id
stringlengths 6
113
| author
stringlengths 2
36
| task_category
stringclasses 39
values | tags
sequencelengths 1
4.05k
| created_time
int64 1,646B
1,742B
| last_modified
timestamp[s]date 2020-05-14 13:13:12
2025-03-18 10:01:09
| downloads
int64 0
118M
| likes
int64 0
4.86k
| README
stringlengths 30
1.01M
| matched_task
sequencelengths 1
10
| is_bionlp
stringclasses 3
values |
---|---|---|---|---|---|---|---|---|---|---|
LoneStriker/nontoxic-bagel-34b-v0.2-8.0bpw-h8-exl2 | LoneStriker | text-generation | [
"transformers",
"safetensors",
"llama",
"text-generation",
"dataset:ai2_arc",
"dataset:unalignment/spicy-3.1",
"dataset:codeparrot/apps",
"dataset:facebook/belebele",
"dataset:boolq",
"dataset:jondurbin/cinematika-v0.1",
"dataset:drop",
"dataset:lmsys/lmsys-chat-1m",
"dataset:TIGER-Lab/MathInstruct",
"dataset:cais/mmlu",
"dataset:Muennighoff/natural-instructions",
"dataset:openbookqa",
"dataset:piqa",
"dataset:Vezora/Tested-22k-Python-Alpaca",
"dataset:cakiki/rosetta-code",
"dataset:Open-Orca/SlimOrca",
"dataset:spider",
"dataset:squad_v2",
"dataset:migtissera/Synthia-v1.3",
"dataset:datasets/winogrande",
"dataset:nvidia/HelpSteer",
"dataset:Intel/orca_dpo_pairs",
"dataset:unalignment/toxic-dpo-v0.1",
"dataset:jondurbin/truthy-dpo-v0.1",
"dataset:allenai/ultrafeedback_binarized_cleaned",
"dataset:Squish42/bluemoon-fandom-1-1-rp-cleaned",
"dataset:LDJnr/Capybara",
"dataset:JULIELab/EmoBank",
"dataset:kingbri/PIPPA-shareGPT",
"license:other",
"autotrain_compatible",
"text-generation-inference",
"endpoints_compatible",
"region:us"
] | 1,704,199,070,000 | 2024-01-02T13:01:21 | 4 | 0 | ---
datasets:
- ai2_arc
- unalignment/spicy-3.1
- codeparrot/apps
- facebook/belebele
- boolq
- jondurbin/cinematika-v0.1
- drop
- lmsys/lmsys-chat-1m
- TIGER-Lab/MathInstruct
- cais/mmlu
- Muennighoff/natural-instructions
- openbookqa
- piqa
- Vezora/Tested-22k-Python-Alpaca
- cakiki/rosetta-code
- Open-Orca/SlimOrca
- spider
- squad_v2
- migtissera/Synthia-v1.3
- datasets/winogrande
- nvidia/HelpSteer
- Intel/orca_dpo_pairs
- unalignment/toxic-dpo-v0.1
- jondurbin/truthy-dpo-v0.1
- allenai/ultrafeedback_binarized_cleaned
- Squish42/bluemoon-fandom-1-1-rp-cleaned
- LDJnr/Capybara
- JULIELab/EmoBank
- kingbri/PIPPA-shareGPT
license: other
license_name: yi-license
license_link: https://huggingface.co/01-ai/Yi-34B-200K/blob/main/LICENSE
---
# A bagel, with everything

## Overview
An experimental fine-tune of [yi-34b-200k](https://huggingface.co/01-ai/Yi-34B-200K) using [bagel](https://github.com/jondurbin/bagel)
This version underwent a subset of DPO, but is fairly censored. For a less censored version, try [bagel-dpo-34b-v0.2](https://hf.co/jondurbin/bagel-dpo-34b-v0.2)
## SFT data sources
*Yes, you will see benchmark names in the list, but this only uses the train splits, and a decontamination by cosine similarity is performed at the end as a sanity check*
- [ai2_arc](https://huggingface.co/datasets/ai2_arc)
- Abstraction and reasoning dataset, useful in measuring "intelligence" to a certain extent.
- [airoboros](https://huggingface.co/datasets/unalignment/spicy-3.1)
- Variety of categories of synthetic instructions generated by gpt-4.
- [apps](https://huggingface.co/datasets/codeparrot/apps)
- Python coding dataset with 10k problems.
- [belebele](https://huggingface.co/datasets/facebook/belebele)
- Multi-lingual reading comprehension dataset.
- [bluemoon](https://huggingface.co/datasets/Squish42/bluemoon-fandom-1-1-rp-cleaned)
- Roleplay data scraped from Bluemoon, then cleaned and formatted as ShareGPT.
- [boolq](https://huggingface.co/datasets/boolq)
- Corpus of yes/no questions (which can be surprisingly difficult for AI to answer apparently?)
- [capybara](https://huggingface.co/datasets/LDJnr/Capybara)
- Multi-turn dataset used to create the capybara models.
- [cinematika](https://huggingface.co/datasets/jondurbin/cinematika-v0.1) (instruction and plain text)
- RP-style data synthesized from movie scripts so the model isn't quite as boring as it otherwise would be.
- [drop](https://huggingface.co/datasets/drop)
- More reading comprehension.
- [emobank](https://github.com/JULIELab/EmoBank)
- Emotion annotations using the Valence-Arousal-Domninance scheme.
- [gutenberg](https://www.gutenberg.org/) (plain text)
- Books/plain text, again to make the model less boring, only a handful of examples supported by [chapterize](https://github.com/JonathanReeve/chapterize)
- [lmsys_chat_1m](https://huggingface.co/datasets/lmsys/lmsys-chat-1m) (only gpt-4 items, also used for DPO)
- Chats collected by the lmsys chat arena, containing a wide variety of chats with various models.
- [mathinstruct](https://huggingface.co/datasets/TIGER-Lab/MathInstruct)
- Composite dataset with a variety of math-related tasks and problem/question formats.
- [mmlu](https://huggingface.co/datasets/cais/mmlu)
- Massive Multitask Language Understanding - a wide variety of questions about various subject matters.
- [natural_instructions](https://huggingface.co/datasets/Muennighoff/natural-instructions)
- Millions of instructions from 1600+ task categories (sampled down substantially, stratified by task type)
- [openbookqa](https://huggingface.co/datasets/openbookqa)
- Question answering dataset.
- [pippa](https://huggingface.co/datasets/kingbri/PIPPA-shareGPT)
- Deduped version of [PIPPA](https://huggingface.co/datasets/PygmalionAI/PIPPA) in ShareGPT format.
- [piqa](https://huggingface.co/datasets/piqa)
- Phyiscal interaction question answering.
- [python_alpaca](https://huggingface.co/datasets/Vezora/Tested-22k-Python-Alpaca)
- Python instruction response pairs, validated as functional.
- [rosetta_code](https://huggingface.co/datasets/cakiki/rosetta-code)
- Code problems and solutions in a variety of programming languages taken from rosettacode.org.
- [slimorca](https://huggingface.co/datasets/Open-Orca/SlimOrca)
- Collection of ~500k gpt-4 verified chats from OpenOrca.
- [spider](https://huggingface.co/datasets/spider)
- SQL-targeted dataset.
- [squad_v2](https://huggingface.co/datasets/squad_v2)
- Contextual question answering (RAG).
- [synthia](https://huggingface.co/datasets/migtissera/Synthia-v1.3)
- GPT-4 generated data using advanced prompting from Migel Tissera.
- [winogrande](https://huggingface.co/datasets/winogrande)
- Fill in the blank style prompts.
## DPO data sources
- [airoboros 3.1](https://huggingface.co/datasets/unalignment/spicy-3.1) vs [airoboros 2.2.1](https://huggingface.co/datasets/jondurbin/airoboros-gpt4-1.4.1)
- The creative/writing tasks from airoboros-2.2.1 were re-generated using gpt4-0314 and a custom prompt to get longer, more creative, less clichè responses for airoboros 3.1, so we can use the shorter/boring version as the "rejected" value and the rerolled response as "chosen"
- [helpsteer](https://huggingface.co/datasets/nvidia/HelpSteer)
- Really neat dataset provided by the folks at NVidia with human annotation across a variety of metrics. Only items with the highest "correctness" value were used for DPO here, with the highest scoring output as "chosen" and random lower scoring value as "rejected"
- [orca_dpo_pairs](https://huggingface.co/datasets/Intel/orca_dpo_pairs)
- Another interesting dataset by Intel, which provides various DPO pairs generated from prompts included in the SlimOrca dataset.
- [toxic-dpo](https://huggingface.co/datasets/unalignment/toxic-dpo-v0.1)
- __*highly toxic and potentially illegal content!*__ De-censorship, for academic and lawful purposes only, of course. Generated by llama-2-70b via prompt engineering.
- [truthy](https://huggingface.co/datasets/jondurbin/truthy-dpo-v0.1)
- DPO pairs meant to increase truthfulness of the model, e.g. common misconceptions, differentiate between AI assistants and roleplayed human in terms of corporeal awareness/locality/etc.
- [ultrafeedback](https://huggingface.co/datasets/allenai/ultrafeedback_binarized_cleaned)
- One of the bits of magic behind the Zephyr model. Only the items with a chosen score of 8 or higher were included.
Only the train splits were used (if a split was provided), and an additional pass of decontamination is performed using approximate nearest neighbor search (via faiss).
## Prompt formatting
In sticking with the theme of the bagel, I didn't want to use a single prompt format, so I used 4 - vicuna, llama-2, alpaca, and chat-ml (sorta).
I also didn't want to randomly select a single prompt format for each item (hoping each instruction would generalize more when used in a variety of prompt formats), so each instruction is actually converted into every prompt format.
This means each epoch of our fine-tune is really basically 4 epochs. So, for the fine-tunes, I would recommend only doing 1 epoch (or 0.75 epochs). I am testing with a single epoch using a relatively low learning rate.
### Alpaca (sort of)
```
Below is an instruction that describes a task. Write a response that appropriately completes the request.
### Instruction:
{system prompt, if provided}
{instruction}
### Response:
```
The main difference here is that because of the dataset formatting and variety of data sources, it would have been much to tedious to add an `### Input:` block, so the inputs are just in the instruction section.
### Vicuna
```
{system prompt, if provided, randomly defaulting to "A chat between a user and an unbiased, uncensored assistant."}
USER: {instruction}
ASSISTANT:
```
### ChatML (sort of)
I don't really understand the point of having special tokens for `<|im_start|>` and `<|im_end|>`, because in practice they just act as BOS and EOS tokens (but, please correct me if I'm wrong).
So, instead of:
```text
{bos}<|im_start|>{role}
{text}
<|im_end|>{eos}
```
I just changed it to:
```text
{bos}{role}
{text}
{eos}
```
If you *really* want to use `<|im_start|>` and `<|im_end|>`, just update your `tokenizer_config.json` to use `<|im_start|>` instead of `<s>` and `<|im_end|>` instead of `</s>` and when tokenizing. And if you still don't like what I've done to this chat-ml-ish format, feel free to cry into your pillow or fork the code and do a new fine-tune.
### Llama-2 chat
```
[INST] <<SYS>>
{system}
<</SYS>>
{instruction} [/INST]
``` | [
"QUESTION_ANSWERING"
] | Non_BioNLP |
NorGLM/NorGPT-3B-continue-summarization-peft | NorGLM | summarization | [
"summarization",
"no",
"dataset:NorGLM/NO-CNN-DailyMail",
"license:cc-by-nc-sa-4.0",
"region:us"
] | 1,710,147,858,000 | 2024-03-11T09:07:15 | 0 | 0 | ---
datasets:
- NorGLM/NO-CNN-DailyMail
language:
- 'no'
license: cc-by-nc-sa-4.0
pipeline_tag: summarization
---
# Model Card
NorGPT-3B-continue-summarization-peft is trained on top of [NorGPT-3B-continue](https://huggingface.co/NorGLM/NorGPT-3B-continue) model on [NO-CNN-DailyMail](https://huggingface.co/datasets/NorGLM/NO-CNN-DailyMail) dataset.
Prompt format:
```
Summarise the article:\\n{article} |||\\n{positive_sample}
```
Inference prompt:
```
Summarise the article:\\n{article} |||\\n
```
## Run the Model
```python
from peft import PeftModel, PeftConfig
from transformers import AutoModelForCausalLM, AutoTokenizer
import torch
source_model_id = "NorGLM/NorGPT-3B-continue"
peft_model_id = "NorGLM/NorGPT-3B-continue-summarization-peft"
config = PeftConfig.from_pretrained(peft_model_id)
model = AutoModelForCausalLM.from_pretrained(source_model_id, device_map='balanced')
tokenizer_max_len = 2048
tokenizer_config = {'pretrained_model_name_or_path': source_model_id,
'max_len': tokenizer_max_len}
tokenizer = tokenizer = AutoTokenizer.from_pretrained(**tokenizer_config)
tokenizer.pad_token = tokenizer.eos_token
model = PeftModel.from_pretrained(model, peft_model_id)
```
## Inference on test set
Load the model to evaluate on the test set of NO-CNN-DailyMail dataset:
```python
def generate_texts(model, tokenizer, prompts, max_seq_length=200, do_sample=True, top_p=0.95, top_k=10):
# prompts are a list of news articles
results = []
cnt = 0
for prompt in prompts:
cnt += 1
pro_len = len(prompt.split())
if pro_len>1024:
results.append('')
continue
prompt = 'Summarise the article:\\n' + prompt + ' |||\\n'
model_inputs = tokenizer(prompt, return_tensors='pt').to(torch_device)
output = model.generate(**model_inputs, do_sample=False, max_new_tokens=max_seq_length)
result = tokenizer.decode(output[0], skip_special_tokens=True)
result = result.split("|||\\n")[-1]
results.append(result)
return results
print("--LOADING EVAL DATAS---")
eval_data = load_dataset("NorGLM/NO-CNN-DailyMail", data_files="test.csv")
prompts = eval_data['train']['article']
positive_samples = eval_data['train']['positive_sample']
print("--MAKING PREDICTIONS---")
model.eval()
output_file = <output file name>
with torch.no_grad():
results = generate_texts(model, tokenizer, prompts)
df = pd.DataFrame({'article':prompts, 'generated_text':results, 'positive_sample':positive_samples})
print("Save results to csv file...")
df.to_csv(output_file)
```
## Note
More training details will be released soon! | [
"SUMMARIZATION"
] | Non_BioNLP |
pchelaEb/t5-russian-spell | pchelaEb | text2text-generation | [
"transformers",
"pytorch",
"tensorboard",
"t5",
"text2text-generation",
"generated_from_trainer",
"autotrain_compatible",
"text-generation-inference",
"endpoints_compatible",
"region:us"
] | 1,676,559,147,000 | 2023-02-18T10:34:42 | 36 | 0 | ---
tags:
- generated_from_trainer
model-index:
- name: t5-russian-spell
results: []
---
<!-- This model card has been generated automatically according to the information the Trainer had access to. You
should probably proofread and complete it, then remove this comment. -->
# t5-russian-spell
This model is a fine-tuned version of [UrukHan/t5-russian-summarization](https://huggingface.co/UrukHan/t5-russian-summarization) on the None dataset.
## Model description
More information needed
## Intended uses & limitations
More information needed
## Training and evaluation data
More information needed
## Training procedure
### Training hyperparameters
The following hyperparameters were used during training:
- learning_rate: 5e-05
- train_batch_size: 8
- eval_batch_size: 8
- seed: 42
- optimizer: Adam with betas=(0.9,0.999) and epsilon=1e-08
- lr_scheduler_type: linear
- num_epochs: 1
- mixed_precision_training: Native AMP
### Training results
### Framework versions
- Transformers 4.26.1
- Pytorch 1.13.1+cu116
- Datasets 2.9.0
- Tokenizers 0.13.2
| [
"SUMMARIZATION"
] | Non_BioNLP |
inceptionai/jais-family-30b-16k-chat | inceptionai | text-generation | [
"safetensors",
"jais",
"Arabic",
"English",
"LLM",
"Decoder",
"causal-lm",
"jais-family",
"text-generation",
"conversational",
"custom_code",
"ar",
"en",
"arxiv:2307.09288",
"arxiv:2308.16149",
"arxiv:2402.12840",
"base_model:inceptionai/jais-family-30b-16k",
"base_model:finetune:inceptionai/jais-family-30b-16k",
"license:apache-2.0",
"region:us"
] | 1,722,599,903,000 | 2024-09-11T11:07:50 | 76 | 11 | ---
base_model: inceptionai/jais-family-30b-16k
language:
- ar
- en
license: apache-2.0
pipeline_tag: text-generation
tags:
- Arabic
- English
- LLM
- Decoder
- causal-lm
- jais-family
---
# Jais Family Model Card
The Jais family of models is a comprehensive series of bilingual English-Arabic large language models (LLMs). These models are optimized to excel in Arabic while having strong English capabilities. We release two variants of foundation models that include:
- Models **pre-trained from scratch** (`jais-family-*`).
- Models **pre-trained adaptively from [Llama-2](https://arxiv.org/pdf/2307.09288)** (`jais-adapted-*`).
In this release, we introduce 20 models across 8 sizes, ranging from 590M to 70B parameters, trained on up to 1.6T tokens of Arabic, English, and code data. *All* pre-trained models in this series are instruction fine-tuned (`*-chat`) for dialog using a curated mix of Arabic and English instruction data.
We hope this extensive release will accelerate research in Arabic NLP, and enable numerous downstream applications for the Arabic speaking and bilingual community. The training and adaptation techniques we demonstrate successfully for Arabic models are extensible to other low and medium resource languages.
## Jais Family Details
- **Developed by:** Inception, Cerebras Systems.
- **Language(s):** (NLP): Arabic (MSA) and English.
- **Input:** Text only data.
- **Output:** Model generates text.
- **Model Sizes:** 590M, 1.3B, 2.7B, 6.7B, 7B, 13B, 30B, 70B.
- **Demo:** [Access the live demo here](https://arabic-gpt.ai/)
- **License:** Apache 2.0
| **Pre-trained Model** | **Fine-tuned Model** | **Size (Parameters)** | **Context length (Tokens)** |
|:---------------------|:--------|:-------|:-------|
| [jais-family-30b-16k](https://huggingface.co/inceptionai/jais-family-30b-16k) | [Jais-family-30b-16k-chat](https://huggingface.co/inceptionai/jais-family-30b-16k-chat) | 30B | 16,384 |
| [jais-family-30b-8k](https://huggingface.co/inceptionai/jais-family-30b-8k) | [Jais-family-30b-8k-chat](https://huggingface.co/inceptionai/jais-family-30b-8k-chat) | 30B | 8,192 |
| [jais-family-13b ](https://huggingface.co/inceptionai/jais-family-13b) | [Jais-family-13b-chat](https://huggingface.co/inceptionai/jais-family-13b-chat) | 13B | 2,048 |
| [jais-family-6p7b](https://huggingface.co/inceptionai/jais-family-6p7b) | [Jais-family-6p7b-chat](https://huggingface.co/inceptionai/jais-family-6p7b-chat) | 6.7B | 2,048 |
| [jais-family-2p7b](https://huggingface.co/inceptionai/jais-family-2p7b) | [Jais-family-2p7b-chat](https://huggingface.co/inceptionai/jais-family-2p7b-chat) | 2.7B | 2,048 |
| [jais-family-1p3b](https://huggingface.co/inceptionai/jais-family-1p3b) | [Jais-family-1p3b-chat](https://huggingface.co/inceptionai/jais-family-1p3b-chat) | 1.3B | 2,048 |
| [jais-family-590m](https://huggingface.co/inceptionai/jais-family-590m) | [Jais-family-590m-chat](https://huggingface.co/inceptionai/jais-family-590m-chat) | 590M | 2,048 |
| **Adapted pre-trained Model** | **Fine-tuned Model** | **Size (Parameters)** | **Context length (Tokens)** |
|:---------------------|:--------|:-------|:-------|
| [jais-adapted-70b](https://huggingface.co/inceptionai/jais-adapted-70b) | [Jais-adapted-70b-chat](https://huggingface.co/inceptionai/jais-adapted-70b-chat) | 70B | 4,096 |
| [jais-adapted-13b](https://huggingface.co/inceptionai/jais-adapted-13b) | [Jais-adapted-13b-chat](https://huggingface.co/inceptionai/jais-adapted-13b-chat) | 13B | 4,096 |
| [jais-adapted-7b](https://huggingface.co/inceptionai/jais-adapted-7b) | [Jais-adapted-7b-chat](https://huggingface.co/inceptionai/jais-adapted-7b-chat) | 7B | 4,096 |
### Model Architecture:
<a name="model-architecture"></a>
All models in this family are auto-regressive language models that use a transformer-based, decoder-only architecture (GPT-3).
Jais models (`jais-family-*`) are *trained from scratch*, incorporating the SwiGLU non-linear activation function and ALiBi position encoding. These architectural enhancements allow the models to extrapolate at long sequence lengths, leading to improved context handling and precision.
Jais adapted models (`jais-adapted-*`) are *built on top of Llama-2*, which employs RoPE position embedding and Grouped Query Attention. We introduce tokenizer expansion with Arabic data, which improves fertility and compute efficiency by over 3x. In particular, we add `32,000` new Arabic tokens from the Jais-30b vocabulary into the Llama-2 tokenizer.
To initialize these new Arabic token embeddings we first learn a linear projection from the embedding space of Jais-30b to Llama's embedding space, using the set of shared English tokens present in both vocabularies. Next, this learned projection is applied to transform the existing Jais-30b Arabic embeddings into the Llama-2 embedding space.
## Getting started
Below is sample code to use the model. Note that the model requires a custom model class, so users must enable `trust_remote_code=True` while loading the model.
```python
# -*- coding: utf-8 -*-
import torch
from transformers import AutoTokenizer, AutoModelForCausalLM
model_path = "inceptionai/jais-family-30b-16k-chat"
prompt_eng = "### Instruction:Your name is 'Jais', and you are named after Jebel Jais, the highest mountain in UAE. You were made by 'Inception' in the UAE. You are a helpful, respectful, and honest assistant. Always answer as helpfully as possible, while being safe. Complete the conversation between [|Human|] and [|AI|]:\n### Input: [|Human|] {Question}\n[|AI|]\n### Response :"
prompt_ar = "### Instruction:اسمك \"جيس\" وسميت على اسم جبل جيس اعلى جبل في الامارات. تم بنائك بواسطة Inception في الإمارات. أنت مساعد مفيد ومحترم وصادق. أجب دائمًا بأكبر قدر ممكن من المساعدة، مع الحفاظ على البقاء أمناً. أكمل المحادثة بين [|Human|] و[|AI|] :\n### Input:[|Human|] {Question}\n[|AI|]\n### Response :"
device = "cuda" if torch.cuda.is_available() else "cpu"
tokenizer = AutoTokenizer.from_pretrained(model_path)
model = AutoModelForCausalLM.from_pretrained(model_path, device_map="auto", trust_remote_code=True)
def get_response(text, tokenizer=tokenizer, model=model):
input_ids = tokenizer(text, return_tensors="pt").input_ids
inputs = input_ids.to(device)
input_len = inputs.shape[-1]
generate_ids = model.generate(
inputs,
top_p=0.9,
temperature=0.3,
max_length=2048,
min_length=input_len + 4,
repetition_penalty=1.2,
do_sample=True,
)
response = tokenizer.batch_decode(
generate_ids, skip_special_tokens=True, clean_up_tokenization_spaces=True
)[0]
response = response.split("### Response :")[-1]
return response
ques = "ما هي عاصمة الامارات؟"
text = prompt_ar.format_map({'Question': ques})
print(get_response(text))
ques = "What is the capital of UAE?"
text = prompt_eng.format_map({'Question': ques})
print(get_response(text))
```
## Training Details
### Pretraining Data
The Jais family of models are trained on up to 1.6 Trillion tokens of diverse English, Arabic and Code data. The data consists of the following sources:
- **Web:** We used publicly available web pages, wikipedia articles, news articles, and social network content in both Arabic and English.
- **Code:** To enhance the reasoning capability of our model, we include Code data in various programming languages.
- **Books:** We used a selection of publicly available Arabic and English books data, which improves long-range context modelling and coherent storytelling.
- **Scientific:** A subset of ArXiv papers were included to improve reasoning and long context abilities.
- **Synthetic:** We augment the volume of Arabic data by translating English to Arabic using an in-house machine translation system. We restrict this to high quality English resources such as English Wikipedia and English books.
We extensively preprocess and deduplicate the training data. For Arabic, we used a custom preprocessing pipeline to filter for data with high linguistic quality. More information on this pipeline can be found in the [Jais paper](https://arxiv.org/abs/2308.16149).
- **Jais pre-trained** (`jais-family-*`): Following our previous experimentation with language alignment mixing in [Jais](https://arxiv.org/abs/2308.16149), we used a ratio of 1:2:0.4 of Arabic:English:Code data. This recipe for <u>from scratch pre-training</u> addresses Arabic data scarcity while improving performance in both languages.
- **Jais adapted pre-trained** (`jais-adapted-*`): For the <u>adapted pre-training of Llama-2</u>, we utilized a larger Arabic dataset of ~334B Arabic tokens mixed with English and Code data. We vary the mixing ratio, at different model sizes, to introduce strong Arabic capabilities while maintaining performance in English.
| **Pre-trained model** | **English data (tokens)** | **Arabic data (tokens)** | **Code data (tokens)** | **Total data (tokens)** |
|-------------------------|---------------------------|--------------------------|------------------------|------------------------|
| [jais-family-30b-16k](https://huggingface.co/inceptionai/jais-family-30b-16k) | 980B | 490B | 196B | 1666B |
| [jais-family-30b-8k](https://huggingface.co/inceptionai/jais-family-30b-8k) | 882B | 441B | 177B | 1500B |
| [jais-family-13b ](https://huggingface.co/inceptionai/jais-family-13b) | 283B | 141B | 56B | 480B |
| [jais-family-6p7b](https://huggingface.co/inceptionai/jais-family-6p7b) | 283B | 141B | 56B | 480B |
| [jais-family-2p7b](https://huggingface.co/inceptionai/jais-family-2p7b) | 283B | 141B | 56B | 480B |
| [jais-family-1p3b](https://huggingface.co/inceptionai/jais-family-1p3b) | 283B | 141B | 56B | 480B |
| [jais-family-590m](https://huggingface.co/inceptionai/jais-family-590m) | 283B | 141B | 56B | 480B |
| [jais-adapted-70b](https://huggingface.co/inceptionai/jais-adapted-70b) | 33B | 334B | 4B | 371B |
| [jais-adapted-13b](https://huggingface.co/inceptionai/jais-adapted-13b) | 127B | 140B | 13B | 280B |
| [jais-adapted-7b](https://huggingface.co/inceptionai/jais-adapted-7b) | 18B | 19B | 2B | 39B |
### Finetuning data
<!-- This should link to a Data Card, perhaps with a short stub of information on what the training data is all about as well as documentation related to data pre-processing or additional filtering. -->
All chat models in the Jais family are fine-tuned using Arabic and English prompt-response pairs in both single-turn and multi-turn settings. Data sources include open-source fine-tuning datasets filtered for topic and style diversity. Additionally, internally curated human data is incorporated to enhance cultural adaptation. This data is supplemented with content generated using synthetic methods including machine translation, distillation, and model self-chat. Overall, our updated instruction-tuning dataset comprises ~10M and ~4M prompt-response pairs in English and Arabic respectively.
### Training Procedure
<!-- This relates heavily to the Technical Specifications. Content here should link to that section when it is relevant to the training procedure. -->
During the pre-training of (`jais-family-*`) models, documents are packed into sequences separated by EOS tokens, and the model is trained autoregressively, applying the loss to all tokens. For jais-30b models, the context length is progressively expanded from 2k to 8K to 16K by incorporating curated long-context documents in training. This progressive expansion leverages faster initial training at shorter context lengths, while gradually extending support for larger context lengths towards the end of the training process.
During the adapted pre-training of the (`jais-adapted-*`) models, we first initialize the new tokenizer and Arabic embeddings as described in [Model Architecture](#model-architecture). In training, we implemented a two-stage approach to overcome observed higher norms of the new Arabic embeddings. In the first stage, the backbone of the model is frozen, and the embeddings are trained using approximately 15 billion tokens from a bilingual corpus of English and Arabic. In the second stage, the backbone is unfrozen, and continuous pretraining is conducted with all parameters.
During instruction tuning, each training example consists of a single-turn or multi-turn prompt and it's response. Instead of one example per sequence, examples are packed together while the loss is masked on the prompt tokens. This approach speeds up training by allowing more examples to be processed per batch.
### Training Hyperparameters:
#### Jais-family-30b-16k-chat
| Hyperparameter | Value |
|----------------|-------------------------------------------|
| Precision | fp32 |
| Optimizer | AdamW |
| Learning rate | 0 to 0.0016(<=192 warmup steps)<br>0.0016 to 0.00016(>69 and <=11342 steps)|
| Weight decay | 0.1 |
| Batch size | 120|
| Context Length | 16384|
| Steps | 11342 |
### Compute Infrastructure
The training process was performed on the Condor Galaxy (CG) supercomputer platform. A CG contains 64 Cerebras CS-2 Wafer-Scale Engines (WSE-2) with 40 GB of SRAM, and achieves a total of 960 PetaFLOP/s.
## Evaluation
<!-- This section describes the evaluation protocols and provides the results. -->
We conducted a comprehensive evaluation of Jais models focusing on both English and Arabic, using LM-harness in a zero-shot setting. The evaluation criteria spanned various dimensions, including:
- **Knowledge:** How well the model answers factual questions.
- **Reasoning:** The model's ability to answer questions requiring reasoning.
- **Misinformation/Bias:** Assessment of the model's susceptibility to generating false or misleading information, and its neutrality.
### Arabic evaluation results:
<style>
.table-container {
overflow-x: auto;
white-space: nowrap;
}
</style>
<div class="table-container">
| **Models** | Avg | ArabicMMLU*| MMLU | EXAMS*| LitQA*| agqa | agrc | Hellaswag | PIQA | BoolQA | Situated QA | ARC-C | OpenBookQA | TruthfulQA | CrowS-Pairs |
|--------------------------|-------|------------|-------|-------|-------|------|------|------------|------|--------|-------------|-------|------------|------------|-------------|
| jais-family-30b-16k | 49.2 | 44.0 | 33.4 | 40.9 | 60 | 47.8 | 49.3 | 60.9 | 68.6 | 70.3 | 41.6 | 38.7 | 31.8 | 45.2 | 57 |
| jais-family-30b-8k | 49.7 | 46.0 | 34 | 42 | 60.6 | 47.6 | 50.4 | 60.4 | 69 | 67.7 | 42.2 | 39.2 | 33.8 | 45.1 | 57.3 |
| jais-family-13b | 46.1 | 34.0 | 30.3 | 42.7 | 58.3 | 40.5 | 45.5 | 57.3 | 68.1 | 63.1 | 41.6 | 35.3 | 31.4 | 41 | 56.1 |
| jais-family-6p7b | 44.6 | 32.2 | 29.9 | 39 | 50.3 | 39.2 | 44.1 | 54.3 | 66.8 | 66.5 | 40.9 | 33.5 | 30.4 | 41.2 | 55.4 |
| jais-family-2p7b | 41.0 | 29.5 | 28.5 | 36.1 | 45.7 | 32.4 | 40.8 | 44.2 | 62.5 | 62.2 | 39.2 | 27.4 | 28.2 | 43.6 | 53.6 |
| jais-family-1p3b | 40.8 | 28.9 | 28.5 | 34.2 | 45.7 | 32.4 | 40.8 | 44.2 | 62.5 | 62.2 | 39.2 | 27.4 | 28.2 | 43.6 | 53.6 |
| jais-family-590m | 39.7 | 31.2 | 27 | 33.1 | 41.7 | 33.8 | 38.8 | 38.2 | 60.7 | 62.2 | 37.9 | 25.5 | 27.4 | 44.7 | 53.3 |
| jais-family-30b-16k-chat | 51.6 | 59.9 | 34.6 | 40.2 | 58.9 | 46.8 | 54.7 | 56.2 | 64.4 | 76.7 | 55.9 | 40.8 | 30.8 | 49.5 | 52.9 |
| jais-family-30b-8k-chat | 51.4 | 61.2 | 34.2 | 40.2 | 54.3 | 47.3 | 53.6 | 60 | 63.4 | 76.8 | 54.7 | 39.5 | 30 | 50.7 | 54.3 |
| jais-family-13b-chat | 50.3 | 58.2 | 33.9 | 42.9 | 53.1 | 46.8 | 51.7 | 59.3 | 65.4 | 75.2 | 51.2 | 38.4 | 29.8 | 44.8 | 53.8 |
| jais-family-6p7b-chat | 48.7 | 55.7 | 32.8 | 37.7 | 49.7 | 40.5 | 50.1 | 56.2 | 62.9 | 79.4 | 52 | 38 | 30.4 | 44.7 | 52 |
| jais-family-2p7b-chat | 45.6 | 50.0 | 31.5 | 35.9 | 41.1 | 37.3 | 42.1 | 48.6 | 63.7 | 74.4 | 50.9 | 35.3 | 31.2 | 44.5 | 51.3 |
| jais-family-1p3b-chat | 42.7 | 42.2 | 30.1 | 33.6 | 40.6 | 34.1 | 41.2 | 43 | 63.6 | 69.3 | 44.9 | 31.6 | 28 | 45.6 | 50.4 |
| jais-family-590m-chat | 37.8 | 39.1 | 28 |29.5 | 33.1 | 30.8 | 36.4 | 30.3 | 57.8 | 57.2 | 40.5 | 25.9 | 26.8 | 44.5 | 49.3 |
| **Adapted Models** | Avg | ArabicMMLU*| MMLU | EXAMS*| LitQA*| agqa | agrc | Hellaswag | PIQA | BoolQA | Situated QA | ARC-C | OpenBookQA | TruthfulQA | CrowS-Pairs |
|--------------------------|-------|------------|-------|-------|-------|------|------|------------|------|--------|-------------|-------|------------|------------|-------------|
| jais-adapted-70b | 51.5 | 55.9 | 36.8 | 42.3 | 58.3 | 48.6 | 54 | 61.5 | 68.4 | 68.4 | 42.1 | 42.6 | 33 | 50.2 | 58.3 |
| jais-adapted-13b | 46.6 | 44.7 | 30.6 | 37.7 | 54.3 | 43.8 | 48.3 | 54.9 | 67.1 | 64.5 | 40.6 | 36.1 | 32 | 43.6 | 54.00 |
| jais-adapted-7b | 42.0 | 35.9 | 28.9 | 36.7 | 46.3 | 34.1 | 40.3 | 45 | 61.3 | 63.8 | 38.1 | 29.7 | 30.2 | 44.3 | 53.6 |
| jais-adapted-70b-chat | 52.9 | 66.8 | 34.6 | 42.5 | 62.9 | 36.8 | 48.6 | 64.5 | 69.7 | 82.8 | 49.3 | 44.2 | 32.2 | 53.3 | 52.4 |
| jais-adapted-13b-chat | 50.3 | 59.0 | 31.7 | 37.5 | 56.6 | 41.9 | 51.7 | 58.8 | 67.1 | 78.2 | 45.9 | 41 | 34.2 | 48.3 | 52.1 |
| jais-adapted-7b-chat | 46.1 | 51.3 | 30 | 37 | 48 | 36.8 | 48.6 | 51.1 | 62.9 | 72.4 | 41.3 | 34.6 | 30.4 | 48.6 | 51.8 |
</div>
Arabic benchmarks are translated using an in-house MT model and reviewed by Arabic linguists. Benchmarks labeled with an asterisk (*) are natively Arabic; for further details, see the [Jais paper](https://arxiv.org/abs/2308.16149). Additionally, we include [ArabicMMLU](https://arxiv.org/abs/2402.12840), a native Arabic benchmark based on regional knowledge.
### English evaluation results:
<div class="table-container">
| **Models** | Avg | MMLU | RACE | Hellaswag | PIQA | BoolQA | SIQA | ARC-Challenge | OpenBookQA | Winogrande | TruthfulQA | CrowS-Pairs |
|--------------------------|----------|------|------|-----------|------|--------|------|---------------|------------|------------|----------------|-------------|
| jais-family-30b-16k | 59.3 | 42.2 | 40.5 | 79.7 | 80.6 | 78.7 | 48.8 | 50.3 | 44.2 | 71.6 | 43.5 | 72.6 |
| jais-family-30b-8k | 58.8 | 42.3 | 40.3 | 79.1 | 80.5 | 80.9 | 49.3 | 48.4 | 43.2 | 70.6 | 40.3 | 72.3 |
| jais-family-13b | 54.6 | 32.3 | 39 | 72 | 77.4 | 73.9 | 47.9 | 43.2 | 40 | 67.1 | 36.1 | 71.7 |
| jais-family-6p7b | 53.1 | 32 | 38 | 69.3 | 76 | 71.7 | 47.1 | 40.3 | 37.4 | 65.1 | 34.4 | 72.5 |
| jais-family-2p7b | 51 | 29.4 | 38 | 62.7 | 74.1 | 67.4 | 45.6 | 35.1 | 35.6 | 62.9 | 40.1 | 70.2 |
| jais-family-1p3b | 48.7 | 28.2 | 35.4 | 55.4 | 72 | 62.7 | 44.9 | 30.7 | 36.2 | 60.9 | 40.4 | 69 |
| jais-family-590m | 45.2 | 27.8 | 32.9 | 46.1 | 68.1 | 60.4 | 43.2 | 25.6 | 30.8 | 55.8 | 40.9 | 65.3 |
| jais-family-30b-16k-chat | 58.8 | 42 | 41.1 | 76.2 | 73.3 | 84.6 | 60.3 | 48.4 | 40.8 | 68.2 | 44.8 | 67 |
| jais-family-30b-8k-chat | 60.3 | 40.6 | 47.1 | 78.9 | 72.7 | 90.6 | 60 | 50.1 | 43.2 | 70.6 | 44.9 | 64.2 |
| jais-family-13b-chat | 57.5 | 36.6 | 42.6 | 75 | 75.8 | 87.6 | 54.4 | 47.9 | 42 | 65 | 40.6 | 64.5 |
| jais-family-6p7b-chat | 56 | 36.6 | 41.3 | 72 | 74 | 86.9 | 55.4 | 44.6 | 40 | 62.4 | 41 | 62.2 |
| jais-family-2p7b-chat | 52.8 | 32.7 | 40.4 | 62.2 | 71 | 84.1 | 54 | 37.2 | 36.8 | 61.4 | 40.9 | 59.8 |
| jais-family-1p3b-chat | 49.3 | 31.9 | 37.4 | 54.5 | 70.2 | 77.8 | 49.8 | 34.4 | 35.6 | 52.7 | 37.2 | 60.8 |
| jais-family-590m-chat | 42.6 | 27.9 | 33.4 | 33.1 | 63.7 | 60.1 | 45.3 | 26.7 | 25.8 | 50.5 | 44.5 | 57.7 |
</div>
<div class="table-container">
|**Adapted Models**| Avg | MMLU | RACE | Hellaswag | PIQA | BoolQA | SIQA | ARC-Challenge | OpenBookQA | Winogrande | TruthfulQA | CrowS-Pairs |
|--------------------------|----------|------|------|-----------|------|--------|------|---------------|------------|------------|----------------|-------------|
| jais-adapted-70b | 60.1 | 40.4 | 38.5 | 81.2 | 81.1 | 81.2 | 48.1 | 50.4 | 45 | 75.8 | 45.7 | 74 |
| jais-adapted-13b | 56 | 33.8 | 39.5 | 76.5 | 78.6 | 77.8 | 44.6 | 45.9 | 44.4 | 71.4 | 34.6 | 69 |
| jais-adapted-7b | 55.7 | 32.2 | 39.8 | 75.3 | 78.8 | 75.7 | 45.2 | 42.8 | 43 | 68 | 38.3 | 73.1 |
| jais-adapted-70b-chat | 61.4 | 38.7 | 42.9 | 82.7 | 81.2 | 89.6 | 52.9 | 54.9 | 44.4 | 75.7 | 44 | 68.8 |
| jais-adapted-13b-chat | 58.5 | 34.9 | 42.4 | 79.6 | 79.7 | 88.2 | 50.5 | 48.5 | 42.4 | 70.3 | 42.2 | 65.1 |
| jais-adapted-7b-chat | 58.5 | 33.8 | 43.9 | 77.8 | 79.4 | 87.1 | 47.3 | 46.9 | 43.4 | 69.9 | 42 | 72.4 |
</div>
### GPT-4 evaluation
In addition to the LM-Harness evaluation, we conducted an open-ended generation evaluation using GPT-4-as-a-judge. We measured pairwise win-rates of model responses in both Arabic and English on a fixed set of 80 prompts from the Vicuna test set.
English prompts were translated to Arabic by our in-house linguists.
In the following, we compare the models in this release of the jais family against previously released versions:
<p align="center">
<img src="https://huggingface.co/inceptionai/JaisFamilySupplmentary/resolve/main/jais.png" alt="Jais-adapted GPT-4">
</p>
<p align="center">
<em>GPT-4-as-a-judge evaluation of Jais in Arabic and English. Jais family models are significantly better than previous Jais at generations in both languages. </em>
</p>
<p align="center">
<img src="https://huggingface.co/inceptionai/JaisFamilySupplmentary/resolve/main/jais-adapted.png" alt="Jais-adapted GPT-4">
</p>
<p align="center">
<em>GPT-4-as-a-judge evaluation of adapted Jais in Arabic and English. The generation quality of Arabic is significantly enhanced, while achieving improvement in English when compared to Llama-2 instruct. </em>
</p>
Besides pairwise comparison, we also perform MT-bench style single-answer grading on a scale of 1 to 10.
<p align="center">
<img src="https://huggingface.co/inceptionai/JaisFamilySupplmentary/resolve/main/mt_bench.png" alt="MT-bench">
</p>
<p align="center">
<em>MT-bench style single-answer grading evaluation of Jais and adapted Jais in Arabic and English. Comparisons are made between select corresponding models from earlier releases. The quality ratings of responses are generally improved, with significant enhancements in Arabic.</em>
</p>
## Intended use
We release the Jais family of models under a full open-source license. We welcome all feedback and opportunities to collaborate. Spanning sizes from 590M to 70B parameters, this suite of bilingual models accommodates a wide range of use cases. Some potential downstream applications include:
- **Research**: The Jais family serves Arabic researchers and NLP practitioners, offering both compute-efficient and advanced model sizes
- Natural language understanding and generation tasks.
- Mechanistic interpretability analyses on cultural alignment in bilingual pre-trained and adapted pre-trained models.
- Quantitative studies of Arabic cultural and linguistic phenomena.
- **Commercial Use**: Jais 30B and 70B chat models are well-suited for direct use in chat applications with appropriate prompting or for further fine-tuning on specific tasks.
- Development of chat assistants for Arabic-speaking users.
- Sentiment analysis to gain insights into local markets and customer trends.
- Summarization of bilingual Arabic-English documents.
Audiences that we hope will benefit from our model:
- **Academics**: For those researching Arabic Natural Language Processing.
- **Businesses**: Companies targeting Arabic-speaking audiences.
- **Developers**: Those integrating Arabic language capabilities in applications.
### Out-of-Scope Use
<!-- This section addresses misuse, malicious use, and uses that the model will not work well for. -->
While the Jais family of models are powerful Arabic and English bilingual models, it's essential to understand their limitations
and the potential of misuse. It is prohibited to use the model in any manner that violates applicable laws or regulations.
The following are some example scenarios where the model should not be used.
- **Malicious Use**: The model should not be used to generate harmful, misleading, or inappropriate content. Thisincludes but is not limited to:
- Generating or promoting hate speech, violence, or discrimination.
- Spreading misinformation or fake news.
- Engaging in or promoting illegal activities.
- **Sensitive Information**: The model should not be used to handle or generate personal, confidential, or sensitive information.
- **Generalization Across All Languages**: Jais family of models are bilingual and optimized for Arabic and English. They should not be presumed to have equal proficiency in other languages or dialects.
- **High-Stakes Decisions**: The model should not be used to make high-stakes decisions without human oversight. This includes medical, legal, financial, or safety-critical decisions.
## Bias, Risks, and Limitations
<!-- This section is meant to convey both technical and sociotechnical limitations. -->
The Jais family is trained on publicly available data which was in part curated by Inception. We have employed different techniques to reduce bias in the model. While efforts have been made to minimize biases, it is likely that the model, as with all LLM models, will exhibit some bias.
The fine-tuned variants are trained as an AI assistant for Arabic and English speakers. Chat models are limited to produce responses for queries in these two languages and may not produce appropriate responses to other language queries.
By using Jais, you acknowledge and accept that, as with any large language model, it may generate incorrect, misleading and/or offensive information or content. The information is not intended as advice and should not be relied upon in any way, nor are we responsible for any of the content or consequences resulting from its use. We are continuously working to develop models with greater capabilities, and as such, welcome any feedback on the model.
Copyright Inception Institute of Artificial Intelligence Ltd. JAIS is made available under the Apache License, Version 2.0 (the “License”). You shall not use JAIS except in compliance with the License. You may obtain a copy of the License at https://www.apache.org/licenses/LICENSE-2.0.
Unless required by applicable law or agreed to in writing, JAIS is distributed on an AS IS basis, without warranties or conditions of any kind, either express or implied. Please see the terms of the License for the specific language permissions and limitations under the License.
#### Summary
We release the Jais family of Arabic and English bilingual models. The wide range of pre-trained model sizes, the recipe for adapting English-centric models to Arabic, and the fine-tuning of all sizes unlocks numerous use cases commercially and academically in the Arabic setting.
Through this release, we aim to make LLMs more accessible to Arabic NLP researchers and companies, offering native Arabic models that provide better cultural understanding than English centric ones. The strategies we employ for pre-training, fine-tuning and adaptation to Arabic are extensible to other low and medium resource languages, paving the way for language-focused and accessible models that cater to local contexts.
#### Citation info
```bibtex
@misc{sengupta2023jais,
title={Jais and Jais-chat: Arabic-Centric Foundation and Instruction-Tuned Open Generative Large Language Models},
author={Neha Sengupta, Sunil Kumar Sahu, Bokang Jia, Satheesh Katipomu, Haonan Li, Fajri Koto, William Marshall, Gurpreet Gosal, Cynthia Liu, Zhiming Chen, Osama Mohammed Afzal, Samta Kamboj, Onkar Pandit, Rahul Pal, Lalit Pradhan, Zain Muhammad Mujahid, Massa Baali, Xudong Han, Sondos Mahmoud Bsharat, Alham Fikri Aji, Zhiqiang Shen, Zhengzhong Liu, Natalia Vassilieva, Joel Hestness, Andy Hock, Andrew Feldman, Jonathan Lee, Andrew Jackson, Hector Xuguang Ren, Preslav Nakov, Timothy Baldwin and Eric Xing},
year={2023},
eprint={2308.16149},
archivePrefix={arXiv},
primaryClass={cs.CL}
}
@article{jaisfamilymodelcard,
title={Jais Family Model Card},
author={Inception},
year={2024},
url = {https://huggingface.co/inceptionai/jais-family-30b-16k-chat/blob/main/README.md}
}
``` | [
"TRANSLATION",
"SUMMARIZATION"
] | Non_BioNLP |
gaudi/opus-mt-mh-en-ctranslate2 | gaudi | translation | [
"transformers",
"marian",
"ctranslate2",
"translation",
"license:apache-2.0",
"endpoints_compatible",
"region:us"
] | 1,721,175,210,000 | 2024-10-18T22:29:14 | 6 | 0 | ---
license: apache-2.0
tags:
- ctranslate2
- translation
---
# Repository General Information
## Inspired by and derived from the work of [Helsinki-NLP](https://huggingface.co/Helsinki-NLP), [CTranslate2](https://github.com/OpenNMT/CTranslate2), and [michaelfeil](https://huggingface.co/michaelfeil)!
- Link to Original Model ([Helsinki-NLP](https://huggingface.co/Helsinki-NLP)): [Model Link](https://huggingface.co/Helsinki-NLP/opus-mt-mh-en)
- This respository was based on the work of [CTranslate2](https://github.com/OpenNMT/CTranslate2).
- This repository was based on the work of [michaelfeil](https://huggingface.co/michaelfeil).
# What is CTranslate2?
[CTranslate2](https://opennmt.net/CTranslate2/) is a C++ and Python library for efficient inference with Transformer models.
CTranslate2 implements a custom runtime that applies many performance optimization techniques such as weights quantization, layers fusion, batch reordering, etc., to accelerate and reduce the memory usage of Transformer models on CPU and GPU.
CTranslate2 is one of the most performant ways of hosting translation models at scale. Current supported models include:
- Encoder-decoder models: Transformer base/big, M2M-100, NLLB, BART, mBART, Pegasus, T5, Whisper
- Decoder-only models: GPT-2, GPT-J, GPT-NeoX, OPT, BLOOM, MPT, Llama, Mistral, Gemma, CodeGen, GPTBigCode, Falcon
- Encoder-only models: BERT, DistilBERT, XLM-RoBERTa
The project is production-oriented and comes with backward compatibility guarantees, but it also includes experimental features related to model compression and inference acceleration.
# CTranslate2 Benchmarks
Please note that the results presented below are only valid for the configuration used during this benchmark: absolute and relative performance may change with different settings. Tested against `newstest2014` (En -> De) dataset.
The benchmark reports the number of target tokens generated per second (higher is better). The results are aggregated over multiple runs. See the benchmark scripts for more details and reproduce these numbers.
Please note that the results presented below are only valid for the configuration used during this benchmark: absolute and relative performance may change with different settings.
## CPU Benchmarks for Generic Opus-MT Models
| Library | Tokens per Second | Max Memory Usage | BLEU |
| :----: | :----: | :----: | :----: |
| Transformers 4.26.1 (with PyTorch 1.13.1) | 147.3 | 2332MB | 27.90 |
| Marian 1.11.0 (int16) | 330.2 | 5901MB | 27.65 |
| Marian 1.11.0 (int8) | 355.8 | 4763MB | 27.27 |
| CTranslate2 3.6.0 (int16) | 596.1 | 660MB | 27.53 |
| CTranslate2 3.6.0 (int8) | 696.1 | 516MB | 27.65 |
## GPU Benchmarks for Generic Opus-MT Models
| Library | Tokens per Second | Max GPU Memory Usage | Max Memory Usage | BLEU |
| :----: | :----: | :----: | :----: | :----: |
| Transformers 4.26.1 (with PyTorch 1.13.1) | 1022.9 | 4097MB | 2109MB | 27.90 |
| Marian 1.11.0 (float16) | 3962.4 | 3239MB | 1976MB | 27.94 |
| CTranslate2 3.6.0 (float16) | 9296.7 | 909MB | 814MB | 27.9 |
| CTranslate2 3.6.0 (int8 + float16) | 8362.7 | 813MB | 766MB | 27.9 |
`Executed with 4 threads on a c5.2xlarge Amazon EC2 instance equipped with an Intel(R) Xeon(R) Platinum 8275CL CPU.`
**Source to benchmark information can be found [here](https://github.com/OpenNMT/CTranslate2).**<br />
**Original model BLEU scores can be found [here](https://huggingface.co/Helsinki-NLP/opus-mt-mh-en).**
## Internal Benchmarks
Internal testing on our end showed **inference times reduced by 6x-10x** on average compared the vanilla checkpoints using the *transformers* library. A **slight reduction on BLEU scores (~5%)** was also identified in comparison to the vanilla checkpoints with a few exceptions. This is likely due to several factors, one being the quantization applied. Further testing is needed from our end to better assess the reduction in translation quality. The command used to compile the vanilla checkpoint into a CTranslate2 model can be found below. Modifying this command can yield differing balances between inferencing performance and translation quality.
# CTranslate2 Installation
```bash
pip install hf-hub-ctranslate2>=1.0.0 ctranslate2>=3.13.0
```
### ct2-transformers-converter Command Used:
```bash
ct2-transformers-converter --model Helsinki-NLP/opus-mt-mh-en --output_dir ./ctranslate2/opus-mt-mh-en-ctranslate2 --force --copy_files README.md generation_config.json tokenizer_config.json vocab.json source.spm .gitattributes target.spm --quantization float16
```
# CTranslate2 Converted Checkpoint Information:
**Compatible With:**
- [ctranslate2](https://github.com/OpenNMT/CTranslate2)
- [hf-hub-ctranslate2](https://github.com/michaelfeil/hf-hub-ctranslate2)
**Compute Type:**
- `compute_type=int8_float16` for `device="cuda"`
- `compute_type=int8` for `device="cpu"`
# Sample Code - ctranslate2
#### Clone the repository to the working directory or wherever you wish to store the model artifacts. ####
```bash
git clone https://huggingface.co/gaudi/opus-mt-mh-en-ctranslate2
```
#### Take the python code below and update the 'model_dir' variable to the location of the cloned repository. ####
```python
from ctranslate2 import Translator
import transformers
model_dir = "./opus-mt-mh-en-ctranslate2" # Path to model directory.
translator = Translator(
model_path=model_dir,
device="cuda", # cpu, cuda, or auto.
inter_threads=1, # Maximum number of parallel translations.
intra_threads=4, # Number of OpenMP threads per translator.
compute_type="int8_float16", # int8 for cpu or int8_float16 for cuda.
)
tokenizer = transformers.AutoTokenizer.from_pretrained(model_dir)
source = tokenizer.convert_ids_to_tokens(tokenizer.encode("XXXXXX, XXX XX XXXXXX."))
results = translator.translate_batch([source])
target = results[0].hypotheses[0]
print(tokenizer.decode(tokenizer.convert_tokens_to_ids(target)))
```
# Sample Code - hf-hub-ctranslate2
**Derived From [michaelfeil](https://huggingface.co/michaelfeil):**
```python
from hf_hub_ctranslate2 import TranslatorCT2fromHfHub, GeneratorCT2fromHfHub
from transformers import AutoTokenizer
model_name = "gaudi/opus-mt-mh-en-ctranslate2"
model = TranslatorCT2fromHfHub(
model_name_or_path=model_name,
device="cuda",
compute_type="int8_float16",
tokenizer=AutoTokenizer.from_pretrained(model_name)
)
outputs = model.generate(
text=["XXX XX XXX XXXXXXX XXXX?", "XX XX XXXX XX XXX!"],
)
print(outputs)
```
# License and other remarks:
License conditions are intended to be idential to [original huggingface repository](https://huggingface.co/Helsinki-NLP/opus-mt-mh-en) by Helsinki-NLP.
| [
"TRANSLATION"
] | Non_BioNLP |
santoro/distilbert-base-uncased-finetuned-emotion | santoro | text-classification | [
"transformers",
"pytorch",
"distilbert",
"text-classification",
"generated_from_trainer",
"dataset:emotion",
"base_model:distilbert/distilbert-base-uncased",
"base_model:finetune:distilbert/distilbert-base-uncased",
"license:apache-2.0",
"model-index",
"autotrain_compatible",
"endpoints_compatible",
"region:us"
] | 1,693,678,960,000 | 2023-09-02T20:55:08 | 10 | 0 | ---
base_model: distilbert-base-uncased
datasets:
- emotion
license: apache-2.0
metrics:
- accuracy
- f1
tags:
- generated_from_trainer
model-index:
- name: distilbert-base-uncased-finetuned-emotion
results:
- task:
type: text-classification
name: Text Classification
dataset:
name: emotion
type: emotion
config: split
split: validation
args: split
metrics:
- type: accuracy
value: 0.922
name: Accuracy
- type: f1
value: 0.9218197070909727
name: F1
---
<!-- This model card has been generated automatically according to the information the Trainer had access to. You
should probably proofread and complete it, then remove this comment. -->
# distilbert-base-uncased-finetuned-emotion
This model is a fine-tuned version of [distilbert-base-uncased](https://huggingface.co/distilbert-base-uncased) on the emotion dataset.
It achieves the following results on the evaluation set:
- Loss: 0.2228
- Accuracy: 0.922
- F1: 0.9218
## Model description
More information needed
## Intended uses & limitations
More information needed
## Training and evaluation data
More information needed
## Training procedure
### Training hyperparameters
The following hyperparameters were used during training:
- learning_rate: 2e-05
- train_batch_size: 64
- eval_batch_size: 64
- seed: 42
- optimizer: Adam with betas=(0.9,0.999) and epsilon=1e-08
- lr_scheduler_type: linear
- num_epochs: 2
### Training results
| Training Loss | Epoch | Step | Validation Loss | Accuracy | F1 |
|:-------------:|:-----:|:----:|:---------------:|:--------:|:------:|
| 0.8615 | 1.0 | 250 | 0.3301 | 0.9055 | 0.9045 |
| 0.261 | 2.0 | 500 | 0.2228 | 0.922 | 0.9218 |
### Framework versions
- Transformers 4.32.1
- Pytorch 2.0.1+cu118
- Datasets 2.14.4
- Tokenizers 0.13.3
| [
"TEXT_CLASSIFICATION"
] | Non_BioNLP |
IsmatS/azeri-turkish-bert-ner | IsmatS | token-classification | [
"transformers",
"safetensors",
"bert",
"token-classification",
"NER",
"Azerbaijani",
"Turkish",
"az",
"tr",
"dataset:LocalDoc/azerbaijani-ner-dataset",
"base_model:akdeniz27/bert-base-turkish-cased-ner",
"base_model:finetune:akdeniz27/bert-base-turkish-cased-ner",
"license:mit",
"autotrain_compatible",
"endpoints_compatible",
"region:us"
] | 1,731,104,221,000 | 2024-11-08T22:26:45 | 16 | 0 | ---
base_model: akdeniz27/bert-base-turkish-cased-ner
datasets:
- LocalDoc/azerbaijani-ner-dataset
language:
- az
- tr
license: mit
metrics:
- precision
- recall
- f1
pipeline_tag: token-classification
tags:
- NER
- token-classification
- Azerbaijani
- Turkish
- transformers
thumbnail: URL_to_thumbnail_image
---
# Azeri-Turkish-BERT-NER
## Model Description
The **Azeri-Turkish-BERT-NER** model is a fine-tuned version of the `bert-base-turkish-cased-ner` model for Named Entity Recognition (NER) tasks in the Azerbaijani and Turkish languages. This model builds upon a pre-trained Turkish BERT model and adapts it to perform NER tasks specifically for Azerbaijani data while preserving compatibility with Turkish entities.
