNLL 2025 NER Dataset β Unlocking Entity Recognition in Text
Extract the Building Blocks of Meaning π
The CoNLL 2025 NER Dataset is a powerful collection of 143,709 entries designed for Named Entity Recognition (NER). With tokenized text and 36 expertly annotated NER tags (e.g., ποΈ DATE, πΈ MONEY, π’ ORG), this dataset enables AI to identify entities in text for applications like knowledge graphs π, intelligent search π, and automated content analysis π.
This 6.38 MB dataset is lightweight, developer-friendly, and ideal for advancing natural language processing (NLP), information extraction, and text mining. Whether you're building chatbots π€, analyzing news articles π°, or structuring data for AI π οΈ, this dataset is your key to unlocking structured insights from text.
Download Now π
Table of Contents π
- What is NER? β
- Why CoNLL 2025 NER Dataset? π
- Dataset Snapshot π
- Key Features β¨
- NER Tags & Purposes π·οΈ
- Installation π οΈ
- Download Instructions π₯
- Quickstart: Dive In π
- Data Structure π
- Use Cases π
- Preprocessing Guide π§
- Visualizing NER Tags π
- Comparison to Other Datasets βοΈ
- Source π±
- Tags π·οΈ
- License π
- Credits π
- Community & Support π
- Last Updated π
What is NER? β
Named Entity Recognition (NER) is a core NLP task that identifies and classifies named entities in text into categories like people π€, organizations π’, locations π, dates ποΈ, and more. For example:
- Sentence: "Microsoft opened a store in Tokyo on January 2025."
- NER Output:
- Microsoft β π’ ORG
- Tokyo β π GPE
- January 2025 β ποΈ DATE
NER powers applications by extracting structured data from unstructured text, enabling smarter search, content analysis, and knowledge extraction.
Why CoNLL 2025 NER Dataset? π
- Rich Entity Coverage π·οΈ: 36 NER tags capturing entities like ποΈ DATE, πΈ MONEY, and π€ PERSON.
- Compact & Scalable β‘: Only 6.38 MB, ideal for edge devices and large-scale NLP projects.
- Real-World Impact π: Drives AI for search systems, knowledge graphs, and automated analysis.
- Developer-Friendly π§βπ»: Integrates with Python π, Hugging Face π€, and NLP frameworks like spaCy and transformers.
βThe CoNLL 2025 NER Dataset transformed our text analysis pipeline!β β Data Scientist π¬
Dataset Snapshot π
Metric | Value |
---|---|
Total Entries | 143,709 |
Columns | 3 (split, tokens, ner_tags) |
Missing Values | 0 |
File Size | 6.38 MB |
Splits | Train (size TBD) |
Unique Tokens | To be calculated |
NER Tag Types | 36 (B-/I- tags + O) |
Note: Exact split sizes and token counts require dataset analysis.
Key Features β¨
- Diverse NER Tags π·οΈ: Covers 18 entity types with B- (beginning) and I- (inside) tags, plus O for non-entities.
- Lightweight Design πΎ: 6.38 MB Parquet file fits anywhere, from IoT devices to cloud servers.
- Versatile Applications π: Supports NLP tasks like entity extraction, text annotation, and knowledge base creation.
- High-Quality Annotations π: Expert-curated tags ensure precision for production-grade AI.
NER Tags & Purposes π·οΈ
The dataset uses the BIO tagging scheme:
- B- (Beginning): Marks the start of an entity.
- I- (Inside): Marks continuation of an entity.
- O: Non-entity token.
Below is a table of the 36 NER tags with their purposes and emojis for visual appeal:
Tag Name | Purpose | Emoji |
---|---|---|
B-CARDINAL | Beginning of a cardinal number (e.g., "1000") | π’ |
B-DATE | Beginning of a date (e.g., "January") | ποΈ |
B-EVENT | Beginning of an event (e.g., "Olympics") | π |
B-FAC | Beginning of a facility (e.g., "Eiffel Tower") | ποΈ |
B-GPE | Beginning of a geopolitical entity (e.g., "Tokyo") | π |
B-LANGUAGE | Beginning of a language (e.g., "Spanish") | π£οΈ |
B-LAW | Beginning of a law or legal document (e.g., "Constitution") | π |
B-LOC | Beginning of a non-GPE location (e.g., "Pacific Ocean") | πΊοΈ |
B-MONEY | Beginning of a monetary value (e.g., "$100") | πΈ |
B-NORP | Beginning of a nationality/religious/political group (e.g., "Democrat") | π³οΈ |
B-ORDINAL | Beginning of an ordinal number (e.g., "first") | π₯ |
B-ORG | Beginning of an organization (e.g., "Microsoft") | π’ |
B-PERCENT | Beginning of a percentage (e.g., "50%") | π |
B-PERSON | Beginning of a personβs name (e.g., "Elon Musk") | π€ |
B-PRODUCT | Beginning of a product (e.g., "iPhone") | π± |
B-QUANTITY | Beginning of a quantity (e.g., "two liters") | βοΈ |
B-TIME | Beginning of a time (e.g., "noon") | β° |
B-WORK_OF_ART | Beginning of a work of art (e.g., "Mona Lisa") | π¨ |
I-CARDINAL | Inside of a cardinal number (e.g., "000" in "1000") | π’ |
I-DATE | Inside of a date (e.g., "2025" in "January 2025") | ποΈ |
I-EVENT | Inside of an event name | π |
I-FAC | Inside of a facility name | ποΈ |
I-GPE | Inside of a geopolitical entity | π |
I-LANGUAGE | Inside of a language name | π£οΈ |
I-LAW | Inside of a legal document title | π |
I-LOC | Inside of a location | πΊοΈ |
I-MONEY | Inside of a monetary value | πΈ |
I-NORP | Inside of a NORP entity | π³οΈ |
I-ORDINAL | Inside of an ordinal number | π₯ |
I-ORG | Inside of an organization name | π’ |
I-PERCENT | Inside of a percentage | π |
I-PERSON | Inside of a personβs name | π€ |
I-PRODUCT | Inside of a product name | π± |
I-QUANTITY | Inside of a quantity | βοΈ |
I-TIME | Inside of a time phrase | β° |
I-WORK_OF_ART | Inside of a work of art title | π¨ |
O | Outside of any named entity (e.g., "the", "is") | π« |
Example
For "Microsoft opened in Tokyo on January 2025"
:
- Tokens:
["Microsoft", "opened", "in", "Tokyo", "on", "January", "2025"]
- Tags:
[B-ORG, O, O, B-GPE, O, B-DATE, I-DATE]
Installation π οΈ
Install dependencies to work with the dataset:
pip install datasets pandas pyarrow
- Requirements π: Python 3.8+, ~6.38 MB storage.
- Optional π§: Add
transformers
,spaCy
, orflair
for advanced NER tasks.
Download Instructions π₯
Direct Download
- Grab the dataset from the Hugging Face repository π.
- Load it with pandas πΌ, Hugging Face
datasets
π€, or your preferred tool.
Quickstart: Dive In π
Jump into the dataset with this Python code:
import pandas as pd
from datasets import Dataset
# Load Parquet
df = pd.read_parquet("conll2025_ner.parquet")
# Convert to Hugging Face Dataset
dataset = Dataset.from_pandas(df)
# Preview first entry
print(dataset[0])
Sample Output π
{
"split": "train",
"tokens": ["Big", "Managers", "on", "Campus"],
"ner_tags": ["O", "O", "O", "O"]
}
Convert to CSV π
To convert to CSV:
import pandas as pd
# Load Parquet
df = pd.read_parquet("conll2025_ner.parquet")
# Save as CSV
df.to_csv("conll2025_ner.csv", index=False)
Data Structure π
Field | Type | Description |
---|---|---|
split | String | Dataset split (e.g., "train") |
tokens | List | Tokenized text (e.g., ["Big", "Managers", ...]) |
ner_tags | List | NER tags (e.g., ["O", "O", "O", "O"]) |
Example Entry
{
"split": "train",
"tokens": ["In", "recent", "years"],
"ner_tags": ["O", "B-DATE", "I-DATE"]
}
Use Cases π
The CoNLL 2025 NER Dataset unlocks a wide range of applications:
- Information Extraction π: Extract ποΈ dates, π€ people, or π’ organizations from news, reports, or social media.
- Intelligent Search Systems π: Enable entity-based search (e.g., "find articles mentioning Tokyo in 2025").
- Knowledge Graph Construction π: Link entities like π€ PERSON and π’ ORG to build structured knowledge bases.
- Chatbots & Virtual Assistants π€: Enhance context understanding by recognizing entities in user queries.
- Document Annotation π: Automate tagging of entities in legal π, medical π©Ί, or financial πΈ documents.
- News Analysis π°: Track mentions of π GPEs or π EVENTs in real-time news feeds.
- E-commerce Personalization π: Identify π± PRODUCT or βοΈ QUANTITY in customer reviews for better recommendations.
- Fraud Detection π΅οΈ: Detect suspicious πΈ MONEY or π€ PERSON entities in financial transactions.
- Social Media Monitoring π±: Analyze π³οΈ NORP or π GPE mentions for trend detection.
- Academic Research π: Study entity distributions in historical texts or corpora.
- Geospatial Analysis πΊοΈ: Map π GPE and πΊοΈ LOC entities for location-based insights.