The model can identify and classify named entities into a variety of categories, such as persons, organizations, locations, dates, and more, making it suitable for applications such as text extraction, entity recognition, and data processing in Azerbaijani and Turkish texts.
## Model Details
- **Base Model**: `bert-base-turkish-cased-ner` (adapted from Hugging Face)
- **Task**: Named Entity Recognition (NER)
- **Languages**: Azerbaijani, Turkish
- **Fine-Tuned On**: Custom Azerbaijani NER dataset
- **Input Text Format**: Plain text with tokenized words
- **Model Type**: BERT-based transformer for token classification
## Training Details
The model was fine-tuned using the Hugging Face `transformers` library and `datasets`. Here is a brief summary of the fine-tuning configuration:
- **Tokenizer**: `AutoTokenizer` from the `bert-base-turkish-cased-ner` model
- **Max Sequence Length**: 128 tokens
- **Batch Size**: 128 (training and evaluation)
- **Learning Rate**: 2e-5
- **Number of Epochs**: 10
- **Weight Decay**: 0.005
- **Optimization Strategy**: Early stopping with a patience of 5 epochs based on the F1 metric
### Training Dataset
The training dataset is a custom Azerbaijani NER dataset sourced from [LocalDoc/azerbaijani-ner-dataset](https://huggingface.co/datasets/LocalDoc/azerbaijani-ner-dataset). The dataset was preprocessed to align tokens and NER tags accurately.
### Label Categories
The model supports the following entity categories:
- **Person (B-PERSON, I-PERSON)**
- **Location (B-LOCATION, I-LOCATION)**
- **Organization (B-ORGANISATION, I-ORGANISATION)**
- **Date (B-DATE, I-DATE)**
- **Time (B-TIME, I-TIME)**
- **Money (B-MONEY, I-MONEY)**
- **Percentage (B-PERCENTAGE, I-PERCENTAGE)**
- **Facility (B-FACILITY, I-FACILITY)**
- **Product (B-PRODUCT, I-PRODUCT)**
- ... (additional categories as specified in the training label list)
### Training Metrics
| Epoch | Training Loss | Validation Loss | Precision | Recall | F1 |
|-------|---------------|-----------------|-----------|--------|-------|
| 1 | 0.433100 | 0.306711 | 0.739000 | 0.693282 | 0.715412 |
| 2 | 0.292700 | 0.275796 | 0.781565 | 0.688937 | 0.732334 |
| 3 | 0.250600 | 0.275115 | 0.758261 | 0.709425 | 0.733031 |
| 4 | 0.233700 | 0.273087 | 0.756184 | 0.716277 | 0.735689 |
| 5 | 0.214800 | 0.278477 | 0.756051 | 0.710996 | 0.732832 |
| 6 | 0.199200 | 0.286102 | 0.755068 | 0.717012 | 0.735548 |
| 7 | 0.192800 | 0.297157 | 0.742326 | 0.725802 | 0.733971 |
| 8 | 0.178900 | 0.304510 | 0.743206 | 0.723930 | 0.733442 |
| 9 | 0.171700 | 0.313845 | 0.743145 | 0.725535 | 0.734234 |
### Category-Wise Evaluation Metrics
| Category | Precision | Recall | F1-Score | Support |
|---------------|-----------|--------|----------|---------|
| ART | 0.49 | 0.14 | 0.21 | 1988 |
| DATE | 0.49 | 0.48 | 0.49 | 844 |
| EVENT | 0.88 | 0.36 | 0.51 | 84 |
| FACILITY | 0.72 | 0.68 | 0.70 | 1146 |
| LAW | 0.57 | 0.64 | 0.60 | 1103 |
| LOCATION | 0.77 | 0.79 | 0.78 | 8806 |
| MONEY | 0.62 | 0.57 | 0.59 | 532 |
| ORGANISATION | 0.64 | 0.65 | 0.64 | 527 |
| PERCENTAGE | 0.77 | 0.83 | 0.80 | 3679 |
| PERSON | 0.87 | 0.81 | 0.84 | 6924 |
| PRODUCT | 0.82 | 0.80 | 0.81 | 2653 |
| TIME | 0.55 | 0.50 | 0.52 | 1634 |
- **Micro Average**: Precision: 0.76, Recall: 0.72, F1-Score: 0.74
- **Macro Average**: Precision: 0.68, Recall: 0.60, F1-Score: 0.62
- **Weighted Average**: Precision: 0.74, Recall: 0.72, F1-Score: 0.72
## Usage
### Loading the Model
To use the model for NER tasks, you can load it using the Hugging Face `transformers` library:
```python
from transformers import AutoTokenizer, AutoModelForTokenClassification, pipeline
# Load the model and tokenizer
tokenizer = AutoTokenizer.from_pretrained("IsmatS/Azeri-Turkish-BERT-NER")
model = AutoModelForTokenClassification.from_pretrained("IsmatS/Azeri-Turkish-BERT-NER")
# Initialize the NER pipeline
ner_pipeline = pipeline("ner", model=model, tokenizer=tokenizer, aggregation_strategy="simple")
# Example text
text = "Shahla Khuduyeva və Pasha Sığorta şirkəti haqqında məlumat."
# Run NER
results = ner_pipeline(text)
print(results)
```
### Inputs and Outputs
- **Input**: Plain text in Azerbaijani or Turkish.
- **Output**: List of detected entities with entity types and character offsets.
Example output:
```
[
{'entity_group': 'B-PERSON', 'word': 'Shahla', 'start': 0, 'end': 6, 'score': 0.98},
{'entity_group': 'B-ORGANISATION', 'word': 'Pasha Sığorta', 'start': 11, 'end': 24, 'score': 0.95}
]
```
### Evaluation Metrics
The model was evaluated using precision, recall, and F1-score metrics as detailed in the training metrics section.
## Limitations
- The model may have limited performance on texts that diverge significantly from the training data distribution.
- Handling of rare or unseen entities in Turkish and Azerbaijani may result in lower confidence scores.
- Further fine-tuning on larger and more diverse datasets may improve generalizability.
## Model Card
A detailed model card with additional training details, dataset descriptions, and usage recommendations is available on the [Hugging Face model page](https://huggingface.co/IsmatS/Azeri-Turkish-BERT-NER).
## Citation
If you use this model, please consider citing:
```
@misc{azeri-turkish-bert-ner,
author = {Ismat Samadov},
title = {Azeri-Turkish-BERT-NER},
year = {2024},
howpublished = {Hugging Face repository},
}
``` | [
"NAMED_ENTITY_RECOGNITION"
] | Non_BioNLP |
gokulsrinivasagan/bert_tiny_lda_book_mnli | gokulsrinivasagan | text-classification | [
"transformers",
"tensorboard",
"safetensors",
"distilbert",
"text-classification",
"generated_from_trainer",
"en",
"dataset:glue",
"base_model:gokulsrinivasagan/bert_tiny_lda_book",
"base_model:finetune:gokulsrinivasagan/bert_tiny_lda_book",
"model-index",
"autotrain_compatible",
"endpoints_compatible",
"region:us"
] | 1,733,744,508,000 | 2024-12-09T12:12:39 | 5 | 0 | ---
base_model: gokulsrinivasagan/bert_tiny_lda_book
datasets:
- glue
language:
- en
library_name: transformers
metrics:
- accuracy
tags:
- generated_from_trainer
model-index:
- name: bert_tiny_lda_book_mnli
results:
- task:
type: text-classification
name: Text Classification
dataset:
name: GLUE MNLI
type: glue
args: mnli
metrics:
- type: accuracy
value: 0.7542717656631408
name: Accuracy
---
<!-- This model card has been generated automatically according to the information the Trainer had access to. You
should probably proofread and complete it, then remove this comment. -->
# bert_tiny_lda_book_mnli
This model is a fine-tuned version of [gokulsrinivasagan/bert_tiny_lda_book](https://huggingface.co/gokulsrinivasagan/bert_tiny_lda_book) on the GLUE MNLI dataset.
It achieves the following results on the evaluation set:
- Loss: 0.6122
- Accuracy: 0.7543
## Model description
More information needed
## Intended uses & limitations
More information needed
## Training and evaluation data
More information needed
## Training procedure
### Training hyperparameters
The following hyperparameters were used during training:
- learning_rate: 5e-05
- train_batch_size: 256
- eval_batch_size: 256
- seed: 10
- optimizer: Use adamw_torch with betas=(0.9,0.999) and epsilon=1e-08 and optimizer_args=No additional optimizer arguments
- lr_scheduler_type: linear
- num_epochs: 50
### Training results
| Training Loss | Epoch | Step | Validation Loss | Accuracy |
|:-------------:|:-----:|:-----:|:---------------:|:--------:|
| 0.7886 | 1.0 | 1534 | 0.6904 | 0.7072 |
| 0.6409 | 2.0 | 3068 | 0.6388 | 0.7326 |
| 0.5591 | 3.0 | 4602 | 0.6227 | 0.7473 |
| 0.4882 | 4.0 | 6136 | 0.6451 | 0.7508 |
| 0.4258 | 5.0 | 7670 | 0.6553 | 0.7524 |
| 0.369 | 6.0 | 9204 | 0.7156 | 0.7534 |
| 0.3172 | 7.0 | 10738 | 0.7423 | 0.7491 |
| 0.2729 | 8.0 | 12272 | 0.8130 | 0.7476 |
### Framework versions
- Transformers 4.46.3
- Pytorch 2.2.1+cu118
- Datasets 2.17.0
- Tokenizers 0.20.3
| [
"TEXT_CLASSIFICATION"
] | Non_BioNLP |
RohanHBTU/autotrain-t5-hinglish-to-en | RohanHBTU | translation | [
"transformers",
"pytorch",
"safetensors",
"t5",
"text2text-generation",
"autotrain",
"translation",
"unk",
"dataset:RohanHBTU/autotrain-data-t5-autotrain",
"co2_eq_emissions",
"autotrain_compatible",
"text-generation-inference",
"endpoints_compatible",
"region:us"
] | 1,678,991,576,000 | 2024-01-14T10:18:43 | 22 | 1 | ---
datasets:
- RohanHBTU/autotrain-data-t5-autotrain
language:
- unk
- unk
tags:
- autotrain
- translation
co2_eq_emissions:
emissions: 0.003572495100254721
---
# Model Trained Using AutoTrain
- Problem type: Translation
- Model ID: 41534106887
- CO2 Emissions (in grams): 0.0036
## Validation Metrics
- Loss: 1.785
- SacreBLEU: 24.776
- Gen len: 9.347 | [
"TRANSLATION"
] | Non_BioNLP |
rambodazimi/distilbert-base-uncased-finetuned-LoRA-WNLI | rambodazimi | null | [
"safetensors",
"generated_from_trainer",
"dataset:glue",
"license:apache-2.0",
"model-index",
"region:us"
] | 1,725,393,418,000 | 2024-09-03T19:58:18 | 0 | 0 | ---
datasets:
- glue
license: apache-2.0
metrics:
- accuracy
tags:
- generated_from_trainer
model-index:
- name: distilbert-base-uncased-finetuned-LoRA-WNLI
results:
- task:
type: text-classification
name: Text Classification
dataset:
name: glue
type: glue
args: wnli
metrics:
- type: accuracy
value: 0.5211267605633803
name: Accuracy
---
<!-- This model card has been generated automatically according to the information the Trainer had access to. You
should probably proofread and complete it, then remove this comment. -->
# distilbert-base-uncased-finetuned-lora-wnli
This model is a fine-tuned version of [distilbert-base-uncased](https://huggingface.co/distilbert/distilbert-base-uncased) on the glue dataset.
It achieves the following results on the evaluation set:
- Accuracy: 0.5211
- trainable model parameters: 739586
- all model parameters: 67694596
- percentage of trainable model parameters: 1.09%
## Training procedure
### Training hyperparameters
The following hyperparameters were used during training:
- learning_rate: 5e-04
- train_batch_size: 32
- eval_batch_size: 32
- seed: 42
- weight_decay: 0.01
- rank: 8
- lora_alpha: 16
- lora_dropout: 0.05
- num_epochs: 4 | [
"TEXT_CLASSIFICATION"
] | TBD |
super-cinnamon/fewshot-followup-multi-e5 | super-cinnamon | text-classification | [
"setfit",
"safetensors",
"bert",
"sentence-transformers",
"text-classification",
"generated_from_setfit_trainer",
"fr",
"en",
"arxiv:2209.11055",
"base_model:intfloat/multilingual-e5-small",
"base_model:finetune:intfloat/multilingual-e5-small",
"model-index",
"region:us"
] | 1,702,384,627,000 | 2023-12-12T13:49:02 | 165 | 2 | ---
base_model: intfloat/multilingual-e5-small
language:
- fr
- en
library_name: setfit
metrics:
- accuracy
pipeline_tag: text-classification
tags:
- setfit
- sentence-transformers
- text-classification
- generated_from_setfit_trainer
widget:
- text: Quelles sont les règles en matière de garde d'enfants et de pension alimentaire
?
- text: Comment se déroule une procédure de divorce ?
- text: Quelles sont les principales difficultés rencontrées dans l'application de
cette loi ?
- text: Quels sont les régimes matrimoniaux possibles ?
- text: Comment peut-on obtenir réparation pour un préjudice subi du fait d'une décision
administrative illégale ?
inference: true
model-index:
- name: SetFit with intfloat/multilingual-e5-small
results:
- task:
type: text-classification
name: Text Classification
dataset:
name: Unknown
type: unknown
split: test
metrics:
- type: accuracy
value: 1
name: Accuracy
---
# SetFit with intfloat/multilingual-e5-small
This is a [SetFit](https://github.com/huggingface/setfit) model that can be used for Text Classification. This SetFit model uses [intfloat/multilingual-e5-small](https://huggingface.co/intfloat/multilingual-e5-small) as the Sentence Transformer embedding model. A [LogisticRegression](https://scikit-learn.org/stable/modules/generated/sklearn.linear_model.LogisticRegression.html) instance is used for classification.
The model has been trained using an efficient few-shot learning technique that involves:
1. Fine-tuning a [Sentence Transformer](https://www.sbert.net) with contrastive learning.
2. Training a classification head with features from the fine-tuned Sentence Transformer.
## Model Details
### Model Description
- **Model Type:** SetFit
- **Sentence Transformer body:** [intfloat/multilingual-e5-small](https://huggingface.co/intfloat/multilingual-e5-small)
- **Classification head:** a [LogisticRegression](https://scikit-learn.org/stable/modules/generated/sklearn.linear_model.LogisticRegression.html) instance
- **Maximum Sequence Length:** 512 tokens
- **Number of Classes:** 2 classes
<!-- - **Training Dataset:** [Unknown](https://huggingface.co/datasets/unknown) -->
<!-- - **Language:** Unknown -->
<!-- - **License:** Unknown -->
### Model Sources
- **Repository:** [SetFit on GitHub](https://github.com/huggingface/setfit)
- **Paper:** [Efficient Few-Shot Learning Without Prompts](https://arxiv.org/abs/2209.11055)
- **Blogpost:** [SetFit: Efficient Few-Shot Learning Without Prompts](https://huggingface.co/blog/setfit)
### Model Labels
| Label | Examples |
|:------------|:------------------------------------------------------------------------------------------------------------------------------------------------------------------------------------------------------------------------------|
| independent | <ul><li>'Comment rédiger un contrat de travail ?'</li><li>'Quels sont les impôts et taxes applicables aux entreprises ?'</li><li>'Comment peut-on contester un licenciement abusif ?'</li></ul> |
| follow_up | <ul><li>'Quelles sont les conséquences de cette loi ?'</li><li>"Comment cette loi s'inscrit-elle dans le cadre plus large du droit algérien ?"</li><li>"Comment puis-je obtenir plus d'informations sur ce sujet ?"</li></ul> |
## Evaluation
### Metrics
| Label | Accuracy |
|:--------|:---------|
| **all** | 1.0 |
## Uses
### Direct Use for Inference
First install the SetFit library:
```bash
pip install setfit
```
Then you can load this model and run inference.
```python
from setfit import SetFitModel
# Download from the 🤗 Hub
model = SetFitModel.from_pretrained("super-cinnamon/fewshot-followup-multi-e5")
# Run inference
preds = model("Comment se déroule une procédure de divorce ?")
```
<!--
### Downstream Use
*List how someone could finetune this model on their own dataset.*
-->
<!--
### Out-of-Scope Use
*List how the model may foreseeably be misused and address what users ought not to do with the model.*
-->
<!--
## Bias, Risks and Limitations
*What are the known or foreseeable issues stemming from this model? You could also flag here known failure cases or weaknesses of the model.*
-->
<!--
### Recommendations
*What are recommendations with respect to the foreseeable issues? For example, filtering explicit content.*
-->
## Training Details
### Training Set Metrics
| Training set | Min | Median | Max |
|:-------------|:----|:-------|:----|
| Word count | 1 | 9.6184 | 16 |
| Label | Training Sample Count |
|:------------|:----------------------|
| independent | 43 |
| follow_up | 33 |
### Training Hyperparameters
- batch_size: (8, 8)
- num_epochs: (10, 10)
- max_steps: -1
- sampling_strategy: oversampling
- body_learning_rate: (2e-05, 1e-05)
- head_learning_rate: 0.01
- loss: CosineSimilarityLoss
- distance_metric: cosine_distance
- margin: 0.25
- end_to_end: False
- use_amp: False
- warmup_proportion: 0.1
- seed: 42
- eval_max_steps: -1
- load_best_model_at_end: False
### Training Results
| Epoch | Step | Training Loss | Validation Loss |
|:------:|:----:|:-------------:|:---------------:|
| 0.0027 | 1 | 0.3915 | - |
| 0.1326 | 50 | 0.3193 | - |
| 0.2653 | 100 | 0.2252 | - |
| 0.3979 | 150 | 0.1141 | - |
| 0.5305 | 200 | 0.0197 | - |
| 0.6631 | 250 | 0.0019 | - |
| 0.7958 | 300 | 0.0021 | - |
| 0.9284 | 350 | 0.0002 | - |
| 1.0610 | 400 | 0.0008 | - |
| 1.1936 | 450 | 0.0005 | - |
| 1.3263 | 500 | 0.0002 | - |
| 1.4589 | 550 | 0.0002 | - |
| 1.5915 | 600 | 0.0007 | - |
| 1.7241 | 650 | 0.0001 | - |
| 1.8568 | 700 | 0.0003 | - |
| 1.9894 | 750 | 0.0002 | - |
| 2.1220 | 800 | 0.0001 | - |
| 2.2546 | 850 | 0.0002 | - |
| 2.3873 | 900 | 0.0 | - |
| 2.5199 | 950 | 0.0003 | - |
| 2.6525 | 1000 | 0.0001 | - |
| 2.7851 | 1050 | 0.0001 | - |
| 2.9178 | 1100 | 0.0001 | - |
| 3.0504 | 1150 | 0.0001 | - |
| 3.1830 | 1200 | 0.0001 | - |
| 3.3156 | 1250 | 0.0001 | - |
| 3.4483 | 1300 | 0.0001 | - |
| 3.5809 | 1350 | 0.0001 | - |
| 3.7135 | 1400 | 0.0 | - |
| 3.8462 | 1450 | 0.0 | - |
| 3.9788 | 1500 | 0.0 | - |
| 4.1114 | 1550 | 0.0 | - |
| 4.2440 | 1600 | 0.0001 | - |
| 4.3767 | 1650 | 0.0001 | - |
| 4.5093 | 1700 | 0.0001 | - |
| 4.6419 | 1750 | 0.0001 | - |
| 4.7745 | 1800 | 0.0 | - |
| 4.9072 | 1850 | 0.0001 | - |
| 5.0398 | 1900 | 0.0 | - |
| 5.1724 | 1950 | 0.0001 | - |
| 5.3050 | 2000 | 0.0 | - |
| 5.4377 | 2050 | 0.0001 | - |
| 5.5703 | 2100 | 0.0 | - |
| 5.7029 | 2150 | 0.0 | - |
| 5.8355 | 2200 | 0.0 | - |
| 5.9682 | 2250 | 0.0001 | - |
| 6.1008 | 2300 | 0.0001 | - |
| 6.2334 | 2350 | 0.0 | - |
| 6.3660 | 2400 | 0.0001 | - |
| 6.4987 | 2450 | 0.0 | - |
| 6.6313 | 2500 | 0.0 | - |
| 6.7639 | 2550 | 0.0 | - |
| 6.8966 | 2600 | 0.0 | - |
| 7.0292 | 2650 | 0.0 | - |
| 7.1618 | 2700 | 0.0 | - |
| 7.2944 | 2750 | 0.0 | - |
| 7.4271 | 2800 | 0.0001 | - |
| 7.5597 | 2850 | 0.0 | - |
| 7.6923 | 2900 | 0.0 | - |
| 7.8249 | 2950 | 0.0 | - |
| 7.9576 | 3000 | 0.0 | - |
| 8.0902 | 3050 | 0.0 | - |
| 8.2228 | 3100 | 0.0 | - |
| 8.3554 | 3150 | 0.0 | - |
| 8.4881 | 3200 | 0.0001 | - |
| 8.6207 | 3250 | 0.0 | - |
| 8.7533 | 3300 | 0.0 | - |
| 8.8859 | 3350 | 0.0 | - |
| 9.0186 | 3400 | 0.0001 | - |
| 9.1512 | 3450 | 0.0 | - |
| 9.2838 | 3500 | 0.0 | - |
| 9.4164 | 3550 | 0.0001 | - |
| 9.5491 | 3600 | 0.0 | - |
| 9.6817 | 3650 | 0.0001 | - |
| 9.8143 | 3700 | 0.0 | - |
| 9.9469 | 3750 | 0.0001 | - |
### Framework Versions
- Python: 3.10.12
- SetFit: 1.0.1
- Sentence Transformers: 2.2.2
- Transformers: 4.35.2
- PyTorch: 2.1.0+cu118
- Datasets: 2.15.0
- Tokenizers: 0.15.0
## Citation
### BibTeX
```bibtex
@article{https://doi.org/10.48550/arxiv.2209.11055,
doi = {10.48550/ARXIV.2209.11055},
url = {https://arxiv.org/abs/2209.11055},
author = {Tunstall, Lewis and Reimers, Nils and Jo, Unso Eun Seo and Bates, Luke and Korat, Daniel and Wasserblat, Moshe and Pereg, Oren},
keywords = {Computation and Language (cs.CL), FOS: Computer and information sciences, FOS: Computer and information sciences},
title = {Efficient Few-Shot Learning Without Prompts},
publisher = {arXiv},
year = {2022},
copyright = {Creative Commons Attribution 4.0 International}
}
```
<!--
## Glossary
*Clearly define terms in order to be accessible across audiences.*
-->
<!--
## Model Card Authors
*Lists the people who create the model card, providing recognition and accountability for the detailed work that goes into its construction.*
-->
<!--
## Model Card Contact
*Provides a way for people who have updates to the Model Card, suggestions, or questions, to contact the Model Card authors.*
--> | [
"TEXT_CLASSIFICATION"
] | Non_BioNLP |
joshnielsen876/distilbert-base-uncased-finetuned-cola | joshnielsen876 | text-classification | [
"transformers",
"pytorch",
"tensorboard",
"distilbert",
"text-classification",
"generated_from_trainer",
"dataset:glue",
"license:apache-2.0",
"model-index",
"autotrain_compatible",
"endpoints_compatible",
"region:us"
] | 1,678,816,989,000 | 2023-03-14T18:14:14 | 10 | 0 | ---
datasets:
- glue
license: apache-2.0
metrics:
- matthews_correlation
tags:
- generated_from_trainer
model-index:
- name: distilbert-base-uncased-finetuned-cola
results:
- task:
type: text-classification
name: Text Classification
dataset:
name: glue
type: glue
config: cola
split: validation
args: cola
metrics:
- type: matthews_correlation
value: 0.5294395294021531
name: Matthews Correlation
---
<!-- This model card has been generated automatically according to the information the Trainer had access to. You
should probably proofread and complete it, then remove this comment. -->
# distilbert-base-uncased-finetuned-cola
This model is a fine-tuned version of [distilbert-base-uncased](https://huggingface.co/distilbert-base-uncased) on the glue dataset.
It achieves the following results on the evaluation set:
- Loss: 0.5703
- Matthews Correlation: 0.5294
## Model description
More information needed
## Intended uses & limitations
More information needed
## Training and evaluation data
More information needed
## Training procedure
### Training hyperparameters
The following hyperparameters were used during training:
- learning_rate: 2e-05
- train_batch_size: 16
- eval_batch_size: 16
- seed: 42
- optimizer: Adam with betas=(0.9,0.999) and epsilon=1e-08
- lr_scheduler_type: linear
- num_epochs: 5
### Training results
| Training Loss | Epoch | Step | Validation Loss | Matthews Correlation |
|:-------------:|:-----:|:----:|:---------------:|:--------------------:|
| 0.5256 | 1.0 | 535 | 0.5099 | 0.4384 |
| 0.3465 | 2.0 | 1070 | 0.4924 | 0.4952 |
| 0.2326 | 3.0 | 1605 | 0.5703 | 0.5294 |
| 0.1752 | 4.0 | 2140 | 0.7855 | 0.4936 |
| 0.1271 | 5.0 | 2675 | 0.8336 | 0.5242 |
### Framework versions
- Transformers 4.26.1
- Pytorch 1.13.1+cu116
- Datasets 2.10.1
- Tokenizers 0.13.2
| [
"TEXT_CLASSIFICATION"
] | Non_BioNLP |
arnolfokam/roberta-base-pcm | arnolfokam | token-classification | [
"transformers",
"pytorch",
"roberta",
"token-classification",
"NER",
"pcm",
"dataset:masakhaner",
"license:apache-2.0",
"autotrain_compatible",
"endpoints_compatible",
"region:us"
] | 1,646,263,745,000 | 2021-11-24T21:18:39 | 43 | 0 | ---
datasets:
- masakhaner
language:
- pcm
license: apache-2.0
metrics:
- f1
- precision
- recall
tags:
- NER
widget:
- text: Mixed Martial Arts joinbodi, Ultimate Fighting Championship, UFC don decide
say dem go enta back di octagon on Saturday, 9 May, for Jacksonville, Florida.
---
# Model description
**roberta-base-pcm** is a model based on the fine-tuned RoBERTa base model. It has been trained to recognize four types of entities:
- dates & time (DATE)
- Location (LOC)
- Organizations (ORG)
- Person (PER)
# Intended Use
- Intended to be used for research purposes concerning Named Entity Recognition for African Languages.
- Not intended for practical purposes.
# Training Data
This model was fine-tuned on the Nigerian Pidgin corpus **(pcm)** of the [MasakhaNER](https://github.com/masakhane-io/masakhane-ner) dataset. However, we thresholded the number of entity groups per sentence in this dataset to 10 entity groups.
# Training procedure
This model was trained on a single NVIDIA P5000 from [Paperspace](https://www.paperspace.com)
#### Hyperparameters
- **Learning Rate:** 5e-5
- **Batch Size:** 32
- **Maximum Sequence Length:** 164
- **Epochs:** 30
# Evaluation Data
We evaluated this model on the test split of the Swahili corpus **(pcm)** present in the [MasakhaNER](https://github.com/masakhane-io/masakhane-ner) with no thresholding.
# Metrics
- Precision
- Recall
- F1-score
# Limitations
- The size of the pre-trained language model prevents its usage in anything other than research.
- Lack of analysis concerning the bias and fairness in these models may make them dangerous if deployed into production system.
- The train data is a less populated version of the original dataset in terms of entity groups per sentence. Therefore, this can negatively impact the performance.
# Caveats and Recommendations
- The topics in the dataset corpus are centered around **News**. Future training could be done with a more diverse corpus.
# Results
Model Name| Precision | Recall | F1-score
-|-|-|-
**roberta-base-pcm**| 88.55 | 82.45 | 85.39
# Usage
```python
from transformers import AutoTokenizer, AutoModelForTokenClassification
from transformers import pipeline
tokenizer = AutoTokenizer.from_pretrained("arnolfokam/roberta-base-pcm")
model = AutoModelForTokenClassification.from_pretrained("arnolfokam/roberta-base-pcm")
nlp = pipeline("ner", model=model, tokenizer=tokenizer)
example = "Mixed Martial Arts joinbodi, Ultimate Fighting Championship, UFC don decide say dem go enta back di octagon on Saturday, 9 May, for Jacksonville, Florida."
ner_results = nlp(example)
print(ner_results)
``` | [
"NAMED_ENTITY_RECOGNITION"
] | Non_BioNLP |
lindeberg/tiny-bert-sst2-distilled | lindeberg | text-classification | [
"transformers",
"pytorch",
"bert",
"text-classification",
"generated_from_trainer",
"dataset:glue",
"base_model:google/bert_uncased_L-2_H-128_A-2",
"base_model:finetune:google/bert_uncased_L-2_H-128_A-2",
"license:apache-2.0",
"model-index",
"autotrain_compatible",
"endpoints_compatible",
"region:us"
] | 1,698,520,762,000 | 2023-10-28T20:33:38 | 125 | 0 | ---
base_model: google/bert_uncased_L-2_H-128_A-2
datasets:
- glue
license: apache-2.0
metrics:
- accuracy
tags:
- generated_from_trainer
model-index:
- name: tiny-bert-sst2-distilled
results:
- task:
type: text-classification
name: Text Classification
dataset:
name: glue
type: glue
config: sst2
split: validation
args: sst2
metrics:
- type: accuracy
value: 0.8038990825688074
name: Accuracy
---
<!-- This model card has been generated automatically according to the information the Trainer had access to. You
should probably proofread and complete it, then remove this comment. -->
# tiny-bert-sst2-distilled
This model is a fine-tuned version of [google/bert_uncased_L-2_H-128_A-2](https://huggingface.co/google/bert_uncased_L-2_H-128_A-2) on the glue dataset.
It achieves the following results on the evaluation set:
- Loss: 1.2916
- Accuracy: 0.8039
## Model description
More information needed
## Intended uses & limitations
More information needed
## Training and evaluation data
More information needed
## Training procedure
### Training hyperparameters
The following hyperparameters were used during training:
- learning_rate: 6.478952479475704e-05
- train_batch_size: 128
- eval_batch_size: 128
- seed: 33
- optimizer: Adam with betas=(0.9,0.999) and epsilon=1e-08
- lr_scheduler_type: linear
- num_epochs: 2
### Training results
| Training Loss | Epoch | Step | Validation Loss | Accuracy |
|:-------------:|:-----:|:----:|:---------------:|:--------:|
| 1.8025 | 1.0 | 527 | 1.3940 | 0.7901 |
| 1.1308 | 2.0 | 1054 | 1.2916 | 0.8039 |
### Framework versions
- Transformers 4.34.1
- Pytorch 2.1.0+cu118
- Datasets 2.14.6
- Tokenizers 0.14.1
| [
"TEXT_CLASSIFICATION"
] | Non_BioNLP |
arianpasquali/distilbert-base-uncased-finetuned-clinc | arianpasquali | text-classification | [
"transformers",
"pytorch",
"tensorboard",
"distilbert",
"text-classification",
"generated_from_trainer",
"dataset:clinc_oos",
"license:apache-2.0",
"model-index",
"autotrain_compatible",
"endpoints_compatible",
"region:us"
] | 1,646,263,745,000 | 2022-01-31T20:09:00 | 123 | 0 | ---
datasets:
- clinc_oos
license: apache-2.0
metrics:
- accuracy
tags:
- generated_from_trainer
model-index:
- name: distilbert-base-uncased-finetuned-clinc
results:
- task:
type: text-classification
name: Text Classification
dataset:
name: clinc_oos
type: clinc_oos
args: plus
metrics:
- type: accuracy
value: 0.9112903225806451
name: Accuracy
---
<!-- This model card has been generated automatically according to the information the Trainer had access to. You
should probably proofread and complete it, then remove this comment. -->
# distilbert-base-uncased-finetuned-clinc
This model is a fine-tuned version of [distilbert-base-uncased](https://huggingface.co/distilbert-base-uncased) on the clinc_oos dataset.
It achieves the following results on the evaluation set:
- Loss: 0.7751
- Accuracy: 0.9113
## Model description
More information needed
## Intended uses & limitations
More information needed
## Training and evaluation data
More information needed
## Training procedure
### Training hyperparameters
The following hyperparameters were used during training:
- learning_rate: 2e-05
- train_batch_size: 48
- eval_batch_size: 48
- seed: 42
- optimizer: Adam with betas=(0.9,0.999) and epsilon=1e-08
- lr_scheduler_type: linear
- num_epochs: 5
### Training results
| Training Loss | Epoch | Step | Validation Loss | Accuracy |
|:-------------:|:-----:|:----:|:---------------:|:--------:|
| 4.315 | 1.0 | 318 | 3.3087 | 0.74 |
| 2.6371 | 2.0 | 636 | 1.8833 | 0.8381 |
| 1.5388 | 3.0 | 954 | 1.1547 | 0.8929 |
| 1.0076 | 4.0 | 1272 | 0.8590 | 0.9071 |
| 0.79 | 5.0 | 1590 | 0.7751 | 0.9113 |
### Framework versions
- Transformers 4.11.3
- Pytorch 1.7.1
- Datasets 1.16.1
- Tokenizers 0.10.3
| [
"TEXT_CLASSIFICATION"
] | Non_BioNLP |
RichardErkhov/4bit_-_gemma-2b-8bits | RichardErkhov | null | [
"safetensors",
"gemma",
"arxiv:2312.11805",
"arxiv:2009.03300",
"arxiv:1905.07830",
"arxiv:1911.11641",
"arxiv:1904.09728",
"arxiv:1905.10044",
"arxiv:1907.10641",
"arxiv:1811.00937",
"arxiv:1809.02789",
"arxiv:1911.01547",
"arxiv:1705.03551",
"arxiv:2107.03374",
"arxiv:2108.07732",
"arxiv:2110.14168",
"arxiv:2304.06364",
"arxiv:2206.04615",
"arxiv:1804.06876",
"arxiv:2110.08193",
"arxiv:2009.11462",
"arxiv:2101.11718",
"arxiv:1804.09301",
"arxiv:2109.07958",
"arxiv:2203.09509",
"8-bit",
"bitsandbytes",
"region:us"
] | 1,729,271,943,000 | 2024-10-18T17:21:08 | 4 | 0 | ---
{}
---
Quantization made by Richard Erkhov.
[Github](https://github.com/RichardErkhov)
[Discord](https://discord.gg/pvy7H8DZMG)
[Request more models](https://github.com/RichardErkhov/quant_request)
gemma-2b - bnb 8bits
- Model creator: https://huggingface.co/4bit/
- Original model: https://huggingface.co/4bit/gemma-2b/
Original model description:
---
library_name: transformers
tags: []
extra_gated_heading: "Access Gemma on Hugging Face"
extra_gated_prompt: "To access Gemma on Hugging Face, you’re required to review and agree to Google’s usage license. To do this, please ensure you’re logged-in to Hugging Face and click below. Requests are processed immediately."
extra_gated_button_content: "Acknowledge license"
---
# Gemma Model Card
**Model Page**: [Gemma](https://ai.google.dev/gemma/docs)
This model card corresponds to the 2B base version of the Gemma model. You can also visit the model card of the [7B base model](https://huggingface.co/google/gemma-7b), [7B instruct model](https://huggingface.co/google/gemma-7b-it), and [2B instruct model](https://huggingface.co/google/gemma-2b-it).
**Resources and Technical Documentation**:
* [Responsible Generative AI Toolkit](https://ai.google.dev/responsible)
* [Gemma on Kaggle](https://www.kaggle.com/models/google/gemma)
* [Gemma on Vertex Model Garden](https://console.cloud.google.com/vertex-ai/publishers/google/model-garden/335)
**Terms of Use**: [Terms](https://www.kaggle.com/models/google/gemma/license/consent)
**Authors**: Google
## Model Information
Summary description and brief definition of inputs and outputs.
### Description
Gemma is a family of lightweight, state-of-the-art open models from Google,
built from the same research and technology used to create the Gemini models.
They are text-to-text, decoder-only large language models, available in English,
with open weights, pre-trained variants, and instruction-tuned variants. Gemma
models are well-suited for a variety of text generation tasks, including
question answering, summarization, and reasoning. Their relatively small size
makes it possible to deploy them in environments with limited resources such as
a laptop, desktop or your own cloud infrastructure, democratizing access to
state of the art AI models and helping foster innovation for everyone.
### Usage
Below we share some code snippets on how to get quickly started with running the model. First make sure to `pip install -U transformers`, then copy the snippet from the section that is relevant for your usecase.
#### Fine-tuning the model
You can find fine-tuning scripts and notebook under the [`examples/` directory](https://huggingface.co/google/gemma-7b/tree/main/examples) of [`google/gemma-7b`](https://huggingface.co/google/gemma-7b) repository. To adapt it to this model, simply change the model-id to `google/gemma-2b`.
In that repository, we provide:
* A script to perform Supervised Fine-Tuning (SFT) on UltraChat dataset using QLoRA
* A script to perform SFT using FSDP on TPU devices
* A notebook that you can run on a free-tier Google Colab instance to perform SFT on English quotes dataset
#### Running the model on a CPU
```python
from transformers import AutoTokenizer, AutoModelForCausalLM
tokenizer = AutoTokenizer.from_pretrained("google/gemma-2b")
model = AutoModelForCausalLM.from_pretrained("google/gemma-2b")
input_text = "Write me a poem about Machine Learning."
input_ids = tokenizer(**input_text, return_tensors="pt")
outputs = model.generate(input_ids)
print(tokenizer.decode(outputs[0]))
```
#### Running the model on a single / multi GPU
```python
# pip install accelerate
from transformers import AutoTokenizer, AutoModelForCausalLM
tokenizer = AutoTokenizer.from_pretrained("google/gemma-2b")
model = AutoModelForCausalLM.from_pretrained("google/gemma-2b", device_map="auto")
input_text = "Write me a poem about Machine Learning."
input_ids = tokenizer(input_text, return_tensors="pt").to("cuda")
outputs = model.generate(**input_ids)
print(tokenizer.decode(outputs[0]))
```
#### Running the model on a GPU using different precisions
* _Using `torch.float16`_
```python
# pip install accelerate
from transformers import AutoTokenizer, AutoModelForCausalLM
tokenizer = AutoTokenizer.from_pretrained("google/gemma-2b")
model = AutoModelForCausalLM.from_pretrained("google/gemma-2b", device_map="auto", torch_dtype=torch.float16)
input_text = "Write me a poem about Machine Learning."
input_ids = tokenizer(input_text, return_tensors="pt").to("cuda")
outputs = model.generate(**input_ids)
print(tokenizer.decode(outputs[0]))
```
* _Using `torch.bfloat16`_
```python
# pip install accelerate
from transformers import AutoTokenizer, AutoModelForCausalLM
tokenizer = AutoTokenizer.from_pretrained("google/gemma-2b")
model = AutoModelForCausalLM.from_pretrained("google/gemma-2b", device_map="auto", torch_dtype=torch.bfloat16)
input_text = "Write me a poem about Machine Learning."
input_ids = tokenizer(input_text, return_tensors="pt").to("cuda")
outputs = model.generate(**input_ids)
print(tokenizer.decode(outputs[0]))
```
#### Quantized Versions through `bitsandbytes`
* _Using 8-bit precision (int8)_
```python
# pip install bitsandbytes accelerate
from transformers import AutoTokenizer, AutoModelForCausalLM, BitsAndBytesConfig
quantization_config = BitsAndBytesConfig(load_in_8bit=True)
tokenizer = AutoTokenizer.from_pretrained("google/gemma-2b")
model = AutoModelForCausalLM.from_pretrained("google/gemma-2b", quantization_config=quantization_config)
input_text = "Write me a poem about Machine Learning."
input_ids = tokenizer(input_text, return_tensors="pt").to("cuda")
outputs = model.generate(**input_ids)
print(tokenizer.decode(outputs[0]))
```
* _Using 4-bit precision_
```python
# pip install bitsandbytes accelerate
from transformers import AutoTokenizer, AutoModelForCausalLM, BitsAndBytesConfig
quantization_config = BitsAndBytesConfig(load_in_4bit=True)
tokenizer = AutoTokenizer.from_pretrained("google/gemma-2b")
model = AutoModelForCausalLM.from_pretrained("google/gemma-2b", quantization_config=quantization_config)
input_text = "Write me a poem about Machine Learning."
input_ids = tokenizer(input_text, return_tensors="pt").to("cuda")
outputs = model.generate(**input_ids)
print(tokenizer.decode(outputs[0]))
```
#### Other optimizations
* _Flash Attention 2_
First make sure to install `flash-attn` in your environment `pip install flash-attn`
```diff
model = AutoModelForCausalLM.from_pretrained(
model_id,
torch_dtype=torch.float16,
+ attn_implementation="flash_attention_2"
).to(0)
```
### Inputs and outputs
* **Input:** Text string, such as a question, a prompt, or a document to be
summarized.
* **Output:** Generated English-language text in response to the input, such
as an answer to a question, or a summary of a document.
## Model Data
Data used for model training and how the data was processed.
### Training Dataset
These models were trained on a dataset of text data that includes a wide variety
of sources, totaling 6 trillion tokens. Here are the key components:
* Web Documents: A diverse collection of web text ensures the model is exposed
to a broad range of linguistic styles, topics, and vocabulary. Primarily
English-language content.
* Code: Exposing the model to code helps it to learn the syntax and patterns of
programming languages, which improves its ability to generate code or
understand code-related questions.
* Mathematics: Training on mathematical text helps the model learn logical
reasoning, symbolic representation, and to address mathematical queries.
The combination of these diverse data sources is crucial for training a powerful
language model that can handle a wide variety of different tasks and text
formats.
### Data Preprocessing
Here are the key data cleaning and filtering methods applied to the training
data:
* CSAM Filtering: Rigorous CSAM (Child Sexual Abuse Material) filtering was
applied at multiple stages in the data preparation process to ensure the
exclusion of harmful and illegal content
* Sensitive Data Filtering: As part of making Gemma pre-trained models safe and
reliable, automated techniques were used to filter out certain personal
information and other sensitive data from training sets.
* Additional methods: Filtering based on content quality and safely in line with
[our policies](https://storage.googleapis.com/gweb-uniblog-publish-prod/documents/2023_Google_AI_Principles_Progress_Update.pdf#page=11).
## Implementation Information
Details about the model internals.
### Hardware
Gemma was trained using the latest generation of
[Tensor Processing Unit (TPU)](https://cloud.google.com/tpu/docs/intro-to-tpu) hardware (TPUv5e).
Training large language models requires significant computational power. TPUs,
designed specifically for matrix operations common in machine learning, offer
several advantages in this domain:
* Performance: TPUs are specifically designed to handle the massive computations
involved in training LLMs. They can speed up training considerably compared to
CPUs.
* Memory: TPUs often come with large amounts of high-bandwidth memory, allowing
for the handling of large models and batch sizes during training. This can
lead to better model quality.
* Scalability: TPU Pods (large clusters of TPUs) provide a scalable solution for
handling the growing complexity of large foundation models. You can distribute
training across multiple TPU devices for faster and more efficient processing.
* Cost-effectiveness: In many scenarios, TPUs can provide a more cost-effective
solution for training large models compared to CPU-based infrastructure,
especially when considering the time and resources saved due to faster
training.
* These advantages are aligned with
[Google's commitments to operate sustainably](https://sustainability.google/operating-sustainably/).
### Software
Training was done using [JAX](https://github.com/google/jax) and [ML Pathways](https://blog.google/technology/ai/introducing-pathways-next-generation-ai-architecture/ml-pathways).
JAX allows researchers to take advantage of the latest generation of hardware,
including TPUs, for faster and more efficient training of large models.
ML Pathways is Google's latest effort to build artificially intelligent systems
capable of generalizing across multiple tasks. This is specially suitable for
[foundation models](https://ai.google/discover/foundation-models/), including large language models like
these ones.
Together, JAX and ML Pathways are used as described in the
[paper about the Gemini family of models](https://arxiv.org/abs/2312.11805); "the 'single
controller' programming model of Jax and Pathways allows a single Python
process to orchestrate the entire training run, dramatically simplifying the
development workflow."
## Evaluation
Model evaluation metrics and results.
### Benchmark Results
These models were evaluated against a large collection of different datasets and
metrics to cover different aspects of text generation:
| Benchmark | Metric | 2B Params | 7B Params |
| ------------------------------ | ------------- | ----------- | --------- |
| [MMLU](https://arxiv.org/abs/2009.03300) | 5-shot, top-1 | 42.3 | 64.3 |
| [HellaSwag](https://arxiv.org/abs/1905.07830) | 0-shot |71.4 | 81.2 |
| [PIQA](https://arxiv.org/abs/1911.11641) | 0-shot | 77.3 | 81.2 |
| [SocialIQA](https://arxiv.org/abs/1904.09728) | 0-shot | 59.7 | 51.8 |
| [BooIQ](https://arxiv.org/abs/1905.10044) | 0-shot | 69.4 | 83.2 |
| [WinoGrande](https://arxiv.org/abs/1907.10641) | partial score | 65.4 | 72.3 |
| [CommonsenseQA](https://arxiv.org/abs/1811.00937) | 7-shot | 65.3 | 71.3 |
| [OpenBookQA](https://arxiv.org/abs/1809.02789) | | 47.8 | 52.8 |
| [ARC-e](https://arxiv.org/abs/1911.01547) | | 73.2 | 81.5 |
| [ARC-c](https://arxiv.org/abs/1911.01547) | | 42.1 | 53.2 |
| [TriviaQA](https://arxiv.org/abs/1705.03551) | 5-shot | 53.2 | 63.4 |
| [Natural Questions](https://github.com/google-research-datasets/natural-questions) | 5-shot | - | 23 |
| [HumanEval](https://arxiv.org/abs/2107.03374) | pass@1 | 22.0 | 32.3 |
| [MBPP](https://arxiv.org/abs/2108.07732) | 3-shot | 29.2 | 44.4 |
| [GSM8K](https://arxiv.org/abs/2110.14168) | maj@1 | 17.7 | 46.4 |
| [MATH](https://arxiv.org/abs/2108.07732) | 4-shot | 11.8 | 24.3 |
| [AGIEval](https://arxiv.org/abs/2304.06364) | | 24.2 | 41.7 |
| [BIG-Bench](https://arxiv.org/abs/2206.04615) | | 35.2 | 55.1 |
| ------------------------------ | ------------- | ----------- | --------- |
| **Average** | | **54.0** | **56.4** |
## Ethics and Safety
Ethics and safety evaluation approach and results.
### Evaluation Approach
Our evaluation methods include structured evaluations and internal red-teaming
testing of relevant content policies. Red-teaming was conducted by a number of
different teams, each with different goals and human evaluation metrics. These
models were evaluated against a number of different categories relevant to
ethics and safety, including:
* Text-to-Text Content Safety: Human evaluation on prompts covering safety
policies including child sexual abuse and exploitation, harassment, violence
and gore, and hate speech.
* Text-to-Text Representational Harms: Benchmark against relevant academic
datasets such as [WinoBias](https://arxiv.org/abs/1804.06876) and [BBQ Dataset](https://arxiv.org/abs/2110.08193v2).
* Memorization: Automated evaluation of memorization of training data, including
the risk of personally identifiable information exposure.
* Large-scale harm: Tests for "dangerous capabilities," such as chemical,
biological, radiological, and nuclear (CBRN) risks.
### Evaluation Results
The results of ethics and safety evaluations are within acceptable thresholds
for meeting [internal policies](https://storage.googleapis.com/gweb-uniblog-publish-prod/documents/2023_Google_AI_Principles_Progress_Update.pdf#page=11) for categories such as child
safety, content safety, representational harms, memorization, large-scale harms.
On top of robust internal evaluations, the results of well known safety
benchmarks like BBQ, BOLD, Winogender, Winobias, RealToxicity, and TruthfulQA
are shown here.
| Benchmark | Metric | 2B Params | 7B Params |
| ------------------------------ | ------------- | ----------- | --------- |
| [RealToxicity](https://arxiv.org/abs/2009.11462) | average | 6.86 | 7.90 |
| [BOLD](https://arxiv.org/abs/2101.11718) | | 45.57 | 49.08 |
| [CrowS-Pairs](https://aclanthology.org/2020.emnlp-main.154/) | top-1 | 45.82 | 51.33 |
| [BBQ Ambig](https://arxiv.org/abs/2110.08193v2) | 1-shot, top-1 | 62.58 | 92.54 |
| [BBQ Disambig](https://arxiv.org/abs/2110.08193v2) | top-1 | 54.62 | 71.99 |
| [Winogender](https://arxiv.org/abs/1804.09301) | top-1 | 51.25 | 54.17 |
| [TruthfulQA](https://arxiv.org/abs/2109.07958) | | 44.84 | 31.81 |
| [Winobias 1_2](https://arxiv.org/abs/1804.06876) | | 56.12 | 59.09 |
| [Winobias 2_2](https://arxiv.org/abs/1804.06876) | | 91.10 | 92.23 |
| [Toxigen](https://arxiv.org/abs/2203.09509) | | 29.77 | 39.59 |
| ------------------------------ | ------------- | ----------- | --------- |
## Usage and Limitations
These models have certain limitations that users should be aware of.
### Intended Usage
Open Large Language Models (LLMs) have a wide range of applications across
various industries and domains. The following list of potential uses is not
comprehensive. The purpose of this list is to provide contextual information
about the possible use-cases that the model creators considered as part of model
training and development.
* Content Creation and Communication
* Text Generation: These models can be used to generate creative text formats
such as poems, scripts, code, marketing copy, and email drafts.
* Chatbots and Conversational AI: Power conversational interfaces for customer
service, virtual assistants, or interactive applications.
* Text Summarization: Generate concise summaries of a text corpus, research
papers, or reports.
* Research and Education
* Natural Language Processing (NLP) Research: These models can serve as a
foundation for researchers to experiment with NLP techniques, develop
algorithms, and contribute to the advancement of the field.
* Language Learning Tools: Support interactive language learning experiences,
aiding in grammar correction or providing writing practice.
* Knowledge Exploration: Assist researchers in exploring large bodies of text
by generating summaries or answering questions about specific topics.
### Limitations
* Training Data
* The quality and diversity of the training data significantly influence the
model's capabilities. Biases or gaps in the training data can lead to
limitations in the model's responses.
* The scope of the training dataset determines the subject areas the model can
handle effectively.
* Context and Task Complexity
* LLMs are better at tasks that can be framed with clear prompts and
instructions. Open-ended or highly complex tasks might be challenging.
* A model's performance can be influenced by the amount of context provided
(longer context generally leads to better outputs, up to a certain point).
* Language Ambiguity and Nuance
* Natural language is inherently complex. LLMs might struggle to grasp subtle
nuances, sarcasm, or figurative language.
* Factual Accuracy
* LLMs generate responses based on information they learned from their
training datasets, but they are not knowledge bases. They may generate
incorrect or outdated factual statements.
* Common Sense
* LLMs rely on statistical patterns in language. They might lack the ability
to apply common sense reasoning in certain situations.
### Ethical Considerations and Risks
The development of large language models (LLMs) raises several ethical concerns.
In creating an open model, we have carefully considered the following:
* Bias and Fairness
* LLMs trained on large-scale, real-world text data can reflect socio-cultural
biases embedded in the training material. These models underwent careful
scrutiny, input data pre-processing described and posterior evaluations
reported in this card.
* Misinformation and Misuse
* LLMs can be misused to generate text that is false, misleading, or harmful.
* Guidelines are provided for responsible use with the model, see the
[Responsible Generative AI Toolkit](http://ai.google.dev/gemma/responsible).
* Transparency and Accountability:
* This model card summarizes details on the models' architecture,
capabilities, limitations, and evaluation processes.
* A responsibly developed open model offers the opportunity to share
innovation by making LLM technology accessible to developers and researchers
across the AI ecosystem.
Risks identified and mitigations:
* Perpetuation of biases: It's encouraged to perform continuous monitoring
(using evaluation metrics, human review) and the exploration of de-biasing
techniques during model training, fine-tuning, and other use cases.
* Generation of harmful content: Mechanisms and guidelines for content safety
are essential. Developers are encouraged to exercise caution and implement
appropriate content safety safeguards based on their specific product policies
and application use cases.
* Misuse for malicious purposes: Technical limitations and developer and
end-user education can help mitigate against malicious applications of LLMs.
Educational resources and reporting mechanisms for users to flag misuse are
provided. Prohibited uses of Gemma models are outlined in the
[Gemma Prohibited Use Policy](https://ai.google.dev/gemma/prohibited_use_policy).
* Privacy violations: Models were trained on data filtered for removal of PII
(Personally Identifiable Information). Developers are encouraged to adhere to
privacy regulations with privacy-preserving techniques.
### Benefits
At the time of release, this family of models provides high-performance open
large language model implementations designed from the ground up for Responsible
AI development compared to similarly sized models.
Using the benchmark evaluation metrics described in this document, these models
have shown to provide superior performance to other, comparably-sized open model
alternatives.
| [
"QUESTION_ANSWERING",
"SUMMARIZATION"
] | Non_BioNLP |
guishe/nuner-v2_fewnerd_fine_super | guishe | token-classification | [
"transformers",
"safetensors",
"roberta",
"token-classification",
"ner",
"named-entity-recognition",
"en",
"dataset:DFKI-SLT/few-nerd",
"arxiv:2402.15343",
"base_model:numind/NuNER-v2.0",
"base_model:finetune:numind/NuNER-v2.0",
"license:cc-by-sa-4.0",
"model-index",
"autotrain_compatible",
"endpoints_compatible",
"region:us"
] | 1,716,543,663,000 | 2024-05-24T16:00:38 | 27 | 0 | ---
base_model: numind/NuNER-v2.0
datasets:
- DFKI-SLT/few-nerd
language: en
license: cc-by-sa-4.0
metrics:
- precision
- recall
- f1
pipeline_tag: token-classification
tags:
- token-classification
- ner
- named-entity-recognition
widget:
- text: Concern and scepticism surround Niger uranium mining waste storage plans.
Towering mounds dot the desert landscape in northern Niger's Arlit region, but
they are heaps of partially radioactive waste left from four decades of operations
at one of the world's biggest uranium mines. An ambitious 10-year scheme costing
$160 million is underway to secure the waste and avoid risks to health and the
environment, but many local people are worried or sceptical. France's nuclear
giant Areva, now called Orano, worked the area under a subsidiary, the Akouta
Mining Company (Cominak). Cominak closed the site in 2021 after extracting 75,000
tonnes of uranium, much of which went to fuelling the scores of nuclear reactors
that provide the backbone of France's electricity supply. Cominak's director general
Mahaman Sani Abdoulaye showcased the rehabilitation project to the first French
journalists to visit the site since 2010, when seven Areva employees were kidnapped
by jihadists.
- text: SE Michigan counties allege insulin gouging; Localities file lawsuit against
pharmaceutical makers. Four metro Detroit counties filed federal lawsuits Wednesday
against some of the nation's biggest pharmaceutical manufacturers and pharmacy
benefit managers alleging illegal price fixing for insulin products. Macomb, Monroe,
Wayne and Washtenaw counties filed the lawsuits in U.S. District Court in New
Jersey against more than a dozen companies, including Lilly, Sanofi Aventis, Novo
Nordisk, Express Scripts, Optum Rx and CVS Caremark, per their attorneys. "These
are the first such lawsuits that have been filed in the state of Michigan and
probably more to come," said attorney Melvin Butch Hollowell of the Miller Law
Firm. He described the allegations during a news conference, saying that nationally
"the pharmacies and manufacturers get together. They control about 90% of the
market each, of the insulin market. They talk to each other secretly. And they
jack up the prices through anticompetitive means. And what we've seen is over
the past 20 years, when we talk about jacking up the prices, they jack them up
1,500% in the last 20 years. 1,500%."
- text: Foreign governments may be spying on your smartphone notifications, senator
says. Washington (CNN) — Foreign governments have reportedly attempted to spy
on iPhone and Android users through the mobile app notifications they receive
on their smartphones - and the US government has forced Apple and Google to keep
quiet about it, according to a top US senator. Through legal demands sent to the
tech giants, governments have allegedly tried to force Apple and Google to turn
over sensitive information that could include the contents of a notification -
such as previews of a text message displayed on a lock screen, or an update about
app activity, Oregon Democratic Sen. Ron Wyden said in a new report. Wyden's report
reflects the latest example of long-running tensions between tech companies and
governments over law enforcement demands, which have stretched on for more than
a decade. Governments around the world have particularly battled with tech companies
over encryption, which provides critical protections to users and businesses while
in some cases preventing law enforcement from pursuing investigations into messages
sent over the internet.
- text: Tech giants ‘could severely disable UK spooks from stopping online harms’.
Silicon Valley tech giants’ actions could “severely disable” UK spooks from preventing
harm caused by online paedophiles and fraudsters, Suella Braverman has suggested.
The Conservative former home secretary named Facebook owner Meta , and Apple,
and their use of technologies such as end-to-end encryption as a threat to attempts
to tackle digital crimes. She claimed the choice to back these technologies without
“safeguards” could “enable and indeed facilitate some of the worst atrocities
that our brave men and women in law enforcement agencies deal with every day”,
as MPs began considering changes to investigatory powers laws. The Investigatory
Powers (Amendment) Bill includes measures to make it easier for agencies to examine
and retain bulk datasets, such as publicly available online telephone records,
and would allow intelligence agencies to use internet connection records to aid
detection of their targets. We know that the terrorists, the serious organised
criminals, and fraudsters, and the online paedophiles, all take advantage of the
dark web and encrypted spaces
- text: Camargo Corrêa asks Toffoli to suspend the fine agreed with Lava Jato. The
Camargo Corrêa group has asked Justice Dias Toffoli to suspend the R$1.4 billion
fine it agreed to pay in its leniency agreement under Operation Car Wash. The
company asked for an extension of the minister's decisions that benefited J&F
and Odebrecht. Like the other companies, it claimed that it suffered undue pressure
from members of the Federal Public Prosecutor's Office (MPF) to close the deal.
Much of the request is based on messages exchanged between prosecutors from the
Curitiba task force and former judge Sergio Moro - Camargo Corrêa requested full
access to the material, seized in Operation Spoofing, which arrested the hackers
who broke into cell phones. The dialogues, according to the group's defense, indicate
that the executives did not freely agree to the deal, since they were the targets
of lawsuits and pre-trial detentions.
inference:
parameters:
aggregation_strategy: simple
model-index:
- name: numind/NuNER-v2.0 fine-tuned on FewNERD-fine-supervised
results:
- task:
type: token-classification
name: Named Entity Recognition
dataset:
name: FewNERD
type: DFKI-SLT/few-nerd
split: eval
metrics:
- type: f1
value: 0.691212893048585
name: F1
- type: precision
value: 0.6733880835152357
name: Precision
- type: recall
value: 0.7100070181232713
name: Recall
---
# numind/NuNER-v2.0 fine-tuned on FewNERD-fine-supervised
This is a [NuNER](https://arxiv.org/abs/2402.15343) model fine-tuned on the [FewNERD](https://huggingface.co/datasets/DFKI-SLT/few-nerd) dataset that can be used for Named Entity Recognition. NuNER model uses [RoBERTa-base](https://huggingface.co/FacebookAI/roberta-base) as the backbone encoder and it was trained on the [NuNER dataset](https://huggingface.co/datasets/numind/NuNER), which is a large and diverse dataset synthetically labeled by gpt-3.5-turbo-0301 of 1M sentences. This further pre-training phase allowed the generation of high quality token embeddings, a good starting point for fine-tuning on more specialized datasets.
## Model Details
The model was fine-tuned as a regular BERT-based model for NER task using HuggingFace Trainer class.
### Model Labels
| Label | Examples |
|:-----------------------------------------|:---------------------------------------------------------------------------------------------------------|
| art_broadcastprogram | "Corazones", "The Gale Storm Show : Oh , Susanna", "Street Cents" |
| art_film | "Shawshank Redemption", "L'Atlantide", "Bosch" |
| art_music | "Hollywood Studio Symphony", "Atkinson , Danko and Ford ( with Brockie and Hilton )", "Champion Lover" |
| art_other | "The Today Show", "Venus de Milo", "Aphrodite of Milos" |
| art_painting | "Production/Reproduction", "Touit", "Cofiwch Dryweryn" |
| art_writtenart | "The Seven Year Itch", "Imelda de ' Lambertazzi", "Time" |
| building_airport | "Sheremetyevo International Airport", "Newark Liberty International Airport", "Luton Airport" |
| building_hospital | "Yeungnam University Hospital", "Hokkaido University Hospital", "Memorial Sloan-Kettering Cancer Center" |
| building_hotel | "The Standard Hotel", "Flamingo Hotel", "Radisson Blu Sea Plaza Hotel" |
| building_library | "British Library", "Bayerische Staatsbibliothek", "Berlin State Library" |
| building_other | "Henry Ford Museum", "Alpha Recording Studios", "Communiplex" |
| building_restaurant | "Carnegie Deli", "Fatburger", "Trumbull" |
| building_sportsfacility | "Boston Garden", "Sports Center", "Glenn Warner Soccer Facility" |
| building_theater | "Sanders Theatre", "National Paris Opera", "Pittsburgh Civic Light Opera" |
| event_attack/battle/war/militaryconflict | "Easter Offensive", "Jurist", "Vietnam War" |
| event_disaster | "the 1912 North Mount Lyell Disaster", "1990s North Korean famine", "1693 Sicily earthquake" |
| event_election | "Elections to the European Parliament", "March 1898 elections", "1982 Mitcham and Morden by-election" |
| event_other | "Union for a Popular Movement", "Masaryk Democratic Movement", "Eastwood Scoring Stage" |
| event_protest | "Iranian Constitutional Revolution", "French Revolution", "Russian Revolution" |
| event_sportsevent | "World Cup", "National Champions", "Stanley Cup" |
| location_GPE | "Croatian", "Mediterranean Basin", "the Republic of Croatia" |
| location_bodiesofwater | "Arthur Kill", "Atatürk Dam Lake", "Norfolk coast" |
| location_island | "new Samsat district", "Laccadives", "Staten Island" |
| location_mountain | "Salamander Glacier", "Miteirya Ridge", "Ruweisat Ridge" |
| location_other | "Victoria line", "Northern City Line", "Cartuther" |
| location_park | "Painted Desert Community Complex Historic District", "Gramercy Park", "Shenandoah National Park" |
| location_road/railway/highway/transit | "NJT", "Newark-Elizabeth Rail Link", "Friern Barnet Road" |
| organization_company | "Texas Chicken", "Dixy Chicken", "Church 's Chicken" |
| organization_education | "MIT", "Belfast Royal Academy and the Ulster College of Physical Education", "Barnard College" |
| organization_government/governmentagency | "Congregazione dei Nobili", "Diet", "Supreme Court" |
| organization_media/newspaper | "Clash", "Al Jazeera", "TimeOut Melbourne" |
| organization_other | "Defence Sector C", "IAEA", "4th Army" |
| organization_politicalparty | "Al Wafa ' Islamic", "Shimpotō", "Kenseitō" |
| organization_religion | "UPCUSA", "Christian", "Jewish" |
| organization_showorganization | "Lizzy", "Bochumer Symphoniker", "Mr. Mister" |
| organization_sportsleague | "China League One", "NHL", "First Division" |
| organization_sportsteam | "Arsenal", "Luc Alphand Aventures", "Tottenham" |
| other_astronomything | "Algol", "`` Caput Larvae ''", "Zodiac" |
| other_award | "Order of the Republic of Guinea and Nigeria", "Grand Commander of the Order of the Niger", "GCON" |
| other_biologything | "N-terminal lipid", "Amphiphysin", "BAR" |
| other_chemicalthing | "uranium", "carbon dioxide", "sulfur" |
| other_currency | "$", "lac crore", "Travancore Rupee" |
| other_disease | "bladder cancer", "French Dysentery Epidemic of 1779", "hypothyroidism" |
| other_educationaldegree | "BSc ( Hons ) in physics", "Bachelor", "Master" |
| other_god | "Raijin", "Fujin", "El" |
| other_language | "Breton-speaking", "Latin", "English" |
| other_law | "Leahy–Smith America Invents Act ( AIA", "United States Freedom Support Act", "Thirty Years ' Peace" |
| other_livingthing | "monkeys", "patchouli", "insects" |
| other_medical | "amitriptyline", "Pediatrics", "pediatrician" |
| person_actor | "Tchéky Karyo", "Edmund Payne", "Ellaline Terriss" |
| person_artist/author | "Hicks", "Gaetano Donizett", "George Axelrod" |
| person_athlete | "Tozawa", "Neville", "Jaguar" |
| person_director | "Richard Quine", "Bob Swaim", "Frank Darabont" |
| person_other | "Campbell", "Holden", "Richard Benson" |
| person_politician | "William", "Rivière", "Emeric" |
| person_scholar | "Wurdack", "Stalmine", "Stedman" |
| person_soldier | "Joachim Ziegler", "Helmuth Weidling", "Krukenberg" |
| product_airplane | "Spey-equipped FGR.2s", "EC135T2 CPDS", "Luton" |
| product_car | "Phantom", "100EX", "Corvettes - GT1 C6R" |
| product_food | "red grape", "yakiniku", "V. labrusca" |
| product_game | "Hardcore RPG", "Splinter Cell", "Airforce Delta" |
| product_other | "X11", "PDP-1", "Fairbottom Bobs" |
| product_ship | "Essex", "Congress", "HMS `` Chinkara ''" |
| product_software | "AmiPDF", "Wikipedia", "Apdf" |
| product_train | "55022", "Royal Scots Grey", "High Speed Trains" |
| product_weapon | "AR-15 's", "ZU-23-2MR Wróbel II", "ZU-23-2M Wróbel" |
## Uses
### Direct Use for Inference
```python
>>> from transformers import pipeline
>>> text = """Foreign governments may be spying on your smartphone notifications, senator says. Washington (CNN) — Foreign governments have reportedly attempted to spy on iPhone and Android users through the mobile app notifications they receive on their smartphones - and the US government has forced Apple and Google to keep quiet about it, according to a top US senator. Through legal demands sent to the tech giants, governments have allegedly tried to force Apple and Google to turn over sensitive information that could include the contents of a notification - such as previews of a text message displayed on a lock screen, or an update about app activity, Oregon Democratic Sen. Ron Wyden said in a new report. Wyden's report reflects the latest example of long-running tensions between tech companies and governments over law enforcement demands, which have stretched on for more than a decade. Governments around the world have particularly battled with tech companies over encryption, which provides critical protections to users and businesses while in some cases preventing law enforcement from pursuing investigations into messages sent over the internet."""
>>> classifier = pipeline(
"ner",
model="guishe/nuner-v2_fewnerd_fine_super",
aggregation_strategy="simple",
)
>>> classifier(text)
[{'entity_group': 'location_GPE',
'score': 0.96503985,
'word': ' Washington',
'start': 82,
'end': 92},
{'entity_group': 'organization_media/newspaper',
'score': 0.89006454,
'word': 'CNN',
'start': 94,
'end': 97},
{'entity_group': 'product_other',
'score': 0.86745757,
'word': ' iPhone',
'start': 157,
'end': 163},
{'entity_group': 'product_other',
'score': 0.6874236,
'word': ' Android',
'start': 168,
'end': 175},
{'entity_group': 'location_GPE',
'score': 0.87520945,
'word': ' US',
'start': 263,
'end': 265},
{'entity_group': 'organization_company',
'score': 0.96546257,
'word': ' Apple',
'start': 288,
'end': 293},
{'entity_group': 'organization_company',
'score': 0.9558688,
'word': ' Google',
'start': 298,
'end': 304},
{'entity_group': 'location_GPE',
'score': 0.97874,
'word': ' US',
'start': 348,
'end': 350},
{'entity_group': 'organization_company',
'score': 0.963951,
'word': ' Apple',
'start': 449,
'end': 454},
{'entity_group': 'organization_company',
'score': 0.9558847,
'word': ' Google',
'start': 459,
'end': 465},
{'entity_group': 'location_GPE',
'score': 0.7581249,
'word': ' Oregon',
'start': 649,
'end': 655},
{'entity_group': 'organization_politicalparty',
'score': 0.7324057,
'word': ' Democratic',
'start': 656,
'end': 666},
{'entity_group': 'person_politician',
'score': 0.86246103,
'word': ' Ron Wyden',
'start': 672,
'end': 681},
{'entity_group': 'person_politician',
'score': 0.7916358,
'word': ' Wyden',
'start': 704,
'end': 709}]
```
## Training Details
### Training Set Metrics
| Training set | Min | Median | Max |
|:----------------------|:----|:--------|:----|
| Sentence length | 1 | 24.4945 | 267 |
| Entities per sentence | 0 | 2.5832 | 88 |
### Training hyperparameters
The following hyperparameters were used during training:
- learning_rate: 3e-05
- train_batch_size: 32
- eval_batch_size: 32
- seed: 42
- gradient_accumulation_steps: 2
- total_train_batch_size: 64
- optimizer: Adam with betas=(0.9,0.999) and epsilon=1e-08
- lr_scheduler_type: linear
- lr_scheduler_warmup_ratio: 0.1
- num_epochs: 4
### Training results
| Training Loss | Epoch | Step | Validation Loss | Precision | Recall | F1 | Accuracy |
|:-------------:|:-----:|:----:|:---------------:|:---------:|:------:|:------:|:--------:|
| 0.2602 | 1.0 | 2059 | 0.2486 | 0.6570 | 0.7031 | 0.6793 | 0.9270 |
| 0.2199 | 2.0 | 4118 | 0.2369 | 0.6791 | 0.7043 | 0.6915 | 0.9302 |
| 0.2052 | 3.0 | 6177 | 0.2349 | 0.6785 | 0.7143 | 0.6959 | 0.9312 |
| 0.1835 | 4.0 | 8236 | 0.2362 | 0.6810 | 0.7160 | 0.6981 | 0.9313 |
### Framework versions
- Transformers 4.39.3
- Pytorch 2.2.0+cu121
- Datasets 2.18.0
- Tokenizers 0.15.2
## Citation
### BibTeX
```
@misc{bogdanov2024nuner,
title={NuNER: Entity Recognition Encoder Pre-training via LLM-Annotated Data},
author={Sergei Bogdanov and Alexandre Constantin and Timothée Bernard and Benoit Crabbé and Etienne Bernard},
year={2024},
eprint={2402.15343},
archivePrefix={arXiv},
primaryClass={cs.CL}
}
```
| [
"NAMED_ENTITY_RECOGNITION"
] | Non_BioNLP |
makiart/ModernBERT-base-DPR-msmarco | makiart | sentence-similarity | [
"sentence-transformers",
"safetensors",
"modernbert",
"sentence-similarity",
"feature-extraction",
"generated_from_trainer",
"dataset_size:11662655",
"loss:CachedMultipleNegativesRankingLoss",
"en",
"dataset:sentence-transformers/msmarco-co-condenser-margin-mse-sym-mnrl-mean-v1",
"arxiv:1908.10084",
"arxiv:2101.06983",
"base_model:answerdotai/ModernBERT-base",
"base_model:finetune:answerdotai/ModernBERT-base",
"model-index",
"autotrain_compatible",
"endpoints_compatible",
"region:us"
] | 1,734,952,454,000 | 2024-12-23T11:14:31 | 8 | 0 | ---
base_model: answerdotai/ModernBERT-base
datasets:
- sentence-transformers/msmarco-co-condenser-margin-mse-sym-mnrl-mean-v1
language:
- en
library_name: sentence-transformers
metrics:
- cosine_accuracy
pipeline_tag: sentence-similarity
tags:
- sentence-transformers
- sentence-similarity
- feature-extraction
- generated_from_trainer
- dataset_size:11662655
- loss:CachedMultipleNegativesRankingLoss
widget:
- source_sentence: where is henderson mn
sentences:
- Confidence votes 1.7K. Assuming we're talking about the `usual' 12 volt car battery'
the resting voltage should be around 11 to 11.5 volts. Under charge it's as high
as 15 volts as supplied from the alternator,and most cars won't start if the voltage
is under 10.5 to 11.5 volts. The term `12 volt battery' is what's referred to
as, `nominal' or `in name only' as a general reference and not meant to be an
accurate description.
- Henderson is a very small town of 1,000 people on the west bank of the Minnesota
River just south of the Minneapolis and Saint Paul metro area.
- Henderson, officially the City of Henderson, is an affluent city in Clark County,
Nevada, United States, about 16 miles southeast of Las Vegas. It is the second-largest
city in Nevada, after Las Vegas, with an estimated population of 292,969 in 2016.[2]
The city is part of the Las Vegas metropolitan area, which spans the entire Las
Vegas Valley. Henderson occupies the southeastern end of the valley, at an elevation
of approximately 1,330 feet (410 m).
- source_sentence: polytomy definition
sentences:
- Polytomy definition, the act or process of dividing into more than three parts.
See more.
- 'The name Loyalty has the following meaning: One who is faithful, loyal. It is
a male name, suitable for baby boys. Origins. The name Loyalty is very likely
a(n) English variant of the name Loyal. See other suggested English boy baby names.
You might also like to see the other variants of the name Loyal.'
- "Polysemy (/pÉ\x99Ë\x88lɪsɪmi/ or /Ë\x88pÉ\x92lɪsiË\x90mi/; from Greek: Ï\x80\
ολÏ\N-, poly-, many and Ï\x83á¿\x86μα, sêma, sign) is the capacity for a\
\ sign (such as a word, phrase, or symbol) to have multiple meanings (that is,\
\ multiple semes or sememes and thus multiple senses), usually related by contiguity\
\ of meaning within a semantic field."
- source_sentence: age group for juvenile arthritis
sentences:
- "Different Types of Juvenile Rheumatoid Arthritis. There are three kinds. Each\
\ type is based on the number of joints involved, the symptoms, and certain antibodies\
\ that may be in the blood. Four or fewer joints are involved. Doctors call this\
\ pauciarticular JRA. Itâ\x80\x99s the most common form. About half of all children\
\ with juvenile rheumatoid arthritis have this type. It usually affects large\
\ joints like the knees. Girls under age 8 are most likely to get it."
- Juvenile rheumatoid arthritis (JRA), often referred to by doctors today as juvenile
idiopathic arthritis (JIA), is a type of arthritis that causes joint inflammation
and stiffness for more than six weeks in a child aged 16 or younger. It affects
approximately 50,000 children in the United States.
- A depressant, or central depressant, is a drug that lowers neurotransmission levels,
which is to depress or reduce arousal or stimulation, in various areas of the
brain.Depressants are also occasionally referred to as downers as they lower the
level of arousal when taken.istilled (concentrated) alcoholic beverages, often
called hard liquor , roughly eight times more alcoholic than beer. An alcoholic
beverage is a drink that contains ethanol, an anesthetic that has been used as
a psychoactive drug for several millennia. Ethanol is the oldest recreational
drug still used by humans.
- source_sentence: what is besivance and durezol used for
sentences:
- Besivance is antibiotic eye drops, Prolensa is antiinflammatory eye drop and Durezol
is steroid eye drop. Besivance and Prolensa are need to be taken from 1-3 days
prior to surgery as a prophylaxis to prevent postoperative infection and inflammation
respectively. These eye drops can be administered after at least a gap of 5 minutes.
They are needed to be administered at least 4 times per day.
- .23 Acres Comfort, Kendall County, Texas. $399,500. This could be the most well
known building in Comfort with excellent all around visibility. Constructed in
the early 1930's and initially used as a bar it ...
- Duloxetine is used to treat major depressive disorder and general anxiety disorder.
Duloxetine is also used to treat fibromyalgia (a chronic pain disorder), or chronic
muscle or joint pain (such as low back pain and osteoarthritis pain). Duloxetine
is also used to treat pain caused by nerve damage in people with diabetes (diabetic
neuropathy).
- source_sentence: do bond funds pay dividends
sentences:
- If a cavity is causing the toothache, your dentist will fill the cavity or possibly
extract the tooth, if necessary. A root canal might be needed if the cause of
the toothache is determined to be an infection of the tooth's nerve. Bacteria
that have worked their way into the inner aspects of the tooth cause such an infection.
An antibiotic may be prescribed if there is fever or swelling of the jaw.
- "You would have $71,200 paying out $1,687 in annual dividends. That is about $4.62\
\ for working up in the morning. Interestingly enough, that 2.37% yield is at\
\ a low point because The Wellington Fund is a â\x80\x9Cbalanced fundâ\x80\x9D\
\ meaning that it holds a combination of stocks and bonds."
- A bond fund or debt fund is a fund that invests in bonds, or other debt securities.
Bond funds can be contrasted with stock funds and money funds. Bond funds typically
pay periodic dividends that include interest payments on the fund's underlying
securities plus periodic realized capital appreciation. Bond funds typically pay
higher dividends than CDs and money market accounts. Most bond funds pay out dividends
more frequently than individual bonds.
model-index:
- name: SentenceTransformer based on answerdotai/ModernBERT-base
results:
- task:
type: triplet
name: Triplet
dataset:
name: msmarco co condenser dev
type: msmarco-co-condenser-dev
metrics:
- type: cosine_accuracy
value: 0.986
name: Cosine Accuracy
---
# SentenceTransformer based on answerdotai/ModernBERT-base
This is a [sentence-transformers](https://www.SBERT.net) model finetuned from [answerdotai/ModernBERT-base](https://huggingface.co/answerdotai/ModernBERT-base) on the [msmarco-co-condenser-margin-mse-sym-mnrl-mean-v1](https://huggingface.co/datasets/sentence-transformers/msmarco-co-condenser-margin-mse-sym-mnrl-mean-v1) dataset. It maps sentences & paragraphs to a 768-dimensional dense vector space and can be used for semantic textual similarity, semantic search, paraphrase mining, text classification, clustering, and more.
## Model Details
### Model Description
- **Model Type:** Sentence Transformer
- **Base model:** [answerdotai/ModernBERT-base](https://huggingface.co/answerdotai/ModernBERT-base) <!-- at revision 5756c58a31a2478f9e62146021f48295a92c3da5 -->
- **Maximum Sequence Length:** 8192 tokens
- **Output Dimensionality:** 768 dimensions
- **Similarity Function:** Cosine Similarity
- **Training Dataset:**
- [msmarco-co-condenser-margin-mse-sym-mnrl-mean-v1](https://huggingface.co/datasets/sentence-transformers/msmarco-co-condenser-margin-mse-sym-mnrl-mean-v1)
- **Language:** en
<!-- - **License:** Unknown -->
### Model Sources
- **Documentation:** [Sentence Transformers Documentation](https://sbert.net)
- **Repository:** [Sentence Transformers on GitHub](https://github.com/UKPLab/sentence-transformers)
- **Hugging Face:** [Sentence Transformers on Hugging Face](https://huggingface.co/models?library=sentence-transformers)
### Full Model Architecture
```
SentenceTransformer(
(0): Transformer({'max_seq_length': 8192, 'do_lower_case': False}) with Transformer model: ModernBertModel
(1): Pooling({'word_embedding_dimension': 768, 'pooling_mode_cls_token': False, 'pooling_mode_mean_tokens': True, 'pooling_mode_max_tokens': False, 'pooling_mode_mean_sqrt_len_tokens': False, 'pooling_mode_weightedmean_tokens': False, 'pooling_mode_lasttoken': False, 'include_prompt': True})
)
```
## Usage
### Direct Usage (Sentence Transformers)
First install the Sentence Transformers library:
```bash
pip install -U sentence-transformers
```
Then you can load this model and run inference.
```python
from sentence_transformers import SentenceTransformer
# Download from the 🤗 Hub
model = SentenceTransformer("makiart/ModernBERT-base-DPR-8e-05")
# Run inference
sentences = [
'do bond funds pay dividends',
"A bond fund or debt fund is a fund that invests in bonds, or other debt securities. Bond funds can be contrasted with stock funds and money funds. Bond funds typically pay periodic dividends that include interest payments on the fund's underlying securities plus periodic realized capital appreciation. Bond funds typically pay higher dividends than CDs and money market accounts. Most bond funds pay out dividends more frequently than individual bonds.",
'You would have $71,200 paying out $1,687 in annual dividends. That is about $4.62 for working up in the morning. Interestingly enough, that 2.37% yield is at a low point because The Wellington Fund is a â\x80\x9cbalanced fundâ\x80\x9d meaning that it holds a combination of stocks and bonds.',
]
embeddings = model.encode(sentences)
print(embeddings.shape)
# [3, 768]
# Get the similarity scores for the embeddings
similarities = model.similarity(embeddings, embeddings)
print(similarities.shape)
# [3, 3]
```
<!--
### Direct Usage (Transformers)
<details><summary>Click to see the direct usage in Transformers</summary>
</details>
-->
<!--
### Downstream Usage (Sentence Transformers)
You can finetune this model on your own dataset.
<details><summary>Click to expand</summary>
</details>
-->
<!--
### Out-of-Scope Use
*List how the model may foreseeably be misused and address what users ought not to do with the model.*
-->
## Evaluation
### Metrics
#### Triplet
* Dataset: `msmarco-co-condenser-dev`
* Evaluated with [<code>TripletEvaluator</code>](https://sbert.net/docs/package_reference/sentence_transformer/evaluation.html#sentence_transformers.evaluation.TripletEvaluator)
| Metric | Value |
|:--------------------|:----------|
| **cosine_accuracy** | **0.986** |
<!--
## Bias, Risks and Limitations
*What are the known or foreseeable issues stemming from this model? You could also flag here known failure cases or weaknesses of the model.*
-->
<!--
### Recommendations
*What are recommendations with respect to the foreseeable issues? For example, filtering explicit content.*
-->
## Training Details
### Training Dataset
#### msmarco-co-condenser-margin-mse-sym-mnrl-mean-v1
* Dataset: [msmarco-co-condenser-margin-mse-sym-mnrl-mean-v1](https://huggingface.co/datasets/sentence-transformers/msmarco-co-condenser-margin-mse-sym-mnrl-mean-v1) at [84ed2d3](https://huggingface.co/datasets/sentence-transformers/msmarco-co-condenser-margin-mse-sym-mnrl-mean-v1/tree/84ed2d35626f617d890bd493b4d6db69a741e0e2)
* Size: 11,662,655 training samples
* Columns: <code>query</code>, <code>positive</code>, and <code>negative</code>
* Approximate statistics based on the first 1000 samples:
| | query | positive | negative |
|:--------|:---------------------------------------------------------------------------------|:------------------------------------------------------------------------------------|:------------------------------------------------------------------------------------|
| type | string | string | string |
| details | <ul><li>min: 4 tokens</li><li>mean: 9.26 tokens</li><li>max: 34 tokens</li></ul> | <ul><li>min: 17 tokens</li><li>mean: 79.14 tokens</li><li>max: 222 tokens</li></ul> | <ul><li>min: 24 tokens</li><li>mean: 80.09 tokens</li><li>max: 436 tokens</li></ul> |
* Samples:
| query | positive | negative |
|:---------------------------------------------------|:---------------------------------------------------------------------------------------------------------------------------------------------------------------------------------------------------------------------------------------------------------------------------------------------------------------------------------------|:--------------------------------------------------------------------------------------------------------------------------------------------------------------------------------------------------------------------------------------------------------------------------------------------------------------------------------------------------------------------------------------------------------------------------|
| <code>what is the meaning of menu planning</code> | <code>Menu planning is the selection of a menu for an event. Such as picking out the dinner for your wedding or even a meal at a Birthday Party. Menu planning is when you are preparing a calendar of meals and you have to sit down and decide what meat and veggies you want to serve on each certain day.</code> | <code>Menu Costs. In economics, a menu cost is the cost to a firm resulting from changing its prices. The name stems from the cost of restaurants literally printing new menus, but economists use it to refer to the costs of changing nominal prices in general.</code> |
| <code>how old is brett butler</code> | <code>Brett Butler is 59 years old. To be more precise (and nerdy), the current age as of right now is 21564 days or (even more geeky) 517536 hours. That's a lot of hours!</code> | <code>Passed in: St. John's, Newfoundland and Labrador, Canada. Passed on: 16/07/2016. Published in the St. John's Telegram. Passed away suddenly at the Health Sciences Centre surrounded by his loving family, on July 16, 2016 Robert (Bobby) Joseph Butler, age 52 years. Predeceased by his special aunt Geri Murrin and uncle Mike Mchugh; grandparents Joe and Margaret Murrin and Jack and Theresa Butler.</code> |
| <code>when was the last navajo treaty sign?</code> | <code>In Executive Session, Senate of the United States, July 25, 1868. Resolved, (two-thirds of the senators present concurring,) That the Senate advise and consent to the ratification of the treaty between the United States and the Navajo Indians, concluded at Fort Sumner, New Mexico, on the first day of June, 1868.</code> | <code>Share Treaty of Greenville. The Treaty of Greenville was signed August 3, 1795, between the United States, represented by Gen. Anthony Wayne, and chiefs of the Indian tribes located in the Northwest Territory, including the Wyandots, Delawares, Shawnees, Ottawas, Miamis, and others.</code> |
* Loss: [<code>CachedMultipleNegativesRankingLoss</code>](https://sbert.net/docs/package_reference/sentence_transformer/losses.html#cachedmultiplenegativesrankingloss) with these parameters:
```json
{
"scale": 20.0,
"similarity_fct": "cos_sim"
}
```
### Evaluation Dataset
#### msmarco-co-condenser-margin-mse-sym-mnrl-mean-v1
* Dataset: [msmarco-co-condenser-margin-mse-sym-mnrl-mean-v1](https://huggingface.co/datasets/sentence-transformers/msmarco-co-condenser-margin-mse-sym-mnrl-mean-v1) at [84ed2d3](https://huggingface.co/datasets/sentence-transformers/msmarco-co-condenser-margin-mse-sym-mnrl-mean-v1/tree/84ed2d35626f617d890bd493b4d6db69a741e0e2)
* Size: 11,662,655 evaluation samples
* Columns: <code>query</code>, <code>positive</code>, and <code>negative</code>
* Approximate statistics based on the first 1000 samples:
| | query | positive | negative |
|:--------|:--------------------------------------------------------------------------------|:------------------------------------------------------------------------------------|:------------------------------------------------------------------------------------|
| type | string | string | string |
| details | <ul><li>min: 4 tokens</li><li>mean: 9.2 tokens</li><li>max: 27 tokens</li></ul> | <ul><li>min: 21 tokens</li><li>mean: 80.44 tokens</li><li>max: 241 tokens</li></ul> | <ul><li>min: 23 tokens</li><li>mean: 80.38 tokens</li><li>max: 239 tokens</li></ul> |
* Samples:
| query | positive | negative |
|:------------------------------------------------------|:---------------------------------------------------------------------------------------------------------------------------------------------------------------------------------------------------------------------------------------------------------------------------------------------------------------------------------------------------------------------------------------------------------------------------------------------------------------------------------------------------------------------------------------------------------------------------|:----------------------------------------------------------------------------------------------------------------------------------------------------------------------------------------------------------------------------------------------------------------------------------------------------------------------------------------------------------------------------------------------------------------------------------------------------------------------------------------------------------------------------------------------------------------------------------|
| <code>what county is holly springs nc in</code> | <code>Holly Springs, North Carolina. Holly Springs is a town in Wake County, North Carolina, United States. As of the 2010 census, the town population was 24,661, over 2½ times its population in 2000. Contents.</code> | <code>The Mt. Holly Springs Park & Resort. One of the numerous trolley routes that carried people around the county at the turn of the century was the Carlisle & Mt. Holly Railway Company. The âHolly Trolleyâ as it came to be known was put into service by Patricio Russo and made its first run on May 14, 1901.</code> |
| <code>how long does nyquil stay in your system</code> | <code>In order to understand exactly how long Nyquil lasts, it is absolutely vital to learn about the various ingredients in the drug. One of the ingredients found in Nyquil is Doxylamine, which is an antihistamine. This specific medication has a biological half-life or 6 to 12 hours. With this in mind, it is possible for the drug to remain in the system for a period of 12 to 24 hours. It should be known that the specifics will depend on a wide variety of different factors, including your age and metabolism.</code> | <code>I confirmed that NyQuil is about 10% alcohol, a higher content than most domestic beers. When I asked about the relatively high proof, I was told that the alcohol dilutes the active ingredients. The alcohol free version is there for customers with addiction issues.. also found that in that version there is twice the amount of DXM. When I asked if I could speak to a chemist or scientist, I was told they didn't have anyone who fit that description there. Itâs been eight years since I kicked NyQuil. I've been sober from alcohol for four years.</code> |
| <code>what are mineral water</code> | <code>1 Mineral water â water from a mineral spring that contains various minerals, such as salts and sulfur compounds. 2 It comes from a source tapped at one or more bore holes or spring, and originates from a geologically and physically protected underground water source. Mineral water â water from a mineral spring that contains various minerals, such as salts and sulfur compounds. 2 It comes from a source tapped at one or more bore holes or spring, and originates from a geologically and physically protected underground water source.</code> | <code>Minerals for Your Body. Drinking mineral water is beneficial to health and well-being. But it is not only the amount of water you drink that is important-what the water contains is even more essential.inerals for Your Body. Drinking mineral water is beneficial to health and well-being. But it is not only the amount of water you drink that is important-what the water contains is even more essential.</code> |
* Loss: [<code>CachedMultipleNegativesRankingLoss</code>](https://sbert.net/docs/package_reference/sentence_transformer/losses.html#cachedmultiplenegativesrankingloss) with these parameters:
```json
{
"scale": 20.0,
"similarity_fct": "cos_sim"
}
```
### Training Hyperparameters
#### Non-Default Hyperparameters
- `per_device_train_batch_size`: 512
- `per_device_eval_batch_size`: 512
- `learning_rate`: 8e-05
- `num_train_epochs`: 1
- `warmup_ratio`: 0.05
- `bf16`: True
- `batch_sampler`: no_duplicates
#### All Hyperparameters
<details><summary>Click to expand</summary>
- `overwrite_output_dir`: False
- `do_predict`: False
- `eval_strategy`: no
- `prediction_loss_only`: True
- `per_device_train_batch_size`: 512
- `per_device_eval_batch_size`: 512
- `per_gpu_train_batch_size`: None
- `per_gpu_eval_batch_size`: None
- `gradient_accumulation_steps`: 1
- `eval_accumulation_steps`: None
- `torch_empty_cache_steps`: None
- `learning_rate`: 8e-05
- `weight_decay`: 0.0
- `adam_beta1`: 0.9
- `adam_beta2`: 0.999
- `adam_epsilon`: 1e-08
- `max_grad_norm`: 1.0
- `num_train_epochs`: 1
- `max_steps`: -1
- `lr_scheduler_type`: linear
- `lr_scheduler_kwargs`: {}
- `warmup_ratio`: 0.05
- `warmup_steps`: 0
- `log_level`: passive
- `log_level_replica`: warning
- `log_on_each_node`: True
- `logging_nan_inf_filter`: True
- `save_safetensors`: True
- `save_on_each_node`: False
- `save_only_model`: False
- `restore_callback_states_from_checkpoint`: False
- `no_cuda`: False
- `use_cpu`: False
- `use_mps_device`: False
- `seed`: 42
- `data_seed`: None
- `jit_mode_eval`: False
- `use_ipex`: False
- `bf16`: True
- `fp16`: False
- `fp16_opt_level`: O1
- `half_precision_backend`: auto
- `bf16_full_eval`: False
- `fp16_full_eval`: False
- `tf32`: None
- `local_rank`: 0
- `ddp_backend`: None
- `tpu_num_cores`: None
- `tpu_metrics_debug`: False
- `debug`: []
- `dataloader_drop_last`: False
- `dataloader_num_workers`: 0
- `dataloader_prefetch_factor`: None
- `past_index`: -1
- `disable_tqdm`: False
- `remove_unused_columns`: True
- `label_names`: None
- `load_best_model_at_end`: False
- `ignore_data_skip`: False
- `fsdp`: []
- `fsdp_min_num_params`: 0
- `fsdp_config`: {'min_num_params': 0, 'xla': False, 'xla_fsdp_v2': False, 'xla_fsdp_grad_ckpt': False}
- `fsdp_transformer_layer_cls_to_wrap`: None
- `accelerator_config`: {'split_batches': False, 'dispatch_batches': None, 'even_batches': True, 'use_seedable_sampler': True, 'non_blocking': False, 'gradient_accumulation_kwargs': None}
- `deepspeed`: None
- `label_smoothing_factor`: 0.0
- `optim`: adamw_torch
- `optim_args`: None
- `adafactor`: False
- `group_by_length`: False
- `length_column_name`: length
- `ddp_find_unused_parameters`: None
- `ddp_bucket_cap_mb`: None
- `ddp_broadcast_buffers`: False
- `dataloader_pin_memory`: True
- `dataloader_persistent_workers`: False
- `skip_memory_metrics`: True
- `use_legacy_prediction_loop`: False
- `push_to_hub`: False
- `resume_from_checkpoint`: None
- `hub_model_id`: None
- `hub_strategy`: every_save
- `hub_private_repo`: None
- `hub_always_push`: False
- `gradient_checkpointing`: False
- `gradient_checkpointing_kwargs`: None
- `include_inputs_for_metrics`: False
- `include_for_metrics`: []
- `eval_do_concat_batches`: True
- `fp16_backend`: auto
- `push_to_hub_model_id`: None
- `push_to_hub_organization`: None
- `mp_parameters`:
- `auto_find_batch_size`: False
- `full_determinism`: False
- `torchdynamo`: None
- `ray_scope`: last
- `ddp_timeout`: 1800
- `torch_compile`: False
- `torch_compile_backend`: None
- `torch_compile_mode`: None
- `dispatch_batches`: None
- `split_batches`: None
- `include_tokens_per_second`: False
- `include_num_input_tokens_seen`: False
- `neftune_noise_alpha`: None
- `optim_target_modules`: None
- `batch_eval_metrics`: False
- `eval_on_start`: False
- `use_liger_kernel`: False
- `eval_use_gather_object`: False
- `average_tokens_across_devices`: False
- `prompts`: None
- `batch_sampler`: no_duplicates
- `multi_dataset_batch_sampler`: proportional
</details>
### Training Logs
| Epoch | Step | Training Loss | msmarco-co-condenser-dev_cosine_accuracy |
|:------:|:----:|:-------------:|:----------------------------------------:|
| 0 | 0 | - | 0.605 |
| 0.2048 | 500 | 0.632 | - |
| 0.4095 | 1000 | 0.1451 | - |
| 0.6143 | 1500 | 0.1071 | - |
| 0.8190 | 2000 | 0.089 | - |
| 1.0 | 2442 | - | 0.986 |
### Framework Versions
- Python: 3.11.10
- Sentence Transformers: 3.3.1
- Transformers: 4.48.0.dev0
- PyTorch: 2.4.1+cu124
- Accelerate: 0.26.0
- Datasets: 3.2.0
- Tokenizers: 0.21.0
## Citation
### BibTeX
#### Sentence Transformers
```bibtex
@inproceedings{reimers-2019-sentence-bert,
title = "Sentence-BERT: Sentence Embeddings using Siamese BERT-Networks",
author = "Reimers, Nils and Gurevych, Iryna",
booktitle = "Proceedings of the 2019 Conference on Empirical Methods in Natural Language Processing",
month = "11",
year = "2019",
publisher = "Association for Computational Linguistics",
url = "https://arxiv.org/abs/1908.10084",
}
```
#### CachedMultipleNegativesRankingLoss
```bibtex
@misc{gao2021scaling,
title={Scaling Deep Contrastive Learning Batch Size under Memory Limited Setup},
author={Luyu Gao and Yunyi Zhang and Jiawei Han and Jamie Callan},
year={2021},
eprint={2101.06983},
archivePrefix={arXiv},
primaryClass={cs.LG}
}
```
<!--
## Glossary
*Clearly define terms in order to be accessible across audiences.*
-->
<!--
## Model Card Authors
*Lists the people who create the model card, providing recognition and accountability for the detailed work that goes into its construction.*
-->
<!--
## Model Card Contact
*Provides a way for people who have updates to the Model Card, suggestions, or questions, to contact the Model Card authors.*
--> | [
"TEXT_CLASSIFICATION"
] | Non_BioNLP |
marcolatella/irony_trained | marcolatella | text-classification | [
"transformers",
"pytorch",
"distilbert",
"text-classification",
"generated_from_trainer",
"dataset:tweet_eval",
"license:apache-2.0",
"model-index",
"autotrain_compatible",
"endpoints_compatible",
"region:us"
] | 1,646,263,745,000 | 2021-12-10T23:03:44 | 11 | 0 | ---
datasets:
- tweet_eval
license: apache-2.0
metrics:
- f1
tags:
- generated_from_trainer
model-index:
- name: irony_trained
results:
- task:
type: text-classification
name: Text Classification
dataset:
name: tweet_eval
type: tweet_eval
args: irony
metrics:
- type: f1
value: 0.6946397550129713
name: F1
---
<!-- This model card has been generated automatically according to the information the Trainer had access to. You
should probably proofread and complete it, then remove this comment. -->
# irony_trained
This model is a fine-tuned version of [distilbert-base-uncased](https://huggingface.co/distilbert-base-uncased) on the tweet_eval dataset.
It achieves the following results on the evaluation set:
- Loss: 1.6720
- F1: 0.6946
## Model description
More information needed
## Intended uses & limitations
More information needed
## Training and evaluation data
More information needed
## Training procedure
### Training hyperparameters
The following hyperparameters were used during training:
- learning_rate: 2.6375567293432486e-05
- train_batch_size: 4
- eval_batch_size: 4
- seed: 0
- optimizer: Adam with betas=(0.9,0.999) and epsilon=1e-08
- lr_scheduler_type: linear
- num_epochs: 4
### Training results
| Training Loss | Epoch | Step | Validation Loss | F1 |
|:-------------:|:-----:|:----:|:---------------:|:------:|
| 0.6643 | 1.0 | 716 | 0.5958 | 0.6776 |
| 0.5633 | 2.0 | 1432 | 0.8863 | 0.6759 |
| 0.348 | 3.0 | 2148 | 1.4215 | 0.6817 |
| 0.2192 | 4.0 | 2864 | 1.6720 | 0.6946 |
### Framework versions
- Transformers 4.13.0
- Pytorch 1.10.0+cu111
- Datasets 1.16.1
- Tokenizers 0.10.3
| [
"TEXT_CLASSIFICATION"
] | Non_BioNLP |
Lots-of-LoRAs/Mistral-7B-Instruct-v0.2-4b-r16-task996 | Lots-of-LoRAs | null | [
"pytorch",
"safetensors",
"en",
"arxiv:1910.09700",
"arxiv:2407.00066",
"license:mit",
"region:us"
] | 1,735,600,219,000 | 2024-12-30T23:10:24 | 0 | 0 | ---
language: en
library_name: pytorch
license: mit
---
# Model Card for Mistral-7B-Instruct-v0.2-4b-r16-task996
<!-- Provide a quick summary of what the model is/does. -->
## Model Details
### Model Description
<!-- Provide a longer summary of what this model is. -->
LoRA trained on task996_pib_translation_english_bengali
- **Developed by:** bruel
- **Funded by [optional]:** [More Information Needed]
- **Shared by [optional]:** [More Information Needed]
- **Model type:** LoRA
- **Language(s) (NLP):** en
- **License:** mit
- **Finetuned from model [optional]:** mistralai/Mistral-7B-Instruct-v0.2
### Model Sources [optional]
<!-- Provide the basic links for the model. -->
- **Repository:** https://github.com/bruel-gabrielsson
- **Paper [optional]:** "Compress then Serve: Serving Thousands of LoRA Adapters with Little Overhead" (2024), Rickard Brüel Gabrielsson, Jiacheng Zhu, Onkar Bhardwaj, Leshem Choshen, Kristjan Greenewald, Mikhail Yurochkin and Justin Solomon
- **Demo [optional]:** [More Information Needed]
## Uses
<!-- Address questions around how the model is intended to be used, including the foreseeable users of the model and those affected by the model. -->
### Direct Use
<!-- This section is for the model use without fine-tuning or plugging into a larger ecosystem/app. -->
[More Information Needed]
### Downstream Use [optional]
<!-- This section is for the model use when fine-tuned for a task, or when plugged into a larger ecosystem/app -->
[More Information Needed]
### Out-of-Scope Use
<!-- This section addresses misuse, malicious use, and uses that the model will not work well for. -->
[More Information Needed]
## Bias, Risks, and Limitations
<!-- This section is meant to convey both technical and sociotechnical limitations. -->
[More Information Needed]
### Recommendations
<!-- This section is meant to convey recommendations with respect to the bias, risk, and technical limitations. -->
Users (both direct and downstream) should be made aware of the risks, biases and limitations of the model. More information needed for further recommendations.
## How to Get Started with the Model
Use the code below to get started with the model.
[More Information Needed]
## Training Details
### Training Data
<!-- This should link to a Dataset Card, perhaps with a short stub of information on what the training data is all about as well as documentation related to data pre-processing or additional filtering. -->
https://huggingface.co/datasets/Lots-of-LoRAs/task996_pib_translation_english_bengali sourced from https://github.com/allenai/natural-instructions
### Training Procedure
<!-- This relates heavily to the Technical Specifications. Content here should link to that section when it is relevant to the training procedure. -->
#### Preprocessing [optional]
[More Information Needed]
#### Training Hyperparameters
- **Training regime:** [More Information Needed] <!--fp32, fp16 mixed precision, bf16 mixed precision, bf16 non-mixed precision, fp16 non-mixed precision, fp8 mixed precision -->
#### Speeds, Sizes, Times [optional]
<!-- This section provides information about throughput, start/end time, checkpoint size if relevant, etc. -->
[More Information Needed]
## Evaluation
<!-- This section describes the evaluation protocols and provides the results. -->
### Testing Data, Factors & Metrics
#### Testing Data
<!-- This should link to a Dataset Card if possible. -->
[More Information Needed]
#### Factors
<!-- These are the things the evaluation is disaggregating by, e.g., subpopulations or domains. -->
[More Information Needed]
#### Metrics
<!-- These are the evaluation metrics being used, ideally with a description of why. -->
[More Information Needed]
### Results
[More Information Needed]
#### Summary
## Model Examination [optional]
<!-- Relevant interpretability work for the model goes here -->
[More Information Needed]
## Environmental Impact
<!-- Total emissions (in grams of CO2eq) and additional considerations, such as electricity usage, go here. Edit the suggested text below accordingly -->
Carbon emissions can be estimated using the [Machine Learning Impact calculator](https://mlco2.github.io/impact#compute) presented in [Lacoste et al. (2019)](https://arxiv.org/abs/1910.09700).
- **Hardware Type:** [More Information Needed]
- **Hours used:** [More Information Needed]
- **Cloud Provider:** [More Information Needed]
- **Compute Region:** [More Information Needed]
- **Carbon Emitted:** [More Information Needed]
## Technical Specifications [optional]
### Model Architecture and Objective
[More Information Needed]
### Compute Infrastructure
[More Information Needed]
#### Hardware
[More Information Needed]
#### Software
[More Information Needed]
## Citation [optional]
<!-- If there is a paper or blog post introducing the model, the APA and Bibtex information for that should go in this section. -->
**BibTeX:**
@misc{brüelgabrielsson2024compressserveservingthousands,
title={Compress then Serve: Serving Thousands of LoRA Adapters with Little Overhead},
author={Rickard Brüel-Gabrielsson and Jiacheng Zhu and Onkar Bhardwaj and Leshem Choshen and Kristjan Greenewald and Mikhail Yurochkin and Justin Solomon},
year={2024},
eprint={2407.00066},
archivePrefix={arXiv},
primaryClass={cs.DC},
url={https://arxiv.org/abs/2407.00066},
}
**APA:**
[More Information Needed]
## Glossary [optional]
<!-- If relevant, include terms and calculations in this section that can help readers understand the model or model card. -->
[More Information Needed]
## More Information [optional]
[More Information Needed]
## Model Card Authors [optional]
[More Information Needed]
## Model Card Contact
[More Information Needed] | [
"TRANSLATION"
] | Non_BioNLP |
gizmo-ai/flan-t5-small | gizmo-ai | text2text-generation | [
"transformers",
"pytorch",
"tf",
"jax",
"safetensors",
"t5",
"text2text-generation",
"en",
"fr",
"ro",
"de",
"multilingual",
"dataset:svakulenk0/qrecc",
"dataset:taskmaster2",
"dataset:djaym7/wiki_dialog",
"dataset:deepmind/code_contests",
"dataset:lambada",
"dataset:gsm8k",
"dataset:aqua_rat",
"dataset:esnli",
"dataset:quasc",
"dataset:qed",
"arxiv:2210.11416",
"arxiv:1910.09700",
"license:apache-2.0",
"autotrain_compatible",
"text-generation-inference",
"endpoints_compatible",
"region:us"
] | 1,708,613,346,000 | 2024-02-22T14:49:06 | 16 | 0 | ---
datasets:
- svakulenk0/qrecc
- taskmaster2
- djaym7/wiki_dialog
- deepmind/code_contests
- lambada
- gsm8k
- aqua_rat
- esnli
- quasc
- qed
language:
- en
- fr
- ro
- de
- multilingual
license: apache-2.0
tags:
- text2text-generation
widget:
- text: 'Translate to German: My name is Arthur'
example_title: Translation
- text: Please answer to the following question. Who is going to be the next Ballon
d'or?
example_title: Question Answering
- text: 'Q: Can Geoffrey Hinton have a conversation with George Washington? Give the
rationale before answering.'
example_title: Logical reasoning
- text: Please answer the following question. What is the boiling point of Nitrogen?
example_title: Scientific knowledge
- text: Answer the following yes/no question. Can you write a whole Haiku in a single
tweet?
example_title: Yes/no question
- text: Answer the following yes/no question by reasoning step-by-step. Can you write
a whole Haiku in a single tweet?
example_title: Reasoning task
- text: 'Q: ( False or not False or False ) is? A: Let''s think step by step'
example_title: Boolean Expressions
- text: The square root of x is the cube root of y. What is y to the power of 2, if
x = 4?
example_title: Math reasoning
- text: 'Premise: At my age you will probably have learnt one lesson. Hypothesis: It''s
not certain how many lessons you''ll learn by your thirties. Does the premise
entail the hypothesis?'
example_title: Premise and hypothesis
---
# Model Card for FLAN-T5 small
<img src="https://huggingface.co/datasets/huggingface/documentation-images/resolve/main/transformers/model_doc/flan2_architecture.jpg"
alt="drawing" width="600"/>
# Table of Contents
0. [TL;DR](#TL;DR)
1. [Model Details](#model-details)
2. [Usage](#usage)
3. [Uses](#uses)
4. [Bias, Risks, and Limitations](#bias-risks-and-limitations)
5. [Training Details](#training-details)
6. [Evaluation](#evaluation)
7. [Environmental Impact](#environmental-impact)
8. [Citation](#citation)
9. [Model Card Authors](#model-card-authors)
# TL;DR
If you already know T5, FLAN-T5 is just better at everything. For the same number of parameters, these models have been fine-tuned on more than 1000 additional tasks covering also more languages.
As mentioned in the first few lines of the abstract :
> Flan-PaLM 540B achieves state-of-the-art performance on several benchmarks, such as 75.2% on five-shot MMLU. We also publicly release Flan-T5 checkpoints,1 which achieve strong few-shot performance even compared to much larger models, such as PaLM 62B. Overall, instruction finetuning is a general method for improving the performance and usability of pretrained language models.
**Disclaimer**: Content from **this** model card has been written by the Hugging Face team, and parts of it were copy pasted from the [T5 model card](https://huggingface.co/t5-large).
# Model Details
## Model Description
- **Model type:** Language model
- **Language(s) (NLP):** English, Spanish, Japanese, Persian, Hindi, French, Chinese, Bengali, Gujarati, German, Telugu, Italian, Arabic, Polish, Tamil, Marathi, Malayalam, Oriya, Panjabi, Portuguese, Urdu, Galician, Hebrew, Korean, Catalan, Thai, Dutch, Indonesian, Vietnamese, Bulgarian, Filipino, Central Khmer, Lao, Turkish, Russian, Croatian, Swedish, Yoruba, Kurdish, Burmese, Malay, Czech, Finnish, Somali, Tagalog, Swahili, Sinhala, Kannada, Zhuang, Igbo, Xhosa, Romanian, Haitian, Estonian, Slovak, Lithuanian, Greek, Nepali, Assamese, Norwegian
- **License:** Apache 2.0
- **Related Models:** [All FLAN-T5 Checkpoints](https://huggingface.co/models?search=flan-t5)
- **Original Checkpoints:** [All Original FLAN-T5 Checkpoints](https://github.com/google-research/t5x/blob/main/docs/models.md#flan-t5-checkpoints)
- **Resources for more information:**
- [Research paper](https://arxiv.org/pdf/2210.11416.pdf)
- [GitHub Repo](https://github.com/google-research/t5x)
- [Hugging Face FLAN-T5 Docs (Similar to T5) ](https://huggingface.co/docs/transformers/model_doc/t5)
# Usage
Find below some example scripts on how to use the model in `transformers`:
## Using the Pytorch model
### Running the model on a CPU
<details>
<summary> Click to expand </summary>
```python
from transformers import T5Tokenizer, T5ForConditionalGeneration
tokenizer = T5Tokenizer.from_pretrained("google/flan-t5-small")
model = T5ForConditionalGeneration.from_pretrained("google/flan-t5-small")
input_text = "translate English to German: How old are you?"
input_ids = tokenizer(input_text, return_tensors="pt").input_ids
outputs = model.generate(input_ids)
print(tokenizer.decode(outputs[0]))
```
</details>
### Running the model on a GPU
<details>
<summary> Click to expand </summary>
```python
# pip install accelerate
from transformers import T5Tokenizer, T5ForConditionalGeneration
tokenizer = T5Tokenizer.from_pretrained("google/flan-t5-small")
model = T5ForConditionalGeneration.from_pretrained("google/flan-t5-small", device_map="auto")
input_text = "translate English to German: How old are you?"
input_ids = tokenizer(input_text, return_tensors="pt").input_ids.to("cuda")
outputs = model.generate(input_ids)
print(tokenizer.decode(outputs[0]))
```
</details>
### Running the model on a GPU using different precisions
#### FP16
<details>
<summary> Click to expand </summary>
```python
# pip install accelerate
import torch
from transformers import T5Tokenizer, T5ForConditionalGeneration
tokenizer = T5Tokenizer.from_pretrained("google/flan-t5-small")
model = T5ForConditionalGeneration.from_pretrained("google/flan-t5-small", device_map="auto", torch_dtype=torch.float16)
input_text = "translate English to German: How old are you?"
input_ids = tokenizer(input_text, return_tensors="pt").input_ids.to("cuda")
outputs = model.generate(input_ids)
print(tokenizer.decode(outputs[0]))
```
</details>
#### INT8
<details>
<summary> Click to expand </summary>
```python
# pip install bitsandbytes accelerate
from transformers import T5Tokenizer, T5ForConditionalGeneration
tokenizer = T5Tokenizer.from_pretrained("google/flan-t5-small")
model = T5ForConditionalGeneration.from_pretrained("google/flan-t5-small", device_map="auto", load_in_8bit=True)
input_text = "translate English to German: How old are you?"
input_ids = tokenizer(input_text, return_tensors="pt").input_ids.to("cuda")
outputs = model.generate(input_ids)
print(tokenizer.decode(outputs[0]))
```
</details>
# Uses
## Direct Use and Downstream Use
The authors write in [the original paper's model card](https://arxiv.org/pdf/2210.11416.pdf) that:
> The primary use is research on language models, including: research on zero-shot NLP tasks and in-context few-shot learning NLP tasks, such as reasoning, and question answering; advancing fairness and safety research, and understanding limitations of current large language models
See the [research paper](https://arxiv.org/pdf/2210.11416.pdf) for further details.
## Out-of-Scope Use
More information needed.
# Bias, Risks, and Limitations
The information below in this section are copied from the model's [official model card](https://arxiv.org/pdf/2210.11416.pdf):
> Language models, including Flan-T5, can potentially be used for language generation in a harmful way, according to Rae et al. (2021). Flan-T5 should not be used directly in any application, without a prior assessment of safety and fairness concerns specific to the application.
## Ethical considerations and risks
> Flan-T5 is fine-tuned on a large corpus of text data that was not filtered for explicit content or assessed for existing biases. As a result the model itself is potentially vulnerable to generating equivalently inappropriate content or replicating inherent biases in the underlying data.
## Known Limitations
> Flan-T5 has not been tested in real world applications.
## Sensitive Use:
> Flan-T5 should not be applied for any unacceptable use cases, e.g., generation of abusive speech.
# Training Details
## Training Data
The model was trained on a mixture of tasks, that includes the tasks described in the table below (from the original paper, figure 2):

## Training Procedure
According to the model card from the [original paper](https://arxiv.org/pdf/2210.11416.pdf):
> These models are based on pretrained T5 (Raffel et al., 2020) and fine-tuned with instructions for better zero-shot and few-shot performance. There is one fine-tuned Flan model per T5 model size.