Preprocessing Guide π§
Prepare the dataset for your NER project:
Load the Data π:
import pandas as pd df = pd.read_parquet("conll2025_ner.parquet")
Filter by Split π:
train_data = df[df["split"] == "train"]
Validate BIO Tags π·οΈ:
def validate_bio(tags): valid_tags = set([ "O", "B-CARDINAL", "I-CARDINAL", "B-DATE", "I-DATE", "B-EVENT", "I-EVENT", "B-FAC", "I-FAC", "B-GPE", "I-GPE", "B-LANGUAGE", "I-LANGUAGE", "B-LAW", "I-LAW", "B-LOC", "I-LOC", "B-MONEY", "I-MONEY", "B-NORP", "I-NORP", "B-ORDINAL", "I-ORDINAL", "B-ORG", "I-ORG", "B-PERCENT", "I-PERCENT", "B-PERSON", "I-PERSON", "B-PRODUCT", "I-PRODUCT", "B-QUANTITY", "I-QUANTITY", "B-TIME", "I-TIME", "B-WORK_OF_ART", "I-WORK_OF_ART" ]) return all(tag in valid_tags for tag in tags) df["valid_bio"] = df["ner_tags"].apply(validate_bio)
Encode Tags for Training π’:
from sklearn.preprocessing import LabelEncoder all_tags = [tag for tags in df["ner_tags"] for tag in tags] le = LabelEncoder() encoded_tags = le.fit_transform(all_tags)
Save Processed Data πΎ:
df.to_parquet("preprocessed_conll2025_ner.parquet")
Tokenize further with transformers
π€ or NeuroNER
for model training.
Visualizing NER Tags π
Visualize the NER tag distribution to understand entity prevalence. Since exact counts are unavailable, the chart below uses estimated data for demonstration. Replace with actual counts after analysis.
To compute actual counts:
import pandas as pd
from collections import Counter
import matplotlib.pyplot as plt
# Load dataset
df = pd.read_parquet("conll2025_ner.parquet")
# Flatten ner_tags
all_tags = [tag for tags in df["ner_tags"] for tag in tags]
tag_counts = Counter(all_tags)
# Plot
plt.figure(figsize=(12, 7))
plt.bar(tag_counts.keys(), tag_counts.values(), color="#36A2EB")
plt.title("CoNLL 2025 NER: Tag Distribution")
plt.xlabel("NER Tag")
plt.ylabel("Count")
plt.xticks(rotation=45, ha="right")
plt.grid(axis="y", linestyle="--", alpha=0.7)
plt.tight_layout()
plt.savefig("ner_tag_distribution.png")
Comparison to Other Datasets βοΈ
Dataset | Entries | Size | Focus | Tasks Supported |
---|---|---|---|---|
CoNLL 2025 NER | 143,709 | 6.38 MB | Comprehensive NER (18 entity types) | NER, NLP |
CoNLL 2003 | ~20K | ~5 MB | NER (PERSON, ORG, LOC, MISC) | NER |
OntoNotes 5.0 | ~1.7M | ~200 MB | NER, coreference, POS | NER, Coreference, POS Tagging |
WikiANN | ~40K | ~10 MB | Multilingual NER | NER |
The CoNLL 2025 NER Dataset excels with its broad entity coverage, compact size, and modern annotations, making it suitable for both research and production.
Source π±
- Text Sources π: Curated from diverse texts, including user-generated content, news, and research corpora.
- Annotations π·οΈ: Expert-labeled for high accuracy and consistency.
- Mission π―: To advance NLP by providing a robust dataset for entity recognition.
Tags π·οΈ
#CoNLL2025NER
#NamedEntityRecognition
#NER
#NLP
#MachineLearning
#DataScience
#ArtificialIntelligence
#TextAnalysis
#InformationExtraction
#DeepLearning
#AIResearch
#TextMining
#KnowledgeGraphs
#AIInnovation
#NaturalLanguageProcessing
#BigData
#AIForGood
#Dataset2025
License π
MIT License: Free to use, modify, and distribute. See LICENSE. π³οΈ
Credits π
- Curated By: boltuix π¨βπ»
- Sources: Open datasets, research contributions, and community efforts π
- Powered By: Hugging Face
datasets
π€
Community & Support π
Join the NER community:
- π Explore the Hugging Face dataset page π
- π οΈ Report issues or contribute at the repository π§
- π¬ Discuss on Hugging Face forums or submit pull requests π£οΈ
- π Learn more via Hugging Face Datasets docs π
Your feedback shapes the CoNLL 2025 NER Dataset! π
Last Updated π
May 28, 2025 β Released with 36 NER tags, enhanced use cases, and visualizations.
- Downloads last month
- 439
Models trained or fine-tuned on boltuix/conll2025-ner
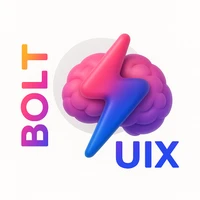