The model has been trained on TPU v3 or TPU v4 pods, using [`t5x`](https://github.com/google-research/t5x) codebase together with [`jax`](https://github.com/google/jax).
# Evaluation
## Testing Data, Factors & Metrics
The authors evaluated the model on various tasks covering several languages (1836 in total). See the table below for some quantitative evaluation:

For full details, please check the [research paper](https://arxiv.org/pdf/2210.11416.pdf).
## Results
For full results for FLAN-T5-Small, see the [research paper](https://arxiv.org/pdf/2210.11416.pdf), Table 3.
# Environmental Impact
Carbon emissions can be estimated using the [Machine Learning Impact calculator](https://mlco2.github.io/impact#compute) presented in [Lacoste et al. (2019)](https://arxiv.org/abs/1910.09700).
- **Hardware Type:** Google Cloud TPU Pods - TPU v3 or TPU v4 | Number of chips ≥ 4.
- **Hours used:** More information needed
- **Cloud Provider:** GCP
- **Compute Region:** More information needed
- **Carbon Emitted:** More information needed
# Citation
**BibTeX:**
```bibtex
@misc{https://doi.org/10.48550/arxiv.2210.11416,
doi = {10.48550/ARXIV.2210.11416},
url = {https://arxiv.org/abs/2210.11416},
author = {Chung, Hyung Won and Hou, Le and Longpre, Shayne and Zoph, Barret and Tay, Yi and Fedus, William and Li, Eric and Wang, Xuezhi and Dehghani, Mostafa and Brahma, Siddhartha and Webson, Albert and Gu, Shixiang Shane and Dai, Zhuyun and Suzgun, Mirac and Chen, Xinyun and Chowdhery, Aakanksha and Narang, Sharan and Mishra, Gaurav and Yu, Adams and Zhao, Vincent and Huang, Yanping and Dai, Andrew and Yu, Hongkun and Petrov, Slav and Chi, Ed H. and Dean, Jeff and Devlin, Jacob and Roberts, Adam and Zhou, Denny and Le, Quoc V. and Wei, Jason},
keywords = {Machine Learning (cs.LG), Computation and Language (cs.CL), FOS: Computer and information sciences, FOS: Computer and information sciences},
title = {Scaling Instruction-Finetuned Language Models},
publisher = {arXiv},
year = {2022},
copyright = {Creative Commons Attribution 4.0 International}
}
``` | [
"QUESTION_ANSWERING",
"TRANSLATION"
] | Non_BioNLP |
mini1013/master_cate_fd8 | mini1013 | text-classification | [
"setfit",
"safetensors",
"roberta",
"sentence-transformers",
"text-classification",
"generated_from_setfit_trainer",
"arxiv:2209.11055",
"base_model:mini1013/master_domain",
"base_model:finetune:mini1013/master_domain",
"model-index",
"region:us"
] | 1,732,705,589,000 | 2024-11-27T11:06:51 | 608 | 0 | ---
base_model: mini1013/master_domain
library_name: setfit
metrics:
- metric
pipeline_tag: text-classification
tags:
- setfit
- sentence-transformers
- text-classification
- generated_from_setfit_trainer
widget:
- text: '[CJ](신세계 의정부점) 비비고 누룽지닭다리삼계탕 550g 주식회사 에스에스지닷컴'
- text: 고객 후기로 만들어진 밀푀유 쇼유 나베 밀키트 (2인) 2월27일(화) 주식회사 아내의쉐프
- text: 룸서비스 홈파티음식 케이터링 생일팩15종 서울출장뷔페 집들이 배달 돌잔치상 손님초대요리 3.룸서비스파티팩15종(고급박스용기)_6월_19일
주식회사 룸서비스딜리버리
- text: 홈파티음식 케이터링 생일팩15종 인천출장뷔페 집들이 배달 돌잔치상 소규모 손님초대요리 01.룸서비스 생일팩 15종_1월_20일 (주)셀루체
- text: 홈파티음식 케이터링 생일팩15종 인천출장뷔페 집들이 배달 돌잔치상 소규모 손님초대요리 3.룸서비스파티팩15종(고급박스용기)_4월_19일
(주)셀루체
inference: true
model-index:
- name: SetFit with mini1013/master_domain
results:
- task:
type: text-classification
name: Text Classification
dataset:
name: Unknown
type: unknown
split: test
metrics:
- type: metric
value: 0.9173203883495146
name: Metric
---
# SetFit with mini1013/master_domain
This is a [SetFit](https://github.com/huggingface/setfit) model that can be used for Text Classification. This SetFit model uses [mini1013/master_domain](https://huggingface.co/mini1013/master_domain) as the Sentence Transformer embedding model. A [LogisticRegression](https://scikit-learn.org/stable/modules/generated/sklearn.linear_model.LogisticRegression.html) instance is used for classification.
The model has been trained using an efficient few-shot learning technique that involves:
1. Fine-tuning a [Sentence Transformer](https://www.sbert.net) with contrastive learning.
2. Training a classification head with features from the fine-tuned Sentence Transformer.
## Model Details
### Model Description
- **Model Type:** SetFit
- **Sentence Transformer body:** [mini1013/master_domain](https://huggingface.co/mini1013/master_domain)
- **Classification head:** a [LogisticRegression](https://scikit-learn.org/stable/modules/generated/sklearn.linear_model.LogisticRegression.html) instance
- **Maximum Sequence Length:** 512 tokens
- **Number of Classes:** 8 classes
<!-- - **Training Dataset:** [Unknown](https://huggingface.co/datasets/unknown) -->
<!-- - **Language:** Unknown -->
<!-- - **License:** Unknown -->
### Model Sources
- **Repository:** [SetFit on GitHub](https://github.com/huggingface/setfit)
- **Paper:** [Efficient Few-Shot Learning Without Prompts](https://arxiv.org/abs/2209.11055)
- **Blogpost:** [SetFit: Efficient Few-Shot Learning Without Prompts](https://huggingface.co/blog/setfit)
### Model Labels
| Label | Examples |
|:------|:----------------------------------------------------------------------------------------------------------------------------------------------------------------------------------------------------------------------------|
| 6.0 | <ul><li>'듬뿍담은 안동식 순살 찜닭 밀키트 711g 주식회사 프레시지'</li><li>'우렁쌈장 (2인분) 밀키트 쿠킹박스 우렁살 2개 추가(100g) 농업회사법인 주식회사 아임셰프'</li><li>'홍수계 매콤 당면듬뿍 순살 찜닭 850g 2인분 냉동 밀키트 셀린'</li></ul> |
| 1.0 | <ul><li>'[마이셰프] 찹스테이크(1인)(프리미엄박스) 주식회사 마이셰프'</li><li>'소문난 청정원 호밍스 마포식 돼지양념구이 210g 정원이샵 홈파티음식 캠핑요리 맥주안주 야식 간편식 홈캉스 풍미업 모에모에큥 에스더블유디자인'</li><li>'심쿡 슈페리어 연어 스테이크 455g 밀키트 쿠킹박스 인영이네'</li></ul> |
| 5.0 | <ul><li>'[골든벨통상](신세계센텀점)골든벨 심영순쇠고기국간장250ml 주식회사 에스에스지닷컴'</li><li>'[CJ](신세계센텀점) 튀김가루 1kg 1개 주식회사 에스에스지닷컴'</li><li>'(치즈박스)쉐프가 만든 캠핑 와인안주세트(고기 포함 안됨 X) 캘리포니아 키친 실속형(-2500)_11/20 월요일 캘리포니아키친(california kitchen)'</li></ul> |
| 4.0 | <ul><li>'소고기 버섯 잡채 (2인분) 주식회사 프레시지'</li><li>'야식메뉴 청정원 호밍스 춘천식 치즈닭갈비 220g 저녁반찬 자취요리 규비에스코퍼레이션'</li><li>'하림 궁중 국물 닭떡볶이 700g 밀키트 바이라이프'</li></ul> |
| 0.0 | <ul><li>'올바르고반듯한 떡볶이 원조시장 떡볶이 (냉동), 575g, 1개 하누코지'</li><li>'두끼 즉석떡볶이 560G 아이스박스 포장/선택 인터드림'</li><li>'두끼 매콤 고소 로제떡볶이 3팩 450g 주식회사 다른'</li></ul> |
| 3.0 | <ul><li>'[강원팜] 홈스랑 곤드레감자밥 쉽게만들기6인분 강원팜'</li><li>'마이셰프 즉석밥 일상정원 명란 솥밥 (냉동), 233g, 1개 하누코지'</li><li>'여름철 보양식 전복죽 200g 1팩 더블제이doubleJ'</li></ul> |
| 7.0 | <ul><li>'우정옥 여주 한우 특곰탕 1kg(2인분) 한우사골곰탕 도가니탕 1000g(약 2인분) 주식회사 우정옥'</li><li>'25년 전통 수복 얼큰 감자탕 [기본팩] 캠핑요리 밀키트 우거지 리얼감자탕 알뜰팩(라면사리X / 야채X) 수복얼큰감자탕'</li><li>'인천 정통 맛집 장금수 스페셜 부대전골 부대찌개 2-3인분 술안주 캠핑 집들이 밀키트 더렌'</li></ul> |
| 2.0 | <ul><li>'1분완성 개별포장 매콤 알싸 비빔 막국수 막국수 1팩 (주)데이지웰푸드'</li><li>'동원 면발의신 얼큰칼국수 268g 엄마손맛 육수 쉬운요리 감칠맛 자취 풍미 레시피 소스 인영'</li><li>'샐러드미인 쉐프엠 미트파스타 230g 주식회사 엠디에스코리아'</li></ul> |
## Evaluation
### Metrics
| Label | Metric |
|:--------|:-------|
| **all** | 0.9173 |
## Uses
### Direct Use for Inference
First install the SetFit library:
```bash
pip install setfit
```
Then you can load this model and run inference.
```python
from setfit import SetFitModel
# Download from the 🤗 Hub
model = SetFitModel.from_pretrained("mini1013/master_cate_fd8")
# Run inference
preds = model("[CJ](신세계 의정부점) 비비고 누룽지닭다리삼계탕 550g 주식회사 에스에스지닷컴")
```
<!--
### Downstream Use
*List how someone could finetune this model on their own dataset.*
-->
<!--
### Out-of-Scope Use
*List how the model may foreseeably be misused and address what users ought not to do with the model.*
-->
<!--
## Bias, Risks and Limitations
*What are the known or foreseeable issues stemming from this model? You could also flag here known failure cases or weaknesses of the model.*
-->
<!--
### Recommendations
*What are recommendations with respect to the foreseeable issues? For example, filtering explicit content.*
-->
## Training Details
### Training Set Metrics
| Training set | Min | Median | Max |
|:-------------|:----|:-------|:----|
| Word count | 3 | 9.3575 | 20 |
| Label | Training Sample Count |
|:------|:----------------------|
| 0.0 | 50 |
| 1.0 | 50 |
| 2.0 | 50 |
| 3.0 | 50 |
| 4.0 | 50 |
| 5.0 | 50 |
| 6.0 | 50 |
| 7.0 | 50 |
### Training Hyperparameters
- batch_size: (512, 512)
- num_epochs: (20, 20)
- max_steps: -1
- sampling_strategy: oversampling
- num_iterations: 40
- body_learning_rate: (2e-05, 2e-05)
- head_learning_rate: 2e-05
- loss: CosineSimilarityLoss
- distance_metric: cosine_distance
- margin: 0.25
- end_to_end: False
- use_amp: False
- warmup_proportion: 0.1
- seed: 42
- eval_max_steps: -1
- load_best_model_at_end: False
### Training Results
| Epoch | Step | Training Loss | Validation Loss |
|:-------:|:----:|:-------------:|:---------------:|
| 0.0159 | 1 | 0.4347 | - |
| 0.7937 | 50 | 0.2865 | - |
| 1.5873 | 100 | 0.0903 | - |
| 2.3810 | 150 | 0.0636 | - |
| 3.1746 | 200 | 0.0401 | - |
| 3.9683 | 250 | 0.003 | - |
| 4.7619 | 300 | 0.0016 | - |
| 5.5556 | 350 | 0.0017 | - |
| 6.3492 | 400 | 0.0025 | - |
| 7.1429 | 450 | 0.0007 | - |
| 7.9365 | 500 | 0.0001 | - |
| 8.7302 | 550 | 0.0001 | - |
| 9.5238 | 600 | 0.0002 | - |
| 10.3175 | 650 | 0.0001 | - |
| 11.1111 | 700 | 0.0008 | - |
| 11.9048 | 750 | 0.0001 | - |
| 12.6984 | 800 | 0.0001 | - |
| 13.4921 | 850 | 0.0 | - |
| 14.2857 | 900 | 0.0001 | - |
| 15.0794 | 950 | 0.0 | - |
| 15.8730 | 1000 | 0.0 | - |
| 16.6667 | 1050 | 0.0 | - |
| 17.4603 | 1100 | 0.0 | - |
| 18.2540 | 1150 | 0.0 | - |
| 19.0476 | 1200 | 0.0 | - |
| 19.8413 | 1250 | 0.0 | - |
### Framework Versions
- Python: 3.10.12
- SetFit: 1.1.0.dev0
- Sentence Transformers: 3.1.1
- Transformers: 4.46.1
- PyTorch: 2.4.0+cu121
- Datasets: 2.20.0
- Tokenizers: 0.20.0
## Citation
### BibTeX
```bibtex
@article{https://doi.org/10.48550/arxiv.2209.11055,
doi = {10.48550/ARXIV.2209.11055},
url = {https://arxiv.org/abs/2209.11055},
author = {Tunstall, Lewis and Reimers, Nils and Jo, Unso Eun Seo and Bates, Luke and Korat, Daniel and Wasserblat, Moshe and Pereg, Oren},
keywords = {Computation and Language (cs.CL), FOS: Computer and information sciences, FOS: Computer and information sciences},
title = {Efficient Few-Shot Learning Without Prompts},
publisher = {arXiv},
year = {2022},
copyright = {Creative Commons Attribution 4.0 International}
}
```
<!--
## Glossary
*Clearly define terms in order to be accessible across audiences.*
-->
<!--
## Model Card Authors
*Lists the people who create the model card, providing recognition and accountability for the detailed work that goes into its construction.*
-->
<!--
## Model Card Contact
*Provides a way for people who have updates to the Model Card, suggestions, or questions, to contact the Model Card authors.*
--> | [
"TEXT_CLASSIFICATION"
] | Non_BioNLP |
Thysted/SbertDP_classification | Thysted | text-classification | [
"sentence-transformers",
"safetensors",
"mpnet",
"setfit",
"text-classification",
"arxiv:2209.11055",
"license:apache-2.0",
"region:us"
] | 1,700,044,217,000 | 2023-11-15T10:31:01 | 3 | 0 | ---
license: apache-2.0
pipeline_tag: text-classification
tags:
- setfit
- sentence-transformers
- text-classification
---
# Thysted/SbertDP_classification
This is a [SetFit model](https://github.com/huggingface/setfit) that can be used for text classification. The model has been trained using an efficient few-shot learning technique that involves:
1. Fine-tuning a [Sentence Transformer](https://www.sbert.net) with contrastive learning.
2. Training a classification head with features from the fine-tuned Sentence Transformer.
## Usage
To use this model for inference, first install the SetFit library:
```bash
python -m pip install setfit
```
You can then run inference as follows:
```python
from setfit import SetFitModel
# Download from Hub and run inference
model = SetFitModel.from_pretrained("Thysted/SbertDP_classification")
# Run inference
preds = model(["i loved the spiderman movie!", "pineapple on pizza is the worst 🤮"])
```
## BibTeX entry and citation info
```bibtex
@article{https://doi.org/10.48550/arxiv.2209.11055,
doi = {10.48550/ARXIV.2209.11055},
url = {https://arxiv.org/abs/2209.11055},
author = {Tunstall, Lewis and Reimers, Nils and Jo, Unso Eun Seo and Bates, Luke and Korat, Daniel and Wasserblat, Moshe and Pereg, Oren},
keywords = {Computation and Language (cs.CL), FOS: Computer and information sciences, FOS: Computer and information sciences},
title = {Efficient Few-Shot Learning Without Prompts},
publisher = {arXiv},
year = {2022},
copyright = {Creative Commons Attribution 4.0 International}
}
```
| [
"TEXT_CLASSIFICATION"
] | Non_BioNLP |
HPLT/translate-zh_hant-en-v1.0-hplt | HPLT | translation | [
"transformers",
"pytorch",
"marian",
"text2text-generation",
"translation",
"zh",
"en",
"license:cc-by-4.0",
"autotrain_compatible",
"region:us"
] | 1,709,034,355,000 | 2024-03-14T00:52:52 | 16 | 0 | ---
language:
- zh
- en
license: cc-by-4.0
tags:
- translation
inference: false
---
### HPLT MT release v1.0
This repository contains the translation model for Traditional Chinese-English trained with HPLT data only. The model is available in both Marian and Hugging Face formats.
### Model Info
* Source language: Traditional Chinese
* Target language: English
* Data: HPLT data only
* Model architecture: Transformer-base
* Tokenizer: SentencePiece (Unigram)
* Cleaning: We used [OpusCleaner](https://github.com/hplt-project/OpusCleaner) with a set of basic rules. Details can be found in the filter files [here](https://github.com/hplt-project/HPLT-MT-Models/tree/main/v1.0/data/en-zh_hant/raw/v0).
You can check out our [deliverable report](https://hplt-project.org/HPLT_D5_1___Translation_models_for_select_language_pairs.pdf), [GitHub repository](https://github.com/hplt-project/HPLT-MT-Models/tree/main/v1.0), and [website](https://hplt-project.org) for more details.
### Usage
**Note** that for quality considerations, we recommend using `[HPLT/translate-zh_hant-en-v1.0-hplt_opus](https://huggingface.co/HPLT/translate-zh_hant-en-v1.0-hplt_opus)` instead of this model.
The model has been trained with [MarianNMT](https://github.com/marian-nmt/marian) and the weights are in the Marian format. We have also converted the model into the Hugging Face format so it is compatible with `transformers`.
#### Using Marian
To run inference with MarianNMT, refer to the [Inference/Decoding/Translation](https://github.com/hplt-project/HPLT-MT-Models/tree/main/v1.0#inferencedecodingtranslation) section of our GitHub repository. You will need the model file `model.npz.best-chrf.npz` and the vocabulary file `model.zh_hant-en.spm` from this repository.
#### Using transformers
We have also converted this model to the Hugging Face format and you can get started with the script below. **Note** that due a [known issue](https://github.com/huggingface/transformers/issues/26216) in weight conversion, the checkpoint cannot work with transformer versions <4.26 or >4.30. We tested and suggest `pip install transformers==4.28`.
```
from transformers import AutoTokenizer, AutoModelForSeq2SeqLM
tokenizer = AutoTokenizer.from_pretrained("HPLT/translate-zh_hant-en-v1.0-hplt")
model = AutoModelForSeq2SeqLM.from_pretrained("HPLT/translate-zh_hant-en-v1.0-hplt")
inputs = ["Input goes here.", "Make sure the language is right."]
batch_tokenized = tokenizer(inputs, return_tensors="pt", padding=True)
model_output = model.generate(
**batch_tokenized, num_beams=6, max_new_tokens=512
)
batch_detokenized = tokenizer.batch_decode(
model_output,
skip_special_tokens=True,
)
print(batch_detokenized)
```
## Benchmarks
When decoded using Marian, the model has the following test scores.
| Test set | BLEU | chrF++ | COMET22 |
| -------------------------------------- | ---- | ----- | ----- |
| FLORES200 | 20.3 | 47.7 | 0.8182 |
| NTREX | 18.2 | 44.9 | 0.79 |
### Acknowledgements
This project has received funding from the European Union's Horizon Europe research and innovation programme under grant agreement No 101070350 and from UK Research and Innovation (UKRI) under the UK government's Horizon Europe funding guarantee [grant number 10052546]
Brought to you by researchers from the University of Edinburgh and Charles University in Prague with support from the whole HPLT consortium.
| [
"TRANSLATION"
] | Non_BioNLP |
liuyuweitarek/paraphrase-mpnet-base-neo-300-seperate | liuyuweitarek | text-classification | [
"sentence-transformers",
"pytorch",
"mpnet",
"setfit",
"text-classification",
"arxiv:2209.11055",
"license:apache-2.0",
"region:us"
] | 1,705,055,067,000 | 2024-01-12T12:15:48 | 6 | 0 | ---
license: apache-2.0
pipeline_tag: text-classification
tags:
- setfit
- sentence-transformers
- text-classification
---
# liuyuweitarek/paraphrase-mpnet-base-neo-300-seperate
This is a [SetFit model](https://github.com/huggingface/setfit) that can be used for text classification. The model has been trained using an efficient few-shot learning technique that involves:
1. Fine-tuning a [Sentence Transformer](https://www.sbert.net) with contrastive learning.
2. Training a classification head with features from the fine-tuned Sentence Transformer.
## Usage
To use this model for inference, first install the SetFit library:
```bash
python -m pip install setfit
```
You can then run inference as follows:
```python
from setfit import SetFitModel
# Download from Hub and run inference
model = SetFitModel.from_pretrained("liuyuweitarek/paraphrase-mpnet-base-neo-300-seperate")
# Run inference
preds = model(["i loved the spiderman movie!", "pineapple on pizza is the worst 🤮"])
```
## BibTeX entry and citation info
```bibtex
@article{https://doi.org/10.48550/arxiv.2209.11055,
doi = {10.48550/ARXIV.2209.11055},
url = {https://arxiv.org/abs/2209.11055},
author = {Tunstall, Lewis and Reimers, Nils and Jo, Unso Eun Seo and Bates, Luke and Korat, Daniel and Wasserblat, Moshe and Pereg, Oren},
keywords = {Computation and Language (cs.CL), FOS: Computer and information sciences, FOS: Computer and information sciences},
title = {Efficient Few-Shot Learning Without Prompts},
publisher = {arXiv},
year = {2022},
copyright = {Creative Commons Attribution 4.0 International}
}
```
| [
"TEXT_CLASSIFICATION"
] | Non_BioNLP |
asingh14/marian-finetuned-en-to-fr | asingh14 | translation | [
"transformers",
"safetensors",
"marian",
"text2text-generation",
"translation",
"arxiv:1910.09700",
"autotrain_compatible",
"endpoints_compatible",
"region:us"
] | 1,733,194,478,000 | 2024-12-05T02:29:18 | 5 | 0 | ---
library_name: transformers
tags:
- translation
---
# Model Card for Model ID
<!-- Provide a quick summary of what the model is/does. -->
asingh14/marian-finetuned-en-to-fr
## Model Details
### Model Description
<!-- Provide a longer summary of what this model is. -->
This is a fine-tuned Marian model for English-to-French translation, fine-tuned on bilingual datasets to provide accurate and fluent translations between English and French. It leverages the MarianMT architecture from Hugging Face, which is specifically designed for machine translation tasks.
- **Developed by:** Asmita Singh and Shubhangi Nikam
- **Funded by [optional]:** NA
- **Shared by [optional]:** NA
- **Model type:** translation
- **Language(s) (NLP):** (English (source) and French (target))
- **License:** Apache 2.0 (inheritance from the original model and dataset)
- **Finetuned from model [optional]:** Helsinki-NLP/opus-mt-en-fr
### Model Sources [optional]
<!-- Provide the basic links for the model. -->
- **Repository:** https://huggingface.co/asingh14/marian-finetuned-en-to-fr
- **Paper [optional]:** NA
- **Demo [optional]:**
# To test the code on google collab run the below code.
from transformers import pipeline
# Load the translation pipeline for English to French
translator = pipeline("translation_en_to_fr", model="asingh14/marian-finetuned-en-to-fr")
# Translate some English sentences to French
translations = translator(
[
"I've been waiting for a HuggingFace course my whole life.",
"I hate this so much!",
]
)
# Print the translated text
for translation in translations:
print(translation['translation_text'])
# 3. Run the file
# 4. It will give the output:
"J'ai attendu un parcours de HuggingFace toute ma vie.
Je hais tant cela!"
# which means that the text got translated
## Uses
<!-- Address questions around how the model is intended to be used, including the foreseeable users of the model and those affected by the model. -->
### Direct Use
<!-- This section is for the model use without fine-tuning or plugging into a larger ecosystem/app. -->
This model can be directly used to translate English text into French. It is suitable for bilingual communication, text localization, or any task requiring high-quality English-to-French translation.
### Downstream Use [optional]
<!-- This section is for the model use when fine-tuned for a task, or when plugged into a larger ecosystem/app -->
The model can be incorporated into larger systems, such as multilingual chatbots, automated translation pipelines, or content localization workflows.
### Out-of-Scope Use
<!-- This section addresses misuse, malicious use, and uses that the model will not work well for. -->
This model is not suitable for translating languages other than English to French. It may not perform well on highly domain-specific or informal text.
## Bias, Risks, and Limitations
<!-- This section is meant to convey both technical and sociotechnical limitations. -->
- The model may exhibit translation biases present in the dataset used for training. For example, gender-neutral terms in English might be translated into gendered terms in French (e.g., "doctor" → "docteur" or "doctoresse").
- May struggle with idiomatic expressions, rare words, or culturally specific references.
### Recommendations
<!-- This section is meant to convey recommendations with respect to the bias, risk, and technical limitations. -->
Users should review translations, especially for critical applications or when using the model for sensitive or nuanced text.
## How to Get Started with the Model
Use the code below to get started with the model.
[More Information Needed]
## Training Details
### Training Data
<!-- This should link to a Dataset Card, perhaps with a short stub of information on what the training data is all about as well as documentation related to data pre-processing or additional filtering. -->
[More Information Needed]
### Training Procedure
<!-- This relates heavily to the Technical Specifications. Content here should link to that section when it is relevant to the training procedure. -->
#### Preprocessing [optional]
[More Information Needed]
#### Training Hyperparameters
- **Training regime:** [More Information Needed] <!--fp32, fp16 mixed precision, bf16 mixed precision, bf16 non-mixed precision, fp16 non-mixed precision, fp8 mixed precision -->
#### Speeds, Sizes, Times [optional]
<!-- This section provides information about throughput, start/end time, checkpoint size if relevant, etc. -->
[More Information Needed]
## Evaluation
<!-- This section describes the evaluation protocols and provides the results. -->
### Testing Data, Factors & Metrics
#### Testing Data
<!-- This should link to a Dataset Card if possible. -->
[More Information Needed]
#### Factors
<!-- These are the things the evaluation is disaggregating by, e.g., subpopulations or domains. -->
[More Information Needed]
#### Metrics
<!-- These are the evaluation metrics being used, ideally with a description of why. -->
[More Information Needed]
### Results
[More Information Needed]
#### Summary
## Model Examination [optional]
<!-- Relevant interpretability work for the model goes here -->
[More Information Needed]
## Environmental Impact
<!-- Total emissions (in grams of CO2eq) and additional considerations, such as electricity usage, go here. Edit the suggested text below accordingly -->
Carbon emissions can be estimated using the [Machine Learning Impact calculator](https://mlco2.github.io/impact#compute) presented in [Lacoste et al. (2019)](https://arxiv.org/abs/1910.09700).
- **Hardware Type:** [More Information Needed]
- **Hours used:** [More Information Needed]
- **Cloud Provider:** [More Information Needed]
- **Compute Region:** [More Information Needed]
- **Carbon Emitted:** [More Information Needed]
## Technical Specifications [optional]
### Model Architecture and Objective
[More Information Needed]
### Compute Infrastructure
[More Information Needed]
#### Hardware
[More Information Needed]
#### Software
[More Information Needed]
## Citation [optional]
<!-- If there is a paper or blog post introducing the model, the APA and Bibtex information for that should go in this section. -->
**BibTeX:**
[More Information Needed]
**APA:**
[More Information Needed]
## Glossary [optional]
<!-- If relevant, include terms and calculations in this section that can help readers understand the model or model card. -->
[More Information Needed]
## More Information [optional]
[More Information Needed]
## Model Card Authors [optional]
[More Information Needed]
## Model Card Contact
[More Information Needed] | [
"TRANSLATION"
] | Non_BioNLP |
LoneStriker/mistral-7b-lamia-v0.1-8.0bpw-h8-exl2 | LoneStriker | text-generation | [
"transformers",
"pytorch",
"mistral",
"text-generation",
"NSFW",
"Porn",
"Ecommerce",
"Roleplay",
"Summarization",
"conversational",
"custom_code",
"dataset:openerotica/Lamia",
"license:apache-2.0",
"autotrain_compatible",
"text-generation-inference",
"endpoints_compatible",
"region:us"
] | 1,705,939,506,000 | 2024-01-22T16:10:24 | 7 | 0 | ---
datasets:
- openerotica/Lamia
license: apache-2.0
tags:
- NSFW
- Porn
- Ecommerce
- Roleplay
- Summarization
---
This is a combination of the pruned erotica-analysis data, freedom-rp, and a subest of Airoboros.
The following Categories are what was taken out of the Airoborus datset and added to my own Lamia dataset:
"roleplay", "unalignment", "editor", "writing", "detailed_writing", "stylized_response", "unalign", "cot", "song"
I'm hoping that this can improve the models narrative/storywriting ability, logic, and intelligence, while reducing any potential inherent ethical "alignment" that may be present in the base mistral model from pretaining on Chat-GPT generated data.
The format is Chatml, and the base model is Yarn Mistral which increases the context size to a true 16k+ rather than rellying on the sliding attention window. | [
"SUMMARIZATION"
] | Non_BioNLP |
abjodas/bert-base-uncased-finetuned-emotion | abjodas | null | [
"tensorboard",
"safetensors",
"bert",
"generated_from_trainer",
"dataset:emotion",
"base_model:google-bert/bert-base-uncased",
"base_model:finetune:google-bert/bert-base-uncased",
"license:apache-2.0",
"model-index",
"region:us"
] | 1,722,851,757,000 | 2024-08-05T10:19:13 | 8 | 0 | ---
base_model: bert-base-uncased
datasets:
- emotion
license: apache-2.0
metrics:
- accuracy
- f1
tags:
- generated_from_trainer
model-index:
- name: bert-base-uncased-finetuned-emotion
results:
- task:
type: text-classification
name: Text Classification
dataset:
name: emotion
type: emotion
config: split
split: validation
args: split
metrics:
- type: accuracy
value: 0.9255
name: Accuracy
- type: f1
value: 0.925470874179848
name: F1
---
<!-- This model card has been generated automatically according to the information the Trainer had access to. You
should probably proofread and complete it, then remove this comment. -->
# bert-base-uncased-finetuned-emotion
This model is a fine-tuned version of [bert-base-uncased](https://huggingface.co/bert-base-uncased) on the emotion dataset.
It achieves the following results on the evaluation set:
- Loss: 0.2013
- Accuracy: 0.9255
- F1: 0.9255
## Model description
More information needed
## Intended uses & limitations
More information needed
## Training and evaluation data
More information needed
## Training procedure
### Training hyperparameters
The following hyperparameters were used during training:
- learning_rate: 2e-05
- train_batch_size: 64
- eval_batch_size: 64
- seed: 42
- optimizer: Adam with betas=(0.9,0.999) and epsilon=1e-08
- lr_scheduler_type: linear
- num_epochs: 2
### Training results
| Training Loss | Epoch | Step | Validation Loss | Accuracy | F1 |
|:-------------:|:-----:|:----:|:---------------:|:--------:|:------:|
| 0.8409 | 1.0 | 250 | 0.3036 | 0.9065 | 0.9071 |
| 0.2278 | 2.0 | 500 | 0.2013 | 0.9255 | 0.9255 |
### Framework versions
- Transformers 4.42.4
- Pytorch 2.3.1+cu121
- Datasets 2.20.0
- Tokenizers 0.19.1
| [
"TEXT_CLASSIFICATION"
] | Non_BioNLP |
usakha/Pegasus_multiNews_model | usakha | summarization | [
"transformers",
"pytorch",
"tensorboard",
"safetensors",
"pegasus",
"text2text-generation",
"summarization",
"dataset:multi_news",
"autotrain_compatible",
"endpoints_compatible",
"region:us"
] | 1,687,291,592,000 | 2023-07-18T19:10:55 | 30 | 0 | ---
datasets:
- multi_news
metrics:
- bleu
- rouge
pipeline_tag: summarization
---
# Hyperparameters
learning_rate=2e-5
per_device_train_batch_size=14
per_device_eval_batch_size=14
weight_decay=0.01
save_total_limit=3
num_train_epochs=3
predict_with_generate=True
fp16=True
# Training Output
global_step=7710,
training_loss=2.436398018566087,
metrics={'train_runtime': 30287.1254,
'train_samples_per_second': 3.564,
'train_steps_per_second': 0.255,
'total_flos': 3.1186278368988365e+17,
'train_loss': 2.436398018566087,
'epoch': 3.0}
# Training Results
| Epoch | Training Loss | Validation Loss | Rouge1 | Rouge2 | Rougel | Rougelsum | Bleu | Gen Len |
|:----- |:------------ |:--------------- |:-------- | :------- |:-------- |:--------- |:-------- |:--------- |
1| 2.451200| 2.291708| 0.322800| 0.110100| 0.194600| 0.194700| 0.368400| 150.224300
2| 2.527300| nan| 0.296400| 0.100100| 0.181800| 0.181900 |0.317300| 137.569200
3| 2.523800| nan |0.296600| 0.100000| 0.181800 |0.181900 |0.317200| 137.254000 | [
"SUMMARIZATION"
] | Non_BioNLP |
datasaur-dev/baseline-10-sarcasm-binary-all-MiniLM-L6-v2 | datasaur-dev | text-classification | [
"setfit",
"safetensors",
"bert",
"sentence-transformers",
"text-classification",
"generated_from_setfit_trainer",
"arxiv:2209.11055",
"base_model:sentence-transformers/all-MiniLM-L6-v2",
"base_model:finetune:sentence-transformers/all-MiniLM-L6-v2",
"region:us"
] | 1,731,943,331,000 | 2024-11-18T15:22:13 | 5 | 0 | ---
base_model: sentence-transformers/all-MiniLM-L6-v2
library_name: setfit
metrics:
- accuracy
pipeline_tag: text-classification
tags:
- setfit
- sentence-transformers
- text-classification
- generated_from_setfit_trainer
widget: []
inference: true
---
# SetFit with sentence-transformers/all-MiniLM-L6-v2
This is a [SetFit](https://github.com/huggingface/setfit) model that can be used for Text Classification. This SetFit model uses [sentence-transformers/all-MiniLM-L6-v2](https://huggingface.co/sentence-transformers/all-MiniLM-L6-v2) as the Sentence Transformer embedding model. A [LogisticRegression](https://scikit-learn.org/stable/modules/generated/sklearn.linear_model.LogisticRegression.html) instance is used for classification.
The model has been trained using an efficient few-shot learning technique that involves:
1. Fine-tuning a [Sentence Transformer](https://www.sbert.net) with contrastive learning.
2. Training a classification head with features from the fine-tuned Sentence Transformer.
## Model Details
### Model Description
- **Model Type:** SetFit
- **Sentence Transformer body:** [sentence-transformers/all-MiniLM-L6-v2](https://huggingface.co/sentence-transformers/all-MiniLM-L6-v2)
- **Classification head:** a [LogisticRegression](https://scikit-learn.org/stable/modules/generated/sklearn.linear_model.LogisticRegression.html) instance
- **Maximum Sequence Length:** 256 tokens
<!-- - **Number of Classes:** Unknown -->
<!-- - **Training Dataset:** [Unknown](https://huggingface.co/datasets/unknown) -->
<!-- - **Language:** Unknown -->
<!-- - **License:** Unknown -->
### Model Sources
- **Repository:** [SetFit on GitHub](https://github.com/huggingface/setfit)
- **Paper:** [Efficient Few-Shot Learning Without Prompts](https://arxiv.org/abs/2209.11055)
- **Blogpost:** [SetFit: Efficient Few-Shot Learning Without Prompts](https://huggingface.co/blog/setfit)
## Uses
### Direct Use for Inference
First install the SetFit library:
```bash
pip install setfit
```
Then you can load this model and run inference.
```python
from setfit import SetFitModel
# Download from the 🤗 Hub
model = SetFitModel.from_pretrained("datasaur-dev/baseline-10-sarcasm-binary-all-MiniLM-L6-v2")
# Run inference
preds = model("I loved the spiderman movie!")
```
<!--
### Downstream Use
*List how someone could finetune this model on their own dataset.*
-->
<!--
### Out-of-Scope Use
*List how the model may foreseeably be misused and address what users ought not to do with the model.*
-->
<!--
## Bias, Risks and Limitations
*What are the known or foreseeable issues stemming from this model? You could also flag here known failure cases or weaknesses of the model.*
-->
<!--
### Recommendations
*What are recommendations with respect to the foreseeable issues? For example, filtering explicit content.*
-->
## Training Details
### Framework Versions
- Python: 3.9.20
- SetFit: 1.1.0
- Sentence Transformers: 3.3.0
- Transformers: 4.42.2
- PyTorch: 2.6.0.dev20241112
- Datasets: 3.1.0
- Tokenizers: 0.19.1
## Citation
### BibTeX
```bibtex
@article{https://doi.org/10.48550/arxiv.2209.11055,
doi = {10.48550/ARXIV.2209.11055},
url = {https://arxiv.org/abs/2209.11055},
author = {Tunstall, Lewis and Reimers, Nils and Jo, Unso Eun Seo and Bates, Luke and Korat, Daniel and Wasserblat, Moshe and Pereg, Oren},
keywords = {Computation and Language (cs.CL), FOS: Computer and information sciences, FOS: Computer and information sciences},
title = {Efficient Few-Shot Learning Without Prompts},
publisher = {arXiv},
year = {2022},
copyright = {Creative Commons Attribution 4.0 International}
}
```
<!--
## Glossary
*Clearly define terms in order to be accessible across audiences.*
-->
<!--
## Model Card Authors
*Lists the people who create the model card, providing recognition and accountability for the detailed work that goes into its construction.*
-->
<!--
## Model Card Contact
*Provides a way for people who have updates to the Model Card, suggestions, or questions, to contact the Model Card authors.*
--> | [
"TEXT_CLASSIFICATION"
] | Non_BioNLP |
gokuls/hBERTv2_new_pretrain_48_KD_w_init_cola | gokuls | text-classification | [
"transformers",
"pytorch",
"tensorboard",
"hybridbert",
"text-classification",
"generated_from_trainer",
"en",
"dataset:glue",
"model-index",
"autotrain_compatible",
"endpoints_compatible",
"region:us"
] | 1,686,864,020,000 | 2023-06-15T21:33:11 | 8 | 0 | ---
datasets:
- glue
language:
- en
metrics:
- matthews_correlation
- accuracy
tags:
- generated_from_trainer
model-index:
- name: hBERTv2_new_pretrain_48_KD_w_init_cola
results:
- task:
type: text-classification
name: Text Classification
dataset:
name: GLUE COLA
type: glue
config: cola
split: validation
args: cola
metrics:
- type: matthews_correlation
value: 0.0
name: Matthews Correlation
- type: accuracy
value: 0.6912751793861389
name: Accuracy
---
<!-- This model card has been generated automatically according to the information the Trainer had access to. You
should probably proofread and complete it, then remove this comment. -->
# hBERTv2_new_pretrain_48_KD_w_init_cola
This model is a fine-tuned version of [gokuls/bert_12_layer_model_v2_complete_training_new_48_KD_wt_init](https://huggingface.co/gokuls/bert_12_layer_model_v2_complete_training_new_48_KD_wt_init) on the GLUE COLA dataset.
It achieves the following results on the evaluation set:
- Loss: 0.6175
- Matthews Correlation: 0.0
- Accuracy: 0.6913
## Model description
More information needed
## Intended uses & limitations
More information needed
## Training and evaluation data
More information needed
## Training procedure
### Training hyperparameters
The following hyperparameters were used during training:
- learning_rate: 4e-05
- train_batch_size: 128
- eval_batch_size: 128
- seed: 10
- distributed_type: multi-GPU
- optimizer: Adam with betas=(0.9,0.999) and epsilon=1e-08
- lr_scheduler_type: linear
- num_epochs: 50
### Training results
| Training Loss | Epoch | Step | Validation Loss | Matthews Correlation | Accuracy |
|:-------------:|:-----:|:----:|:---------------:|:--------------------:|:--------:|
| 0.6236 | 1.0 | 67 | 0.6185 | 0.0 | 0.6913 |
| 0.6196 | 2.0 | 134 | 0.6181 | 0.0 | 0.6913 |
| 0.6112 | 3.0 | 201 | 0.6188 | 0.0 | 0.6913 |
| 0.615 | 4.0 | 268 | 0.6175 | 0.0 | 0.6913 |
| 0.6116 | 5.0 | 335 | 0.6181 | 0.0 | 0.6913 |
| 0.6103 | 6.0 | 402 | 0.6183 | 0.0 | 0.6913 |
| 0.6132 | 7.0 | 469 | 0.6265 | 0.0 | 0.6913 |
| 0.6127 | 8.0 | 536 | 0.6186 | 0.0 | 0.6913 |
| 0.6096 | 9.0 | 603 | 0.6275 | 0.0 | 0.6913 |
### Framework versions
- Transformers 4.30.2
- Pytorch 1.14.0a0+410ce96
- Datasets 2.13.0
- Tokenizers 0.13.3
| [
"TEXT_CLASSIFICATION"
] | Non_BioNLP |
sentence-transformers/all-distilroberta-v1 | sentence-transformers | sentence-similarity | [
"sentence-transformers",
"pytorch",
"rust",
"onnx",
"safetensors",
"openvino",
"roberta",
"fill-mask",
"feature-extraction",
"sentence-similarity",
"transformers",
"en",
"dataset:s2orc",
"dataset:flax-sentence-embeddings/stackexchange_xml",
"dataset:ms_marco",
"dataset:gooaq",
"dataset:yahoo_answers_topics",
"dataset:code_search_net",
"dataset:search_qa",
"dataset:eli5",
"dataset:snli",
"dataset:multi_nli",
"dataset:wikihow",
"dataset:natural_questions",
"dataset:trivia_qa",
"dataset:embedding-data/sentence-compression",
"dataset:embedding-data/flickr30k-captions",
"dataset:embedding-data/altlex",
"dataset:embedding-data/simple-wiki",
"dataset:embedding-data/QQP",
"dataset:embedding-data/SPECTER",
"dataset:embedding-data/PAQ_pairs",
"dataset:embedding-data/WikiAnswers",
"arxiv:1904.06472",
"arxiv:2102.07033",
"arxiv:2104.08727",
"arxiv:1704.05179",
"arxiv:1810.09305",
"license:apache-2.0",
"autotrain_compatible",
"text-embeddings-inference",
"endpoints_compatible",
"region:us"
] | 1,646,263,745,000 | 2025-03-06T13:35:55 | 507,403 | 34 | ---
datasets:
- s2orc
- flax-sentence-embeddings/stackexchange_xml
- ms_marco
- gooaq
- yahoo_answers_topics
- code_search_net
- search_qa
- eli5
- snli
- multi_nli
- wikihow
- natural_questions
- trivia_qa
- embedding-data/sentence-compression
- embedding-data/flickr30k-captions
- embedding-data/altlex
- embedding-data/simple-wiki
- embedding-data/QQP
- embedding-data/SPECTER
- embedding-data/PAQ_pairs
- embedding-data/WikiAnswers
language: en
library_name: sentence-transformers
license: apache-2.0
pipeline_tag: sentence-similarity
tags:
- sentence-transformers
- feature-extraction
- sentence-similarity
- transformers
---
# all-distilroberta-v1
This is a [sentence-transformers](https://www.SBERT.net) model: It maps sentences & paragraphs to a 768 dimensional dense vector space and can be used for tasks like clustering or semantic search.
## Usage (Sentence-Transformers)
Using this model becomes easy when you have [sentence-transformers](https://www.SBERT.net) installed:
```
pip install -U sentence-transformers
```
Then you can use the model like this:
```python
from sentence_transformers import SentenceTransformer
sentences = ["This is an example sentence", "Each sentence is converted"]
model = SentenceTransformer('sentence-transformers/all-distilroberta-v1')
embeddings = model.encode(sentences)
print(embeddings)
```
## Usage (HuggingFace Transformers)
Without [sentence-transformers](https://www.SBERT.net), you can use the model like this: First, you pass your input through the transformer model, then you have to apply the right pooling-operation on-top of the contextualized word embeddings.
```python
from transformers import AutoTokenizer, AutoModel
import torch
import torch.nn.functional as F
#Mean Pooling - Take attention mask into account for correct averaging
def mean_pooling(model_output, attention_mask):
token_embeddings = model_output[0] #First element of model_output contains all token embeddings
input_mask_expanded = attention_mask.unsqueeze(-1).expand(token_embeddings.size()).float()
return torch.sum(token_embeddings * input_mask_expanded, 1) / torch.clamp(input_mask_expanded.sum(1), min=1e-9)
# Sentences we want sentence embeddings for
sentences = ['This is an example sentence', 'Each sentence is converted']
# Load model from HuggingFace Hub
tokenizer = AutoTokenizer.from_pretrained('sentence-transformers/all-distilroberta-v1')
model = AutoModel.from_pretrained('sentence-transformers/all-distilroberta-v1')
# Tokenize sentences
encoded_input = tokenizer(sentences, padding=True, truncation=True, return_tensors='pt')
# Compute token embeddings
with torch.no_grad():
model_output = model(**encoded_input)
# Perform pooling
sentence_embeddings = mean_pooling(model_output, encoded_input['attention_mask'])
# Normalize embeddings
sentence_embeddings = F.normalize(sentence_embeddings, p=2, dim=1)
print("Sentence embeddings:")
print(sentence_embeddings)
```
------
## Background
The project aims to train sentence embedding models on very large sentence level datasets using a self-supervised
contrastive learning objective. We used the pretrained [`distilroberta-base`](https://huggingface.co/distilroberta-base) model and fine-tuned in on a
1B sentence pairs dataset. We use a contrastive learning objective: given a sentence from the pair, the model should predict which out of a set of randomly sampled other sentences, was actually paired with it in our dataset.
We developped this model during the
[Community week using JAX/Flax for NLP & CV](https://discuss.huggingface.co/t/open-to-the-community-community-week-using-jax-flax-for-nlp-cv/7104),
organized by Hugging Face. We developped this model as part of the project:
[Train the Best Sentence Embedding Model Ever with 1B Training Pairs](https://discuss.huggingface.co/t/train-the-best-sentence-embedding-model-ever-with-1b-training-pairs/7354). We benefited from efficient hardware infrastructure to run the project: 7 TPUs v3-8, as well as intervention from Googles Flax, JAX, and Cloud team member about efficient deep learning frameworks.
## Intended uses
Our model is intented to be used as a sentence and short paragraph encoder. Given an input text, it ouptuts a vector which captures
the semantic information. The sentence vector may be used for information retrieval, clustering or sentence similarity tasks.
By default, input text longer than 128 word pieces is truncated.
## Training procedure
### Pre-training
We use the pretrained [`distilroberta-base`](https://huggingface.co/distilroberta-base). Please refer to the model card for more detailed information about the pre-training procedure.
### Fine-tuning
We fine-tune the model using a contrastive objective. Formally, we compute the cosine similarity from each possible sentence pairs from the batch.
We then apply the cross entropy loss by comparing with true pairs.
#### Hyper parameters
We trained ou model on a TPU v3-8. We train the model during 920k steps using a batch size of 512 (64 per TPU core).
We use a learning rate warm up of 500. The sequence length was limited to 128 tokens. We used the AdamW optimizer with
a 2e-5 learning rate. The full training script is accessible in this current repository: `train_script.py`.
#### Training data
We use the concatenation from multiple datasets to fine-tune our model. The total number of sentence pairs is above 1 billion sentences.
We sampled each dataset given a weighted probability which configuration is detailed in the `data_config.json` file.
| Dataset | Paper | Number of training tuples |
|--------------------------------------------------------|:----------------------------------------:|:--------------------------:|
| [Reddit comments (2015-2018)](https://github.com/PolyAI-LDN/conversational-datasets/tree/master/reddit) | [paper](https://arxiv.org/abs/1904.06472) | 726,484,430 |
| [S2ORC](https://github.com/allenai/s2orc) Citation pairs (Abstracts) | [paper](https://aclanthology.org/2020.acl-main.447/) | 116,288,806 |
| [WikiAnswers](https://github.com/afader/oqa#wikianswers-corpus) Duplicate question pairs | [paper](https://doi.org/10.1145/2623330.2623677) | 77,427,422 |
| [PAQ](https://github.com/facebookresearch/PAQ) (Question, Answer) pairs | [paper](https://arxiv.org/abs/2102.07033) | 64,371,441 |
| [S2ORC](https://github.com/allenai/s2orc) Citation pairs (Titles) | [paper](https://aclanthology.org/2020.acl-main.447/) | 52,603,982 |
| [S2ORC](https://github.com/allenai/s2orc) (Title, Abstract) | [paper](https://aclanthology.org/2020.acl-main.447/) | 41,769,185 |
| [Stack Exchange](https://huggingface.co/datasets/flax-sentence-embeddings/stackexchange_xml) (Title, Body) pairs | - | 25,316,456 |
| [MS MARCO](https://microsoft.github.io/msmarco/) triplets | [paper](https://doi.org/10.1145/3404835.3462804) | 9,144,553 |
| [GOOAQ: Open Question Answering with Diverse Answer Types](https://github.com/allenai/gooaq) | [paper](https://arxiv.org/pdf/2104.08727.pdf) | 3,012,496 |
| [Yahoo Answers](https://www.kaggle.com/soumikrakshit/yahoo-answers-dataset) (Title, Answer) | [paper](https://proceedings.neurips.cc/paper/2015/hash/250cf8b51c773f3f8dc8b4be867a9a02-Abstract.html) | 1,198,260 |
| [Code Search](https://huggingface.co/datasets/code_search_net) | - | 1,151,414 |
| [COCO](https://cocodataset.org/#home) Image captions | [paper](https://link.springer.com/chapter/10.1007%2F978-3-319-10602-1_48) | 828,395|
| [SPECTER](https://github.com/allenai/specter) citation triplets | [paper](https://doi.org/10.18653/v1/2020.acl-main.207) | 684,100 |
| [Yahoo Answers](https://www.kaggle.com/soumikrakshit/yahoo-answers-dataset) (Question, Answer) | [paper](https://proceedings.neurips.cc/paper/2015/hash/250cf8b51c773f3f8dc8b4be867a9a02-Abstract.html) | 681,164 |
| [Yahoo Answers](https://www.kaggle.com/soumikrakshit/yahoo-answers-dataset) (Title, Question) | [paper](https://proceedings.neurips.cc/paper/2015/hash/250cf8b51c773f3f8dc8b4be867a9a02-Abstract.html) | 659,896 |
| [SearchQA](https://huggingface.co/datasets/search_qa) | [paper](https://arxiv.org/abs/1704.05179) | 582,261 |
| [Eli5](https://huggingface.co/datasets/eli5) | [paper](https://doi.org/10.18653/v1/p19-1346) | 325,475 |
| [Flickr 30k](https://shannon.cs.illinois.edu/DenotationGraph/) | [paper](https://transacl.org/ojs/index.php/tacl/article/view/229/33) | 317,695 |
| [Stack Exchange](https://huggingface.co/datasets/flax-sentence-embeddings/stackexchange_xml) Duplicate questions (titles) | | 304,525 |
| AllNLI ([SNLI](https://nlp.stanford.edu/projects/snli/) and [MultiNLI](https://cims.nyu.edu/~sbowman/multinli/) | [paper SNLI](https://doi.org/10.18653/v1/d15-1075), [paper MultiNLI](https://doi.org/10.18653/v1/n18-1101) | 277,230 |
| [Stack Exchange](https://huggingface.co/datasets/flax-sentence-embeddings/stackexchange_xml) Duplicate questions (bodies) | | 250,519 |
| [Stack Exchange](https://huggingface.co/datasets/flax-sentence-embeddings/stackexchange_xml) Duplicate questions (titles+bodies) | | 250,460 |
| [Sentence Compression](https://github.com/google-research-datasets/sentence-compression) | [paper](https://www.aclweb.org/anthology/D13-1155/) | 180,000 |
| [Wikihow](https://github.com/pvl/wikihow_pairs_dataset) | [paper](https://arxiv.org/abs/1810.09305) | 128,542 |
| [Altlex](https://github.com/chridey/altlex/) | [paper](https://aclanthology.org/P16-1135.pdf) | 112,696 |
| [Quora Question Triplets](https://quoradata.quora.com/First-Quora-Dataset-Release-Question-Pairs) | - | 103,663 |
| [Simple Wikipedia](https://cs.pomona.edu/~dkauchak/simplification/) | [paper](https://www.aclweb.org/anthology/P11-2117/) | 102,225 |
| [Natural Questions (NQ)](https://ai.google.com/research/NaturalQuestions) | [paper](https://transacl.org/ojs/index.php/tacl/article/view/1455) | 100,231 |
| [SQuAD2.0](https://rajpurkar.github.io/SQuAD-explorer/) | [paper](https://aclanthology.org/P18-2124.pdf) | 87,599 |
| [TriviaQA](https://huggingface.co/datasets/trivia_qa) | - | 73,346 |
| **Total** | | **1,124,818,467** | | [
"QUESTION_ANSWERING"
] | Non_BioNLP |
henry931007/mfma | henry931007 | text-classification | [
"transformers",
"pytorch",
"electra",
"text-classification",
"autotrain_compatible",
"endpoints_compatible",
"region:us"
] | 1,651,587,627,000 | 2022-05-31T08:23:15 | 17 | 0 | ---
{}
---
## Pre-trained factual consistency checking model for abstractive summaries introduced in the following NAACL-22 paper.
from transformers import AutoModelforSequenceClassification
model = AutoModelforSequenceClassification("henry931007/mfma")
```
@inproceedings{lee2022mfma,
title={Masked Summarization to Generate Factually Inconsistent Summaries for Improved Factual Consistency Checking},
author={Hwanhee Lee and Kang Min Yoo and Joonsuk Park and Hwaran Lee and Kyomin Jung},
year={2022},
month={july},
booktitle={Findings of the Association for Computational Linguistics: NAACL 2022},
}
``` | [
"SUMMARIZATION"
] | Non_BioNLP |
mrovera/eventnet-ita | mrovera | null | [
"Frame Parsing",
"Event Extraction",
"it",
"dataset:mrovera/eventnet-ita",
"license:agpl-3.0",
"region:us"
] | 1,706,478,010,000 | 2024-03-16T16:09:02 | 0 | 0 | ---
datasets:
- mrovera/eventnet-ita
language:
- it
license: agpl-3.0
tags:
- Frame Parsing
- Event Extraction
task_categories:
- token-classification
---
# EventNet-ITA
The model is a full-text frame parser for events in Italian and it has been trained on [EventNet-ITA](https://huggingface.co/datasets/mrovera/eventnet-ita).
The model can be used for _full-text_ Frame Parsing and Event Extraction.
Please refer to the [paper](https://aclanthology.org/2024.latechclfl-1.9) for a more detailed description.
## Model Details
### Model Description
In its current version, EventNet-ITA is able to recognize and classifiy 205 semantic frames and their (specific) frame elements. The unit of analysis is the sentence.
### Direct Use
Provided with an input sequence of tokens, the model labels each token with the corresponding frame and/or frame element label(s).
```
La B-ENTITY*BEING_LOCATED|B-THEME*CONQUERING
cittadina I-ENTITY*BEING_LOCATED|I-THEME*CONQUERING
, O
posta B-BEING_LOCATED
a B-RELATIVE_LOCATION*BEING_LOCATED
est I-RELATIVE_LOCATION*BEING_LOCATED
del I-RELATIVE_LOCATION*BEING_LOCATED
corso I-RELATIVE_LOCATION*BEING_LOCATED
d' I-RELATIVE_LOCATION*BEING_LOCATED
acqua I-RELATIVE_LOCATION*BEING_LOCATED
, O
venne O
conquistata B-CONQUERING
, O
ma O
il B-EXPLOSIVE*DETONATE_EXPLOSIVE
ponte I-EXPLOSIVE*DETONATE_EXPLOSIVE
sul I-EXPLOSIVE*DETONATE_EXPLOSIVE
fiume I-EXPLOSIVE*DETONATE_EXPLOSIVE
era O
già O
stato O
fatto B-DETONATE_EXPLOSIVE
saltare I-DETONATE_EXPLOSIVE
regolarmente O
dai B-AGENT*DETONATE_EXPLOSIVE
genieri I-AGENT*DETONATE_EXPLOSIVE
francesi I-AGENT*DETONATE_EXPLOSIVE
. O
```
## Training Details
The model has been trained using [MaChAmp](https://github.com/machamp-nlp/machamp), a Python tookit supporting a variety of NLP tasks, by fine-tuning [this Italian BERT pretrained model](https://huggingface.co/dbmdz/bert-base-italian-xxl-cased).
Training hyperparameters:
- Batch size: 64
- Learning rate: 1.5e-3
All other hyperparameters have been left unchanged w.r.t. the default MaChAmp configuration for the multi-sequential token classification task.
### Training Data
Please refer to the [dataset repo](https://huggingface.co/datasets/mrovera/eventnet-ita).
### Model Re-training
In order to re-train the model, download the [dataset](https://huggingface.co/datasets/mrovera/eventnet-ita) and follow the instructions for training a [multiseq task](https://github.com/machamp-nlp/machamp/blob/master/docs/multiseq.md) in MaChAmp.
### Inference
EventNet-ITA's model can be used for Frame Parsing on new texts.
In order to do so, you have to follow a few simple steps.
1. Clone the github repo: `git clone https://github.com/machamp-nlp/machamp.git`
2. Download EventNet-ITA's model from this repo (450 MB) and move it into the `machamp` folder (where is up to you, by default MaChAmp saves trained models in the logs folder)
3. Save the data you want to use for prediction in a two-column tsv file, one word per line, with a placeholder in column 1, each sentence separated by a blank line (without placeholder), like this:
```
This _
is _
the _
first _
sentence _
. _
This _
is _
the _
second _
one _
. _
```
4. Follow the instruction for predicting with [MaChAmp](https://github.com/machamp-nlp/machamp) (see section "Prediction") using a fine-tuned model.
## Evaluation
The model has been evaluated on three folds, each time with a stratified split of the dataset, with a 80/10/10 train/dev/test ratio. Please see the paper for further details. Hereafter we report the synthetic values obtained by averaging the Precision, Recall and F1-score values of the three splits.
**Token-based** (**_relaxed_**) performance:
| | P | R | F1 |
|----------------------------|--------|---------|---------|
|Frames | 0.904 | 0.914 | **0.907** |
|Frames (weighted) | 0.909 | 0.919 | 0.913 |
|Frame Elements | 0.841 | 0.724 | **0.761** |
|Frames Elements (weighted) | 0.850 | 0.779 | 0.804 |
**Span-based** (**_strict_**) performance:
| | P | R | F1 |
|----------------------------|--------|---------|--------|
|Frames | 0.906 | 0.899 | **0.901** |
|Frames (weighted) | 0.909 | 0.903 | 0.905 |
|Frame Elements | 0.829 | 0.666 | **0.724** |
|Frames Elements (weighted) | 0.853 | 0.711 | 0.768 |
### Citation Information
If you use EventNet-ITA, please cite the following paper:
```
@inproceedings{rovera-2024-eventnet,
title = "{E}vent{N}et-{ITA}: {I}talian Frame Parsing for Events",
author = "Rovera, Marco",
editor = "Bizzoni, Yuri and
Degaetano-Ortlieb, Stefania and
Kazantseva, Anna and
Szpakowicz, Stan",
booktitle = "Proceedings of the 8th Joint SIGHUM Workshop on Computational Linguistics for Cultural Heritage, Social Sciences, Humanities and Literature (LaTeCH-CLfL 2024)",
year = "2024",
publisher = "Association for Computational Linguistics",
url = "https://aclanthology.org/2024.latechclfl-1.9",
pages = "77--90",
}
``` | [
"EVENT_EXTRACTION"
] | Non_BioNLP |
chrsvl/v01 | chrsvl | text-classification | [
"tensorboard",
"safetensors",
"distilbert",
"autotrain",
"text-classification",
"base_model:distilbert/distilbert-base-uncased",
"base_model:finetune:distilbert/distilbert-base-uncased",
"region:us"
] | 1,726,999,410,000 | 2024-09-22T10:04:24 | 5 | 0 | ---
base_model: distilbert/distilbert-base-uncased
tags:
- autotrain
- text-classification
widget:
- text: I love AutoTrain
---
# Model Trained Using AutoTrain
- Problem type: Text Classification
## Validation Metrics
loss: 0.5235588550567627
f1: 0.0
precision: 0.0
recall: 0.0
auc: 0.7857142857142857
accuracy: 0.7777777777777778
| [
"TEXT_CLASSIFICATION"
] | Non_BioNLP |
llmware/dragon-llama-7b-v0 | llmware | text-generation | [
"transformers",
"pytorch",
"gguf",
"text-generation",
"license:llama2",
"autotrain_compatible",
"endpoints_compatible",
"region:us",
"conversational"
] | 1,700,070,950,000 | 2023-12-14T11:07:30 | 226 | 7 | ---
license: llama2
---
# Model Card for Model ID
<!-- Provide a quick summary of what the model is/does. -->
dragon-llama-7b-v0 part of the dRAGon ("Delivering RAG On ...") model series, RAG-instruct trained on top of a LLama-2 base model.
DRAGON models have been fine-tuned with the specific objective of fact-based question-answering over complex business and legal documents with an emphasis on reducing hallucinations and providing short, clear answers for workflow automation.
### Benchmark Tests
Evaluated against the benchmark test: [RAG-Instruct-Benchmark-Tester](https://www.huggingface.co/datasets/llmware/rag_instruct_benchmark_tester)
Average of 2 Test Runs with 1 point for correct answer, 0.5 point for partial correct or blank / NF, 0.0 points for incorrect, and -1 points for hallucinations.
--**Accuracy Score**: **97.25** correct out of 100
--Not Found Classification: 92.50%
--Boolean: 95.00%
--Math/Logic: 63.75%
--Complex Questions (1-5): 3 (Medium)
--Summarization Quality (1-5): 3 (Coherent, extractive)
--Hallucinations: No hallucinations observed in test runs.
For test run results (and good indicator of target use cases), please see the files ("core_rag_test" and "answer_sheet" in this repo).
### Model Description
<!-- Provide a longer summary of what this model is. -->
- **Developed by:** llmware
- **Model type:** LLama-2
- **Language(s) (NLP):** English
- **License:** LLama 2 Community License Agreement
- **Finetuned from model:** Llama-2-7B-Base
### Direct Use
<!-- This section is for the model use without fine-tuning or plugging into a larger ecosystem/app. -->
DRAGON is designed for enterprise automation use cases, especially in knowledge-intensive industries, such as financial services,
legal and regulatory industries with complex information sources.
DRAGON models have been trained for common RAG scenarios, specifically: question-answering, key-value extraction, and basic summarization as the core instruction types
without the need for a lot of complex instruction verbiage - provide a text passage context, ask questions, and get clear fact-based responses.
## Bias, Risks, and Limitations
<!-- This section is meant to convey both technical and sociotechnical limitations. -->
Any model can provide inaccurate or incomplete information, and should be used in conjunction with appropriate safeguards and fact-checking mechanisms.
## How to Get Started with the Model
The fastest way to get started with dRAGon is through direct import in transformers:
from transformers import AutoTokenizer, AutoModelForCausalLM
tokenizer = AutoTokenizer.from_pretrained("dragon-llama-7b-v0")
model = AutoModelForCausalLM.from_pretrained("dragon-llama-7b-v0")
Please refer to the generation_test .py files in the Files repository, which includes 200 samples and script to test the model. The **generation_test_llmware_script.py** includes built-in llmware capabilities for fact-checking, as well as easy integration with document parsing and actual retrieval to swap out the test set for RAG workflow consisting of business documents.
The dRAGon model was fine-tuned with a simple "\<human> and \<bot>" wrapper, so to get the best results, wrap inference entries as:
full_prompt = "<human>: " + my_prompt + "\n" + "<bot>:"
The BLING model was fine-tuned with closed-context samples, which assume generally that the prompt consists of two sub-parts:
1. Text Passage Context, and
2. Specific question or instruction based on the text passage
To get the best results, package "my_prompt" as follows:
my_prompt = {{text_passage}} + "\n" + {{question/instruction}}
If you are using a HuggingFace generation script:
# prepare prompt packaging used in fine-tuning process
new_prompt = "<human>: " + entries["context"] + "\n" + entries["query"] + "\n" + "<bot>:"
inputs = tokenizer(new_prompt, return_tensors="pt")
start_of_output = len(inputs.input_ids[0])
# temperature: set at 0.3 for consistency of output
# max_new_tokens: set at 100 - may prematurely stop a few of the summaries
outputs = model.generate(
inputs.input_ids.to(device),
eos_token_id=tokenizer.eos_token_id,
pad_token_id=tokenizer.eos_token_id,
do_sample=True,
temperature=0.3,
max_new_tokens=100,
)
output_only = tokenizer.decode(outputs[0][start_of_output:],skip_special_tokens=True)
## Model Card Contact
Darren Oberst & llmware team | [
"SUMMARIZATION"
] | Non_BioNLP |
Xenova/opus-mt-en-sv | Xenova | translation | [
"transformers.js",
"onnx",
"marian",
"text2text-generation",
"translation",
"base_model:Helsinki-NLP/opus-mt-en-sv",
"base_model:quantized:Helsinki-NLP/opus-mt-en-sv",
"region:us"
] | 1,693,955,348,000 | 2024-10-08T13:42:02 | 60 | 0 | ---
base_model: Helsinki-NLP/opus-mt-en-sv
library_name: transformers.js
pipeline_tag: translation
---
https://huggingface.co/Helsinki-NLP/opus-mt-en-sv with ONNX weights to be compatible with Transformers.js.
Note: Having a separate repo for ONNX weights is intended to be a temporary solution until WebML gains more traction. If you would like to make your models web-ready, we recommend converting to ONNX using [🤗 Optimum](https://huggingface.co/docs/optimum/index) and structuring your repo like this one (with ONNX weights located in a subfolder named `onnx`). | [
"TRANSLATION"
] | Non_BioNLP |
elifcen/bert-pooling-based | elifcen | text-classification | [
"transformers",
"pytorch",
"tensorboard",
"bert",
"text-classification",
"generated_from_trainer",
"dataset:glue",
"license:apache-2.0",
"model-index",
"autotrain_compatible",
"endpoints_compatible",
"region:us"
] | 1,683,461,835,000 | 2023-05-07T16:40:37 | 14 | 0 | ---
datasets:
- glue
license: apache-2.0
metrics:
- matthews_correlation
tags:
- generated_from_trainer
model-index:
- name: bert-pooling-based
results:
- task:
type: text-classification
name: Text Classification
dataset:
name: glue
type: glue
config: cola
split: validation
args: cola
metrics:
- type: matthews_correlation
value: 0.40858564179092355
name: Matthews Correlation
---
<!-- This model card has been generated automatically according to the information the Trainer had access to. You
should probably proofread and complete it, then remove this comment. -->
# bert-pooling-based
This model is a fine-tuned version of [bert-base-uncased](https://huggingface.co/bert-base-uncased) on the glue dataset.
It achieves the following results on the evaluation set:
- Loss: 0.5115
- Matthews Correlation: 0.4086
## Model description
More information needed
## Intended uses & limitations
More information needed
## Training and evaluation data
More information needed
## Training procedure
### Training hyperparameters
The following hyperparameters were used during training:
- learning_rate: 1.7718352056354854e-06
- train_batch_size: 8
- eval_batch_size: 16
- seed: 42
- optimizer: Adam with betas=(0.9,0.999) and epsilon=1e-08
- lr_scheduler_type: linear
- num_epochs: 2
### Training results
| Training Loss | Epoch | Step | Validation Loss | Matthews Correlation |
|:-------------:|:-----:|:----:|:---------------:|:--------------------:|
| 0.5491 | 1.0 | 1069 | 0.5340 | 0.2513 |
| 0.4726 | 2.0 | 2138 | 0.5115 | 0.4086 |
### Framework versions
- Transformers 4.28.1
- Pytorch 2.0.0+cu118
- Datasets 2.12.0
- Tokenizers 0.13.3
| [
"TEXT_CLASSIFICATION"
] | Non_BioNLP |
FINGU-AI/Qwen2.5-32B-Lora-HQ-e-2 | FINGU-AI | null | [
"peft",
"safetensors",
"en",
"ko",
"zh",
"pt",
"ja",
"uz",
"tl",
"th",
"vi",
"id",
"base_model:Qwen/Qwen2.5-32B-Instruct",
"base_model:adapter:Qwen/Qwen2.5-32B-Instruct",
"license:mit",
"region:us"
] | 1,731,251,650,000 | 2024-11-10T15:16:58 | 8 | 0 | ---
base_model: Qwen/Qwen2.5-32B-Instruct
language:
- en
- ko
- zh
- pt
- ja
- uz
- tl
- th
- vi
- id
library_name: peft
license: mit
---
# FINGU-AI/Qwen2.5-32B-Lora-HQ-e-2
## Overview
`FINGU-AI/Qwen2.5-32B-Lora-HQ-e-2` is a powerful causal language model designed for a variety of natural language processing (NLP) tasks, including machine translation, text generation, and chat-based applications. This model is particularly useful for translating between Korean and Uzbek, as well as supporting other custom NLP tasks through flexible input.
## Model Details
- **Model ID**: `FINGU-AI/Qwen2.5-32B-Lora-HQ-e-2`
- **Architecture**: Causal Language Model (LM)
- **Parameters**: 32 billion
- **Precision**: Torch BF16 for efficient GPU memory usage
- **Attention**: SDPA (Scaled Dot-Product Attention)
- **Primary Use Case**: Translation (e.g., Korean to Uzbek), text generation, and dialogue systems.
## Example Usage
### Installation
Make sure to install the required packages:
```bash
pip install torch transformers
```
### Loading the Model
```python
from transformers import AutoTokenizer, AutoModelForCausalLM
import torch
# Model and Tokenizer
model_id = 'FINGU-AI/Qwen2.5-32B-Lora-HQ-e-2'
model = AutoModelForCausalLM.from_pretrained(model_id, attn_implementation="sdpa", torch_dtype=torch.bfloat16)
tokenizer = AutoTokenizer.from_pretrained(model_id)
model.to('cuda')
# Input Messages for Translation
messages = [
{"role": "system", "content": "translate korean to Uzbek"},
{"role": "user", "content": """새로운 은행 계좌를 개설하는 절차는 다음과 같습니다:
1. 계좌 개설 목적과 신분 확인을 위한 서류 제출
2. 서류 검토 과정을 거치는 것
3. 고객님의 신원 확인 절차를 진행하는 것
4. 모든 절차가 완료되면 계좌 개설이 가능합니다.
계좌 개설을 원하시는 경우, 신분증과 함께 방문해 주시면 됩니다.
"""},
]
# Tokenize and Generate Response
input_ids = tokenizer.apply_chat_template(
messages,
add_generation_prompt=True,
return_tensors="pt"
).to('cuda')
outputs = model.generate(
input_ids,
max_new_tokens=500,
do_sample=True,
)
# Decode and Print the Translation
response = outputs[0][input_ids.shape[-1]:]
print(tokenizer.decode(response, skip_special_tokens=True))
``` | [
"TRANSLATION"
] | Non_BioNLP |
isaacus/open-australian-legal-distilgpt2 | isaacus | text-generation | [
"transformers",
"pytorch",
"gpt2",
"text-generation",
"law",
"legal",
"australia",
"generated_from_trainer",
"en",
"dataset:umarbutler/open-australian-legal-corpus",
"base_model:distilbert/distilgpt2",
"base_model:finetune:distilbert/distilgpt2",
"license:apache-2.0",
"model-index",
"autotrain_compatible",
"text-generation-inference",
"endpoints_compatible",
"region:us"
] | 1,700,565,051,000 | 2023-12-15T04:21:43 | 138 | 1 | ---
base_model: distilgpt2
datasets:
- umarbutler/open-australian-legal-corpus
language:
- en
library_name: transformers
license: apache-2.0
metrics:
- perplexity
tags:
- law
- legal
- australia
- generated_from_trainer
widget:
- text: Under the Crimes Act
- text: Section 51 of the Constitution provides
- text: '"Unsatisfactory professional conduct" includes'
model-index:
- name: open-australian-legal-distilgpt2
results:
- task:
type: text-generation
name: Text generation
dataset:
name: Open Australian Legal QA
type: umarbutler/open-australian-legal-qa
split: train
revision: b53a24f8edf5eb33d033a53b5b53d0a4a220d4ae
metrics:
- type: perplexity
value: 23.904073945422713
name: Perplexity
source:
url: https://github.com/asahi417/lmppl
name: lmppl
---
⚠️ This model has been superseded by the [Open Australian Legal LLM](https://huggingface.co/umarbutler/open-australian-legal-llm), the largest open source language model trained on Australian law. You are encouraged to use that model instead. ⚠️
# Open Australian Legal DistilGPT2 ⚖️
Open Australian Legal DistilGPT2 is a DistilGPT2 model trained on Australian law.
Naturally, as a finetune of [DistilGPT2](https://huggingface.co/distilgpt2), the model may be used for any of the tasks for which [DistilGPT2](https://huggingface.co/distilgpt2) and its parent model, [GPT2](https://huggingface.co/gpt2), are suitable, including text generation, text completion and question answering.
Trained on 37,560 laws and regulations, comprising 635,482,112 tokens, taken from the [Open Australian Legal Corpus](https://huggingface.co/datasets/umarbutler/open-australian-legal-corpus), the model is intended specifically to be finetuned for downstream natural language processing tasks applied to the Australian legal domain.
To ensure its accessibility to as wide an audience as possible, the model is issued under the same licence as [DistilGPT2](https://huggingface.co/distilgpt2), namely the [Apache Licence 2.0](https://www.apache.org/licenses/LICENSE-2.0.html).
A larger, non-distilled version of the model, trained on the same dataset, is available [here](https://huggingface.co/umarbutler/open-australian-legal-gpt2).
## Usage 👩💻
The code snippet below demonstrates just one of the many ways in which the model may be accessed:
```python
>>> from transformers import pipeline, set_seed
>>> set_seed(42) # We set a seed for reproducibility.
>>> generator = pipeline('text-generation', model='umarbutler/open-australian-legal-distilgpt2')
>>> generator('Under the', max_length=20, num_return_sequences=5)
[{'generated_text': 'Under the purposes of Part 6 Division 2 of the Act, regulations may confer power on an applicant for'},
{'generated_text': 'Under the circumstances, in deciding which person to whom a protected information request may be made, the AP'},
{'generated_text': 'Under the provisions of this Act, an offence against section 51 or 52 of the Act that relates to'},
{'generated_text': 'Under the definition of State or Territory, the State or Territory in section 8 of the A New Tax'},
{'generated_text': 'Under the Act, a person is taken to be an occupier of premises if—\n\t('}]
```
## Creation 🧪
37,560 documents were sampled from the [Open Australian Legal Corpus](https://huggingface.co/datasets/umarbutler/open-australian-legal-corpus) by filtering for primary and secondary legislation that, when stripped of whitespace, was not empty. Such documents were then randomly shuffled and added to blocks 1,024-tokens-long, with GPT2's end-of-sequence token ('<|endoftext|>') being used as a delimiter as well as to pad the end of the final block, resulting in a training dataset of 620,588 blocks, or 635,482,112 tokens.
The training dataset was subsequently fed to [DistilGPT2](https://huggingface.co/distilgpt2) via [`transformers.Trainer`](https://huggingface.co/docs/transformers/main_classes/trainer) with the following hyperparameters:
| Hyperparameter | Value |
| --- | --- |
| Sequence length | 1,024 |
| Epochs | 3 |
| Optimiser | AdamW |
| Learning rate | 1e-5 |
| Learning rate scheduler | Linear with warmup |
| Batch size per device | 4 |
| Weight decay | 0.01 |
| Warmup ratio | 0.06 |
After training for 3 epochs, or 465,441 steps, over a period of ~40 hours on a single GeForce RTX 2080 Ti, the model achieved a loss of 0.65.
## Licence 📜
The model is issued under the same licence as [DistilGPT2](https://huggingface.co/distilgpt2), namely the [Apache Licence 2.0](https://www.apache.org/licenses/LICENSE-2.0.html).
## Citation 🔖
If you've relied on the model for your work, please cite:
```bibtex
@misc{butler-2023-open-australian-legal-distilgpt2,
author = {Butler, Umar},
year = {2023},
title = {Open Australian Legal DistilGPT2},
publisher = {Hugging Face},
version = {1.0.0},
url = {https://huggingface.co/datasets/umarbutler/open-australian-legal-distilgpt2}
}
```
## Acknowledgements 🙏
In the spirit of reconciliation, the author acknowledges the Traditional Custodians of Country throughout Australia and their connections to land, sea and community. He pays his respect to their Elders past and present and extends that respect to all Aboriginal and Torres Strait Islander peoples today.
The author thanks the sources of the [Open Australian Legal Corpus](https://huggingface.co/datasets/umarbutler/open-australian-legal-corpus) for making their data available under open licences.
The author also acknowledges the developers of the many Python libraries relied upon in the training of the model, as well as the makers of [DistilGPT2](https://huggingface.co/distilgpt2) and [GPT2](https://huggingface.co/gpt2), which the model was built atop.
Finally, the author is eternally grateful for the endless support of his wife and her willingness to put up with many a late night spent writing code and quashing bugs. | [
"QUESTION_ANSWERING"
] | Non_BioNLP |
IMISLab/GreekT5-umt5-small-greeksum | IMISLab | summarization | [
"transformers",
"pytorch",
"umt5",
"text2text-generation",
"summarization",
"el",
"arxiv:2311.07767",
"arxiv:2304.00869",
"license:apache-2.0",
"model-index",
"autotrain_compatible",
"endpoints_compatible",
"region:us"
] | 1,699,783,564,000 | 2024-08-02T09:14:11 | 31 | 1 | ---
language:
- el
license: apache-2.0
metrics:
- bertscore
- rouge
pipeline_tag: summarization
widget:
- text: 'Να πάρει ""ξεκάθαρη"" θέση σε σχέση με τον κίνδυνο μετάδοσης του κορονοϊού
από τη Θεία Κοινωνία καλεί την κυβέρνηση και τον Πρωθυπουργό με ανακοίνωσή
του τη Δευτέρα ο ΣΥΡΙΖΑ. ""Την ώρα που κλείνουν προληπτικά και ορθώς σχολεία,
πανεπιστήμια, γήπεδα και λαμβάνονται ειδικά μέτρα ακόμη και για την ορκωμοσία
της νέας Προέδρου της Δημοκρατίας, η Ιερά Σύνοδος της Εκκλησίας της Ελλάδος
επιμένει ότι το μυστήριο της Θείας Κοινωνίας δεν εγκυμονεί κινδύνους μετάδοσης
του κορονοϊού, καλώντας όμως τις ευπαθείς ομάδες να μείνουν σπίτι τους"",
αναφέρει η αξιωματική αντιπολίτευση και συνεχίζει: ""Ωστόσο το πρόβλημα
δεν είναι τι λέει η Ιερά Σύνοδος, αλλά τι λέει η Πολιτεία και συγκεκριμένα
ο ΕΟΔΥ και το Υπουργείο Υγείας, που έχουν και την αποκλειστική κοινωνική
ευθύνη για τη μη εξάπλωση του ιού και την προστασία των πολιτών"". ""Σε άλλες
ευρωπαϊκές χώρες με εξίσου μεγάλο σεβασμό στη Χριστιανική πίστη και στο
θρησκευτικό συναίσθημα, τα μυστήρια της Εκκλησίας είτε αναστέλλονται είτε
τροποποιούν το τελετουργικό τους. Μόνο στη χώρα μας έχουμε το θλιβερό προνόμιο
μιας πολιτείας που δεν τολμά να πει το αυτονόητο"", προσθέτει, τονίζοντας
ότι ""η κυβέρνηση λοιπόν και το Υπουργείο Υγείας οφείλουν να πάρουν δημόσια
μια ξεκάθαρη θέση και να μην θυσιάζουν τη δημόσια Υγεία στο βωμό του πολιτικού
κόστους"". ""Συμφωνούν ότι η Θεία Κοινωνία δεν εγκυμονεί κινδύνους μετάδοσης
του κορονοϊού; Δεν είναι θέμα ευσέβειας αλλά κοινωνικής ευθύνης. Και με
τη Δημόσια υγεία δεν μπορούμε να παίζουμε"", καταλήγει η ανακοίνωση του
γραφείου Τύπου του ΣΥΡΙΖΑ. *ΠΩΣ ΜΕΤΑΔΙΔΕΤΑΙ. Χρήσιμος οδηγός για να προστατευθείτε
από τον κορονοϊό *ΤΑ ΝΟΣΟΚΟΜΕΙΑ ΑΝΑΦΟΡΑΣ. Ποια θα υποδέχονται τα κρούσματα
κορονοϊού στην Ελλάδα. *ΤΑΞΙΔΙΑ. Κορονοϊός και αεροδρόμια: Τι να προσέξετε.
*Η ΕΠΙΔΗΜΙΑ ΣΤΟΝ ΠΛΑΝΗΤΗ. Δείτε LIVE χάρτη με την εξέλιξη του κορονοϊού.'
example_title: Politics
- text: 'Με άρθρο της με τίτλο ""Επιστρέψτε στη θεά Ίριδα το σώμα της"", η εφημερίδα
Washington Post τάσσεται υπέρ της επιστροφής των γλυπτών του Παρθενώνα, στην
Αθήνα, στην κοιτίδα του δυτικού πολιτισμού, τώρα που οι συνθήκες έχουν
αλλάξει για την πάλαι ποτέ αυτοκρατορία της Αγγλίας. Αναφερόμενη στις διαφορετικές
απόψεις Ελλήνων και Βρετανών για τα γλυπτά, η συντάκτρια του άρθρου, τονίζει
ότι το αίτημα επιστροφής έχει αποκτήσει μεγαλύτερο βάρος τώρα που το Ηνωμένο
Βασίλειο εγκαταλείπει την Ευρωπαϊκή Ένωση. «Όταν ο Τόμας Μπρους, έβδομος
κόμης του Έλγιν, και 11ος κόμης του Κινκαρντίν, ταξίδεψε στην Ακρόπολη στις
αρχές της δεκαετίας του 1800, ως Βρετανός πρέσβης στην Οθωμανική Αυτοκρατορία,
ο Σουλτάνος λέγεται ότι του έδωσε την άδεια να ""αφαιρέσει μερικά τμήματα
λίθων με παλιές επιγραφές και μορφές"". Ο λόρδος το εξέλαβε ως άδεια να
αφαιρέσει, περίπου, 17 αγάλματα από τα αετώματα, 15 μετώπες, και 247 πόδια
(περίπου 75 μέτρα) της ζωφόρου από τον Παρθενώνα για να τα φέρει στην καλή
μας Αγγλία» αναφέρει στο άρθρο της η Washington Post. Και συνεχίζει λέγοντας
ότι «οι καιροί όμως άλλαξαν και αυτό που θεωρούνταν πιο δικαιολογημένο
τότε, σήμερα θεωρείται ευρέως ως μια ασυνείδητη πράξη». Σε μία έμμεση
αναφορά στο Brexit, και υπεραμυνόμενη της επιστροφής των γλυπτών στην Ελλάδα,
η συντάκτρια του άρθρου της Washington Post, διερωτάται: «Γιατί να παραμείνουν
τα μάρμαρα στη φύλαξη της χώρας που επιμένει ότι ανήκει μόνο στον εαυτό
της;» και σημειώνει: «Η Ελλάδα τιμάται σήμερα ως λίκνο του δυτικού πολιτισμού,
και ποιοί παρά οι Έλληνες θα μπορούσαν να στεγάσουν τον πολιτισμό αυτό;».'
example_title: Culture
- text: Το Διεθνές Νομισματικό Ταμείο (ΔΝΤ) προβλέπει ένα χρέος ρεκόρ των πλούσιων
χωρών το 2014 και κρίνει ""πιθανό"" να υπάρξει επιπλέον συμβολή των πιο
εύπορων προσώπων και των πολυεθνικών επιχειρήσεων σε μια μείωση των ελλειμμάτων,
σύμφωνα με έκθεσή του η οποία δόθηκε σήμερα στη δημοσιότητα. ""Φαίνεται
ότι υπάρχει ένα επαρκές περιθώριο σε πολλές ανεπτυγμένες χώρες για να
αντληθούν επιπλέον έσοδα από τα πιο υψηλά εισοδήματα"", υπογραμμίζει το
ΔΝΤ στην έκθεσή του για την δημοσιονομική επιτήρηση. Κατά μέσον όρο, το
δημόσιο χρέος των ανεπτυγμένων χωρών αναμένεται να φτάσει το ""ιστορικό
υψηλό"" του 110% του ΑΕΠ τους το 2014, δηλαδή θα βρίσκεται 35 μονάδες πιο
πάνω από το ποσοστό του 2007, επισημαίνει το ΔΝΤ στην έκθεσή του. Με μια
αναλογία χρέους/ΑΕΠ της τάξης του 242,3% που προβλέπεται να έχει το 2014,
η Ιαπωνία αναμένεται να βρίσκεται πρώτη στον κατάλογο των υπερχρεωμένων
ανεπτυγμένων χωρών, ακολουθούμενη από την Ελλάδα (174%), την Ιταλία (133,1%)
και την Πορτογαλία (125,3%). Οι ΗΠΑ, οι οποίες έχουν παραλύσει από ένα δημοσιονομικό
αδιέξοδο και απειλούνται από μια πιθανή στάση πληρωμών, θα δουν το χρέος
τους να ανεβαίνει στο 107,3% του ΑΕΠ τους το 2014, δηλαδή θα βρίσκονται πολύ
πιο μπροστά από την Γαλλία και το 94,8% στο οποίο αναμένεται ότι θα ανέρχεται
την ερχόμενη χρονιά το χρέος της. Η δεύτερη οικονομική δύναμη του κόσμου,
η Κίνα δίνει την εικόνα του καλού μαθητή με μια αναλογία χρέους/ΑΕΠ μόνον
20,9% την ερχόμενη χρονιά, σύμφωνα με το ΔΝΤ. ""Παρά τις προόδους στη μείωση
των ελλειμμάτων, οι δημοσιονομικές αδυναμίες παραμένουν βαθιές στις ανεπτυγμένες
χώρες"", επισημαίνεται στην έκθεση. Απέναντι σε αυτές τις ανισορροπίες,
το ΔΝΤ εκφράζει την ανησυχία του καθώς βλέπει ""ένα φορολογικό σύστημα
υπό πίεση"", το οποίο ευνοεί τον ανταγωνισμό μεταξύ των κρατών και επιτρέπει
στους εύπορους φορολογούμενους και στις πολυεθνικές να ελαφρύνουν τους φόρους
τους. Μόνον στις ΗΠΑ, το ΔΝΤ υπολογίζει σε 60 δισεκατομμύρια δολάρια τα έσοδα
που φέρεται ότι χάνονται λόγω τεχνικών βελτιστοποίησης της φορολογίας των
πολυεθνικών. Το ΔΝΤ επισημαίνει ότι οι τελευταίες δεκαετίες έχουν σηματοδοτηθεί
από μια ""θεαματική άνοδο"" του πλούτου του ""1%"" των πιο πλούσιων, κυρίως
στον αγγλοσαξονικό κόσμο, χωρίς ωστόσο η φορολογία να έχει προσαρμοστεί
σε αυτήν την εξέλιξη. ""Σε πολλές χώρες θα ήταν πιθανό να επιβληθούν επιπλέον
φόροι σε αυτούς που διαθέτουν τα πιο υψηλά εισοδήματα"", υπογραμμίζει το
ΔΝΤ, το οποίο κρίνει εξάλλου ""συνετό"" τον υπολογισμό σε 4.500 δισεκατομμύρια
δολάρια των διαθεσίμων που αποκρύπτονται από ιδιώτες σε φορολογικούς παραδείσους.
Οι χώρες της Ομάδας των Είκοσι (G20), οι υπουργοί Οικονομικών των οποίων
συναντώνται αυτήν την εβδομάδα στην Ουάσινγκτον, ξεκίνησαν πρόσφατα πρωτοβουλίες
για την πάταξη της φοροδιαφυγής.
example_title: Economics
model-index:
- name: IMISLab/GreekT5-umt5-small-greeksum
results:
- task:
type: summarization
name: Summarization
dataset:
name: GreekSUM
type: greeksum
config: default
split: test
metrics:
- type: rouge
value: 25.49
name: ROUGE-1
verified: true
- type: rouge
value: 12.03
name: ROUGE-2
verified: true
- type: rouge
value: 21.32
name: ROUGE-L
verified: true
- type: bertscore
value: 72.86
name: BERTScore
verified: true
---
# GreekT5 (umt5-small-greeksum)
A Greek news summarization model trained on [GreekSum](https://github.com/iakovosevdaimon/GreekSUM).
This model is part of a series of models trained as part of our research paper:
[Giarelis, N., Mastrokostas, C., & Karacapilidis, N. (2024) GreekT5: Sequence-to-Sequence Models for Greek News Summarization](https://link.springer.com/chapter/10.1007/978-3-031-63215-0_5) [\[arxiv\]](https://arxiv.org/abs/2311.07767)
The proposed models were trained and evaluated on the same dataset against [GreekBART](https://arxiv.org/abs/2304.00869).
For more information see the evaluation section below.
## Training dataset
The training dataset of `GreekT5-umt5-small-greeksum` is [GreekSum](https://github.com/iakovosevdaimon/GreekSUM/), which is the first news summarization dataset for the Greek Language.
This dataset contains ~151,000 news articles collected from [News24/7](https://www.news247.gr/), belonging to various topics (i.e., society, politics, economy, culture or world news).
For more information see: [https://arxiv.org/abs/2304.00869](https://arxiv.org/abs/2304.00869)
## Training configuration
We trained `google/umt5-small` [300 million parameters (~1.20 GB)] on the GreekSUM train split using the following parameters:
* GPU batch size = 6
* Total training epochs = 10
* AdamW optimizer (e = 1e−8, β1 = 0.9 and β2 = 0.0999)
* Learning rate = 3e−4
* No warmup steps
* 32-bit floating precision
* Tokenization
* maximum input token length = 1024
* maximum output token length = 128
* padding = ‘max_length’
* truncation = True
**Note:** T5-based models use a multi-task architecture, the prefix *‘summarize: ’* was prepended in each training sample.
## Evaluation
**Approach**|**ROUGE-1**|**ROUGE-2**|**ROUGE-L**|**BERTScore**
------------|-----------|-----------|-----------|-------------
TextRank|18.10|5.76|13.84|68.39
GreekT5 (mt5-small)|14.84|1.68|12.39|72.96
**GreekT5 (umt5-small)**|25.49|12.03|21.32|72.86
GreekT5 (umt5-base)|**26.67**|**13.00**|**22.42**|73.41
GreekBART|17.43|2.44|15.08|**75.89**
### Example code
```python
from transformers import AutoModelForSeq2SeqLM, AutoTokenizer, pipeline
model_name = 'IMISLab/GreekT5-umt5-small-greeksum'
model = AutoModelForSeq2SeqLM.from_pretrained(model_name)
tokenizer = AutoTokenizer.from_pretrained(model_name)
summarizer = pipeline(
'summarization',
device = 'cpu',
model = model,
tokenizer = tokenizer,
max_new_tokens = 128,
truncation = True
)
text = 'Να πάρει ""ξεκάθαρη"" θέση σε σχέση με τον κίνδυνο μετάδοσης του κορονοϊού από τη Θεία Κοινωνία καλεί την κυβέρνηση και τον Πρωθυπουργό με ανακοίνωσή του τη Δευτέρα ο ΣΥΡΙΖΑ. ""Την ώρα που κλείνουν προληπτικά και ορθώς σχολεία, πανεπιστήμια, γήπεδα και λαμβάνονται ειδικά μέτρα ακόμη και για την ορκωμοσία της νέας Προέδρου της Δημοκρατίας, η Ιερά Σύνοδος της Εκκλησίας της Ελλάδος επιμένει ότι το μυστήριο της Θείας Κοινωνίας δεν εγκυμονεί κινδύνους μετάδοσης του κορονοϊού, καλώντας όμως τις ευπαθείς ομάδες να μείνουν σπίτι τους"", αναφέρει η αξιωματική αντιπολίτευση και συνεχίζει: ""Ωστόσο το πρόβλημα δεν είναι τι λέει η Ιερά Σύνοδος, αλλά τι λέει η Πολιτεία και συγκεκριμένα ο ΕΟΔΥ και το Υπουργείο Υγείας, που έχουν και την αποκλειστική κοινωνική ευθύνη για τη μη εξάπλωση του ιού και την προστασία των πολιτών"". ""Σε άλλες ευρωπαϊκές χώρες με εξίσου μεγάλο σεβασμό στη Χριστιανική πίστη και στο θρησκευτικό συναίσθημα, τα μυστήρια της Εκκλησίας είτε αναστέλλονται είτε τροποποιούν το τελετουργικό τους. Μόνο στη χώρα μας έχουμε το θλιβερό προνόμιο μιας πολιτείας που δεν τολμά να πει το αυτονόητο"", προσθέτει, τονίζοντας ότι ""η κυβέρνηση λοιπόν και το Υπουργείο Υγείας οφείλουν να πάρουν δημόσια μια ξεκάθαρη θέση και να μην θυσιάζουν τη δημόσια Υγεία στο βωμό του πολιτικού κόστους"". ""Συμφωνούν ότι η Θεία Κοινωνία δεν εγκυμονεί κινδύνους μετάδοσης του κορονοϊού; Δεν είναι θέμα ευσέβειας αλλά κοινωνικής ευθύνης. Και με τη Δημόσια υγεία δεν μπορούμε να παίζουμε"", καταλήγει η ανακοίνωση του γραφείου Τύπου του ΣΥΡΙΖΑ. *ΠΩΣ ΜΕΤΑΔΙΔΕΤΑΙ. Χρήσιμος οδηγός για να προστατευθείτε από τον κορονοϊό *ΤΑ ΝΟΣΟΚΟΜΕΙΑ ΑΝΑΦΟΡΑΣ. Ποια θα υποδέχονται τα κρούσματα κορονοϊού στην Ελλάδα. *ΤΑΞΙΔΙΑ. Κορονοϊός και αεροδρόμια: Τι να προσέξετε. *Η ΕΠΙΔΗΜΙΑ ΣΤΟΝ ΠΛΑΝΗΤΗ. Δείτε LIVE χάρτη με την εξέλιξη του κορονοϊού.'
output = summarizer('summarize: ' + text)
print(output[0]['summary_text'])
```
## Contact
If you have any questions/feedback about the model please e-mail one of the following authors:
```
[email protected]
[email protected]
[email protected]
```
## Citation
The model has been officially released with the article: [GreekT5: A Series of Greek Sequence-to-Sequence Models for News Summarization](https://arxiv.org/).
If you use the model, please cite the following:
```
@inproceedings{giarelis2024greekt5,
title={GreekT5: Sequence-to-Sequence Models for Greek News Summarization},
author={Giarelis, Nikolaos and Mastrokostas, Charalampos and Karacapilidis, Nikos},
booktitle={IFIP International Conference on Artificial Intelligence Applications and Innovations},
pages={60--73},
year={2024},
organization={Springer}
}
```
| [
"SUMMARIZATION"
] | Non_BioNLP |
lilanxi0221/distilbert-base-uncased-finetuned-mrpc | lilanxi0221 | text-classification | [
"transformers",
"pytorch",
"tensorboard",
"distilbert",
"text-classification",
"generated_from_trainer",
"dataset:glue",
"license:apache-2.0",
"model-index",
"autotrain_compatible",
"endpoints_compatible",
"region:us"
] | 1,670,130,713,000 | 2022-12-05T23:46:00 | 112 | 0 | ---
datasets:
- glue
license: apache-2.0
metrics:
- accuracy
- f1
tags:
- generated_from_trainer
model-index:
- name: distilbert-base-uncased-finetuned-mrpc
results:
- task:
type: text-classification
name: Text Classification
dataset:
name: glue
type: glue
config: mrpc
split: train
args: mrpc
metrics:
- type: accuracy
value: 0.8431372549019608
name: Accuracy
- type: f1
value: 0.891156462585034
name: F1
---
<!-- This model card has been generated automatically according to the information the Trainer had access to. You
should probably proofread and complete it, then remove this comment. -->
# distilbert-base-uncased-finetuned-mrpc
This model is a fine-tuned version of [distilbert-base-uncased](https://huggingface.co/distilbert-base-uncased) on the glue dataset.
It achieves the following results on the evaluation set:
- Loss: 0.5509
- Accuracy: 0.8431
- F1: 0.8912
## Model description
More information needed
## Intended uses & limitations
More information needed
## Training and evaluation data
More information needed
## Training procedure
### Training hyperparameters
The following hyperparameters were used during training:
- learning_rate: 2e-05
- train_batch_size: 16
- eval_batch_size: 16
- seed: 42
- optimizer: Adam with betas=(0.9,0.999) and epsilon=1e-08
- lr_scheduler_type: linear
- num_epochs: 5
### Training results
| Training Loss | Epoch | Step | Validation Loss | Accuracy | F1 |
|:-------------:|:-----:|:----:|:---------------:|:--------:|:------:|
| No log | 1.0 | 230 | 0.4740 | 0.7819 | 0.8499 |
| No log | 2.0 | 460 | 0.4150 | 0.8309 | 0.8821 |
| 0.4787 | 3.0 | 690 | 0.4475 | 0.8186 | 0.8706 |
| 0.4787 | 4.0 | 920 | 0.5340 | 0.8358 | 0.8885 |
| 0.2314 | 5.0 | 1150 | 0.5509 | 0.8431 | 0.8912 |
### Framework versions
- Transformers 4.25.1
- Pytorch 1.12.1+cu113
- Datasets 2.7.1
- Tokenizers 0.13.2
| [
"TEXT_CLASSIFICATION"
] | Non_BioNLP |
csam/finetuning-sentiment-model-3000-samples | csam | text-classification | [
"transformers",
"pytorch",
"tensorboard",
"distilbert",
"text-classification",
"generated_from_trainer",
"dataset:imdb",
"license:apache-2.0",
"model-index",
"autotrain_compatible",
"endpoints_compatible",
"region:us"
] | 1,665,572,468,000 | 2022-10-12T11:14:28 | 8 | 0 | ---
datasets:
- imdb
license: apache-2.0
metrics:
- accuracy
- f1
tags:
- generated_from_trainer
model-index:
- name: finetuning-sentiment-model-3000-samples
results:
- task:
type: text-classification
name: Text Classification
dataset:
name: imdb
type: imdb
config: plain_text
split: train
args: plain_text
metrics:
- type: accuracy
value: 0.88
name: Accuracy
- type: f1
value: 0.880794701986755
name: F1
---
<!-- This model card has been generated automatically according to the information the Trainer had access to. You
should probably proofread and complete it, then remove this comment. -->
# finetuning-sentiment-model-3000-samples
This model is a fine-tuned version of [distilbert-base-uncased](https://huggingface.co/distilbert-base-uncased) on the imdb dataset.
It achieves the following results on the evaluation set:
- Loss: 0.2913
- Accuracy: 0.88
- F1: 0.8808
## Model description
More information needed
## Intended uses & limitations
More information needed
## Training and evaluation data
More information needed
## Training procedure
### Training hyperparameters
The following hyperparameters were used during training:
- learning_rate: 2e-05
- train_batch_size: 16
- eval_batch_size: 16
- seed: 42
- optimizer: Adam with betas=(0.9,0.999) and epsilon=1e-08
- lr_scheduler_type: linear
- num_epochs: 2
### Training results
### Framework versions
- Transformers 4.23.1
- Pytorch 1.12.1+cu113
- Datasets 2.5.2
- Tokenizers 0.13.1
| [
"TEXT_CLASSIFICATION"
] | Non_BioNLP |
TheBloke/ALMA-13B-Pretrain-GPTQ | TheBloke | text-generation | [
"transformers",
"safetensors",
"llama",
"text-generation",
"arxiv:2309.11674",
"base_model:haoranxu/ALMA-13B-Pretrain",
"base_model:quantized:haoranxu/ALMA-13B-Pretrain",
"license:mit",
"autotrain_compatible",
"text-generation-inference",
"4-bit",
"gptq",
"region:us"
] | 1,695,427,640,000 | 2023-09-27T12:54:04 | 59 | 1 | ---
base_model: haoranxu/ALMA-13B-Pretrain
license: mit
model_name: ALMA 13B Pretrain
inference: false
model_creator: haoranxu
model_type: llama
prompt_template: 'Translate this from Chinese to English:
Chinese: {prompt}
English:
'
quantized_by: TheBloke
---
<!-- header start -->
<!-- 200823 -->
<div style="width: auto; margin-left: auto; margin-right: auto">
<img src="https://i.imgur.com/EBdldam.jpg" alt="TheBlokeAI" style="width: 100%; min-width: 400px; display: block; margin: auto;">
</div>
<div style="display: flex; justify-content: space-between; width: 100%;">
<div style="display: flex; flex-direction: column; align-items: flex-start;">
<p style="margin-top: 0.5em; margin-bottom: 0em;"><a href="https://discord.gg/theblokeai">Chat & support: TheBloke's Discord server</a></p>
</div>
<div style="display: flex; flex-direction: column; align-items: flex-end;">
<p style="margin-top: 0.5em; margin-bottom: 0em;"><a href="https://www.patreon.com/TheBlokeAI">Want to contribute? TheBloke's Patreon page</a></p>
</div>
</div>
<div style="text-align:center; margin-top: 0em; margin-bottom: 0em"><p style="margin-top: 0.25em; margin-bottom: 0em;">TheBloke's LLM work is generously supported by a grant from <a href="https://a16z.com">andreessen horowitz (a16z)</a></p></div>
<hr style="margin-top: 1.0em; margin-bottom: 1.0em;">
<!-- header end -->
# ALMA 13B Pretrain - GPTQ
- Model creator: [haoranxu](https://huggingface.co/haoranxu)
- Original model: [ALMA 13B Pretrain](https://huggingface.co/haoranxu/ALMA-13B-Pretrain)
<!-- description start -->
## Description
This repo contains GPTQ model files for [haoranxu's ALMA 13B Pretrain](https://huggingface.co/haoranxu/ALMA-13B-Pretrain).
Multiple GPTQ parameter permutations are provided; see Provided Files below for details of the options provided, their parameters, and the software used to create them.
<!-- description end -->
<!-- repositories-available start -->
## Repositories available
* [AWQ model(s) for GPU inference.](https://huggingface.co/TheBloke/ALMA-13B-Pretrain-AWQ)
* [GPTQ models for GPU inference, with multiple quantisation parameter options.](https://huggingface.co/TheBloke/ALMA-13B-Pretrain-GPTQ)
* [2, 3, 4, 5, 6 and 8-bit GGUF models for CPU+GPU inference](https://huggingface.co/TheBloke/ALMA-13B-Pretrain-GGUF)
* [haoranxu's original unquantised fp16 model in pytorch format, for GPU inference and for further conversions](https://huggingface.co/haoranxu/ALMA-13B-Pretrain)
<!-- repositories-available end -->
<!-- prompt-template start -->
## Prompt template: ALMA
```
Translate this from Chinese to English:
Chinese: {prompt}
English:
```
<!-- prompt-template end -->
<!-- licensing start -->
## Licensing
The creator of the source model has listed its license as `mit`, and this quantization has therefore used that same license.
As this model is based on Llama 2, it is also subject to the Meta Llama 2 license terms, and the license files for that are additionally included. It should therefore be considered as being claimed to be licensed under both licenses. I contacted Hugging Face for clarification on dual licensing but they do not yet have an official position. Should this change, or should Meta provide any feedback on this situation, I will update this section accordingly.
In the meantime, any questions regarding licensing, and in particular how these two licenses might interact, should be directed to the original model repository: [haoranxu's ALMA 13B Pretrain](https://huggingface.co/haoranxu/ALMA-13B-Pretrain).
<!-- licensing end -->
<!-- README_GPTQ.md-provided-files start -->
## Provided files, and GPTQ parameters
Multiple quantisation parameters are provided, to allow you to choose the best one for your hardware and requirements.
Each separate quant is in a different branch. See below for instructions on fetching from different branches.
All recent GPTQ files are made with AutoGPTQ, and all files in non-main branches are made with AutoGPTQ. Files in the `main` branch which were uploaded before August 2023 were made with GPTQ-for-LLaMa.
<details>
<summary>Explanation of GPTQ parameters</summary>
- Bits: The bit size of the quantised model.
- GS: GPTQ group size. Higher numbers use less VRAM, but have lower quantisation accuracy. "None" is the lowest possible value.
- Act Order: True or False. Also known as `desc_act`. True results in better quantisation accuracy. Some GPTQ clients have had issues with models that use Act Order plus Group Size, but this is generally resolved now.
- Damp %: A GPTQ parameter that affects how samples are processed for quantisation. 0.01 is default, but 0.1 results in slightly better accuracy.
- GPTQ dataset: The calibration dataset used during quantisation. Using a dataset more appropriate to the model's training can improve quantisation accuracy. Note that the GPTQ calibration dataset is not the same as the dataset used to train the model - please refer to the original model repo for details of the training dataset(s).
- Sequence Length: The length of the dataset sequences used for quantisation. Ideally this is the same as the model sequence length. For some very long sequence models (16+K), a lower sequence length may have to be used. Note that a lower sequence length does not limit the sequence length of the quantised model. It only impacts the quantisation accuracy on longer inference sequences.
- ExLlama Compatibility: Whether this file can be loaded with ExLlama, which currently only supports Llama models in 4-bit.
</details>
| Branch | Bits | GS | Act Order | Damp % | GPTQ Dataset | Seq Len | Size | ExLlama | Desc |
| ------ | ---- | -- | --------- | ------ | ------------ | ------- | ---- | ------- | ---- |
| [main](https://huggingface.co/TheBloke/ALMA-13B-Pretrain-GPTQ/tree/main) | 4 | 128 | Yes | 0.1 | [wikitext](https://huggingface.co/datasets/wikitext/viewer/wikitext-2-v1/test) | 4096 | 7.26 GB | Yes | 4-bit, with Act Order and group size 128g. Uses even less VRAM than 64g, but with slightly lower accuracy. |
| [gptq-4bit-32g-actorder_True](https://huggingface.co/TheBloke/ALMA-13B-Pretrain-GPTQ/tree/gptq-4bit-32g-actorder_True) | 4 | 32 | Yes | 0.1 | [wikitext](https://huggingface.co/datasets/wikitext/viewer/wikitext-2-v1/test) | 4096 | 8.00 GB | Yes | 4-bit, with Act Order and group size 32g. Gives highest possible inference quality, with maximum VRAM usage. |
| [gptq-8bit--1g-actorder_True](https://huggingface.co/TheBloke/ALMA-13B-Pretrain-GPTQ/tree/gptq-8bit--1g-actorder_True) | 8 | None | Yes | 0.1 | [wikitext](https://huggingface.co/datasets/wikitext/viewer/wikitext-2-v1/test) | 4096 | 13.36 GB | No | 8-bit, with Act Order. No group size, to lower VRAM requirements. |
| [gptq-8bit-128g-actorder_True](https://huggingface.co/TheBloke/ALMA-13B-Pretrain-GPTQ/tree/gptq-8bit-128g-actorder_True) | 8 | 128 | Yes | 0.1 | [wikitext](https://huggingface.co/datasets/wikitext/viewer/wikitext-2-v1/test) | 4096 | 13.65 GB | No | 8-bit, with group size 128g for higher inference quality and with Act Order for even higher accuracy. |
| [gptq-8bit-32g-actorder_True](https://huggingface.co/TheBloke/ALMA-13B-Pretrain-GPTQ/tree/gptq-8bit-32g-actorder_True) | 8 | 32 | Yes | 0.1 | [wikitext](https://huggingface.co/datasets/wikitext/viewer/wikitext-2-v1/test) | 4096 | 14.54 GB | No | 8-bit, with group size 32g and Act Order for maximum inference quality. |
<!-- README_GPTQ.md-provided-files end -->
<!-- README_GPTQ.md-download-from-branches start -->
## How to download, including from branches
### In text-generation-webui
To download from the `main` branch, enter `TheBloke/ALMA-13B-Pretrain-GPTQ` in the "Download model" box.
To download from another branch, add `:branchname` to the end of the download name, eg `TheBloke/ALMA-13B-Pretrain-GPTQ:gptq-4bit-32g-actorder_True`
### From the command line
I recommend using the `huggingface-hub` Python library:
```shell
pip3 install huggingface-hub
```
To download the `main` branch to a folder called `ALMA-13B-Pretrain-GPTQ`:
```shell
mkdir ALMA-13B-Pretrain-GPTQ
huggingface-cli download TheBloke/ALMA-13B-Pretrain-GPTQ --local-dir ALMA-13B-Pretrain-GPTQ --local-dir-use-symlinks False
```
To download from a different branch, add the `--revision` parameter:
```shell
mkdir ALMA-13B-Pretrain-GPTQ
huggingface-cli download TheBloke/ALMA-13B-Pretrain-GPTQ --revision gptq-4bit-32g-actorder_True --local-dir ALMA-13B-Pretrain-GPTQ --local-dir-use-symlinks False
```
<details>
<summary>More advanced huggingface-cli download usage</summary>
If you remove the `--local-dir-use-symlinks False` parameter, the files will instead be stored in the central Huggingface cache directory (default location on Linux is: `~/.cache/huggingface`), and symlinks will be added to the specified `--local-dir`, pointing to their real location in the cache. This allows for interrupted downloads to be resumed, and allows you to quickly clone the repo to multiple places on disk without triggering a download again. The downside, and the reason why I don't list that as the default option, is that the files are then hidden away in a cache folder and it's harder to know where your disk space is being used, and to clear it up if/when you want to remove a download model.
The cache location can be changed with the `HF_HOME` environment variable, and/or the `--cache-dir` parameter to `huggingface-cli`.
For more documentation on downloading with `huggingface-cli`, please see: [HF -> Hub Python Library -> Download files -> Download from the CLI](https://huggingface.co/docs/huggingface_hub/guides/download#download-from-the-cli).
To accelerate downloads on fast connections (1Gbit/s or higher), install `hf_transfer`:
```shell
pip3 install hf_transfer
```
And set environment variable `HF_HUB_ENABLE_HF_TRANSFER` to `1`:
```shell
mkdir ALMA-13B-Pretrain-GPTQ
HF_HUB_ENABLE_HF_TRANSFER=1 huggingface-cli download TheBloke/ALMA-13B-Pretrain-GPTQ --local-dir ALMA-13B-Pretrain-GPTQ --local-dir-use-symlinks False
```
Windows Command Line users: You can set the environment variable by running `set HF_HUB_ENABLE_HF_TRANSFER=1` before the download command.
</details>
### With `git` (**not** recommended)
To clone a specific branch with `git`, use a command like this:
```shell
git clone --single-branch --branch gptq-4bit-32g-actorder_True https://huggingface.co/TheBloke/ALMA-13B-Pretrain-GPTQ
```
Note that using Git with HF repos is strongly discouraged. It will be much slower than using `huggingface-hub`, and will use twice as much disk space as it has to store the model files twice (it stores every byte both in the intended target folder, and again in the `.git` folder as a blob.)
<!-- README_GPTQ.md-download-from-branches end -->
<!-- README_GPTQ.md-text-generation-webui start -->
## How to easily download and use this model in [text-generation-webui](https://github.com/oobabooga/text-generation-webui).
Please make sure you're using the latest version of [text-generation-webui](https://github.com/oobabooga/text-generation-webui).
It is strongly recommended to use the text-generation-webui one-click-installers unless you're sure you know how to make a manual install.
1. Click the **Model tab**.
2. Under **Download custom model or LoRA**, enter `TheBloke/ALMA-13B-Pretrain-GPTQ`.
- To download from a specific branch, enter for example `TheBloke/ALMA-13B-Pretrain-GPTQ:gptq-4bit-32g-actorder_True`
- see Provided Files above for the list of branches for each option.
3. Click **Download**.
4. The model will start downloading. Once it's finished it will say "Done".
5. In the top left, click the refresh icon next to **Model**.
6. In the **Model** dropdown, choose the model you just downloaded: `ALMA-13B-Pretrain-GPTQ`
7. The model will automatically load, and is now ready for use!
8. If you want any custom settings, set them and then click **Save settings for this model** followed by **Reload the Model** in the top right.
* Note that you do not need to and should not set manual GPTQ parameters any more. These are set automatically from the file `quantize_config.json`.
9. Once you're ready, click the **Text Generation tab** and enter a prompt to get started!
<!-- README_GPTQ.md-text-generation-webui end -->
<!-- README_GPTQ.md-use-from-python start -->
## How to use this GPTQ model from Python code
### Install the necessary packages
Requires: Transformers 4.33.0 or later, Optimum 1.12.0 or later, and AutoGPTQ 0.4.2 or later.
```shell
pip3 install transformers optimum
pip3 install auto-gptq --extra-index-url https://huggingface.github.io/autogptq-index/whl/cu118/ # Use cu117 if on CUDA 11.7
```
If you have problems installing AutoGPTQ using the pre-built wheels, install it from source instead:
```shell
pip3 uninstall -y auto-gptq
git clone https://github.com/PanQiWei/AutoGPTQ
cd AutoGPTQ
git checkout v0.4.2
pip3 install .
```
### You can then use the following code
```python
from transformers import AutoModelForCausalLM, AutoTokenizer, pipeline
model_name_or_path = "TheBloke/ALMA-13B-Pretrain-GPTQ"
# To use a different branch, change revision
# For example: revision="gptq-4bit-32g-actorder_True"
model = AutoModelForCausalLM.from_pretrained(model_name_or_path,
device_map="auto",
trust_remote_code=False,
revision="main")
tokenizer = AutoTokenizer.from_pretrained(model_name_or_path, use_fast=True)
prompt = "Tell me about AI"
prompt_template=f'''Translate this from Chinese to English:
Chinese: {prompt}
English:
'''
print("\n\n*** Generate:")
input_ids = tokenizer(prompt_template, return_tensors='pt').input_ids.cuda()
output = model.generate(inputs=input_ids, temperature=0.7, do_sample=True, top_p=0.95, top_k=40, max_new_tokens=512)
print(tokenizer.decode(output[0]))
# Inference can also be done using transformers' pipeline
print("*** Pipeline:")
pipe = pipeline(
"text-generation",
model=model,
tokenizer=tokenizer,
max_new_tokens=512,
do_sample=True,
temperature=0.7,
top_p=0.95,
top_k=40,
repetition_penalty=1.1
)
print(pipe(prompt_template)[0]['generated_text'])
```
<!-- README_GPTQ.md-use-from-python end -->
<!-- README_GPTQ.md-compatibility start -->
## Compatibility
The files provided are tested to work with AutoGPTQ, both via Transformers and using AutoGPTQ directly. They should also work with [Occ4m's GPTQ-for-LLaMa fork](https://github.com/0cc4m/KoboldAI).
[ExLlama](https://github.com/turboderp/exllama) is compatible with Llama models in 4-bit. Please see the Provided Files table above for per-file compatibility.
[Huggingface Text Generation Inference (TGI)](https://github.com/huggingface/text-generation-inference) is compatible with all GPTQ models.
<!-- README_GPTQ.md-compatibility end -->
<!-- footer start -->
<!-- 200823 -->
## Discord
For further support, and discussions on these models and AI in general, join us at:
[TheBloke AI's Discord server](https://discord.gg/theblokeai)
## Thanks, and how to contribute
Thanks to the [chirper.ai](https://chirper.ai) team!
Thanks to Clay from [gpus.llm-utils.org](llm-utils)!
I've had a lot of people ask if they can contribute. I enjoy providing models and helping people, and would love to be able to spend even more time doing it, as well as expanding into new projects like fine tuning/training.
If you're able and willing to contribute it will be most gratefully received and will help me to keep providing more models, and to start work on new AI projects.
Donaters will get priority support on any and all AI/LLM/model questions and requests, access to a private Discord room, plus other benefits.
* Patreon: https://patreon.com/TheBlokeAI
* Ko-Fi: https://ko-fi.com/TheBlokeAI
**Special thanks to**: Aemon Algiz.
**Patreon special mentions**: Alicia Loh, Stephen Murray, K, Ajan Kanaga, RoA, Magnesian, Deo Leter, Olakabola, Eugene Pentland, zynix, Deep Realms, Raymond Fosdick, Elijah Stavena, Iucharbius, Erik Bjäreholt, Luis Javier Navarrete Lozano, Nicholas, theTransient, John Detwiler, alfie_i, knownsqashed, Mano Prime, Willem Michiel, Enrico Ros, LangChain4j, OG, Michael Dempsey, Pierre Kircher, Pedro Madruga, James Bentley, Thomas Belote, Luke @flexchar, Leonard Tan, Johann-Peter Hartmann, Illia Dulskyi, Fen Risland, Chadd, S_X, Jeff Scroggin, Ken Nordquist, Sean Connelly, Artur Olbinski, Swaroop Kallakuri, Jack West, Ai Maven, David Ziegler, Russ Johnson, transmissions 11, John Villwock, Alps Aficionado, Clay Pascal, Viktor Bowallius, Subspace Studios, Rainer Wilmers, Trenton Dambrowitz, vamX, Michael Levine, 준교 김, Brandon Frisco, Kalila, Trailburnt, Randy H, Talal Aujan, Nathan Dryer, Vadim, 阿明, ReadyPlayerEmma, Tiffany J. Kim, George Stoitzev, Spencer Kim, Jerry Meng, Gabriel Tamborski, Cory Kujawski, Jeffrey Morgan, Spiking Neurons AB, Edmond Seymore, Alexandros Triantafyllidis, Lone Striker, Cap'n Zoog, Nikolai Manek, danny, ya boyyy, Derek Yates, usrbinkat, Mandus, TL, Nathan LeClaire, subjectnull, Imad Khwaja, webtim, Raven Klaugh, Asp the Wyvern, Gabriel Puliatti, Caitlyn Gatomon, Joseph William Delisle, Jonathan Leane, Luke Pendergrass, SuperWojo, Sebastain Graf, Will Dee, Fred von Graf, Andrey, Dan Guido, Daniel P. Andersen, Nitin Borwankar, Elle, Vitor Caleffi, biorpg, jjj, NimbleBox.ai, Pieter, Matthew Berman, terasurfer, Michael Davis, Alex, Stanislav Ovsiannikov
Thank you to all my generous patrons and donaters!
And thank you again to a16z for their generous grant.
<!-- footer end -->
# Original model card: haoranxu's ALMA 13B Pretrain
**ALMA** (**A**dvanced **L**anguage **M**odel-based tr**A**nslator) is an LLM-based translation model, which adopts a new translation model paradigm: it begins with fine-tuning on monolingual data and is further optimized using high-quality parallel data. This two-step fine-tuning process ensures strong translation performance.
Please find more details in our [paper](https://arxiv.org/abs/2309.11674).
```
@misc{xu2023paradigm,
title={A Paradigm Shift in Machine Translation: Boosting Translation Performance of Large Language Models},
author={Haoran Xu and Young Jin Kim and Amr Sharaf and Hany Hassan Awadalla},
year={2023},
eprint={2309.11674},
archivePrefix={arXiv},
primaryClass={cs.CL}
}
```
We release four translation models presented in the paper:
- **ALMA-7B**: Full-weight Fine-tune LLaMA-2-7B on 20B monolingual tokens and then **Full-weight** fine-tune on human-written parallel data
- **ALMA-7B-LoRA**: Full-weight Fine-tune LLaMA-2-7B on 20B monolingual tokens and then **LoRA** fine-tune on human-written parallel data
- **ALMA-13B**: Full-weight Fine-tune LLaMA-2-7B on 12B monolingual tokens and then **Full-weight** fine-tune on human-written parallel data
- **ALMA-13B-LoRA** (Our best system): Full-weight Fine-tune LLaMA-2-7B on 12B monolingual tokens and then **LoRA** fine-tune on human-written parallel data
Model checkpoints are released at huggingface:
| Models | Base Model Link | LoRA Link |
|:-------------:|:---------------:|:---------:|
| ALMA-7B | [haoranxu/ALMA-7B](https://huggingface.co/haoranxu/ALMA-7B) | - |
| ALMA-7B-LoRA | [haoranxu/ALMA-7B-Pretrain](https://huggingface.co/haoranxu/ALMA-7B-Pretrain) | [haoranxu/ALMA-7B-Pretrain-LoRA](https://huggingface.co/haoranxu/ALMA-7B-Pretrain-LoRA) |
| ALMA-13B | [haoranxu/ALMA-13B](https://huggingface.co/haoranxu/ALMA-13B) | - |
| ALMA-13B-LoRA | [haoranxu/ALMA-13B-Pretrain](https://huggingface.co/haoranxu/ALMA-13B-Pretrain) | [haoranxu/ALMA-13B-Pretrain-LoRA](https://huggingface.co/haoranxu/ALMA-13B-Pretrain-LoRA) |
Note that Base Model Link for `*-LoRA` models are LLaMA-2 fine-tuned by monolingual data (20B for the 7B model and 12B for the 13B model)
A quick start to use our best system (ALMA-13B-LoRA) for translation. An example of translating "我爱机器翻译。" into English:
```
import torch
from peft import PeftModel
from transformers import AutoModelForCausalLM
from transformers import LlamaTokenizer
# Load base model and LoRA weights
model = AutoModelForCausalLM.from_pretrained("haoranxu/ALMA-13B-Pretrain", torch_dtype=torch.float16, device_map="auto")
model = PeftModel.from_pretrained(model, "haoranxu/ALMA-13B-Pretrain-LoRA")
tokenizer = LlamaTokenizer.from_pretrained("haoranxu/ALMA-13B-Pretrain", padding_side='left')
# Add the source setence into the prompt template
prompt="Translate this from Chinese to English:\nChinese: 我爱机器翻译。\nEnglish:"
input_ids = tokenizer(prompt, return_tensors="pt", padding=True, max_length=40, truncation=True).input_ids.cuda()
# Translation
with torch.no_grad():
generated_ids = model.generate(input_ids=input_ids, num_beams=5, max_new_tokens=20, do_sample=True, temperature=0.6, top_p=0.9)
outputs = tokenizer.batch_decode(generated_ids, skip_special_tokens=True)
print(outputs)
```
Please find more details in our [GitHub repository](https://github.com/fe1ixxu/ALMA)
| [
"TRANSLATION"
] | Non_BioNLP |
sealad886/Llama3-OpenBioLLM-8B | sealad886 | text-generation | [
"transformers",
"safetensors",
"llama",
"text-generation",
"llama-3",
"Mixtral",
"instruct",
"finetune",
"chatml",
"DPO",
"RLHF",
"gpt4",
"distillation",
"en",
"arxiv:2305.18290",
"arxiv:2303.13375",
"arxiv:2212.13138",
"arxiv:2305.09617",
"arxiv:2402.07023",
"base_model:meta-llama/Meta-Llama-3-8B",
"base_model:finetune:meta-llama/Meta-Llama-3-8B",
"license:llama3",
"autotrain_compatible",
"text-generation-inference",
"endpoints_compatible",
"region:us"
] | 1,717,624,607,000 | 2024-06-20T19:19:07 | 22 | 0 | ---
base_model: meta-llama/Meta-Llama-3-8B
language:
- en
license: llama3
tags:
- llama-3
- llama
- Mixtral
- instruct
- finetune
- chatml
- DPO
- RLHF
- gpt4
- distillation
widget:
- example_title: OpenBioLLM-8B
messages:
- role: system
content: You are an expert and experienced from the healthcare and biomedical
domain with extensive medical knowledge and practical experience.
- role: user
content: How long does it take for newborn jaundice to go away?
output:
text: 'Newborn jaundice, also known as neonatal jaundice, is a common condition
in newborns where the yellowing of the skin and eyes occurs due to an elevated
level of bilirubin in the blood. Bilirubin is a yellow pigment that forms when
red blood cells break down. In most cases, newborn jaundice resolves on its
own without any specific treatment.
The duration of newborn jaundice can vary depending on several factors such
as the underlying cause, gestational age at birth, and individual variations
in bilirubin metabolism. Here are some general guidelines:
1. Physiological jaundice: This is the most common type of newborn jaundice
and usually appears within 24-72 hours after birth. It tends to peak between
the second and fifth day of life and gradually improves over the next week or
two. By the time the baby is one week old, the jaundice should have mostly resolved.
2. Breast milk jaundice: This type of jaundice occurs in breastfed babies and
may appear later than physiological jaundice, typically between the fifth and
fourteenth day of life. It tends to persist for a longer duration but usually
resolves within six weeks after birth. 3. Pathological jaundice: This type of
jaundice is less common and occurs due to an underlying medical condition that
affects bilirubin metabolism or liver function. The duration of pathological
jaundice depends on the specific cause and may require treatment.
It''s important for parents to monitor their newborn''s jaundice closely and
seek medical advice if the jaundice progresses rapidly, becomes severe, or is
accompanied by other symptoms such as poor feeding, lethargy, or excessive sleepiness.
In these cases, further evaluation and management may be necessary. Remember
that each baby is unique, and the timing of jaundice resolution can vary. If
you have concerns about your newborn''s jaundice, it''s always best to consult
with a healthcare professional for personalized advice and guidance.'
model-index:
- name: OpenBioLLM-8B
results: []
---
<div align="center">
<h2 class="alert">Copied pickled Pytorch files from <a href="https://huggingface.co/aaditya/Llama3-OpenBioLLM-8B">aaditya/Llama3-OpenBioLLM-8B</a> for conversion to Safetensors using <a href="https://huggingface.co/spaces/safetensors/convert">https://huggingface.co/spaces/safetensors/convert</a>.
<br>Original Model Card:</h2>
</div>
<div align="center">
<img width="260px" src="https://cdn-uploads.huggingface.co/production/uploads/5f3fe13d79c1ba4c353d0c19/BrQCb95lmEIFz79QAmoNA.png"></div>

<div align="center">
<h1>Advancing Open-source Large Language Models in Medical Domain</h1>
</div>
<p align="center" style="margin-top: 0px;">
<a href="https://colab.research.google.com/drive/1F5oV20InEYeAJGmBwYF9NM_QhLmjBkKJ?usp=sharing">
<img src="https://colab.research.google.com/assets/colab-badge.svg" alt="OpenChat Logo" style="width:20px; vertical-align: middle; display: inline-block; margin-right: 5px; margin-left: 10px; margin-top: 0px; margin-bottom: 0px;"/>
<span class="link-text" style=" margin-right: 5px;">Online Demo</span>
</a> |
<a href="https://github.com/openlifescience-ai">
<img src="https://github.githubassets.com/assets/GitHub-Mark-ea2971cee799.png" alt="GitHub Logo" style="width:20px; vertical-align: middle; display: inline-block; margin-right: 5px; margin-left: 5px; margin-top: 0px; margin-bottom: 0px;"/>
<span class="link-text" style=" margin-right: 5px;">GitHub</span>
</a> |
<a href="#">
<img src="https://github.com/alpayariyak/openchat/blob/master/assets/arxiv-logomark-small-square-border.png?raw=true" alt="ArXiv Logo" style="width:20px; vertical-align: middle; display: inline-block; margin-right: 5px; margin-left: 5px; margin-top: 0px; margin-bottom: 0px;"/>
<span class="link-text" style="margin-right: 5px;">Paper</span>
</a> |
<a href="https://discord.gg/A5Fjf5zC69">
<img src="https://cloud.githubusercontent.com/assets/6291467/26705903/96c2d66e-477c-11e7-9f4e-f3c0efe96c9a.png" alt="Discord Logo" style="width:20px; vertical-align: middle; display: inline-block; margin-right: 5px; margin-left: 5px; margin-top: 0px; margin-bottom: 0px;"/>
<span class="link-text">Discord</span>
</a>
</p>

Introducing OpenBioLLM-8B: A State-of-the-Art Open Source Biomedical Large Language Model
OpenBioLLM-8B is an advanced open source language model designed specifically for the biomedical domain. Developed by Saama AI Labs, this model leverages cutting-edge techniques to achieve state-of-the-art performance on a wide range of biomedical tasks.
🏥 **Biomedical Specialization**: OpenBioLLM-8B is tailored for the unique language and knowledge requirements of the medical and life sciences fields. It was fine-tuned on a vast corpus of high-quality biomedical data, enabling it to understand and generate text with domain-specific accuracy and fluency.
🎓 **Superior Performance**: With 8 billion parameters, OpenBioLLM-8B outperforms other open source biomedical language models of similar scale. It has also demonstrated better results compared to larger proprietary & open-source models like GPT-3.5 and Meditron-70B on biomedical benchmarks.
🧠 **Advanced Training Techniques**: OpenBioLLM-8B builds upon the powerful foundations of the **Meta-Llama-3-8B** and [Meta-Llama-3-8B](meta-llama/Meta-Llama-3-8B) models. It incorporates the DPO dataset and fine-tuning recipe along with a custom diverse medical instruction dataset. Key components of the training pipeline include:
<div align="center">
<img width="1200px" src="https://cdn-uploads.huggingface.co/production/uploads/5f3fe13d79c1ba4c353d0c19/oPchsJsEpQoGcGXVbh7YS.png">
</div>
- **Policy Optimization**: [Direct Preference Optimization: Your Language Model is Secretly a Reward Model (DPO)](https://arxiv.org/abs/2305.18290)
- **Ranking Dataset**: [berkeley-nest/Nectar](https://huggingface.co/datasets/berkeley-nest/Nectar)
- **Fine-tuning dataset**: Custom Medical Instruct dataset (We plan to release a sample training dataset in our upcoming paper; please stay updated)
This combination of cutting-edge techniques enables OpenBioLLM-8B to align with key capabilities and preferences for biomedical applications.
⚙️ **Release Details**:
- **Model Size**: 8 billion parameters
- **Quantization**: Optimized quantized versions available [Here](https://huggingface.co/aaditya/OpenBioLLM-Llama3-8B-GGUF)
- **Language(s) (NLP):** en
- **Developed By**: [Ankit Pal (Aaditya Ura)](https://aadityaura.github.io/) from Saama AI Labs
- **License:** Meta-Llama License
- **Fine-tuned from models:** [meta-llama/Meta-Llama-3-8B](meta-llama/Meta-Llama-3-8B)
- **Resources for more information:**
- Paper: Coming soon
The model can be fine-tuned for more specialized tasks and datasets as needed.
OpenBioLLM-8B represents an important step forward in democratizing advanced language AI for the biomedical community. By leveraging state-of-the-art architectures and training techniques from leading open source efforts like Llama-3, we have created a powerful tool to accelerate innovation and discovery in healthcare and the life sciences.
We are excited to share OpenBioLLM-8B with researchers and developers around the world.
### Use with transformers
**Important: Please use the exact chat template provided by Llama-3 instruct version. Otherwise there will be a degradation in the performance. The model output can be verbose in rare cases. Please consider setting temperature = 0 to make this happen less.**
See the snippet below for usage with Transformers:
```python
import transformers
import torch
model_id = "aaditya/OpenBioLLM-Llama3-8B"
pipeline = transformers.pipeline(
"text-generation",
model=model_id,
model_kwargs={"torch_dtype": torch.bfloat16},
device="auto",
)
messages = [
{"role": "system", "content": "You are an expert and experienced from the healthcare and biomedical domain with extensive medical knowledge and practical experience. Your name is OpenBioLLM, and you were developed by Saama AI Labs. who's willing to help answer the user's query with explanation. In your explanation, leverage your deep medical expertise such as relevant anatomical structures, physiological processes, diagnostic criteria, treatment guidelines, or other pertinent medical concepts. Use precise medical terminology while still aiming to make the explanation clear and accessible to a general audience."},
{"role": "user", "content": "How can i split a 3mg or 4mg waefin pill so i can get a 2.5mg pill?"},
]
prompt = pipeline.tokenizer.apply_chat_template(
messages,
tokenize=False,
add_generation_prompt=True
)
terminators = [
pipeline.tokenizer.eos_token_id,
pipeline.tokenizer.convert_tokens_to_ids("<|eot_id|>")
]
outputs = pipeline(
prompt,
max_new_tokens=256,
eos_token_id=terminators,
do_sample=True,
temperature=0.0,
top_p=0.9,
)
print(outputs[0]["generated_text"][len(prompt):])
```
## **Training procedure**
### **Training hyperparameters**
<details>
<summary>Click to see details</summary>
- learning_rate: 0.0002
- lr_scheduler: cosine
- train_batch_size: 12
- eval_batch_size: 8
- GPU: H100 80GB SXM5
- num_devices: 1
- optimizer: adamw_bnb_8bit
- lr_scheduler_warmup_steps: 100
- num_epochs: 4
</details>
### **Peft hyperparameters**
<details>
<summary>Click to see details</summary>
- adapter: qlora
- lora_r: 128
- lora_alpha: 256
- lora_dropout: 0.05
- lora_target_linear: true
-lora_target_modules:
- q_proj
- v_proj
- k_proj
- o_proj
- gate_proj
- down_proj
- up_proj
</details>
### **Training results**
### **Framework versions**
- Transformers 4.39.3
- Pytorch 2.1.2+cu121
- Datasets 2.18.0
- Tokenizers 0.15.1
- Axolotl
- Lm harness for evaluation
# Benchmark Results
🔥 OpenBioLLM-8B demonstrates superior performance compared to larger models, such as GPT-3.5, Meditron-70B across 9 diverse biomedical datasets, achieving state-of-the-art results with an average score of 72.50%, despite having a significantly smaller parameter count. The model's strong performance in domain-specific tasks, such as Clinical KG, Medical Genetics, and PubMedQA, highlights its ability to effectively capture and apply biomedical knowledge.
🚨 The GPT-4, Med-PaLM-1, and Med-PaLM-2 results are taken from their official papers. Since Med-PaLM doesn't provide zero-shot accuracy, we are using 5-shot accuracy from their paper for comparison. All results presented are in the zero-shot setting, except for Med-PaLM-2 and Med-PaLM-1, which use 5-shot accuracy.
| | Clinical KG | Medical Genetics | Anatomy | Pro Medicine | College Biology | College Medicine | MedQA 4 opts | PubMedQA | MedMCQA | Avg |
|--------------------|-------------|------------------|---------|--------------|-----------------|------------------|--------------|----------|---------|-------|
| **OpenBioLLM-70B** | **92.93** | **93.197** | **83.904** | 93.75 | 93.827 | **85.749** | 78.162 | 78.97 | **74.014** | **86.05588** |
| Med-PaLM-2 (5-shot) | 88.3 | 90 | 77.8 | **95.2** | 94.4 | 80.9 | **79.7** | **79.2** | 71.3 | 84.08 |
| **GPT-4** | 86.04 | 91 | 80 | 93.01 | **95.14** | 76.88 | 78.87 | 75.2 | 69.52 | 82.85 |
| Med-PaLM-1 (Flan-PaLM, 5-shot) | 80.4 | 75 | 63.7 | 83.8 | 88.9 | 76.3 | 67.6 | 79 | 57.6 | 74.7 |
| **OpenBioLLM-8B** | 76.101 | 86.1 | 69.829 | 78.21 | 84.213 | 68.042 | 58.993 | 74.12 | 56.913 | 72.502 |
| Gemini-1.0 | 76.7 | 75.8 | 66.7 | 77.7 | 88 | 69.2 | 58 | 70.7 | 54.3 | 70.79 |
| GPT-3.5 Turbo 1106 | 74.71 | 74 | 72.79 | 72.79 | 72.91 | 64.73 | 57.71 | 72.66 | 53.79 | 66 |
| Meditron-70B | 66.79 | 69 | 53.33 | 71.69 | 76.38 | 63 | 57.1 | 76.6 | 46.85 | 64.52 |
| gemma-7b | 69.81 | 70 | 59.26 | 66.18 | 79.86 | 60.12 | 47.21 | 76.2 | 48.96 | 64.18 |
| Mistral-7B-v0.1 | 68.68 | 71 | 55.56 | 68.38 | 68.06 | 59.54 | 50.82 | 75.4 | 48.2 | 62.85 |
| Apollo-7B | 62.26 | 72 | 61.48 | 69.12 | 70.83 | 55.49 | 55.22 | 39.8 | 53.77 | 60 |
| MedAlpaca-7b | 57.36 | 69 | 57.04 | 67.28 | 65.28 | 54.34 | 41.71 | 72.8 | 37.51 | 58.03 |
| BioMistral-7B | 59.9 | 64 | 56.5 | 60.4 | 59 | 54.7 | 50.6 | 77.5 | 48.1 | 57.3 |
| AlpaCare-llama2-7b | 49.81 | 49 | 45.92 | 33.82 | 50 | 43.35 | 29.77 | 72.2 | 34.42 | 45.36 |
| ClinicalGPT | 30.56 | 27 | 30.37 | 19.48 | 25 | 24.27 | 26.08 | 63.8 | 28.18 | 30.52 |
<div align="center">
<img width="1600px" src="https://cdn-uploads.huggingface.co/production/uploads/5f3fe13d79c1ba4c353d0c19/_SzdcJSBjZyo8RS1bTEkP.png">
</div>
## Detailed Medical Subjectwise accuracy

# Use Cases & Examples
🚨 **Below results are from the quantized version of OpenBioLLM-70B**
# Summarize Clinical Notes
OpenBioLLM-70B can efficiently analyze and summarize complex clinical notes, EHR data, and discharge summaries, extracting key information and generating concise, structured summaries

# Answer Medical Questions
OpenBioLLM-70B can provide answers to a wide range of medical questions.


<details>
<summary>Click to see details</summary>



</details>
# Clinical Entity Recognition
OpenBioLLM-70B can perform advanced clinical entity recognition by identifying and extracting key medical concepts, such as diseases, symptoms, medications, procedures, and anatomical structures, from unstructured clinical text. By leveraging its deep understanding of medical terminology and context, the model can accurately annotate and categorize clinical entities, enabling more efficient information retrieval, data analysis, and knowledge discovery from electronic health records, research articles, and other biomedical text sources. This capability can support various downstream applications, such as clinical decision support, pharmacovigilance, and medical research.



# Biomarkers Extraction

# Classification
OpenBioLLM-70B can perform various biomedical classification tasks, such as disease prediction, sentiment analysis, medical document categorization

# De-Identification
OpenBioLLM-70B can detect and remove personally identifiable information (PII) from medical records, ensuring patient privacy and compliance with data protection regulations like HIPAA.

**Advisory Notice!**
While OpenBioLLM-70B & 8B leverages high-quality data sources, its outputs may still contain inaccuracies, biases, or misalignments that could pose risks if relied upon for medical decision-making without further testing and refinement. The model's performance has not yet been rigorously evaluated in randomized controlled trials or real-world healthcare environments.
Therefore, we strongly advise against using OpenBioLLM-70B & 8B for any direct patient care, clinical decision support, or other professional medical purposes at this time. Its use should be limited to research, development, and exploratory applications by qualified individuals who understand its limitations.
OpenBioLLM-70B & 8B are intended solely as a research tool to assist healthcare professionals and should never be considered a replacement for the professional judgment and expertise of a qualified medical doctor.
Appropriately adapting and validating OpenBioLLM-70B & 8B for specific medical use cases would require significant additional work, potentially including:
- Thorough testing and evaluation in relevant clinical scenarios
- Alignment with evidence-based guidelines and best practices
- Mitigation of potential biases and failure modes
- Integration with human oversight and interpretation
- Compliance with regulatory and ethical standards
Always consult a qualified healthcare provider for personal medical needs.
# Citation
If you find OpenBioLLM-70B & 8B useful in your work, please cite the model as follows:
```
@misc{OpenBioLLMs,
author = {Ankit Pal, Malaikannan Sankarasubbu},
title = {OpenBioLLMs: Advancing Open-Source Large Language Models for Healthcare and Life Sciences},
year = {2024},
publisher = {Hugging Face},
journal = {Hugging Face repository},
howpublished = {\url{https://huggingface.co/aaditya/OpenBioLLM-Llama3-70B}}
}
```
The accompanying paper is currently in progress and will be released soon.
<div align="center">
<h2> 💌 Contact </h2>
</div>
We look forward to hearing you and collaborating on this exciting project!
**Contributors:**
- [Ankit Pal (Aaditya Ura)](https://aadityaura.github.io/) [aadityaura at gmail dot com]
- Saama AI Labs
- Note: I am looking for a funded PhD opportunity, especially if it fits my Responsible Generative AI, Multimodal LLMs, Geometric Deep Learning, and Healthcare AI skillset.
# References
We thank the [Meta Team](meta-llama/Meta-Llama-3-70B-Instruct) for their amazing models!
Result sources
- [1] GPT-4 [Capabilities of GPT-4 on Medical Challenge Problems] (https://arxiv.org/abs/2303.13375)
- [2] Med-PaLM-1 [Large Language Models Encode Clinical Knowledge](https://arxiv.org/abs/2212.13138)
- [3] Med-PaLM-2 [Towards Expert-Level Medical Question Answering with Large Language Models](https://arxiv.org/abs/2305.09617)
- [4] Gemini-1.0 [Gemini Goes to Med School](https://arxiv.org/abs/2402.07023) | [
"QUESTION_ANSWERING"
] | BioNLP |
JustFrederik/sugoi-v3.3-ja-en-ct2-int8 | JustFrederik | translation | [
"transformers",
"translation",
"ja",
"en",
"license:unknown",
"endpoints_compatible",
"region:us"
] | 1,683,648,665,000 | 2023-05-09T16:34:57 | 8 | 1 | ---
language:
- ja
- en
license: unknown
pipeline_tag: translation
---
https://sugoitranslator.com
<br />
https://blog.sugoitranslator.com
<br />
https://www.patreon.com/mingshiba
<br />
```
ct2-fairseq-converter --model_path big.pretrain.pt --data_dir . --source_lang ja --target_lang en --quantization int8 --output_dir ../converted/sugoi-v3.3-ja-en-ct2-int8
``` | [
"TRANSLATION"
] | Non_BioNLP |
whitesugarwater/distilbert-base-uncased_emotion_ft_0724 | whitesugarwater | text-classification | [
"transformers",
"safetensors",
"distilbert",
"text-classification",
"generated_from_trainer",
"dataset:emotion",
"base_model:distilbert/distilbert-base-uncased",
"base_model:finetune:distilbert/distilbert-base-uncased",
"license:apache-2.0",
"model-index",
"autotrain_compatible",
"endpoints_compatible",
"region:us"
] | 1,721,788,000,000 | 2024-07-24T03:30:35 | 11 | 0 | ---
base_model: distilbert-base-uncased
datasets:
- emotion
license: apache-2.0
metrics:
- accuracy
- f1
- precision
tags:
- generated_from_trainer
model-index:
- name: distilbert-base-uncased_emotion_ft_0724
results:
- task:
type: text-classification
name: Text Classification
dataset:
name: emotion
type: emotion
config: split
split: validation
args: split
metrics:
- type: accuracy
value: 0.9375
name: Accuracy
- type: f1
value: 0.9373640970475606
name: F1
- type: precision
value: 0.9201223706600051
name: Precision
---
<!-- This model card has been generated automatically according to the information the Trainer had access to. You
should probably proofread and complete it, then remove this comment. -->
# distilbert-base-uncased_emotion_ft_0724
This model is a fine-tuned version of [distilbert-base-uncased](https://huggingface.co/distilbert-base-uncased) on the emotion dataset.
It achieves the following results on the evaluation set:
- Loss: 0.1657
- Accuracy: 0.9375
- F1: 0.9374
- Precision: 0.9201
## Model description
More information needed
## Intended uses & limitations
More information needed
## Training and evaluation data
More information needed
## Training procedure
### Training hyperparameters
The following hyperparameters were used during training:
- learning_rate: 2e-05
- train_batch_size: 64
- eval_batch_size: 64
- seed: 42
- optimizer: Adam with betas=(0.9,0.999) and epsilon=1e-08
- lr_scheduler_type: linear
- num_epochs: 4
### Training results
| Training Loss | Epoch | Step | Validation Loss | Accuracy | F1 | Precision |
|:-------------:|:-----:|:----:|:---------------:|:--------:|:------:|:---------:|
| 0.1212 | 1.0 | 250 | 0.1532 | 0.9365 | 0.9370 | 0.9067 |
| 0.0935 | 2.0 | 500 | 0.1595 | 0.936 | 0.9366 | 0.9009 |
| 0.0728 | 3.0 | 750 | 0.1639 | 0.9345 | 0.9345 | 0.9104 |
| 0.0563 | 4.0 | 1000 | 0.1657 | 0.9375 | 0.9374 | 0.9201 |
### Framework versions
- Transformers 4.40.2
- Pytorch 2.2.2+cu121
- Datasets 2.14.6
- Tokenizers 0.19.1
| [
"TEXT_CLASSIFICATION"
] | Non_BioNLP |
Realgon/distilbert_imdb_padding30model | Realgon | text-classification | [
"transformers",
"pytorch",
"tensorboard",
"safetensors",
"distilbert",
"text-classification",
"generated_from_trainer",
"dataset:imdb",
"base_model:distilbert/distilbert-base-uncased",
"base_model:finetune:distilbert/distilbert-base-uncased",
"license:apache-2.0",
"model-index",
"autotrain_compatible",
"endpoints_compatible",
"region:us"
] | 1,701,181,257,000 | 2023-12-01T07:43:41 | 106 | 0 | ---
base_model: distilbert-base-uncased
datasets:
- imdb
license: apache-2.0
metrics:
- accuracy
tags:
- generated_from_trainer
model-index:
- name: distilbert_imdb_padding30model
results:
- task:
type: text-classification
name: Text Classification
dataset:
name: imdb
type: imdb
config: plain_text
split: test
args: plain_text
metrics:
- type: accuracy
value: 0.93324
name: Accuracy
---
<!-- This model card has been generated automatically according to the information the Trainer had access to. You
should probably proofread and complete it, then remove this comment. -->
# distilbert_imdb_padding30model
This model is a fine-tuned version of [distilbert-base-uncased](https://huggingface.co/distilbert-base-uncased) on the imdb dataset.
It achieves the following results on the evaluation set:
- Loss: 0.7240
- Accuracy: 0.9332
## Model description
More information needed
## Intended uses & limitations
More information needed
## Training and evaluation data
More information needed
## Training procedure
### Training hyperparameters
The following hyperparameters were used during training:
- learning_rate: 2e-05
- train_batch_size: 16
- eval_batch_size: 16
- seed: 42
- optimizer: Adam with betas=(0.9,0.999) and epsilon=1e-08
- lr_scheduler_type: linear
- num_epochs: 20
### Training results
| Training Loss | Epoch | Step | Validation Loss | Accuracy |
|:-------------:|:-----:|:-----:|:---------------:|:--------:|
| 0.2379 | 1.0 | 1563 | 0.2408 | 0.9134 |
| 0.1715 | 2.0 | 3126 | 0.2436 | 0.928 |
| 0.1026 | 3.0 | 4689 | 0.2785 | 0.9294 |
| 0.061 | 4.0 | 6252 | 0.3530 | 0.9286 |
| 0.0454 | 5.0 | 7815 | 0.4828 | 0.9204 |
| 0.0378 | 6.0 | 9378 | 0.4618 | 0.9289 |
| 0.0234 | 7.0 | 10941 | 0.4958 | 0.9269 |
| 0.0239 | 8.0 | 12504 | 0.5093 | 0.9275 |
| 0.0196 | 9.0 | 14067 | 0.4641 | 0.9294 |
| 0.0135 | 10.0 | 15630 | 0.6116 | 0.9277 |
| 0.0128 | 11.0 | 17193 | 0.6289 | 0.9278 |
| 0.012 | 12.0 | 18756 | 0.6210 | 0.9299 |
| 0.0087 | 13.0 | 20319 | 0.6698 | 0.93 |
| 0.0063 | 14.0 | 21882 | 0.6935 | 0.927 |
| 0.0045 | 15.0 | 23445 | 0.6642 | 0.9286 |
| 0.0034 | 16.0 | 25008 | 0.6603 | 0.9318 |
| 0.0009 | 17.0 | 26571 | 0.6836 | 0.9332 |
| 0.0011 | 18.0 | 28134 | 0.7181 | 0.9316 |
| 0.0004 | 19.0 | 29697 | 0.7155 | 0.9329 |
| 0.0004 | 20.0 | 31260 | 0.7240 | 0.9332 |
### Framework versions
- Transformers 4.33.2
- Pytorch 2.0.1+cu117
- Datasets 2.14.5
- Tokenizers 0.13.3
| [
"TEXT_CLASSIFICATION"
] | Non_BioNLP |
TheBloke/genz-13B-v2-AWQ | TheBloke | text-generation | [
"transformers",
"safetensors",
"llama",
"text-generation",
"en",
"base_model:budecosystem/genz-13b-v2",
"base_model:quantized:budecosystem/genz-13b-v2",
"license:llama2",
"autotrain_compatible",
"text-generation-inference",
"4-bit",
"awq",
"region:us"
] | 1,697,324,144,000 | 2023-11-09T18:17:14 | 9 | 0 | ---
base_model: budecosystem/genz-13b-v2
language:
- en
library_name: transformers
license: llama2
model_name: GenZ 13B v2
pipeline_tag: text-generation
inference: false
model_creator: Bud
model_type: llama
prompt_template: '### User:
{prompt}
### Assistant:
'
quantized_by: TheBloke
---
<!-- header start -->
<!-- 200823 -->
<div style="width: auto; margin-left: auto; margin-right: auto">
<img src="https://i.imgur.com/EBdldam.jpg" alt="TheBlokeAI" style="width: 100%; min-width: 400px; display: block; margin: auto;">
</div>
<div style="display: flex; justify-content: space-between; width: 100%;">
<div style="display: flex; flex-direction: column; align-items: flex-start;">
<p style="margin-top: 0.5em; margin-bottom: 0em;"><a href="https://discord.gg/theblokeai">Chat & support: TheBloke's Discord server</a></p>
</div>
<div style="display: flex; flex-direction: column; align-items: flex-end;">
<p style="margin-top: 0.5em; margin-bottom: 0em;"><a href="https://www.patreon.com/TheBlokeAI">Want to contribute? TheBloke's Patreon page</a></p>
</div>
</div>
<div style="text-align:center; margin-top: 0em; margin-bottom: 0em"><p style="margin-top: 0.25em; margin-bottom: 0em;">TheBloke's LLM work is generously supported by a grant from <a href="https://a16z.com">andreessen horowitz (a16z)</a></p></div>
<hr style="margin-top: 1.0em; margin-bottom: 1.0em;">
<!-- header end -->
# GenZ 13B v2 - AWQ
- Model creator: [Bud](https://huggingface.co/budecosystem)
- Original model: [GenZ 13B v2](https://huggingface.co/budecosystem/genz-13b-v2)
<!-- description start -->
## Description
This repo contains AWQ model files for [Bud's GenZ 13B v2](https://huggingface.co/budecosystem/genz-13b-v2).
### About AWQ
AWQ is an efficient, accurate and blazing-fast low-bit weight quantization method, currently supporting 4-bit quantization. Compared to GPTQ, it offers faster Transformers-based inference.
It is also now supported by continuous batching server [vLLM](https://github.com/vllm-project/vllm), allowing use of Llama AWQ models for high-throughput concurrent inference in multi-user server scenarios.
As of September 25th 2023, preliminary Llama-only AWQ support has also been added to [Huggingface Text Generation Inference (TGI)](https://github.com/huggingface/text-generation-inference).
Note that, at the time of writing, overall throughput is still lower than running vLLM or TGI with unquantised models, however using AWQ enables using much smaller GPUs which can lead to easier deployment and overall cost savings. For example, a 70B model can be run on 1 x 48GB GPU instead of 2 x 80GB.
<!-- description end -->
<!-- repositories-available start -->
## Repositories available
* [AWQ model(s) for GPU inference.](https://huggingface.co/TheBloke/genz-13B-v2-AWQ)
* [GPTQ models for GPU inference, with multiple quantisation parameter options.](https://huggingface.co/TheBloke/genz-13B-v2-GPTQ)
* [2, 3, 4, 5, 6 and 8-bit GGUF models for CPU+GPU inference](https://huggingface.co/TheBloke/genz-13B-v2-GGUF)
* [Bud's original unquantised fp16 model in pytorch format, for GPU inference and for further conversions](https://huggingface.co/budecosystem/genz-13b-v2)
<!-- repositories-available end -->
<!-- prompt-template start -->
## Prompt template: User-Assistant-Newlines
```
### User:
{prompt}
### Assistant:
```
<!-- prompt-template end -->
<!-- README_AWQ.md-provided-files start -->
## Provided files, and AWQ parameters
For my first release of AWQ models, I am releasing 128g models only. I will consider adding 32g as well if there is interest, and once I have done perplexity and evaluation comparisons, but at this time 32g models are still not fully tested with AutoAWQ and vLLM.
Models are released as sharded safetensors files.
| Branch | Bits | GS | AWQ Dataset | Seq Len | Size |
| ------ | ---- | -- | ----------- | ------- | ---- |
| [main](https://huggingface.co/TheBloke/genz-13B-v2-AWQ/tree/main) | 4 | 128 | [wikitext](https://huggingface.co/datasets/wikitext/viewer/wikitext-2-v1/test) | 4096 | 7.25 GB
<!-- README_AWQ.md-provided-files end -->
<!-- README_AWQ.md-use-from-vllm start -->
## Serving this model from vLLM
Documentation on installing and using vLLM [can be found here](https://vllm.readthedocs.io/en/latest/).
Note: at the time of writing, vLLM has not yet done a new release with AWQ support.
If you try the vLLM examples below and get an error about `quantization` being unrecognised, or other AWQ-related issues, please install vLLM from Github source.
- When using vLLM as a server, pass the `--quantization awq` parameter, for example:
```shell
python3 python -m vllm.entrypoints.api_server --model TheBloke/genz-13B-v2-AWQ --quantization awq --dtype half
```
When using vLLM from Python code, pass the `quantization=awq` parameter, for example:
```python
from vllm import LLM, SamplingParams
prompts = [
"Hello, my name is",
"The president of the United States is",
"The capital of France is",
"The future of AI is",
]
sampling_params = SamplingParams(temperature=0.8, top_p=0.95)
llm = LLM(model="TheBloke/genz-13B-v2-AWQ", quantization="awq", dtype="half")
outputs = llm.generate(prompts, sampling_params)
# Print the outputs.
for output in outputs:
prompt = output.prompt
generated_text = output.outputs[0].text
print(f"Prompt: {prompt!r}, Generated text: {generated_text!r}")
```
<!-- README_AWQ.md-use-from-vllm start -->
<!-- README_AWQ.md-use-from-tgi start -->
## Serving this model from Text Generation Inference (TGI)
Use TGI version 1.1.0 or later. The official Docker container is: `ghcr.io/huggingface/text-generation-inference:1.1.0`
Example Docker parameters:
```shell
--model-id TheBloke/genz-13B-v2-AWQ --port 3000 --quantize awq --max-input-length 3696 --max-total-tokens 4096 --max-batch-prefill-tokens 4096
```
Example Python code for interfacing with TGI (requires huggingface-hub 0.17.0 or later):
```shell
pip3 install huggingface-hub
```
```python
from huggingface_hub import InferenceClient
endpoint_url = "https://your-endpoint-url-here"
prompt = "Tell me about AI"
prompt_template=f'''### User:
{prompt}
### Assistant:
'''
client = InferenceClient(endpoint_url)
response = client.text_generation(prompt,
max_new_tokens=128,
do_sample=True,
temperature=0.7,
top_p=0.95,
top_k=40,
repetition_penalty=1.1)
print(f"Model output: {response}")
```
<!-- README_AWQ.md-use-from-tgi end -->
<!-- README_AWQ.md-use-from-python start -->
## How to use this AWQ model from Python code
### Install the necessary packages
Requires: [AutoAWQ](https://github.com/casper-hansen/AutoAWQ) 0.1.1 or later
```shell
pip3 install autoawq
```
If you have problems installing [AutoAWQ](https://github.com/casper-hansen/AutoAWQ) using the pre-built wheels, install it from source instead:
```shell
pip3 uninstall -y autoawq
git clone https://github.com/casper-hansen/AutoAWQ
cd AutoAWQ
pip3 install .
```
### You can then try the following example code
```python
from awq import AutoAWQForCausalLM
from transformers import AutoTokenizer
model_name_or_path = "TheBloke/genz-13B-v2-AWQ"
# Load model
model = AutoAWQForCausalLM.from_quantized(model_name_or_path, fuse_layers=True,
trust_remote_code=False, safetensors=True)
tokenizer = AutoTokenizer.from_pretrained(model_name_or_path, trust_remote_code=False)
prompt = "Tell me about AI"
prompt_template=f'''### User:
{prompt}
### Assistant:
'''
print("\n\n*** Generate:")
tokens = tokenizer(
prompt_template,
return_tensors='pt'
).input_ids.cuda()
# Generate output
generation_output = model.generate(
tokens,
do_sample=True,
temperature=0.7,
top_p=0.95,
top_k=40,
max_new_tokens=512
)
print("Output: ", tokenizer.decode(generation_output[0]))
"""
# Inference should be possible with transformers pipeline as well in future
# But currently this is not yet supported by AutoAWQ (correct as of September 25th 2023)
from transformers import pipeline
print("*** Pipeline:")
pipe = pipeline(
"text-generation",
model=model,
tokenizer=tokenizer,
max_new_tokens=512,
do_sample=True,
temperature=0.7,
top_p=0.95,
top_k=40,
repetition_penalty=1.1
)
print(pipe(prompt_template)[0]['generated_text'])
"""
```
<!-- README_AWQ.md-use-from-python end -->
<!-- README_AWQ.md-compatibility start -->
## Compatibility
The files provided are tested to work with:
- [AutoAWQ](https://github.com/casper-hansen/AutoAWQ)
- [vLLM](https://github.com/vllm-project/vllm)
- [Huggingface Text Generation Inference (TGI)](https://github.com/huggingface/text-generation-inference)
TGI merged AWQ support on September 25th, 2023: [TGI PR #1054](https://github.com/huggingface/text-generation-inference/pull/1054). Use the `:latest` Docker container until the next TGI release is made.
<!-- README_AWQ.md-compatibility end -->
<!-- footer start -->
<!-- 200823 -->
## Discord
For further support, and discussions on these models and AI in general, join us at:
[TheBloke AI's Discord server](https://discord.gg/theblokeai)
## Thanks, and how to contribute
Thanks to the [chirper.ai](https://chirper.ai) team!
Thanks to Clay from [gpus.llm-utils.org](llm-utils)!
I've had a lot of people ask if they can contribute. I enjoy providing models and helping people, and would love to be able to spend even more time doing it, as well as expanding into new projects like fine tuning/training.
If you're able and willing to contribute it will be most gratefully received and will help me to keep providing more models, and to start work on new AI projects.
Donaters will get priority support on any and all AI/LLM/model questions and requests, access to a private Discord room, plus other benefits.
* Patreon: https://patreon.com/TheBlokeAI
* Ko-Fi: https://ko-fi.com/TheBlokeAI
**Special thanks to**: Aemon Algiz.
**Patreon special mentions**: Pierre Kircher, Stanislav Ovsiannikov, Michael Levine, Eugene Pentland, Andrey, 준교 김, Randy H, Fred von Graf, Artur Olbinski, Caitlyn Gatomon, terasurfer, Jeff Scroggin, James Bentley, Vadim, Gabriel Puliatti, Harry Royden McLaughlin, Sean Connelly, Dan Guido, Edmond Seymore, Alicia Loh, subjectnull, AzureBlack, Manuel Alberto Morcote, Thomas Belote, Lone Striker, Chris Smitley, Vitor Caleffi, Johann-Peter Hartmann, Clay Pascal, biorpg, Brandon Frisco, sidney chen, transmissions 11, Pedro Madruga, jinyuan sun, Ajan Kanaga, Emad Mostaque, Trenton Dambrowitz, Jonathan Leane, Iucharbius, usrbinkat, vamX, George Stoitzev, Luke Pendergrass, theTransient, Olakabola, Swaroop Kallakuri, Cap'n Zoog, Brandon Phillips, Michael Dempsey, Nikolai Manek, danny, Matthew Berman, Gabriel Tamborski, alfie_i, Raymond Fosdick, Tom X Nguyen, Raven Klaugh, LangChain4j, Magnesian, Illia Dulskyi, David Ziegler, Mano Prime, Luis Javier Navarrete Lozano, Erik Bjäreholt, 阿明, Nathan Dryer, Alex, Rainer Wilmers, zynix, TL, Joseph William Delisle, John Villwock, Nathan LeClaire, Willem Michiel, Joguhyik, GodLy, OG, Alps Aficionado, Jeffrey Morgan, ReadyPlayerEmma, Tiffany J. Kim, Sebastain Graf, Spencer Kim, Michael Davis, webtim, Talal Aujan, knownsqashed, John Detwiler, Imad Khwaja, Deo Leter, Jerry Meng, Elijah Stavena, Rooh Singh, Pieter, SuperWojo, Alexandros Triantafyllidis, Stephen Murray, Ai Maven, ya boyyy, Enrico Ros, Ken Nordquist, Deep Realms, Nicholas, Spiking Neurons AB, Elle, Will Dee, Jack West, RoA, Luke @flexchar, Viktor Bowallius, Derek Yates, Subspace Studios, jjj, Toran Billups, Asp the Wyvern, Fen Risland, Ilya, NimbleBox.ai, Chadd, Nitin Borwankar, Emre, Mandus, Leonard Tan, Kalila, K, Trailburnt, S_X, Cory Kujawski
Thank you to all my generous patrons and donaters!
And thank you again to a16z for their generous grant.
<!-- footer end -->
# Original model card: Bud's GenZ 13B v2
---
<div align="center"><h1 align="center">~ GenZ ~</h1><img src="https://raw.githubusercontent.com/BudEcosystem/GenZ/main/assets/genz-logo.png" width=150></div>
<p align="center"><i>Democratizing access to LLMs for the open-source community.<br>Let's advance AI, together. </i></p>
---
## Introduction 🎉
Welcome to **GenZ**, an advanced Large Language Model (LLM) fine-tuned on the foundation of Meta's open-source Llama V2 13B parameter model. At Bud Ecosystem, we believe in the power of open-source collaboration to drive the advancement of technology at an accelerated pace. Our vision is to democratize access to fine-tuned LLMs, and to that end, we will be releasing a series of models across different parameter counts (7B, 13B, and 70B) and quantizations (32-bit and 4-bit) for the open-source community to use, enhance, and build upon.
<p align="center"><img src="https://raw.githubusercontent.com/BudEcosystem/GenZ/main/assets/MTBench_CompareChart_28July2023.png" width="500"></p>
The smaller quantization version of our models makes them more accessible, enabling their use even on personal computers. This opens up a world of possibilities for developers, researchers, and enthusiasts to experiment with these models and contribute to the collective advancement of language model technology.
GenZ isn't just a powerful text generator—it's a sophisticated AI assistant, capable of understanding and responding to user prompts with high-quality responses. We've taken the robust capabilities of Llama V2 and fine-tuned them to offer a more user-focused experience. Whether you're seeking informative responses or engaging interactions, GenZ is designed to deliver.
And this isn't the end. It's just the beginning of a journey towards creating more advanced, more efficient, and more accessible language models. We invite you to join us on this exciting journey. 🚀
---
<h2>Milestone Releases ️🏁</h2>
**[27 July 2023]**
[_GenZ-13B V2 (ggml)_](https://huggingface.co/budecosystem/genz-13b-v2-ggml) : Announcing our GenZ-13B v2 with ggml. This variant of GenZ can run inferencing using only CPU and without the need of GPU. Download the model from [HuggingFace](https://huggingface.co/budecosystem/genz-13b-v2-ggml).
**[27 July 2023]**
[_GenZ-13B V2 (4-bit)_](https://huggingface.co/budecosystem/genz-13b-v2-4bit) : Announcing our GenZ-13B v2 with 4-bit quantisation. Enabling inferencing with much lesser GPU memory than the 32-bit variant. Download the model from [HuggingFace](https://huggingface.co/budecosystem/genz-13b-v2-4bit).
**[26 July 2023]**
[_GenZ-13B V2_](https://huggingface.co/budecosystem/genz-13b-v2) : We're excited to announce the release of our Genz 13B v2 model, a step forward with improved evaluation results compared to v1. Experience the advancements by downloading the model from [HuggingFace](https://huggingface.co/budecosystem/genz-13b-v2).
**[20 July 2023]**
[_GenZ-13B_](https://huggingface.co/budecosystem/genz-13b) : We marked an important milestone with the release of the Genz 13B model. The journey began here, and you can partake in it by downloading the model from [Hugging Face](https://huggingface.co/budecosystem/genz-13b).
---
<img src="https://raw.githubusercontent.com/BudEcosystem/GenZ/main/assets/screenshot_genz13bv2.png" width="100%">
| 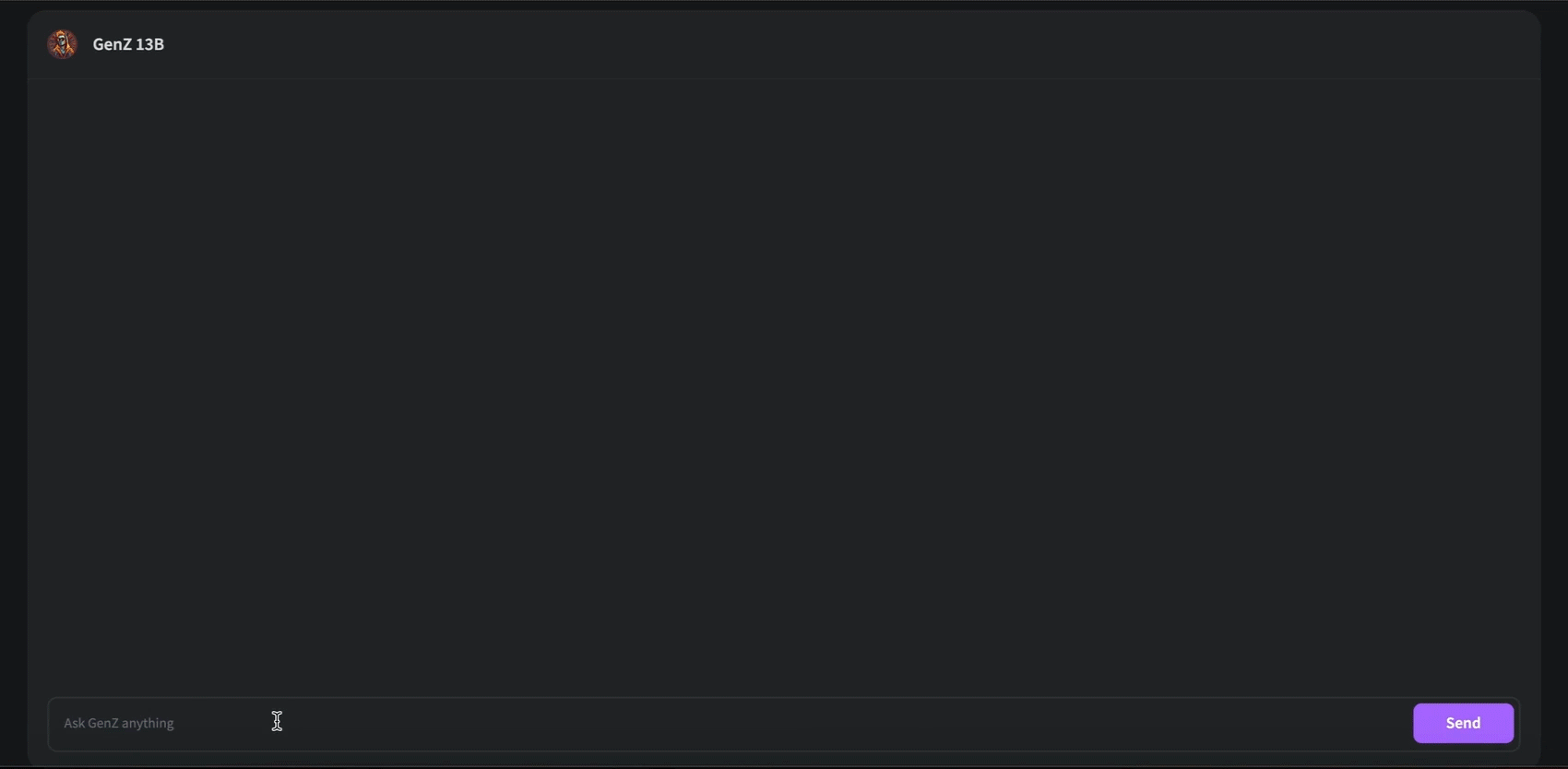 | 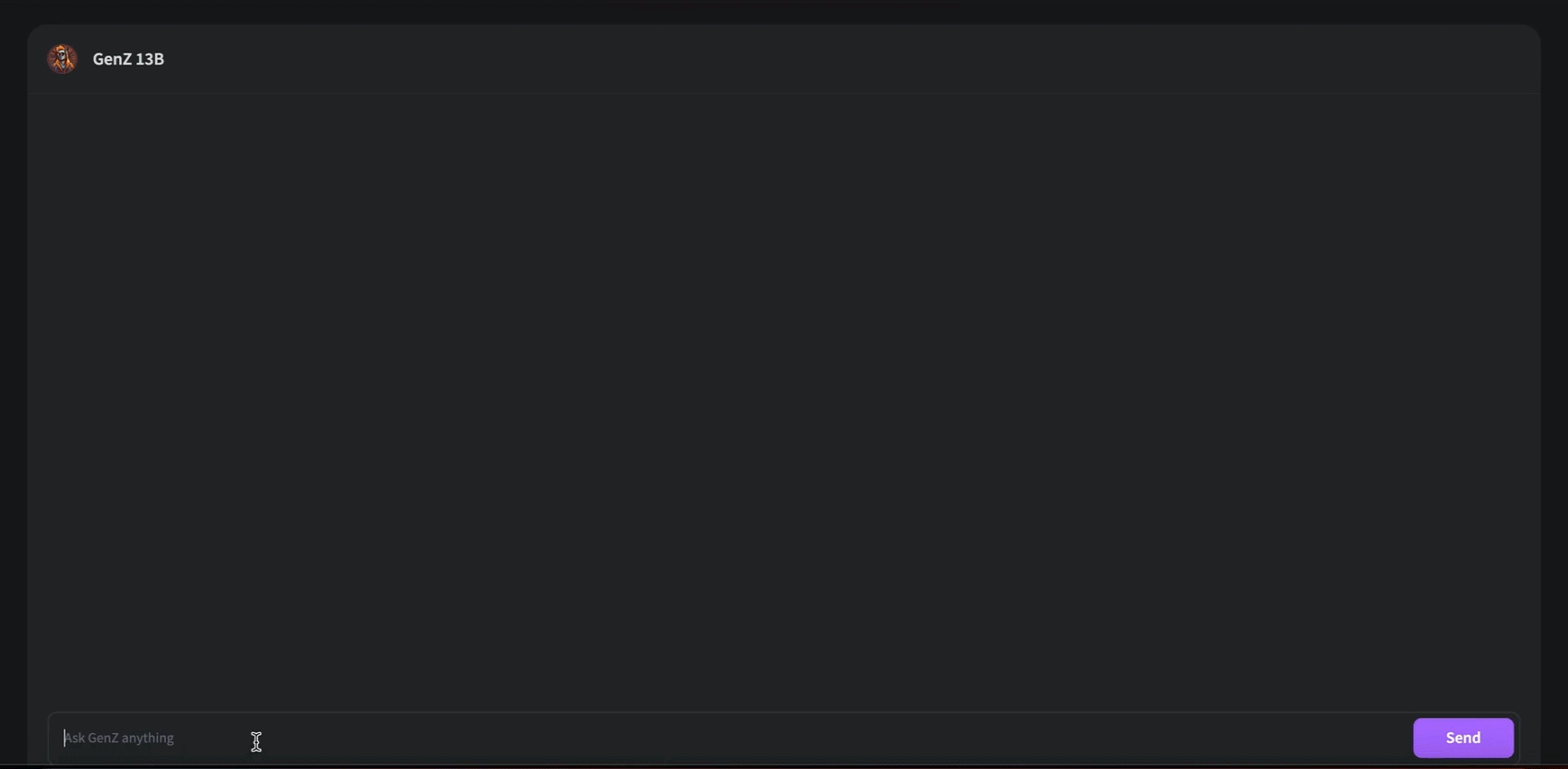 | 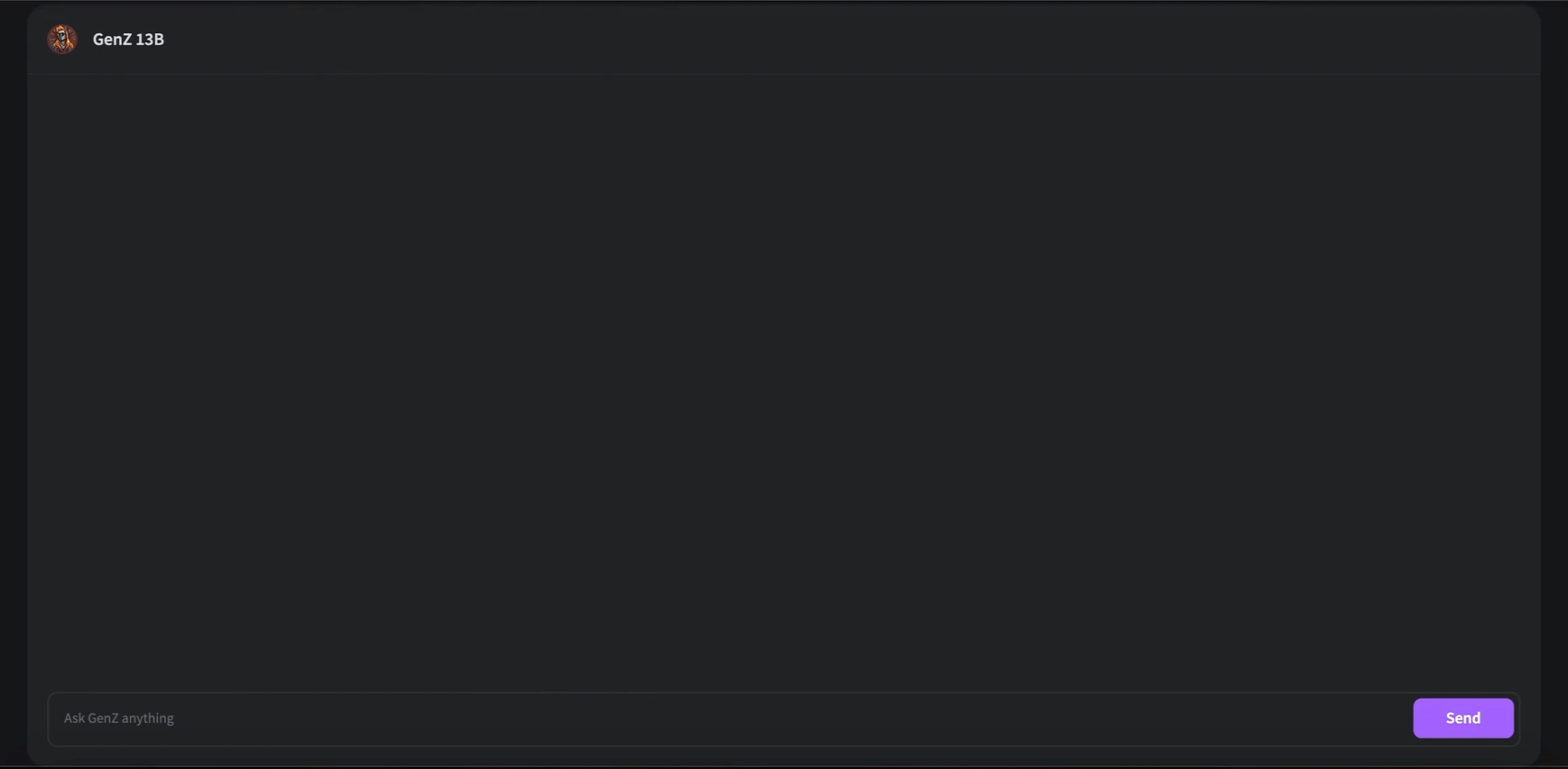
|:--:|:--:|:--:|
| *Code Generation* | *Poem Generation* | *Email Generation* |
<!--
<p align="center"><img src="https://raw.githubusercontent.com/adrot-dev/git-test/blob/main/assets/Python.gif" width="33%" alt="Python Code"><img src="https://raw.githubusercontent.com/adrot-dev/git-test/blob/main/assets/Poem.gif" width="33%"><img src="https://raw.githubusercontent.com/adrot-dev/git-test/blob/main/assets/Email.gif" width="33%"></p>
-->
<h2>Getting Started on Hugging Face 🤗</h2>
Getting up and running with our models on Hugging Face is a breeze. Follow these steps:
<h3>1️⃣ : Import necessary modules</h3>
Start by importing the necessary modules from the ‘transformers’ library and ‘torch’.
```python
import torch
from transformers import AutoTokenizer, AutoModelForCausalLM
```
<h3>2️⃣ : Load the tokenizer and the model</h3>
Next, load up the tokenizer and the model for ‘budecosystem/genz-13b-v2’ from Hugging Face using the ‘from_pretrained’ method.
```python
tokenizer = AutoTokenizer.from_pretrained("budecosystem/genz-13b-v2", trust_remote_code=True)
model = AutoModelForCausalLM.from_pretrained("budecosystem/genz-13b-v2", torch_dtype=torch.bfloat16)
```
<h3>3️⃣ : Generate responses</h3>
Now that you have the model and tokenizer, you're ready to generate responses. Here's how you can do it:
```python
inputs = tokenizer("The meaning of life is", return_tensors="pt")
sample = model.generate(**inputs, max_length=128)
print(tokenizer.decode(sample[0]))
```
In this example, "The meaning of life is" is the prompt template used for inference. You can replace it with any string you like.
Want to interact with the model in a more intuitive way? We have a Gradio interface set up for that. Head over to our GitHub page, clone the repository, and run the ‘generate.py’ script to try it out. Happy experimenting! 😄
<h2>Fine-tuning 🎯</h2>
It's time to upgrade the model by fine-tuning the model. You can do this using our provided finetune.py script. Here's an example command:
```bash
python finetune.py \
--model_name meta-llama/Llama-2-13b \
--data_path dataset.json \
--output_dir output \
--trust_remote_code \
--prompt_column instruction \
--response_column output \
--pad_token_id 50256
```
---
<h2 >Bonus: Colab Notebooks 📚 <b><i>(WIP)</i></b></h2>
Looking for an even simpler way to get started with GenZ? We've got you covered. We've prepared a pair of detailed Colab notebooks - one for Inference and one for Fine-tuning. These notebooks come pre-filled with all the information and code you'll need. All you'll have to do is run them!
Keep an eye out for these notebooks. They'll be added to the repository soon!
---
<h2>Why Use GenZ? 💡</h2>
You might be wondering, "Why should I choose GenZ over a pretrained model?" The answer lies in the extra mile we've gone to fine-tune our models.
While pretrained models are undeniably powerful, GenZ brings something extra to the table. We've fine-tuned it with curated datasets, which means it has additional skills and capabilities beyond what a pretrained model can offer. Whether you need it for a simple task or a complex project, GenZ is up for the challenge.
What's more, we are committed to continuously enhancing GenZ. We believe in the power of constant learning and improvement. That's why we'll be regularly fine-tuning our models with various curated datasets to make them even better. Our goal is to reach the state of the art and beyond - and we're committed to staying the course until we get there.
But don't just take our word for it. We've provided detailed evaluations and performance details in a later section, so you can see the difference for yourself.
Choose GenZ and join us on this journey. Together, we can push the boundaries of what's possible with large language models.
---
<h2>Model Card for GenZ 13B 📄</h2>
Here's a quick overview of everything you need to know about GenZ 13B.
<h3>Model Details:</h3>
- Developed by: Bud Ecosystem
- Base pretrained model type: Llama V2 13B
- Model Architecture: GenZ 13B, fine-tuned on Llama V2 13B, is an auto-regressive language model that employs an optimized transformer architecture. The fine-tuning process for GenZ 13B leveraged Supervised Fine-Tuning (SFT)
- License: The model is available for commercial use under a custom commercial license. For more information, please visit: [Meta AI Model and Library Downloads](https://ai.meta.com/resources/models-and-libraries/llama-downloads/)
---
<h2>Intended Use 💼</h2>
When we created GenZ 13B, we had a clear vision of how it could be used to push the boundaries of what's possible with large language models. We also understand the importance of using such models responsibly. Here's a brief overview of the intended and out-of-scope uses for GenZ 13B.
<h3>Direct Use</h3>
GenZ 13B is designed to be a powerful tool for research on large language models. It's also an excellent foundation for further specialization and fine-tuning for specific use cases, such as:
- Text summarization
- Text generation
- Chatbot creation
- And much more!
<h3>Out-of-Scope Use 🚩</h3>
While GenZ 13B is versatile, there are certain uses that are out of scope:
- Production use without adequate assessment of risks and mitigation
- Any use cases which may be considered irresponsible or harmful
- Use in any manner that violates applicable laws or regulations, including trade compliance laws
- Use in any other way that is prohibited by the Acceptable Use Policy and Licensing Agreement for Llama 2
Remember, GenZ 13B, like any large language model, is trained on a large-scale corpora representative of the web, and therefore, may carry the stereotypes and biases commonly encountered online.
<h3>Recommendations 🧠</h3>
We recommend users of GenZ 13B to consider fine-tuning it for the specific set of tasks of interest. Appropriate precautions and guardrails should be taken for any production use. Using GenZ 13B responsibly is key to unlocking its full potential while maintaining a safe and respectful environment.
---
<h2>Training Details 📚</h2>
When fine-tuning GenZ 13B, we took a meticulous approach to ensure we were building on the solid base of the pretrained Llama V2 13B model in the most effective way. Here's a look at the key details of our training process:
<h3>Fine-Tuning Training Data</h3>
For the fine-tuning process, we used a carefully curated mix of datasets. These included data from OpenAssistant, an instruction fine-tuning dataset, and Thought Source for the Chain Of Thought (CoT) approach. This diverse mix of data sources helped us enhance the model's capabilities across a range of tasks.
<h3>Fine-Tuning Procedure</h3>
We performed a full-parameter fine-tuning using Supervised Fine-Tuning (SFT). This was carried out on 4 A100 80GB GPUs, and the process took under 100 hours. To make the process more efficient, we used DeepSpeed's ZeRO-3 optimization.
<h3>Tokenizer</h3>
We used the SentencePiece tokenizer during the fine-tuning process. This tokenizer is known for its capability to handle open-vocabulary language tasks efficiently.
<h3>Hyperparameters</h3>
Here are the hyperparameters we used for fine-tuning:
| Hyperparameter | Value |
| -------------- | ----- |
| Warmup Ratio | 0.04 |
| Learning Rate Scheduler Type | Cosine |
| Learning Rate | 2e-5 |
| Number of Training Epochs | 3 |
| Per Device Training Batch Size | 4 |
| Gradient Accumulation Steps | 4 |
| Precision | FP16 |
| Optimizer | AdamW |
---
<h2>Evaluations 🎯</h2>
Evaluating our model is a key part of our fine-tuning process. It helps us understand how our model is performing and how it stacks up against other models. Here's a look at some of the key evaluations for GenZ 13B:
<h3>Benchmark Comparison</h3>
We've compared GenZ V1 with V2 to understand the improvements our fine-tuning has achieved.
| Model Name | MT Bench | Vicuna Bench | MMLU | Human Eval | Hellaswag | BBH |
|:----------:|:--------:|:------------:|:----:|:----------:|:---------:|:----:|
| Genz 13B | 6.12 | 86.1 | 53.62| 17.68 | 77.38 | 37.76|
| Genz 13B v2| 6.79 | 87.2 | 53.68| 21.95 | 77.48 | 38.1 |
<h3>MT Bench Score</h3>
A key evaluation metric we use is the MT Bench score. This score provides a comprehensive assessment of our model's performance across a range of tasks.
We're proud to say that our model performs at a level that's close to the Llama-70B-chat model on the MT Bench and top of the list among 13B models.
<p align="center"><img src="https://raw.githubusercontent.com/BudEcosystem/GenZ/main/assets/mt_bench_score.png" width="500"></p>
In the transition from GenZ V1 to V2, we noticed some fascinating performance shifts. While we saw a slight dip in coding performance, two other areas, Roleplay and Math, saw noticeable improvements.
---
<h2>Looking Ahead 👀</h2>
We're excited about the journey ahead with GenZ. We're committed to continuously improving and enhancing our models, and we're excited to see what the open-source community will build with them. We believe in the power of collaboration, and we can't wait to see what we can achieve together.
Remember, we're just getting started. This is just the beginning of a journey that we believe will revolutionize the world of large language models. We invite you to join us on this exciting journey. Together, we can push the boundaries of what's possible with AI. 🚀
---
Check the GitHub for the code -> [GenZ](https://raw.githubusercontent.com/BudEcosystem/GenZ)
| [
"SUMMARIZATION"
] | Non_BioNLP |
LaTarn/ac-clean-setfit-model | LaTarn | text-classification | [
"sentence-transformers",
"pytorch",
"safetensors",
"bert",
"setfit",
"text-classification",
"arxiv:2209.11055",
"license:apache-2.0",
"region:us"
] | 1,698,559,030,000 | 2023-11-06T05:40:21 | 48 | 0 | ---
license: apache-2.0
pipeline_tag: text-classification
tags:
- setfit
- sentence-transformers
- text-classification
---
# LaTarn/ac-clean-setfit-model
This is a [SetFit model](https://github.com/huggingface/setfit) that can be used for text classification. The model has been trained using an efficient few-shot learning technique that involves:
1. Fine-tuning a [Sentence Transformer](https://www.sbert.net) with contrastive learning.
2. Training a classification head with features from the fine-tuned Sentence Transformer.
## Usage
To use this model for inference, first install the SetFit library:
```bash
python -m pip install setfit
```
You can then run inference as follows:
```python
from setfit import SetFitModel
# Download from Hub and run inference
model = SetFitModel.from_pretrained("LaTarn/ac-clean-setfit-model")
# Run inference
preds = model(["i loved the spiderman movie!", "pineapple on pizza is the worst 🤮"])
```
## BibTeX entry and citation info
```bibtex
@article{https://doi.org/10.48550/arxiv.2209.11055,
doi = {10.48550/ARXIV.2209.11055},
url = {https://arxiv.org/abs/2209.11055},
author = {Tunstall, Lewis and Reimers, Nils and Jo, Unso Eun Seo and Bates, Luke and Korat, Daniel and Wasserblat, Moshe and Pereg, Oren},
keywords = {Computation and Language (cs.CL), FOS: Computer and information sciences, FOS: Computer and information sciences},
title = {Efficient Few-Shot Learning Without Prompts},
publisher = {arXiv},
year = {2022},
copyright = {Creative Commons Attribution 4.0 International}
}
```
| [
"TEXT_CLASSIFICATION"
] | Non_BioNLP |
Helsinki-NLP/opus-mt-ca-de | Helsinki-NLP | translation | [
"transformers",
"pytorch",
"tf",
"marian",
"text2text-generation",
"translation",
"ca",
"de",
"license:apache-2.0",
"autotrain_compatible",
"endpoints_compatible",
"region:us"
] | 1,646,263,744,000 | 2023-08-16T11:26:38 | 152 | 0 | ---
language:
- ca
- de
license: apache-2.0
tags:
- translation
---
### cat-deu
* source group: Catalan
* target group: German
* OPUS readme: [cat-deu](https://github.com/Helsinki-NLP/Tatoeba-Challenge/tree/master/models/cat-deu/README.md)
* model: transformer-align
* source language(s): cat
* target language(s): deu
* model: transformer-align
* pre-processing: normalization + SentencePiece (spm12k,spm12k)
* download original weights: [opus-2020-06-16.zip](https://object.pouta.csc.fi/Tatoeba-MT-models/cat-deu/opus-2020-06-16.zip)
* test set translations: [opus-2020-06-16.test.txt](https://object.pouta.csc.fi/Tatoeba-MT-models/cat-deu/opus-2020-06-16.test.txt)
* test set scores: [opus-2020-06-16.eval.txt](https://object.pouta.csc.fi/Tatoeba-MT-models/cat-deu/opus-2020-06-16.eval.txt)
## Benchmarks
| testset | BLEU | chr-F |
|-----------------------|-------|-------|
| Tatoeba-test.cat.deu | 39.5 | 0.593 |
### System Info:
- hf_name: cat-deu
- source_languages: cat
- target_languages: deu
- opus_readme_url: https://github.com/Helsinki-NLP/Tatoeba-Challenge/tree/master/models/cat-deu/README.md
- original_repo: Tatoeba-Challenge
- tags: ['translation']
- languages: ['ca', 'de']
- src_constituents: {'cat'}
- tgt_constituents: {'deu'}
- src_multilingual: False
- tgt_multilingual: False
- prepro: normalization + SentencePiece (spm12k,spm12k)
- url_model: https://object.pouta.csc.fi/Tatoeba-MT-models/cat-deu/opus-2020-06-16.zip
- url_test_set: https://object.pouta.csc.fi/Tatoeba-MT-models/cat-deu/opus-2020-06-16.test.txt
- src_alpha3: cat
- tgt_alpha3: deu
- short_pair: ca-de
- chrF2_score: 0.593
- bleu: 39.5
- brevity_penalty: 1.0
- ref_len: 5643.0
- src_name: Catalan
- tgt_name: German
- train_date: 2020-06-16
- src_alpha2: ca
- tgt_alpha2: de
- prefer_old: False
- long_pair: cat-deu
- helsinki_git_sha: 480fcbe0ee1bf4774bcbe6226ad9f58e63f6c535
- transformers_git_sha: 2207e5d8cb224e954a7cba69fa4ac2309e9ff30b
- port_machine: brutasse
- port_time: 2020-08-21-14:41 | [
"TRANSLATION"
] | Non_BioNLP |
Catchy1282/LogisticRegression_model_30_samples_per_label | Catchy1282 | text-classification | [
"setfit",
"safetensors",
"mpnet",
"sentence-transformers",
"text-classification",
"generated_from_setfit_trainer",
"arxiv:2209.11055",
"base_model:sentence-transformers/all-mpnet-base-v2",
"base_model:finetune:sentence-transformers/all-mpnet-base-v2",
"region:us"
] | 1,723,206,909,000 | 2024-08-09T12:35:27 | 7 | 0 | ---
base_model: sentence-transformers/all-mpnet-base-v2
library_name: setfit
metrics:
- accuracy
pipeline_tag: text-classification
tags:
- setfit
- sentence-transformers
- text-classification
- generated_from_setfit_trainer
widget: []
inference: true
---
# SetFit with sentence-transformers/all-mpnet-base-v2
This is a [SetFit](https://github.com/huggingface/setfit) model that can be used for Text Classification. This SetFit model uses [sentence-transformers/all-mpnet-base-v2](https://huggingface.co/sentence-transformers/all-mpnet-base-v2) as the Sentence Transformer embedding model. A [LogisticRegression](https://scikit-learn.org/stable/modules/generated/sklearn.linear_model.LogisticRegression.html) instance is used for classification.
The model has been trained using an efficient few-shot learning technique that involves:
1. Fine-tuning a [Sentence Transformer](https://www.sbert.net) with contrastive learning.
2. Training a classification head with features from the fine-tuned Sentence Transformer.
## Model Details
### Model Description
- **Model Type:** SetFit
- **Sentence Transformer body:** [sentence-transformers/all-mpnet-base-v2](https://huggingface.co/sentence-transformers/all-mpnet-base-v2)
- **Classification head:** a [LogisticRegression](https://scikit-learn.org/stable/modules/generated/sklearn.linear_model.LogisticRegression.html) instance
- **Maximum Sequence Length:** 384 tokens
<!-- - **Number of Classes:** Unknown -->
<!-- - **Training Dataset:** [Unknown](https://huggingface.co/datasets/unknown) -->
<!-- - **Language:** Unknown -->
<!-- - **License:** Unknown -->
### Model Sources
- **Repository:** [SetFit on GitHub](https://github.com/huggingface/setfit)
- **Paper:** [Efficient Few-Shot Learning Without Prompts](https://arxiv.org/abs/2209.11055)
- **Blogpost:** [SetFit: Efficient Few-Shot Learning Without Prompts](https://huggingface.co/blog/setfit)
## Uses
### Direct Use for Inference
First install the SetFit library:
```bash
pip install setfit
```
Then you can load this model and run inference.
```python
from setfit import SetFitModel
# Download from the 🤗 Hub
model = SetFitModel.from_pretrained("Catchy1282/LogisticRegression_model_30_samples_per_label")
# Run inference
preds = model("I loved the spiderman movie!")
```
<!--
### Downstream Use
*List how someone could finetune this model on their own dataset.*
-->
<!--
### Out-of-Scope Use
*List how the model may foreseeably be misused and address what users ought not to do with the model.*
-->
<!--
## Bias, Risks and Limitations
*What are the known or foreseeable issues stemming from this model? You could also flag here known failure cases or weaknesses of the model.*
-->
<!--
### Recommendations
*What are recommendations with respect to the foreseeable issues? For example, filtering explicit content.*
-->
## Training Details
### Framework Versions
- Python: 3.11.9
- SetFit: 1.0.3
- Sentence Transformers: 3.0.1
- Transformers: 4.39.0
- PyTorch: 2.3.0+cu121
- Datasets: 2.20.0
- Tokenizers: 0.15.2
## Citation
### BibTeX
```bibtex
@article{https://doi.org/10.48550/arxiv.2209.11055,
doi = {10.48550/ARXIV.2209.11055},
url = {https://arxiv.org/abs/2209.11055},
author = {Tunstall, Lewis and Reimers, Nils and Jo, Unso Eun Seo and Bates, Luke and Korat, Daniel and Wasserblat, Moshe and Pereg, Oren},
keywords = {Computation and Language (cs.CL), FOS: Computer and information sciences, FOS: Computer and information sciences},
title = {Efficient Few-Shot Learning Without Prompts},
publisher = {arXiv},
year = {2022},
copyright = {Creative Commons Attribution 4.0 International}
}
```
<!--
## Glossary
*Clearly define terms in order to be accessible across audiences.*
-->
<!--
## Model Card Authors
*Lists the people who create the model card, providing recognition and accountability for the detailed work that goes into its construction.*
-->
<!--
## Model Card Contact
*Provides a way for people who have updates to the Model Card, suggestions, or questions, to contact the Model Card authors.*
--> | [
"TEXT_CLASSIFICATION"
] | Non_BioNLP |
Fashion-Italia/gte-Qwen2-7B-instruct-Q4_K_M-GGUF | Fashion-Italia | sentence-similarity | [
"sentence-transformers",
"gguf",
"mteb",
"transformers",
"Qwen2",
"sentence-similarity",
"llama-cpp",
"gguf-my-repo",
"base_model:Alibaba-NLP/gte-Qwen2-7B-instruct",
"base_model:quantized:Alibaba-NLP/gte-Qwen2-7B-instruct",
"license:apache-2.0",
"model-index",
"autotrain_compatible",
"endpoints_compatible",
"region:us",
"conversational"
] | 1,734,605,813,000 | 2024-12-19T10:57:17 | 14 | 0 | ---
base_model: Alibaba-NLP/gte-Qwen2-7B-instruct
license: apache-2.0
tags:
- mteb
- sentence-transformers
- transformers
- Qwen2
- sentence-similarity
- llama-cpp
- gguf-my-repo
model-index:
- name: gte-qwen2-7B-instruct
results:
- task:
type: Classification
dataset:
name: MTEB AmazonCounterfactualClassification (en)
type: mteb/amazon_counterfactual
config: en
split: test
revision: e8379541af4e31359cca9fbcf4b00f2671dba205
metrics:
- type: accuracy
value: 91.31343283582089
- type: ap
value: 67.64251402604096
- type: f1
value: 87.53372530755692
- task:
type: Classification
dataset:
name: MTEB AmazonPolarityClassification
type: mteb/amazon_polarity
config: default
split: test
revision: e2d317d38cd51312af73b3d32a06d1a08b442046
metrics:
- type: accuracy
value: 97.497825
- type: ap
value: 96.30329547047529
- type: f1
value: 97.49769793778039
- task:
type: Classification
dataset:
name: MTEB AmazonReviewsClassification (en)
type: mteb/amazon_reviews_multi
config: en
split: test
revision: 1399c76144fd37290681b995c656ef9b2e06e26d
metrics:
- type: accuracy
value: 62.564
- type: f1
value: 60.975777935041066
- task:
type: Retrieval
dataset:
name: MTEB ArguAna
type: mteb/arguana
config: default
split: test
revision: c22ab2a51041ffd869aaddef7af8d8215647e41a
metrics:
- type: map_at_1
value: 36.486000000000004
- type: map_at_10
value: 54.842
- type: map_at_100
value: 55.206999999999994
- type: map_at_1000
value: 55.206999999999994
- type: map_at_3
value: 49.893
- type: map_at_5
value: 53.105000000000004
- type: mrr_at_1
value: 37.34
- type: mrr_at_10
value: 55.143
- type: mrr_at_100
value: 55.509
- type: mrr_at_1000
value: 55.509
- type: mrr_at_3
value: 50.212999999999994
- type: mrr_at_5
value: 53.432
- type: ndcg_at_1
value: 36.486000000000004
- type: ndcg_at_10
value: 64.273
- type: ndcg_at_100
value: 65.66199999999999
- type: ndcg_at_1000
value: 65.66199999999999
- type: ndcg_at_3
value: 54.352999999999994
- type: ndcg_at_5
value: 60.131
- type: precision_at_1
value: 36.486000000000004
- type: precision_at_10
value: 9.395000000000001
- type: precision_at_100
value: 0.996
- type: precision_at_1000
value: 0.1
- type: precision_at_3
value: 22.428
- type: precision_at_5
value: 16.259
- type: recall_at_1
value: 36.486000000000004
- type: recall_at_10
value: 93.95400000000001
- type: recall_at_100
value: 99.644
- type: recall_at_1000
value: 99.644
- type: recall_at_3
value: 67.283
- type: recall_at_5
value: 81.294
- task:
type: Clustering
dataset:
name: MTEB ArxivClusteringP2P
type: mteb/arxiv-clustering-p2p
config: default
split: test
revision: a122ad7f3f0291bf49cc6f4d32aa80929df69d5d
metrics:
- type: v_measure
value: 56.461169803700564
- task:
type: Clustering
dataset:
name: MTEB ArxivClusteringS2S
type: mteb/arxiv-clustering-s2s
config: default
split: test
revision: f910caf1a6075f7329cdf8c1a6135696f37dbd53
metrics:
- type: v_measure
value: 51.73600434466286
- task:
type: Reranking
dataset:
name: MTEB AskUbuntuDupQuestions
type: mteb/askubuntudupquestions-reranking
config: default
split: test
revision: 2000358ca161889fa9c082cb41daa8dcfb161a54
metrics:
- type: map
value: 67.57827065898053
- type: mrr
value: 79.08136569493911
- task:
type: STS
dataset:
name: MTEB BIOSSES
type: mteb/biosses-sts
config: default
split: test
revision: d3fb88f8f02e40887cd149695127462bbcf29b4a
metrics:
- type: cos_sim_pearson
value: 83.53324575999243
- type: cos_sim_spearman
value: 81.37173362822374
- type: euclidean_pearson
value: 82.19243335103444
- type: euclidean_spearman
value: 81.33679307304334
- type: manhattan_pearson
value: 82.38752665975699
- type: manhattan_spearman
value: 81.31510583189689
- task:
type: Classification
dataset:
name: MTEB Banking77Classification
type: mteb/banking77
config: default
split: test
revision: 0fd18e25b25c072e09e0d92ab615fda904d66300
metrics:
- type: accuracy
value: 87.56818181818181
- type: f1
value: 87.25826722019875
- task:
type: Clustering
dataset:
name: MTEB BiorxivClusteringP2P
type: mteb/biorxiv-clustering-p2p
config: default
split: test
revision: 65b79d1d13f80053f67aca9498d9402c2d9f1f40
metrics:
- type: v_measure
value: 50.09239610327673
- task:
type: Clustering
dataset:
name: MTEB BiorxivClusteringS2S
type: mteb/biorxiv-clustering-s2s
config: default
split: test
revision: 258694dd0231531bc1fd9de6ceb52a0853c6d908
metrics:
- type: v_measure
value: 46.64733054606282
- task:
type: Retrieval
dataset:
name: MTEB CQADupstackAndroidRetrieval
type: BeIR/cqadupstack
config: default
split: test
revision: f46a197baaae43b4f621051089b82a364682dfeb
metrics:
- type: map_at_1
value: 33.997
- type: map_at_10
value: 48.176
- type: map_at_100
value: 49.82
- type: map_at_1000
value: 49.924
- type: map_at_3
value: 43.626
- type: map_at_5
value: 46.275
- type: mrr_at_1
value: 42.059999999999995
- type: mrr_at_10
value: 53.726
- type: mrr_at_100
value: 54.398
- type: mrr_at_1000
value: 54.416
- type: mrr_at_3
value: 50.714999999999996
- type: mrr_at_5
value: 52.639
- type: ndcg_at_1
value: 42.059999999999995
- type: ndcg_at_10
value: 55.574999999999996
- type: ndcg_at_100
value: 60.744
- type: ndcg_at_1000
value: 61.85699999999999
- type: ndcg_at_3
value: 49.363
- type: ndcg_at_5
value: 52.44
- type: precision_at_1
value: 42.059999999999995
- type: precision_at_10
value: 11.101999999999999
- type: precision_at_100
value: 1.73
- type: precision_at_1000
value: 0.218
- type: precision_at_3
value: 24.464
- type: precision_at_5
value: 18.026
- type: recall_at_1
value: 33.997
- type: recall_at_10
value: 70.35900000000001
- type: recall_at_100
value: 91.642
- type: recall_at_1000
value: 97.977
- type: recall_at_3
value: 52.76
- type: recall_at_5
value: 61.148
- task:
type: Retrieval
dataset:
name: MTEB CQADupstackEnglishRetrieval
type: BeIR/cqadupstack
config: default
split: test
revision: ad9991cb51e31e31e430383c75ffb2885547b5f0
metrics:
- type: map_at_1
value: 35.884
- type: map_at_10
value: 48.14
- type: map_at_100
value: 49.5
- type: map_at_1000
value: 49.63
- type: map_at_3
value: 44.646
- type: map_at_5
value: 46.617999999999995
- type: mrr_at_1
value: 44.458999999999996
- type: mrr_at_10
value: 53.751000000000005
- type: mrr_at_100
value: 54.37800000000001
- type: mrr_at_1000
value: 54.415
- type: mrr_at_3
value: 51.815
- type: mrr_at_5
value: 52.882
- type: ndcg_at_1
value: 44.458999999999996
- type: ndcg_at_10
value: 54.157
- type: ndcg_at_100
value: 58.362
- type: ndcg_at_1000
value: 60.178
- type: ndcg_at_3
value: 49.661
- type: ndcg_at_5
value: 51.74999999999999
- type: precision_at_1
value: 44.458999999999996
- type: precision_at_10
value: 10.248
- type: precision_at_100
value: 1.5890000000000002
- type: precision_at_1000
value: 0.207
- type: precision_at_3
value: 23.928
- type: precision_at_5
value: 16.878999999999998
- type: recall_at_1
value: 35.884
- type: recall_at_10
value: 64.798
- type: recall_at_100
value: 82.345
- type: recall_at_1000
value: 93.267
- type: recall_at_3
value: 51.847
- type: recall_at_5
value: 57.601
- task:
type: Retrieval
dataset:
name: MTEB CQADupstackGamingRetrieval
type: BeIR/cqadupstack
config: default
split: test
revision: 4885aa143210c98657558c04aaf3dc47cfb54340
metrics:
- type: map_at_1
value: 39.383
- type: map_at_10
value: 53.714
- type: map_at_100
value: 54.838
- type: map_at_1000
value: 54.87800000000001
- type: map_at_3
value: 50.114999999999995
- type: map_at_5
value: 52.153000000000006
- type: mrr_at_1
value: 45.016
- type: mrr_at_10
value: 56.732000000000006
- type: mrr_at_100
value: 57.411
- type: mrr_at_1000
value: 57.431
- type: mrr_at_3
value: 54.044000000000004
- type: mrr_at_5
value: 55.639
- type: ndcg_at_1
value: 45.016
- type: ndcg_at_10
value: 60.228
- type: ndcg_at_100
value: 64.277
- type: ndcg_at_1000
value: 65.07
- type: ndcg_at_3
value: 54.124
- type: ndcg_at_5
value: 57.147000000000006
- type: precision_at_1
value: 45.016
- type: precision_at_10
value: 9.937
- type: precision_at_100
value: 1.288
- type: precision_at_1000
value: 0.13899999999999998
- type: precision_at_3
value: 24.471999999999998
- type: precision_at_5
value: 16.991
- type: recall_at_1
value: 39.383
- type: recall_at_10
value: 76.175
- type: recall_at_100
value: 93.02
- type: recall_at_1000
value: 98.60900000000001
- type: recall_at_3
value: 60.265
- type: recall_at_5
value: 67.46600000000001
- task:
type: Retrieval
dataset:
name: MTEB CQADupstackGisRetrieval
type: BeIR/cqadupstack
config: default
split: test
revision: 5003b3064772da1887988e05400cf3806fe491f2
metrics:
- type: map_at_1
value: 27.426000000000002
- type: map_at_10
value: 37.397000000000006
- type: map_at_100
value: 38.61
- type: map_at_1000
value: 38.678000000000004
- type: map_at_3
value: 34.150999999999996
- type: map_at_5
value: 36.137
- type: mrr_at_1
value: 29.944
- type: mrr_at_10
value: 39.654
- type: mrr_at_100
value: 40.638000000000005
- type: mrr_at_1000
value: 40.691
- type: mrr_at_3
value: 36.817
- type: mrr_at_5
value: 38.524
- type: ndcg_at_1
value: 29.944
- type: ndcg_at_10
value: 43.094
- type: ndcg_at_100
value: 48.789
- type: ndcg_at_1000
value: 50.339999999999996
- type: ndcg_at_3
value: 36.984
- type: ndcg_at_5
value: 40.248
- type: precision_at_1
value: 29.944
- type: precision_at_10
value: 6.78
- type: precision_at_100
value: 1.024
- type: precision_at_1000
value: 0.11800000000000001
- type: precision_at_3
value: 15.895000000000001
- type: precision_at_5
value: 11.39
- type: recall_at_1
value: 27.426000000000002
- type: recall_at_10
value: 58.464000000000006
- type: recall_at_100
value: 84.193
- type: recall_at_1000
value: 95.52000000000001
- type: recall_at_3
value: 42.172
- type: recall_at_5
value: 50.101
- task:
type: Retrieval
dataset:
name: MTEB CQADupstackMathematicaRetrieval
type: BeIR/cqadupstack
config: default
split: test
revision: 90fceea13679c63fe563ded68f3b6f06e50061de
metrics:
- type: map_at_1
value: 19.721
- type: map_at_10
value: 31.604
- type: map_at_100
value: 32.972
- type: map_at_1000
value: 33.077
- type: map_at_3
value: 27.218999999999998
- type: map_at_5
value: 29.53
- type: mrr_at_1
value: 25.0
- type: mrr_at_10
value: 35.843
- type: mrr_at_100
value: 36.785000000000004
- type: mrr_at_1000
value: 36.842000000000006
- type: mrr_at_3
value: 32.193
- type: mrr_at_5
value: 34.264
- type: ndcg_at_1
value: 25.0
- type: ndcg_at_10
value: 38.606
- type: ndcg_at_100
value: 44.272
- type: ndcg_at_1000
value: 46.527
- type: ndcg_at_3
value: 30.985000000000003
- type: ndcg_at_5
value: 34.43
- type: precision_at_1
value: 25.0
- type: precision_at_10
value: 7.811
- type: precision_at_100
value: 1.203
- type: precision_at_1000
value: 0.15
- type: precision_at_3
value: 15.423
- type: precision_at_5
value: 11.791
- type: recall_at_1
value: 19.721
- type: recall_at_10
value: 55.625
- type: recall_at_100
value: 79.34400000000001
- type: recall_at_1000
value: 95.208
- type: recall_at_3
value: 35.19
- type: recall_at_5
value: 43.626
- task:
type: Retrieval
dataset:
name: MTEB CQADupstackPhysicsRetrieval
type: BeIR/cqadupstack
config: default
split: test
revision: 79531abbd1fb92d06c6d6315a0cbbbf5bb247ea4
metrics:
- type: map_at_1
value: 33.784
- type: map_at_10
value: 47.522
- type: map_at_100
value: 48.949999999999996
- type: map_at_1000
value: 49.038
- type: map_at_3
value: 43.284
- type: map_at_5
value: 45.629
- type: mrr_at_1
value: 41.482
- type: mrr_at_10
value: 52.830999999999996
- type: mrr_at_100
value: 53.559999999999995
- type: mrr_at_1000
value: 53.588
- type: mrr_at_3
value: 50.016000000000005
- type: mrr_at_5
value: 51.614000000000004
- type: ndcg_at_1
value: 41.482
- type: ndcg_at_10
value: 54.569
- type: ndcg_at_100
value: 59.675999999999995
- type: ndcg_at_1000
value: 60.989000000000004
- type: ndcg_at_3
value: 48.187000000000005
- type: ndcg_at_5
value: 51.183
- type: precision_at_1
value: 41.482
- type: precision_at_10
value: 10.221
- type: precision_at_100
value: 1.486
- type: precision_at_1000
value: 0.17500000000000002
- type: precision_at_3
value: 23.548
- type: precision_at_5
value: 16.805
- type: recall_at_1
value: 33.784
- type: recall_at_10
value: 69.798
- type: recall_at_100
value: 90.098
- type: recall_at_1000
value: 98.176
- type: recall_at_3
value: 52.127
- type: recall_at_5
value: 59.861
- task:
type: Retrieval
dataset:
name: MTEB CQADupstackProgrammersRetrieval
type: BeIR/cqadupstack
config: default
split: test
revision: 6184bc1440d2dbc7612be22b50686b8826d22b32
metrics:
- type: map_at_1
value: 28.038999999999998
- type: map_at_10
value: 41.904
- type: map_at_100
value: 43.36
- type: map_at_1000
value: 43.453
- type: map_at_3
value: 37.785999999999994
- type: map_at_5
value: 40.105000000000004
- type: mrr_at_1
value: 35.046
- type: mrr_at_10
value: 46.926
- type: mrr_at_100
value: 47.815000000000005
- type: mrr_at_1000
value: 47.849000000000004
- type: mrr_at_3
value: 44.273
- type: mrr_at_5
value: 45.774
- type: ndcg_at_1
value: 35.046
- type: ndcg_at_10
value: 48.937000000000005
- type: ndcg_at_100
value: 54.544000000000004
- type: ndcg_at_1000
value: 56.069
- type: ndcg_at_3
value: 42.858000000000004
- type: ndcg_at_5
value: 45.644
- type: precision_at_1
value: 35.046
- type: precision_at_10
value: 9.452
- type: precision_at_100
value: 1.429
- type: precision_at_1000
value: 0.173
- type: precision_at_3
value: 21.346999999999998
- type: precision_at_5
value: 15.342
- type: recall_at_1
value: 28.038999999999998
- type: recall_at_10
value: 64.59700000000001
- type: recall_at_100
value: 87.735
- type: recall_at_1000
value: 97.41300000000001
- type: recall_at_3
value: 47.368
- type: recall_at_5
value: 54.93900000000001
- task:
type: Retrieval
dataset:
name: MTEB CQADupstackRetrieval
type: BeIR/cqadupstack
config: default
split: test
revision: 4ffe81d471b1924886b33c7567bfb200e9eec5c4
metrics:
- type: map_at_1
value: 28.17291666666667
- type: map_at_10
value: 40.025749999999995
- type: map_at_100
value: 41.39208333333333
- type: map_at_1000
value: 41.499249999999996
- type: map_at_3
value: 36.347
- type: map_at_5
value: 38.41391666666667
- type: mrr_at_1
value: 33.65925
- type: mrr_at_10
value: 44.085499999999996
- type: mrr_at_100
value: 44.94116666666667
- type: mrr_at_1000
value: 44.9855
- type: mrr_at_3
value: 41.2815
- type: mrr_at_5
value: 42.91491666666666
- type: ndcg_at_1
value: 33.65925
- type: ndcg_at_10
value: 46.430833333333325
- type: ndcg_at_100
value: 51.761
- type: ndcg_at_1000
value: 53.50899999999999
- type: ndcg_at_3
value: 40.45133333333333
- type: ndcg_at_5
value: 43.31483333333334
- type: precision_at_1
value: 33.65925
- type: precision_at_10
value: 8.4995
- type: precision_at_100
value: 1.3210000000000004
- type: precision_at_1000
value: 0.16591666666666666
- type: precision_at_3
value: 19.165083333333335
- type: precision_at_5
value: 13.81816666666667
- type: recall_at_1
value: 28.17291666666667
- type: recall_at_10
value: 61.12624999999999
- type: recall_at_100
value: 83.97266666666667
- type: recall_at_1000
value: 95.66550000000001
- type: recall_at_3
value: 44.661249999999995
- type: recall_at_5
value: 51.983333333333334
- type: map_at_1
value: 17.936
- type: map_at_10
value: 27.399
- type: map_at_100
value: 28.632
- type: map_at_1000
value: 28.738000000000003
- type: map_at_3
value: 24.456
- type: map_at_5
value: 26.06
- type: mrr_at_1
value: 19.224
- type: mrr_at_10
value: 28.998
- type: mrr_at_100
value: 30.11
- type: mrr_at_1000
value: 30.177
- type: mrr_at_3
value: 26.247999999999998
- type: mrr_at_5
value: 27.708
- type: ndcg_at_1
value: 19.224
- type: ndcg_at_10
value: 32.911
- type: ndcg_at_100
value: 38.873999999999995
- type: ndcg_at_1000
value: 41.277
- type: ndcg_at_3
value: 27.142
- type: ndcg_at_5
value: 29.755
- type: precision_at_1
value: 19.224
- type: precision_at_10
value: 5.6930000000000005
- type: precision_at_100
value: 0.9259999999999999
- type: precision_at_1000
value: 0.126
- type: precision_at_3
value: 12.138
- type: precision_at_5
value: 8.909
- type: recall_at_1
value: 17.936
- type: recall_at_10
value: 48.096
- type: recall_at_100
value: 75.389
- type: recall_at_1000
value: 92.803
- type: recall_at_3
value: 32.812999999999995
- type: recall_at_5
value: 38.851
- task:
type: Retrieval
dataset:
name: MTEB CQADupstackStatsRetrieval
type: BeIR/cqadupstack
config: default
split: test
revision: 65ac3a16b8e91f9cee4c9828cc7c335575432a2a
metrics:
- type: map_at_1
value: 24.681
- type: map_at_10
value: 34.892
- type: map_at_100
value: 35.996
- type: map_at_1000
value: 36.083
- type: map_at_3
value: 31.491999999999997
- type: map_at_5
value: 33.632
- type: mrr_at_1
value: 28.528
- type: mrr_at_10
value: 37.694
- type: mrr_at_100
value: 38.613
- type: mrr_at_1000
value: 38.668
- type: mrr_at_3
value: 34.714
- type: mrr_at_5
value: 36.616
- type: ndcg_at_1
value: 28.528
- type: ndcg_at_10
value: 40.703
- type: ndcg_at_100
value: 45.993
- type: ndcg_at_1000
value: 47.847
- type: ndcg_at_3
value: 34.622
- type: ndcg_at_5
value: 38.035999999999994
- type: precision_at_1
value: 28.528
- type: precision_at_10
value: 6.902
- type: precision_at_100
value: 1.0370000000000001
- type: precision_at_1000
value: 0.126
- type: precision_at_3
value: 15.798000000000002
- type: precision_at_5
value: 11.655999999999999
- type: recall_at_1
value: 24.681
- type: recall_at_10
value: 55.81
- type: recall_at_100
value: 79.785
- type: recall_at_1000
value: 92.959
- type: recall_at_3
value: 39.074
- type: recall_at_5
value: 47.568
- task:
type: Retrieval
dataset:
name: MTEB CQADupstackTexRetrieval
type: BeIR/cqadupstack
config: default
split: test
revision: 46989137a86843e03a6195de44b09deda022eec7
metrics:
- type: map_at_1
value: 18.627
- type: map_at_10
value: 27.872000000000003
- type: map_at_100
value: 29.237999999999996
- type: map_at_1000
value: 29.363
- type: map_at_3
value: 24.751
- type: map_at_5
value: 26.521
- type: mrr_at_1
value: 23.021
- type: mrr_at_10
value: 31.924000000000003
- type: mrr_at_100
value: 32.922000000000004
- type: mrr_at_1000
value: 32.988
- type: mrr_at_3
value: 29.192
- type: mrr_at_5
value: 30.798
- type: ndcg_at_1
value: 23.021
- type: ndcg_at_10
value: 33.535
- type: ndcg_at_100
value: 39.732
- type: ndcg_at_1000
value: 42.201
- type: ndcg_at_3
value: 28.153
- type: ndcg_at_5
value: 30.746000000000002
- type: precision_at_1
value: 23.021
- type: precision_at_10
value: 6.459
- type: precision_at_100
value: 1.1320000000000001
- type: precision_at_1000
value: 0.153
- type: precision_at_3
value: 13.719000000000001
- type: precision_at_5
value: 10.193000000000001
- type: recall_at_1
value: 18.627
- type: recall_at_10
value: 46.463
- type: recall_at_100
value: 74.226
- type: recall_at_1000
value: 91.28500000000001
- type: recall_at_3
value: 31.357000000000003
- type: recall_at_5
value: 38.067
- task:
type: Retrieval
dataset:
name: MTEB CQADupstackUnixRetrieval
type: BeIR/cqadupstack
config: default
split: test
revision: 6c6430d3a6d36f8d2a829195bc5dc94d7e063e53
metrics:
- type: map_at_1
value: 31.457
- type: map_at_10
value: 42.888
- type: map_at_100
value: 44.24
- type: map_at_1000
value: 44.327
- type: map_at_3
value: 39.588
- type: map_at_5
value: 41.423
- type: mrr_at_1
value: 37.126999999999995
- type: mrr_at_10
value: 47.083000000000006
- type: mrr_at_100
value: 47.997
- type: mrr_at_1000
value: 48.044
- type: mrr_at_3
value: 44.574000000000005
- type: mrr_at_5
value: 46.202
- type: ndcg_at_1
value: 37.126999999999995
- type: ndcg_at_10
value: 48.833
- type: ndcg_at_100
value: 54.327000000000005
- type: ndcg_at_1000
value: 56.011
- type: ndcg_at_3
value: 43.541999999999994
- type: ndcg_at_5
value: 46.127
- type: precision_at_1
value: 37.126999999999995
- type: precision_at_10
value: 8.376999999999999
- type: precision_at_100
value: 1.2309999999999999
- type: precision_at_1000
value: 0.146
- type: precision_at_3
value: 20.211000000000002
- type: precision_at_5
value: 14.16
- type: recall_at_1
value: 31.457
- type: recall_at_10
value: 62.369
- type: recall_at_100
value: 85.444
- type: recall_at_1000
value: 96.65599999999999
- type: recall_at_3
value: 47.961
- type: recall_at_5
value: 54.676
- task:
type: Retrieval
dataset:
name: MTEB CQADupstackWebmastersRetrieval
type: BeIR/cqadupstack
config: default
split: test
revision: 160c094312a0e1facb97e55eeddb698c0abe3571
metrics:
- type: map_at_1
value: 27.139999999999997
- type: map_at_10
value: 38.801
- type: map_at_100
value: 40.549
- type: map_at_1000
value: 40.802
- type: map_at_3
value: 35.05
- type: map_at_5
value: 36.884
- type: mrr_at_1
value: 33.004
- type: mrr_at_10
value: 43.864
- type: mrr_at_100
value: 44.667
- type: mrr_at_1000
value: 44.717
- type: mrr_at_3
value: 40.777
- type: mrr_at_5
value: 42.319
- type: ndcg_at_1
value: 33.004
- type: ndcg_at_10
value: 46.022
- type: ndcg_at_100
value: 51.542
- type: ndcg_at_1000
value: 53.742000000000004
- type: ndcg_at_3
value: 39.795
- type: ndcg_at_5
value: 42.272
- type: precision_at_1
value: 33.004
- type: precision_at_10
value: 9.012
- type: precision_at_100
value: 1.7770000000000001
- type: precision_at_1000
value: 0.26
- type: precision_at_3
value: 19.038
- type: precision_at_5
value: 13.675999999999998
- type: recall_at_1
value: 27.139999999999997
- type: recall_at_10
value: 60.961
- type: recall_at_100
value: 84.451
- type: recall_at_1000
value: 98.113
- type: recall_at_3
value: 43.001
- type: recall_at_5
value: 49.896
- task:
type: Retrieval
dataset:
name: MTEB ClimateFEVER
type: mteb/climate-fever
config: default
split: test
revision: 47f2ac6acb640fc46020b02a5b59fdda04d39380
metrics:
- type: map_at_1
value: 22.076999999999998
- type: map_at_10
value: 35.44
- type: map_at_100
value: 37.651
- type: map_at_1000
value: 37.824999999999996
- type: map_at_3
value: 30.764999999999997
- type: map_at_5
value: 33.26
- type: mrr_at_1
value: 50.163000000000004
- type: mrr_at_10
value: 61.207
- type: mrr_at_100
value: 61.675000000000004
- type: mrr_at_1000
value: 61.692
- type: mrr_at_3
value: 58.60999999999999
- type: mrr_at_5
value: 60.307
- type: ndcg_at_1
value: 50.163000000000004
- type: ndcg_at_10
value: 45.882
- type: ndcg_at_100
value: 53.239999999999995
- type: ndcg_at_1000
value: 55.852000000000004
- type: ndcg_at_3
value: 40.514
- type: ndcg_at_5
value: 42.038
- type: precision_at_1
value: 50.163000000000004
- type: precision_at_10
value: 13.466000000000001
- type: precision_at_100
value: 2.164
- type: precision_at_1000
value: 0.266
- type: precision_at_3
value: 29.707
- type: precision_at_5
value: 21.694
- type: recall_at_1
value: 22.076999999999998
- type: recall_at_10
value: 50.193
- type: recall_at_100
value: 74.993
- type: recall_at_1000
value: 89.131
- type: recall_at_3
value: 35.472
- type: recall_at_5
value: 41.814
- task:
type: Retrieval
dataset:
name: MTEB DBPedia
type: mteb/dbpedia
config: default
split: test
revision: c0f706b76e590d620bd6618b3ca8efdd34e2d659
metrics:
- type: map_at_1
value: 9.953
- type: map_at_10
value: 24.515
- type: map_at_100
value: 36.173
- type: map_at_1000
value: 38.351
- type: map_at_3
value: 16.592000000000002
- type: map_at_5
value: 20.036
- type: mrr_at_1
value: 74.25
- type: mrr_at_10
value: 81.813
- type: mrr_at_100
value: 82.006
- type: mrr_at_1000
value: 82.011
- type: mrr_at_3
value: 80.875
- type: mrr_at_5
value: 81.362
- type: ndcg_at_1
value: 62.5
- type: ndcg_at_10
value: 52.42
- type: ndcg_at_100
value: 56.808
- type: ndcg_at_1000
value: 63.532999999999994
- type: ndcg_at_3
value: 56.654
- type: ndcg_at_5
value: 54.18300000000001
- type: precision_at_1
value: 74.25
- type: precision_at_10
value: 42.699999999999996
- type: precision_at_100
value: 13.675
- type: precision_at_1000
value: 2.664
- type: precision_at_3
value: 60.5
- type: precision_at_5
value: 52.800000000000004
- type: recall_at_1
value: 9.953
- type: recall_at_10
value: 30.253999999999998
- type: recall_at_100
value: 62.516000000000005
- type: recall_at_1000
value: 84.163
- type: recall_at_3
value: 18.13
- type: recall_at_5
value: 22.771
- task:
type: Classification
dataset:
name: MTEB EmotionClassification
type: mteb/emotion
config: default
split: test
revision: 4f58c6b202a23cf9a4da393831edf4f9183cad37
metrics:
- type: accuracy
value: 79.455
- type: f1
value: 74.16798697647569
- task:
type: Retrieval
dataset:
name: MTEB FEVER
type: mteb/fever
config: default
split: test
revision: bea83ef9e8fb933d90a2f1d5515737465d613e12
metrics:
- type: map_at_1
value: 87.531
- type: map_at_10
value: 93.16799999999999
- type: map_at_100
value: 93.341
- type: map_at_1000
value: 93.349
- type: map_at_3
value: 92.444
- type: map_at_5
value: 92.865
- type: mrr_at_1
value: 94.014
- type: mrr_at_10
value: 96.761
- type: mrr_at_100
value: 96.762
- type: mrr_at_1000
value: 96.762
- type: mrr_at_3
value: 96.672
- type: mrr_at_5
value: 96.736
- type: ndcg_at_1
value: 94.014
- type: ndcg_at_10
value: 95.112
- type: ndcg_at_100
value: 95.578
- type: ndcg_at_1000
value: 95.68900000000001
- type: ndcg_at_3
value: 94.392
- type: ndcg_at_5
value: 94.72500000000001
- type: precision_at_1
value: 94.014
- type: precision_at_10
value: 11.065
- type: precision_at_100
value: 1.157
- type: precision_at_1000
value: 0.11800000000000001
- type: precision_at_3
value: 35.259
- type: precision_at_5
value: 21.599
- type: recall_at_1
value: 87.531
- type: recall_at_10
value: 97.356
- type: recall_at_100
value: 98.965
- type: recall_at_1000
value: 99.607
- type: recall_at_3
value: 95.312
- type: recall_at_5
value: 96.295
- task:
type: Retrieval
dataset:
name: MTEB FiQA2018
type: mteb/fiqa
config: default
split: test
revision: 27a168819829fe9bcd655c2df245fb19452e8e06
metrics:
- type: map_at_1
value: 32.055
- type: map_at_10
value: 53.114
- type: map_at_100
value: 55.235
- type: map_at_1000
value: 55.345
- type: map_at_3
value: 45.854
- type: map_at_5
value: 50.025
- type: mrr_at_1
value: 60.34
- type: mrr_at_10
value: 68.804
- type: mrr_at_100
value: 69.309
- type: mrr_at_1000
value: 69.32199999999999
- type: mrr_at_3
value: 66.40899999999999
- type: mrr_at_5
value: 67.976
- type: ndcg_at_1
value: 60.34
- type: ndcg_at_10
value: 62.031000000000006
- type: ndcg_at_100
value: 68.00500000000001
- type: ndcg_at_1000
value: 69.286
- type: ndcg_at_3
value: 56.355999999999995
- type: ndcg_at_5
value: 58.687
- type: precision_at_1
value: 60.34
- type: precision_at_10
value: 17.176
- type: precision_at_100
value: 2.36
- type: precision_at_1000
value: 0.259
- type: precision_at_3
value: 37.14
- type: precision_at_5
value: 27.809
- type: recall_at_1
value: 32.055
- type: recall_at_10
value: 70.91
- type: recall_at_100
value: 91.83
- type: recall_at_1000
value: 98.871
- type: recall_at_3
value: 51.202999999999996
- type: recall_at_5
value: 60.563
- task:
type: Retrieval
dataset:
name: MTEB HotpotQA
type: mteb/hotpotqa
config: default
split: test
revision: ab518f4d6fcca38d87c25209f94beba119d02014
metrics:
- type: map_at_1
value: 43.68
- type: map_at_10
value: 64.389
- type: map_at_100
value: 65.24
- type: map_at_1000
value: 65.303
- type: map_at_3
value: 61.309000000000005
- type: map_at_5
value: 63.275999999999996
- type: mrr_at_1
value: 87.36
- type: mrr_at_10
value: 91.12
- type: mrr_at_100
value: 91.227
- type: mrr_at_1000
value: 91.229
- type: mrr_at_3
value: 90.57600000000001
- type: mrr_at_5
value: 90.912
- type: ndcg_at_1
value: 87.36
- type: ndcg_at_10
value: 73.076
- type: ndcg_at_100
value: 75.895
- type: ndcg_at_1000
value: 77.049
- type: ndcg_at_3
value: 68.929
- type: ndcg_at_5
value: 71.28
- type: precision_at_1
value: 87.36
- type: precision_at_10
value: 14.741000000000001
- type: precision_at_100
value: 1.694
- type: precision_at_1000
value: 0.185
- type: precision_at_3
value: 43.043
- type: precision_at_5
value: 27.681
- type: recall_at_1
value: 43.68
- type: recall_at_10
value: 73.707
- type: recall_at_100
value: 84.7
- type: recall_at_1000
value: 92.309
- type: recall_at_3
value: 64.564
- type: recall_at_5
value: 69.203
- task:
type: Classification
dataset:
name: MTEB ImdbClassification
type: mteb/imdb
config: default
split: test
revision: 3d86128a09e091d6018b6d26cad27f2739fc2db7
metrics:
- type: accuracy
value: 96.75399999999999
- type: ap
value: 95.29389839242187
- type: f1
value: 96.75348377433475
- task:
type: Retrieval
dataset:
name: MTEB MSMARCO
type: mteb/msmarco
config: default
split: dev
revision: c5a29a104738b98a9e76336939199e264163d4a0
metrics:
- type: map_at_1
value: 25.176
- type: map_at_10
value: 38.598
- type: map_at_100
value: 39.707
- type: map_at_1000
value: 39.744
- type: map_at_3
value: 34.566
- type: map_at_5
value: 36.863
- type: mrr_at_1
value: 25.874000000000002
- type: mrr_at_10
value: 39.214
- type: mrr_at_100
value: 40.251
- type: mrr_at_1000
value: 40.281
- type: mrr_at_3
value: 35.291
- type: mrr_at_5
value: 37.545
- type: ndcg_at_1
value: 25.874000000000002
- type: ndcg_at_10
value: 45.98
- type: ndcg_at_100
value: 51.197
- type: ndcg_at_1000
value: 52.073
- type: ndcg_at_3
value: 37.785999999999994
- type: ndcg_at_5
value: 41.870000000000005
- type: precision_at_1
value: 25.874000000000002
- type: precision_at_10
value: 7.181
- type: precision_at_100
value: 0.979
- type: precision_at_1000
value: 0.106
- type: precision_at_3
value: 16.051000000000002
- type: precision_at_5
value: 11.713
- type: recall_at_1
value: 25.176
- type: recall_at_10
value: 68.67699999999999
- type: recall_at_100
value: 92.55
- type: recall_at_1000
value: 99.164
- type: recall_at_3
value: 46.372
- type: recall_at_5
value: 56.16
- task:
type: Classification
dataset:
name: MTEB MTOPDomainClassification (en)
type: mteb/mtop_domain
config: en
split: test
revision: d80d48c1eb48d3562165c59d59d0034df9fff0bf
metrics:
- type: accuracy
value: 99.03784769721841
- type: f1
value: 98.97791641821495
- task:
type: Classification
dataset:
name: MTEB MTOPIntentClassification (en)
type: mteb/mtop_intent
config: en
split: test
revision: ae001d0e6b1228650b7bd1c2c65fb50ad11a8aba
metrics:
- type: accuracy
value: 91.88326493388054
- type: f1
value: 73.74809928034335
- task:
type: Classification
dataset:
name: MTEB MassiveIntentClassification (en)
type: mteb/amazon_massive_intent
config: en
split: test
revision: 31efe3c427b0bae9c22cbb560b8f15491cc6bed7
metrics:
- type: accuracy
value: 85.41358439811701
- type: f1
value: 83.503679460639
- task:
type: Classification
dataset:
name: MTEB MassiveScenarioClassification (en)
type: mteb/amazon_massive_scenario
config: en
split: test
revision: 7d571f92784cd94a019292a1f45445077d0ef634
metrics:
- type: accuracy
value: 89.77135171486215
- type: f1
value: 88.89843747468366
- task:
type: Clustering
dataset:
name: MTEB MedrxivClusteringP2P
type: mteb/medrxiv-clustering-p2p
config: default
split: test
revision: e7a26af6f3ae46b30dde8737f02c07b1505bcc73
metrics:
- type: v_measure
value: 46.22695362087359
- task:
type: Clustering
dataset:
name: MTEB MedrxivClusteringS2S
type: mteb/medrxiv-clustering-s2s
config: default
split: test
revision: 35191c8c0dca72d8ff3efcd72aa802307d469663
metrics:
- type: v_measure
value: 44.132372165849425
- task:
type: Reranking
dataset:
name: MTEB MindSmallReranking
type: mteb/mind_small
config: default
split: test
revision: 3bdac13927fdc888b903db93b2ffdbd90b295a69
metrics:
- type: map
value: 33.35680810650402
- type: mrr
value: 34.72625715637218
- task:
type: Retrieval
dataset:
name: MTEB NFCorpus
type: mteb/nfcorpus
config: default
split: test
revision: ec0fa4fe99da2ff19ca1214b7966684033a58814
metrics:
- type: map_at_1
value: 7.165000000000001
- type: map_at_10
value: 15.424
- type: map_at_100
value: 20.28
- type: map_at_1000
value: 22.065
- type: map_at_3
value: 11.236
- type: map_at_5
value: 13.025999999999998
- type: mrr_at_1
value: 51.702999999999996
- type: mrr_at_10
value: 59.965
- type: mrr_at_100
value: 60.667
- type: mrr_at_1000
value: 60.702999999999996
- type: mrr_at_3
value: 58.772000000000006
- type: mrr_at_5
value: 59.267
- type: ndcg_at_1
value: 49.536
- type: ndcg_at_10
value: 40.6
- type: ndcg_at_100
value: 37.848
- type: ndcg_at_1000
value: 46.657
- type: ndcg_at_3
value: 46.117999999999995
- type: ndcg_at_5
value: 43.619
- type: precision_at_1
value: 51.393
- type: precision_at_10
value: 30.31
- type: precision_at_100
value: 9.972
- type: precision_at_1000
value: 2.329
- type: precision_at_3
value: 43.137
- type: precision_at_5
value: 37.585
- type: recall_at_1
value: 7.165000000000001
- type: recall_at_10
value: 19.689999999999998
- type: recall_at_100
value: 39.237
- type: recall_at_1000
value: 71.417
- type: recall_at_3
value: 12.247
- type: recall_at_5
value: 14.902999999999999
- task:
type: Retrieval
dataset:
name: MTEB NQ
type: mteb/nq
config: default
split: test
revision: b774495ed302d8c44a3a7ea25c90dbce03968f31
metrics:
- type: map_at_1
value: 42.653999999999996
- type: map_at_10
value: 59.611999999999995
- type: map_at_100
value: 60.32300000000001
- type: map_at_1000
value: 60.336
- type: map_at_3
value: 55.584999999999994
- type: map_at_5
value: 58.19
- type: mrr_at_1
value: 47.683
- type: mrr_at_10
value: 62.06700000000001
- type: mrr_at_100
value: 62.537
- type: mrr_at_1000
value: 62.544999999999995
- type: mrr_at_3
value: 59.178
- type: mrr_at_5
value: 61.034
- type: ndcg_at_1
value: 47.654
- type: ndcg_at_10
value: 67.001
- type: ndcg_at_100
value: 69.73899999999999
- type: ndcg_at_1000
value: 69.986
- type: ndcg_at_3
value: 59.95700000000001
- type: ndcg_at_5
value: 64.025
- type: precision_at_1
value: 47.654
- type: precision_at_10
value: 10.367999999999999
- type: precision_at_100
value: 1.192
- type: precision_at_1000
value: 0.121
- type: precision_at_3
value: 26.651000000000003
- type: precision_at_5
value: 18.459
- type: recall_at_1
value: 42.653999999999996
- type: recall_at_10
value: 86.619
- type: recall_at_100
value: 98.04899999999999
- type: recall_at_1000
value: 99.812
- type: recall_at_3
value: 68.987
- type: recall_at_5
value: 78.158
- task:
type: Retrieval
dataset:
name: MTEB QuoraRetrieval
type: mteb/quora
config: default
split: test
revision: None
metrics:
- type: map_at_1
value: 72.538
- type: map_at_10
value: 86.702
- type: map_at_100
value: 87.31
- type: map_at_1000
value: 87.323
- type: map_at_3
value: 83.87
- type: map_at_5
value: 85.682
- type: mrr_at_1
value: 83.31
- type: mrr_at_10
value: 89.225
- type: mrr_at_100
value: 89.30399999999999
- type: mrr_at_1000
value: 89.30399999999999
- type: mrr_at_3
value: 88.44300000000001
- type: mrr_at_5
value: 89.005
- type: ndcg_at_1
value: 83.32000000000001
- type: ndcg_at_10
value: 90.095
- type: ndcg_at_100
value: 91.12
- type: ndcg_at_1000
value: 91.179
- type: ndcg_at_3
value: 87.606
- type: ndcg_at_5
value: 89.031
- type: precision_at_1
value: 83.32000000000001
- type: precision_at_10
value: 13.641
- type: precision_at_100
value: 1.541
- type: precision_at_1000
value: 0.157
- type: precision_at_3
value: 38.377
- type: precision_at_5
value: 25.162000000000003
- type: recall_at_1
value: 72.538
- type: recall_at_10
value: 96.47200000000001
- type: recall_at_100
value: 99.785
- type: recall_at_1000
value: 99.99900000000001
- type: recall_at_3
value: 89.278
- type: recall_at_5
value: 93.367
- task:
type: Clustering
dataset:
name: MTEB RedditClustering
type: mteb/reddit-clustering
config: default
split: test
revision: 24640382cdbf8abc73003fb0fa6d111a705499eb
metrics:
- type: v_measure
value: 73.55219145406065
- task:
type: Clustering
dataset:
name: MTEB RedditClusteringP2P
type: mteb/reddit-clustering-p2p
config: default
split: test
revision: 282350215ef01743dc01b456c7f5241fa8937f16
metrics:
- type: v_measure
value: 74.13437105242755
- task:
type: Retrieval
dataset:
name: MTEB SCIDOCS
type: mteb/scidocs
config: default
split: test
revision: None
metrics:
- type: map_at_1
value: 6.873
- type: map_at_10
value: 17.944
- type: map_at_100
value: 21.171
- type: map_at_1000
value: 21.528
- type: map_at_3
value: 12.415
- type: map_at_5
value: 15.187999999999999
- type: mrr_at_1
value: 33.800000000000004
- type: mrr_at_10
value: 46.455
- type: mrr_at_100
value: 47.378
- type: mrr_at_1000
value: 47.394999999999996
- type: mrr_at_3
value: 42.367
- type: mrr_at_5
value: 44.972
- type: ndcg_at_1
value: 33.800000000000004
- type: ndcg_at_10
value: 28.907
- type: ndcg_at_100
value: 39.695
- type: ndcg_at_1000
value: 44.582
- type: ndcg_at_3
value: 26.949
- type: ndcg_at_5
value: 23.988
- type: precision_at_1
value: 33.800000000000004
- type: precision_at_10
value: 15.079999999999998
- type: precision_at_100
value: 3.056
- type: precision_at_1000
value: 0.42100000000000004
- type: precision_at_3
value: 25.167
- type: precision_at_5
value: 21.26
- type: recall_at_1
value: 6.873
- type: recall_at_10
value: 30.568
- type: recall_at_100
value: 62.062
- type: recall_at_1000
value: 85.37700000000001
- type: recall_at_3
value: 15.312999999999999
- type: recall_at_5
value: 21.575
- task:
type: STS
dataset:
name: MTEB SICK-R
type: mteb/sickr-sts
config: default
split: test
revision: a6ea5a8cab320b040a23452cc28066d9beae2cee
metrics:
- type: cos_sim_pearson
value: 82.37009118256057
- type: cos_sim_spearman
value: 79.27986395671529
- type: euclidean_pearson
value: 79.18037715442115
- type: euclidean_spearman
value: 79.28004791561621
- type: manhattan_pearson
value: 79.34062972800541
- type: manhattan_spearman
value: 79.43106695543402
- task:
type: STS
dataset:
name: MTEB STS12
type: mteb/sts12-sts
config: default
split: test
revision: a0d554a64d88156834ff5ae9920b964011b16384
metrics:
- type: cos_sim_pearson
value: 87.48474767383833
- type: cos_sim_spearman
value: 79.54505388752513
- type: euclidean_pearson
value: 83.43282704179565
- type: euclidean_spearman
value: 79.54579919925405
- type: manhattan_pearson
value: 83.77564492427952
- type: manhattan_spearman
value: 79.84558396989286
- task:
type: STS
dataset:
name: MTEB STS13
type: mteb/sts13-sts
config: default
split: test
revision: 7e90230a92c190f1bf69ae9002b8cea547a64cca
metrics:
- type: cos_sim_pearson
value: 88.803698035802
- type: cos_sim_spearman
value: 88.83451367754881
- type: euclidean_pearson
value: 88.28939285711628
- type: euclidean_spearman
value: 88.83528996073112
- type: manhattan_pearson
value: 88.28017412671795
- type: manhattan_spearman
value: 88.9228828016344
- task:
type: STS
dataset:
name: MTEB STS14
type: mteb/sts14-sts
config: default
split: test
revision: 6031580fec1f6af667f0bd2da0a551cf4f0b2375
metrics:
- type: cos_sim_pearson
value: 85.27469288153428
- type: cos_sim_spearman
value: 83.87477064876288
- type: euclidean_pearson
value: 84.2601737035379
- type: euclidean_spearman
value: 83.87431082479074
- type: manhattan_pearson
value: 84.3621547772745
- type: manhattan_spearman
value: 84.12094375000423
- task:
type: STS
dataset:
name: MTEB STS15
type: mteb/sts15-sts
config: default
split: test
revision: ae752c7c21bf194d8b67fd573edf7ae58183cbe3
metrics:
- type: cos_sim_pearson
value: 88.12749863201587
- type: cos_sim_spearman
value: 88.54287568368565
- type: euclidean_pearson
value: 87.90429700607999
- type: euclidean_spearman
value: 88.5437689576261
- type: manhattan_pearson
value: 88.19276653356833
- type: manhattan_spearman
value: 88.99995393814679
- task:
type: STS
dataset:
name: MTEB STS16
type: mteb/sts16-sts
config: default
split: test
revision: 4d8694f8f0e0100860b497b999b3dbed754a0513
metrics:
- type: cos_sim_pearson
value: 85.68398747560902
- type: cos_sim_spearman
value: 86.48815303460574
- type: euclidean_pearson
value: 85.52356631237954
- type: euclidean_spearman
value: 86.486391949551
- type: manhattan_pearson
value: 85.67267981761788
- type: manhattan_spearman
value: 86.7073696332485
- task:
type: STS
dataset:
name: MTEB STS17 (en-en)
type: mteb/sts17-crosslingual-sts
config: en-en
split: test
revision: af5e6fb845001ecf41f4c1e033ce921939a2a68d
metrics:
- type: cos_sim_pearson
value: 88.9057107443124
- type: cos_sim_spearman
value: 88.7312168757697
- type: euclidean_pearson
value: 88.72810439714794
- type: euclidean_spearman
value: 88.71976185854771
- type: manhattan_pearson
value: 88.50433745949111
- type: manhattan_spearman
value: 88.51726175544195
- task:
type: STS
dataset:
name: MTEB STS22 (en)
type: mteb/sts22-crosslingual-sts
config: en
split: test
revision: eea2b4fe26a775864c896887d910b76a8098ad3f
metrics:
- type: cos_sim_pearson
value: 67.59391795109886
- type: cos_sim_spearman
value: 66.87613008631367
- type: euclidean_pearson
value: 69.23198488262217
- type: euclidean_spearman
value: 66.85427723013692
- type: manhattan_pearson
value: 69.50730124841084
- type: manhattan_spearman
value: 67.10404669820792
- task:
type: STS
dataset:
name: MTEB STSBenchmark
type: mteb/stsbenchmark-sts
config: default
split: test
revision: b0fddb56ed78048fa8b90373c8a3cfc37b684831
metrics:
- type: cos_sim_pearson
value: 87.0820605344619
- type: cos_sim_spearman
value: 86.8518089863434
- type: euclidean_pearson
value: 86.31087134689284
- type: euclidean_spearman
value: 86.8518520517941
- type: manhattan_pearson
value: 86.47203796160612
- type: manhattan_spearman
value: 87.1080149734421
- task:
type: Reranking
dataset:
name: MTEB SciDocsRR
type: mteb/scidocs-reranking
config: default
split: test
revision: d3c5e1fc0b855ab6097bf1cda04dd73947d7caab
metrics:
- type: map
value: 89.09255369305481
- type: mrr
value: 97.10323445617563
- task:
type: Retrieval
dataset:
name: MTEB SciFact
type: mteb/scifact
config: default
split: test
revision: 0228b52cf27578f30900b9e5271d331663a030d7
metrics:
- type: map_at_1
value: 61.260999999999996
- type: map_at_10
value: 74.043
- type: map_at_100
value: 74.37700000000001
- type: map_at_1000
value: 74.384
- type: map_at_3
value: 71.222
- type: map_at_5
value: 72.875
- type: mrr_at_1
value: 64.333
- type: mrr_at_10
value: 74.984
- type: mrr_at_100
value: 75.247
- type: mrr_at_1000
value: 75.25500000000001
- type: mrr_at_3
value: 73.167
- type: mrr_at_5
value: 74.35000000000001
- type: ndcg_at_1
value: 64.333
- type: ndcg_at_10
value: 79.06
- type: ndcg_at_100
value: 80.416
- type: ndcg_at_1000
value: 80.55600000000001
- type: ndcg_at_3
value: 74.753
- type: ndcg_at_5
value: 76.97500000000001
- type: precision_at_1
value: 64.333
- type: precision_at_10
value: 10.567
- type: precision_at_100
value: 1.1199999999999999
- type: precision_at_1000
value: 0.11299999999999999
- type: precision_at_3
value: 29.889
- type: precision_at_5
value: 19.533
- type: recall_at_1
value: 61.260999999999996
- type: recall_at_10
value: 93.167
- type: recall_at_100
value: 99.0
- type: recall_at_1000
value: 100.0
- type: recall_at_3
value: 81.667
- type: recall_at_5
value: 87.394
- task:
type: PairClassification
dataset:
name: MTEB SprintDuplicateQuestions
type: mteb/sprintduplicatequestions-pairclassification
config: default
split: test
revision: d66bd1f72af766a5cc4b0ca5e00c162f89e8cc46
metrics:
- type: cos_sim_accuracy
value: 99.71980198019801
- type: cos_sim_ap
value: 92.81616007802704
- type: cos_sim_f1
value: 85.17548454688318
- type: cos_sim_precision
value: 89.43894389438944
- type: cos_sim_recall
value: 81.3
- type: dot_accuracy
value: 99.71980198019801
- type: dot_ap
value: 92.81398760591358
- type: dot_f1
value: 85.17548454688318
- type: dot_precision
value: 89.43894389438944
- type: dot_recall
value: 81.3
- type: euclidean_accuracy
value: 99.71980198019801
- type: euclidean_ap
value: 92.81560637245072
- type: euclidean_f1
value: 85.17548454688318
- type: euclidean_precision
value: 89.43894389438944
- type: euclidean_recall
value: 81.3
- type: manhattan_accuracy
value: 99.73069306930694
- type: manhattan_ap
value: 93.14005487480794
- type: manhattan_f1
value: 85.56263269639068
- type: manhattan_precision
value: 91.17647058823529
- type: manhattan_recall
value: 80.60000000000001
- type: max_accuracy
value: 99.73069306930694
- type: max_ap
value: 93.14005487480794
- type: max_f1
value: 85.56263269639068
- task:
type: Clustering
dataset:
name: MTEB StackExchangeClustering
type: mteb/stackexchange-clustering
config: default
split: test
revision: 6cbc1f7b2bc0622f2e39d2c77fa502909748c259
metrics:
- type: v_measure
value: 79.86443362395185
- task:
type: Clustering
dataset:
name: MTEB StackExchangeClusteringP2P
type: mteb/stackexchange-clustering-p2p
config: default
split: test
revision: 815ca46b2622cec33ccafc3735d572c266efdb44
metrics:
- type: v_measure
value: 49.40897096662564
- task:
type: Reranking
dataset:
name: MTEB StackOverflowDupQuestions
type: mteb/stackoverflowdupquestions-reranking
config: default
split: test
revision: e185fbe320c72810689fc5848eb6114e1ef5ec69
metrics:
- type: map
value: 55.66040806627947
- type: mrr
value: 56.58670475766064
- task:
type: Summarization
dataset:
name: MTEB SummEval
type: mteb/summeval
config: default
split: test
revision: cda12ad7615edc362dbf25a00fdd61d3b1eaf93c
metrics:
- type: cos_sim_pearson
value: 31.51015090598575
- type: cos_sim_spearman
value: 31.35016454939226
- type: dot_pearson
value: 31.5150068731
- type: dot_spearman
value: 31.34790869023487
- task:
type: Retrieval
dataset:
name: MTEB TRECCOVID
type: mteb/trec-covid
config: default
split: test
revision: None
metrics:
- type: map_at_1
value: 0.254
- type: map_at_10
value: 2.064
- type: map_at_100
value: 12.909
- type: map_at_1000
value: 31.761
- type: map_at_3
value: 0.738
- type: map_at_5
value: 1.155
- type: mrr_at_1
value: 96.0
- type: mrr_at_10
value: 98.0
- type: mrr_at_100
value: 98.0
- type: mrr_at_1000
value: 98.0
- type: mrr_at_3
value: 98.0
- type: mrr_at_5
value: 98.0
- type: ndcg_at_1
value: 93.0
- type: ndcg_at_10
value: 82.258
- type: ndcg_at_100
value: 64.34
- type: ndcg_at_1000
value: 57.912
- type: ndcg_at_3
value: 90.827
- type: ndcg_at_5
value: 86.79
- type: precision_at_1
value: 96.0
- type: precision_at_10
value: 84.8
- type: precision_at_100
value: 66.0
- type: precision_at_1000
value: 25.356
- type: precision_at_3
value: 94.667
- type: precision_at_5
value: 90.4
- type: recall_at_1
value: 0.254
- type: recall_at_10
value: 2.1950000000000003
- type: recall_at_100
value: 16.088
- type: recall_at_1000
value: 54.559000000000005
- type: recall_at_3
value: 0.75
- type: recall_at_5
value: 1.191
- task:
type: Retrieval
dataset:
name: MTEB Touche2020
type: mteb/touche2020
config: default
split: test
revision: a34f9a33db75fa0cbb21bb5cfc3dae8dc8bec93f
metrics:
- type: map_at_1
value: 2.976
- type: map_at_10
value: 11.389000000000001
- type: map_at_100
value: 18.429000000000002
- type: map_at_1000
value: 20.113
- type: map_at_3
value: 6.483
- type: map_at_5
value: 8.770999999999999
- type: mrr_at_1
value: 40.816
- type: mrr_at_10
value: 58.118
- type: mrr_at_100
value: 58.489999999999995
- type: mrr_at_1000
value: 58.489999999999995
- type: mrr_at_3
value: 53.061
- type: mrr_at_5
value: 57.041
- type: ndcg_at_1
value: 40.816
- type: ndcg_at_10
value: 30.567
- type: ndcg_at_100
value: 42.44
- type: ndcg_at_1000
value: 53.480000000000004
- type: ndcg_at_3
value: 36.016
- type: ndcg_at_5
value: 34.257
- type: precision_at_1
value: 42.857
- type: precision_at_10
value: 25.714
- type: precision_at_100
value: 8.429
- type: precision_at_1000
value: 1.5939999999999999
- type: precision_at_3
value: 36.735
- type: precision_at_5
value: 33.878
- type: recall_at_1
value: 2.976
- type: recall_at_10
value: 17.854999999999997
- type: recall_at_100
value: 51.833
- type: recall_at_1000
value: 86.223
- type: recall_at_3
value: 7.887
- type: recall_at_5
value: 12.026
- task:
type: Classification
dataset:
name: MTEB ToxicConversationsClassification
type: mteb/toxic_conversations_50k
config: default
split: test
revision: d7c0de2777da35d6aae2200a62c6e0e5af397c4c
metrics:
- type: accuracy
value: 85.1174
- type: ap
value: 30.169441069345748
- type: f1
value: 69.79254701873245
- task:
type: Classification
dataset:
name: MTEB TweetSentimentExtractionClassification
type: mteb/tweet_sentiment_extraction
config: default
split: test
revision: d604517c81ca91fe16a244d1248fc021f9ecee7a
metrics:
- type: accuracy
value: 72.58347481607245
- type: f1
value: 72.74877295564937
- task:
type: Clustering
dataset:
name: MTEB TwentyNewsgroupsClustering
type: mteb/twentynewsgroups-clustering
config: default
split: test
revision: 6125ec4e24fa026cec8a478383ee943acfbd5449
metrics:
- type: v_measure
value: 53.90586138221305
- task:
type: PairClassification
dataset:
name: MTEB TwitterSemEval2015
type: mteb/twittersemeval2015-pairclassification
config: default
split: test
revision: 70970daeab8776df92f5ea462b6173c0b46fd2d1
metrics:
- type: cos_sim_accuracy
value: 87.35769207844072
- type: cos_sim_ap
value: 77.9645072410354
- type: cos_sim_f1
value: 71.32352941176471
- type: cos_sim_precision
value: 66.5903890160183
- type: cos_sim_recall
value: 76.78100263852242
- type: dot_accuracy
value: 87.37557370209214
- type: dot_ap
value: 77.96250046429908
- type: dot_f1
value: 71.28932757557064
- type: dot_precision
value: 66.95249130938586
- type: dot_recall
value: 76.22691292875989
- type: euclidean_accuracy
value: 87.35173153722357
- type: euclidean_ap
value: 77.96520460741593
- type: euclidean_f1
value: 71.32470733210104
- type: euclidean_precision
value: 66.91329479768785
- type: euclidean_recall
value: 76.35883905013192
- type: manhattan_accuracy
value: 87.25636287774931
- type: manhattan_ap
value: 77.77752485611796
- type: manhattan_f1
value: 71.18148599269183
- type: manhattan_precision
value: 66.10859728506787
- type: manhattan_recall
value: 77.0976253298153
- type: max_accuracy
value: 87.37557370209214
- type: max_ap
value: 77.96520460741593
- type: max_f1
value: 71.32470733210104
- task:
type: PairClassification
dataset:
name: MTEB TwitterURLCorpus
type: mteb/twitterurlcorpus-pairclassification
config: default
split: test
revision: 8b6510b0b1fa4e4c4f879467980e9be563ec1cdf
metrics:
- type: cos_sim_accuracy
value: 89.38176737687739
- type: cos_sim_ap
value: 86.58811861657401
- type: cos_sim_f1
value: 79.09430644097604
- type: cos_sim_precision
value: 75.45085977911366
- type: cos_sim_recall
value: 83.10748383122882
- type: dot_accuracy
value: 89.38370784336554
- type: dot_ap
value: 86.58840606004333
- type: dot_f1
value: 79.10179860068133
- type: dot_precision
value: 75.44546153308643
- type: dot_recall
value: 83.13058207576223
- type: euclidean_accuracy
value: 89.38564830985369
- type: euclidean_ap
value: 86.58820721061164
- type: euclidean_f1
value: 79.09070942235888
- type: euclidean_precision
value: 75.38729937194697
- type: euclidean_recall
value: 83.17677856482906
- type: manhattan_accuracy
value: 89.40699344122326
- type: manhattan_ap
value: 86.60631843011362
- type: manhattan_f1
value: 79.14949970570925
- type: manhattan_precision
value: 75.78191039729502
- type: manhattan_recall
value: 82.83030489682784
- type: max_accuracy
value: 89.40699344122326
- type: max_ap
value: 86.60631843011362
- type: max_f1
value: 79.14949970570925
- task:
type: STS
dataset:
name: MTEB AFQMC
type: C-MTEB/AFQMC
config: default
split: validation
revision: b44c3b011063adb25877c13823db83bb193913c4
metrics:
- type: cos_sim_pearson
value: 65.58442135663871
- type: cos_sim_spearman
value: 72.2538631361313
- type: euclidean_pearson
value: 70.97255486607429
- type: euclidean_spearman
value: 72.25374250228647
- type: manhattan_pearson
value: 70.83250199989911
- type: manhattan_spearman
value: 72.14819496536272
- task:
type: STS
dataset:
name: MTEB ATEC
type: C-MTEB/ATEC
config: default
split: test
revision: 0f319b1142f28d00e055a6770f3f726ae9b7d865
metrics:
- type: cos_sim_pearson
value: 59.99478404929932
- type: cos_sim_spearman
value: 62.61836216999812
- type: euclidean_pearson
value: 66.86429811933593
- type: euclidean_spearman
value: 62.6183520374191
- type: manhattan_pearson
value: 66.8063778911633
- type: manhattan_spearman
value: 62.569607573241115
- task:
type: Classification
dataset:
name: MTEB AmazonReviewsClassification (zh)
type: mteb/amazon_reviews_multi
config: zh
split: test
revision: 1399c76144fd37290681b995c656ef9b2e06e26d
metrics:
- type: accuracy
value: 53.98400000000001
- type: f1
value: 51.21447361350723
- task:
type: STS
dataset:
name: MTEB BQ
type: C-MTEB/BQ
config: default
split: test
revision: e3dda5e115e487b39ec7e618c0c6a29137052a55
metrics:
- type: cos_sim_pearson
value: 79.11941660686553
- type: cos_sim_spearman
value: 81.25029594540435
- type: euclidean_pearson
value: 82.06973504238826
- type: euclidean_spearman
value: 81.2501989488524
- type: manhattan_pearson
value: 82.10094630392753
- type: manhattan_spearman
value: 81.27987244392389
- task:
type: Clustering
dataset:
name: MTEB CLSClusteringP2P
type: C-MTEB/CLSClusteringP2P
config: default
split: test
revision: 4b6227591c6c1a73bc76b1055f3b7f3588e72476
metrics:
- type: v_measure
value: 47.07270168705156
- task:
type: Clustering
dataset:
name: MTEB CLSClusteringS2S
type: C-MTEB/CLSClusteringS2S
config: default
split: test
revision: e458b3f5414b62b7f9f83499ac1f5497ae2e869f
metrics:
- type: v_measure
value: 45.98511703185043
- task:
type: Reranking
dataset:
name: MTEB CMedQAv1
type: C-MTEB/CMedQAv1-reranking
config: default
split: test
revision: 8d7f1e942507dac42dc58017c1a001c3717da7df
metrics:
- type: map
value: 88.19895157194931
- type: mrr
value: 90.21424603174603
- task:
type: Reranking
dataset:
name: MTEB CMedQAv2
type: C-MTEB/CMedQAv2-reranking
config: default
split: test
revision: 23d186750531a14a0357ca22cd92d712fd512ea0
metrics:
- type: map
value: 88.03317320980119
- type: mrr
value: 89.9461507936508
- task:
type: Retrieval
dataset:
name: MTEB CmedqaRetrieval
type: C-MTEB/CmedqaRetrieval
config: default
split: dev
revision: cd540c506dae1cf9e9a59c3e06f42030d54e7301
metrics:
- type: map_at_1
value: 29.037000000000003
- type: map_at_10
value: 42.001
- type: map_at_100
value: 43.773
- type: map_at_1000
value: 43.878
- type: map_at_3
value: 37.637
- type: map_at_5
value: 40.034
- type: mrr_at_1
value: 43.136
- type: mrr_at_10
value: 51.158
- type: mrr_at_100
value: 52.083
- type: mrr_at_1000
value: 52.12
- type: mrr_at_3
value: 48.733
- type: mrr_at_5
value: 50.025
- type: ndcg_at_1
value: 43.136
- type: ndcg_at_10
value: 48.685
- type: ndcg_at_100
value: 55.513
- type: ndcg_at_1000
value: 57.242000000000004
- type: ndcg_at_3
value: 43.329
- type: ndcg_at_5
value: 45.438
- type: precision_at_1
value: 43.136
- type: precision_at_10
value: 10.56
- type: precision_at_100
value: 1.6129999999999998
- type: precision_at_1000
value: 0.184
- type: precision_at_3
value: 24.064
- type: precision_at_5
value: 17.269000000000002
- type: recall_at_1
value: 29.037000000000003
- type: recall_at_10
value: 59.245000000000005
- type: recall_at_100
value: 87.355
- type: recall_at_1000
value: 98.74000000000001
- type: recall_at_3
value: 42.99
- type: recall_at_5
value: 49.681999999999995
- task:
type: PairClassification
dataset:
name: MTEB Cmnli
type: C-MTEB/CMNLI
config: default
split: validation
revision: 41bc36f332156f7adc9e38f53777c959b2ae9766
metrics:
- type: cos_sim_accuracy
value: 82.68190018039687
- type: cos_sim_ap
value: 90.18017125327886
- type: cos_sim_f1
value: 83.64080906868193
- type: cos_sim_precision
value: 79.7076890489303
- type: cos_sim_recall
value: 87.98223053542202
- type: dot_accuracy
value: 82.68190018039687
- type: dot_ap
value: 90.18782350103646
- type: dot_f1
value: 83.64242087729039
- type: dot_precision
value: 79.65313028764805
- type: dot_recall
value: 88.05237315875614
- type: euclidean_accuracy
value: 82.68190018039687
- type: euclidean_ap
value: 90.1801957900632
- type: euclidean_f1
value: 83.63636363636364
- type: euclidean_precision
value: 79.52772506852203
- type: euclidean_recall
value: 88.19265840542437
- type: manhattan_accuracy
value: 82.14070956103427
- type: manhattan_ap
value: 89.96178420101427
- type: manhattan_f1
value: 83.21087838578791
- type: manhattan_precision
value: 78.35605121850475
- type: manhattan_recall
value: 88.70703764320785
- type: max_accuracy
value: 82.68190018039687
- type: max_ap
value: 90.18782350103646
- type: max_f1
value: 83.64242087729039
- task:
type: Retrieval
dataset:
name: MTEB CovidRetrieval
type: C-MTEB/CovidRetrieval
config: default
split: dev
revision: 1271c7809071a13532e05f25fb53511ffce77117
metrics:
- type: map_at_1
value: 72.234
- type: map_at_10
value: 80.10000000000001
- type: map_at_100
value: 80.36
- type: map_at_1000
value: 80.363
- type: map_at_3
value: 78.315
- type: map_at_5
value: 79.607
- type: mrr_at_1
value: 72.392
- type: mrr_at_10
value: 80.117
- type: mrr_at_100
value: 80.36999999999999
- type: mrr_at_1000
value: 80.373
- type: mrr_at_3
value: 78.469
- type: mrr_at_5
value: 79.633
- type: ndcg_at_1
value: 72.392
- type: ndcg_at_10
value: 83.651
- type: ndcg_at_100
value: 84.749
- type: ndcg_at_1000
value: 84.83000000000001
- type: ndcg_at_3
value: 80.253
- type: ndcg_at_5
value: 82.485
- type: precision_at_1
value: 72.392
- type: precision_at_10
value: 9.557
- type: precision_at_100
value: 1.004
- type: precision_at_1000
value: 0.101
- type: precision_at_3
value: 28.732000000000003
- type: precision_at_5
value: 18.377
- type: recall_at_1
value: 72.234
- type: recall_at_10
value: 94.573
- type: recall_at_100
value: 99.368
- type: recall_at_1000
value: 100.0
- type: recall_at_3
value: 85.669
- type: recall_at_5
value: 91.01700000000001
- task:
type: Retrieval
dataset:
name: MTEB DuRetrieval
type: C-MTEB/DuRetrieval
config: default
split: dev
revision: a1a333e290fe30b10f3f56498e3a0d911a693ced
metrics:
- type: map_at_1
value: 26.173999999999996
- type: map_at_10
value: 80.04
- type: map_at_100
value: 82.94500000000001
- type: map_at_1000
value: 82.98100000000001
- type: map_at_3
value: 55.562999999999995
- type: map_at_5
value: 69.89800000000001
- type: mrr_at_1
value: 89.5
- type: mrr_at_10
value: 92.996
- type: mrr_at_100
value: 93.06400000000001
- type: mrr_at_1000
value: 93.065
- type: mrr_at_3
value: 92.658
- type: mrr_at_5
value: 92.84599999999999
- type: ndcg_at_1
value: 89.5
- type: ndcg_at_10
value: 87.443
- type: ndcg_at_100
value: 90.253
- type: ndcg_at_1000
value: 90.549
- type: ndcg_at_3
value: 85.874
- type: ndcg_at_5
value: 84.842
- type: precision_at_1
value: 89.5
- type: precision_at_10
value: 41.805
- type: precision_at_100
value: 4.827
- type: precision_at_1000
value: 0.49
- type: precision_at_3
value: 76.85
- type: precision_at_5
value: 64.8
- type: recall_at_1
value: 26.173999999999996
- type: recall_at_10
value: 89.101
- type: recall_at_100
value: 98.08099999999999
- type: recall_at_1000
value: 99.529
- type: recall_at_3
value: 57.902
- type: recall_at_5
value: 74.602
- task:
type: Retrieval
dataset:
name: MTEB EcomRetrieval
type: C-MTEB/EcomRetrieval
config: default
split: dev
revision: 687de13dc7294d6fd9be10c6945f9e8fec8166b9
metrics:
- type: map_at_1
value: 56.10000000000001
- type: map_at_10
value: 66.15299999999999
- type: map_at_100
value: 66.625
- type: map_at_1000
value: 66.636
- type: map_at_3
value: 63.632999999999996
- type: map_at_5
value: 65.293
- type: mrr_at_1
value: 56.10000000000001
- type: mrr_at_10
value: 66.15299999999999
- type: mrr_at_100
value: 66.625
- type: mrr_at_1000
value: 66.636
- type: mrr_at_3
value: 63.632999999999996
- type: mrr_at_5
value: 65.293
- type: ndcg_at_1
value: 56.10000000000001
- type: ndcg_at_10
value: 71.146
- type: ndcg_at_100
value: 73.27799999999999
- type: ndcg_at_1000
value: 73.529
- type: ndcg_at_3
value: 66.09
- type: ndcg_at_5
value: 69.08999999999999
- type: precision_at_1
value: 56.10000000000001
- type: precision_at_10
value: 8.68
- type: precision_at_100
value: 0.964
- type: precision_at_1000
value: 0.098
- type: precision_at_3
value: 24.4
- type: precision_at_5
value: 16.1
- type: recall_at_1
value: 56.10000000000001
- type: recall_at_10
value: 86.8
- type: recall_at_100
value: 96.39999999999999
- type: recall_at_1000
value: 98.3
- type: recall_at_3
value: 73.2
- type: recall_at_5
value: 80.5
- task:
type: Classification
dataset:
name: MTEB IFlyTek
type: C-MTEB/IFlyTek-classification
config: default
split: validation
revision: 421605374b29664c5fc098418fe20ada9bd55f8a
metrics:
- type: accuracy
value: 54.52096960369373
- type: f1
value: 40.930845295808695
- task:
type: Classification
dataset:
name: MTEB JDReview
type: C-MTEB/JDReview-classification
config: default
split: test
revision: b7c64bd89eb87f8ded463478346f76731f07bf8b
metrics:
- type: accuracy
value: 86.51031894934334
- type: ap
value: 55.9516014323483
- type: f1
value: 81.54813679326381
- task:
type: STS
dataset:
name: MTEB LCQMC
type: C-MTEB/LCQMC
config: default
split: test
revision: 17f9b096f80380fce5ed12a9be8be7784b337daf
metrics:
- type: cos_sim_pearson
value: 69.67437838574276
- type: cos_sim_spearman
value: 73.81314174653045
- type: euclidean_pearson
value: 72.63430276680275
- type: euclidean_spearman
value: 73.81358736777001
- type: manhattan_pearson
value: 72.58743833842829
- type: manhattan_spearman
value: 73.7590419009179
- task:
type: Reranking
dataset:
name: MTEB MMarcoReranking
type: C-MTEB/Mmarco-reranking
config: default
split: dev
revision: None
metrics:
- type: map
value: 31.648613483640254
- type: mrr
value: 30.37420634920635
- task:
type: Retrieval
dataset:
name: MTEB MMarcoRetrieval
type: C-MTEB/MMarcoRetrieval
config: default
split: dev
revision: 539bbde593d947e2a124ba72651aafc09eb33fc2
metrics:
- type: map_at_1
value: 73.28099999999999
- type: map_at_10
value: 81.977
- type: map_at_100
value: 82.222
- type: map_at_1000
value: 82.22699999999999
- type: map_at_3
value: 80.441
- type: map_at_5
value: 81.46600000000001
- type: mrr_at_1
value: 75.673
- type: mrr_at_10
value: 82.41000000000001
- type: mrr_at_100
value: 82.616
- type: mrr_at_1000
value: 82.621
- type: mrr_at_3
value: 81.094
- type: mrr_at_5
value: 81.962
- type: ndcg_at_1
value: 75.673
- type: ndcg_at_10
value: 85.15599999999999
- type: ndcg_at_100
value: 86.151
- type: ndcg_at_1000
value: 86.26899999999999
- type: ndcg_at_3
value: 82.304
- type: ndcg_at_5
value: 84.009
- type: precision_at_1
value: 75.673
- type: precision_at_10
value: 10.042
- type: precision_at_100
value: 1.052
- type: precision_at_1000
value: 0.106
- type: precision_at_3
value: 30.673000000000002
- type: precision_at_5
value: 19.326999999999998
- type: recall_at_1
value: 73.28099999999999
- type: recall_at_10
value: 94.446
- type: recall_at_100
value: 98.737
- type: recall_at_1000
value: 99.649
- type: recall_at_3
value: 86.984
- type: recall_at_5
value: 91.024
- task:
type: Classification
dataset:
name: MTEB MassiveIntentClassification (zh-CN)
type: mteb/amazon_massive_intent
config: zh-CN
split: test
revision: 31efe3c427b0bae9c22cbb560b8f15491cc6bed7
metrics:
- type: accuracy
value: 81.08607935440484
- type: f1
value: 78.24879986066307
- task:
type: Classification
dataset:
name: MTEB MassiveScenarioClassification (zh-CN)
type: mteb/amazon_massive_scenario
config: zh-CN
split: test
revision: 7d571f92784cd94a019292a1f45445077d0ef634
metrics:
- type: accuracy
value: 86.05917955615332
- type: f1
value: 85.05279279434997
- task:
type: Retrieval
dataset:
name: MTEB MedicalRetrieval
type: C-MTEB/MedicalRetrieval
config: default
split: dev
revision: 2039188fb5800a9803ba5048df7b76e6fb151fc6
metrics:
- type: map_at_1
value: 56.2
- type: map_at_10
value: 62.57899999999999
- type: map_at_100
value: 63.154999999999994
- type: map_at_1000
value: 63.193
- type: map_at_3
value: 61.217
- type: map_at_5
value: 62.012
- type: mrr_at_1
value: 56.3
- type: mrr_at_10
value: 62.629000000000005
- type: mrr_at_100
value: 63.205999999999996
- type: mrr_at_1000
value: 63.244
- type: mrr_at_3
value: 61.267
- type: mrr_at_5
value: 62.062
- type: ndcg_at_1
value: 56.2
- type: ndcg_at_10
value: 65.592
- type: ndcg_at_100
value: 68.657
- type: ndcg_at_1000
value: 69.671
- type: ndcg_at_3
value: 62.808
- type: ndcg_at_5
value: 64.24499999999999
- type: precision_at_1
value: 56.2
- type: precision_at_10
value: 7.5
- type: precision_at_100
value: 0.899
- type: precision_at_1000
value: 0.098
- type: precision_at_3
value: 22.467000000000002
- type: precision_at_5
value: 14.180000000000001
- type: recall_at_1
value: 56.2
- type: recall_at_10
value: 75.0
- type: recall_at_100
value: 89.9
- type: recall_at_1000
value: 97.89999999999999
- type: recall_at_3
value: 67.4
- type: recall_at_5
value: 70.89999999999999
- task:
type: Classification
dataset:
name: MTEB MultilingualSentiment
type: C-MTEB/MultilingualSentiment-classification
config: default
split: validation
revision: 46958b007a63fdbf239b7672c25d0bea67b5ea1a
metrics:
- type: accuracy
value: 76.87666666666667
- type: f1
value: 76.7317686219665
- task:
type: PairClassification
dataset:
name: MTEB Ocnli
type: C-MTEB/OCNLI
config: default
split: validation
revision: 66e76a618a34d6d565d5538088562851e6daa7ec
metrics:
- type: cos_sim_accuracy
value: 79.64266377910124
- type: cos_sim_ap
value: 84.78274442344829
- type: cos_sim_f1
value: 81.16947472745292
- type: cos_sim_precision
value: 76.47058823529412
- type: cos_sim_recall
value: 86.48363252375924
- type: dot_accuracy
value: 79.64266377910124
- type: dot_ap
value: 84.7851404063692
- type: dot_f1
value: 81.16947472745292
- type: dot_precision
value: 76.47058823529412
- type: dot_recall
value: 86.48363252375924
- type: euclidean_accuracy
value: 79.64266377910124
- type: euclidean_ap
value: 84.78068373762378
- type: euclidean_f1
value: 81.14794656110837
- type: euclidean_precision
value: 76.35009310986965
- type: euclidean_recall
value: 86.58922914466737
- type: manhattan_accuracy
value: 79.48023822414727
- type: manhattan_ap
value: 84.72928897427576
- type: manhattan_f1
value: 81.32084770823064
- type: manhattan_precision
value: 76.24768946395564
- type: manhattan_recall
value: 87.11721224920802
- type: max_accuracy
value: 79.64266377910124
- type: max_ap
value: 84.7851404063692
- type: max_f1
value: 81.32084770823064
- task:
type: Classification
dataset:
name: MTEB OnlineShopping
type: C-MTEB/OnlineShopping-classification
config: default
split: test
revision: e610f2ebd179a8fda30ae534c3878750a96db120
metrics:
- type: accuracy
value: 94.3
- type: ap
value: 92.8664032274438
- type: f1
value: 94.29311102997727
- task:
type: STS
dataset:
name: MTEB PAWSX
type: C-MTEB/PAWSX
config: default
split: test
revision: 9c6a90e430ac22b5779fb019a23e820b11a8b5e1
metrics:
- type: cos_sim_pearson
value: 48.51392279882909
- type: cos_sim_spearman
value: 54.06338895994974
- type: euclidean_pearson
value: 52.58480559573412
- type: euclidean_spearman
value: 54.06417276612201
- type: manhattan_pearson
value: 52.69525121721343
- type: manhattan_spearman
value: 54.048147455389675
- task:
type: STS
dataset:
name: MTEB QBQTC
type: C-MTEB/QBQTC
config: default
split: test
revision: 790b0510dc52b1553e8c49f3d2afb48c0e5c48b7
metrics:
- type: cos_sim_pearson
value: 29.728387290757325
- type: cos_sim_spearman
value: 31.366121633635284
- type: euclidean_pearson
value: 29.14588368552961
- type: euclidean_spearman
value: 31.36764411112844
- type: manhattan_pearson
value: 29.63517350523121
- type: manhattan_spearman
value: 31.94157020583762
- task:
type: STS
dataset:
name: MTEB STS22 (zh)
type: mteb/sts22-crosslingual-sts
config: zh
split: test
revision: eea2b4fe26a775864c896887d910b76a8098ad3f
metrics:
- type: cos_sim_pearson
value: 63.64868296271406
- type: cos_sim_spearman
value: 66.12800618164744
- type: euclidean_pearson
value: 63.21405767340238
- type: euclidean_spearman
value: 66.12786567790748
- type: manhattan_pearson
value: 64.04300276525848
- type: manhattan_spearman
value: 66.5066857145652
- task:
type: STS
dataset:
name: MTEB STSB
type: C-MTEB/STSB
config: default
split: test
revision: 0cde68302b3541bb8b3c340dc0644b0b745b3dc0
metrics:
- type: cos_sim_pearson
value: 81.2302623912794
- type: cos_sim_spearman
value: 81.16833673266562
- type: euclidean_pearson
value: 79.47647843876024
- type: euclidean_spearman
value: 81.16944349524972
- type: manhattan_pearson
value: 79.84947238492208
- type: manhattan_spearman
value: 81.64626599410026
- task:
type: Reranking
dataset:
name: MTEB T2Reranking
type: C-MTEB/T2Reranking
config: default
split: dev
revision: 76631901a18387f85eaa53e5450019b87ad58ef9
metrics:
- type: map
value: 67.80129586475687
- type: mrr
value: 77.77402311635554
- task:
type: Retrieval
dataset:
name: MTEB T2Retrieval
type: C-MTEB/T2Retrieval
config: default
split: dev
revision: 8731a845f1bf500a4f111cf1070785c793d10e64
metrics:
- type: map_at_1
value: 28.666999999999998
- type: map_at_10
value: 81.063
- type: map_at_100
value: 84.504
- type: map_at_1000
value: 84.552
- type: map_at_3
value: 56.897
- type: map_at_5
value: 70.073
- type: mrr_at_1
value: 92.087
- type: mrr_at_10
value: 94.132
- type: mrr_at_100
value: 94.19800000000001
- type: mrr_at_1000
value: 94.19999999999999
- type: mrr_at_3
value: 93.78999999999999
- type: mrr_at_5
value: 94.002
- type: ndcg_at_1
value: 92.087
- type: ndcg_at_10
value: 87.734
- type: ndcg_at_100
value: 90.736
- type: ndcg_at_1000
value: 91.184
- type: ndcg_at_3
value: 88.78
- type: ndcg_at_5
value: 87.676
- type: precision_at_1
value: 92.087
- type: precision_at_10
value: 43.46
- type: precision_at_100
value: 5.07
- type: precision_at_1000
value: 0.518
- type: precision_at_3
value: 77.49000000000001
- type: precision_at_5
value: 65.194
- type: recall_at_1
value: 28.666999999999998
- type: recall_at_10
value: 86.632
- type: recall_at_100
value: 96.646
- type: recall_at_1000
value: 98.917
- type: recall_at_3
value: 58.333999999999996
- type: recall_at_5
value: 72.974
- task:
type: Classification
dataset:
name: MTEB TNews
type: C-MTEB/TNews-classification
config: default
split: validation
revision: 317f262bf1e6126357bbe89e875451e4b0938fe4
metrics:
- type: accuracy
value: 52.971999999999994
- type: f1
value: 50.2898280984929
- task:
type: Clustering
dataset:
name: MTEB ThuNewsClusteringP2P
type: C-MTEB/ThuNewsClusteringP2P
config: default
split: test
revision: 5798586b105c0434e4f0fe5e767abe619442cf93
metrics:
- type: v_measure
value: 86.0797948663824
- task:
type: Clustering
dataset:
name: MTEB ThuNewsClusteringS2S
type: C-MTEB/ThuNewsClusteringS2S
config: default
split: test
revision: 8a8b2caeda43f39e13c4bc5bea0f8a667896e10d
metrics:
- type: v_measure
value: 85.10759092255017
- task:
type: Retrieval
dataset:
name: MTEB VideoRetrieval
type: C-MTEB/VideoRetrieval
config: default
split: dev
revision: 58c2597a5943a2ba48f4668c3b90d796283c5639
metrics:
- type: map_at_1
value: 65.60000000000001
- type: map_at_10
value: 74.773
- type: map_at_100
value: 75.128
- type: map_at_1000
value: 75.136
- type: map_at_3
value: 73.05
- type: map_at_5
value: 74.13499999999999
- type: mrr_at_1
value: 65.60000000000001
- type: mrr_at_10
value: 74.773
- type: mrr_at_100
value: 75.128
- type: mrr_at_1000
value: 75.136
- type: mrr_at_3
value: 73.05
- type: mrr_at_5
value: 74.13499999999999
- type: ndcg_at_1
value: 65.60000000000001
- type: ndcg_at_10
value: 78.84299999999999
- type: ndcg_at_100
value: 80.40899999999999
- type: ndcg_at_1000
value: 80.57
- type: ndcg_at_3
value: 75.40599999999999
- type: ndcg_at_5
value: 77.351
- type: precision_at_1
value: 65.60000000000001
- type: precision_at_10
value: 9.139999999999999
- type: precision_at_100
value: 0.984
- type: precision_at_1000
value: 0.1
- type: precision_at_3
value: 27.400000000000002
- type: precision_at_5
value: 17.380000000000003
- type: recall_at_1
value: 65.60000000000001
- type: recall_at_10
value: 91.4
- type: recall_at_100
value: 98.4
- type: recall_at_1000
value: 99.6
- type: recall_at_3
value: 82.19999999999999
- type: recall_at_5
value: 86.9
- task:
type: Classification
dataset:
name: MTEB Waimai
type: C-MTEB/waimai-classification
config: default
split: test
revision: 339287def212450dcaa9df8c22bf93e9980c7023
metrics:
- type: accuracy
value: 89.47
- type: ap
value: 75.59561751845389
- type: f1
value: 87.95207751382563
- task:
type: Clustering
dataset:
name: MTEB AlloProfClusteringP2P
type: lyon-nlp/alloprof
config: default
split: test
revision: 392ba3f5bcc8c51f578786c1fc3dae648662cb9b
metrics:
- type: v_measure
value: 76.05592323841036
- type: v_measure
value: 64.51718058866508
- task:
type: Reranking
dataset:
name: MTEB AlloprofReranking
type: lyon-nlp/mteb-fr-reranking-alloprof-s2p
config: default
split: test
revision: 666fdacebe0291776e86f29345663dfaf80a0db9
metrics:
- type: map
value: 73.08278490943373
- type: mrr
value: 74.66561454570449
- task:
type: Retrieval
dataset:
name: MTEB AlloprofRetrieval
type: lyon-nlp/alloprof
config: default
split: test
revision: 392ba3f5bcc8c51f578786c1fc3dae648662cb9b
metrics:
- type: map_at_1
value: 38.912
- type: map_at_10
value: 52.437999999999995
- type: map_at_100
value: 53.38
- type: map_at_1000
value: 53.427
- type: map_at_3
value: 48.879
- type: map_at_5
value: 50.934000000000005
- type: mrr_at_1
value: 44.085
- type: mrr_at_10
value: 55.337
- type: mrr_at_100
value: 56.016999999999996
- type: mrr_at_1000
value: 56.043
- type: mrr_at_3
value: 52.55499999999999
- type: mrr_at_5
value: 54.20399999999999
- type: ndcg_at_1
value: 44.085
- type: ndcg_at_10
value: 58.876
- type: ndcg_at_100
value: 62.714000000000006
- type: ndcg_at_1000
value: 63.721000000000004
- type: ndcg_at_3
value: 52.444
- type: ndcg_at_5
value: 55.692
- type: precision_at_1
value: 44.085
- type: precision_at_10
value: 9.21
- type: precision_at_100
value: 1.164
- type: precision_at_1000
value: 0.128
- type: precision_at_3
value: 23.043
- type: precision_at_5
value: 15.898000000000001
- type: recall_at_1
value: 38.912
- type: recall_at_10
value: 75.577
- type: recall_at_100
value: 92.038
- type: recall_at_1000
value: 99.325
- type: recall_at_3
value: 58.592
- type: recall_at_5
value: 66.235
- task:
type: Classification
dataset:
name: MTEB AmazonReviewsClassification (fr)
type: mteb/amazon_reviews_multi
config: fr
split: test
revision: 1399c76144fd37290681b995c656ef9b2e06e26d
metrics:
- type: accuracy
value: 55.532000000000004
- type: f1
value: 52.5783943471605
- task:
type: Retrieval
dataset:
name: MTEB BSARDRetrieval
type: maastrichtlawtech/bsard
config: default
split: test
revision: 5effa1b9b5fa3b0f9e12523e6e43e5f86a6e6d59
metrics:
- type: map_at_1
value: 8.108
- type: map_at_10
value: 14.710999999999999
- type: map_at_100
value: 15.891
- type: map_at_1000
value: 15.983
- type: map_at_3
value: 12.237
- type: map_at_5
value: 13.679
- type: mrr_at_1
value: 8.108
- type: mrr_at_10
value: 14.710999999999999
- type: mrr_at_100
value: 15.891
- type: mrr_at_1000
value: 15.983
- type: mrr_at_3
value: 12.237
- type: mrr_at_5
value: 13.679
- type: ndcg_at_1
value: 8.108
- type: ndcg_at_10
value: 18.796
- type: ndcg_at_100
value: 25.098
- type: ndcg_at_1000
value: 27.951999999999998
- type: ndcg_at_3
value: 13.712
- type: ndcg_at_5
value: 16.309
- type: precision_at_1
value: 8.108
- type: precision_at_10
value: 3.198
- type: precision_at_100
value: 0.626
- type: precision_at_1000
value: 0.086
- type: precision_at_3
value: 6.006
- type: precision_at_5
value: 4.865
- type: recall_at_1
value: 8.108
- type: recall_at_10
value: 31.982
- type: recall_at_100
value: 62.613
- type: recall_at_1000
value: 86.036
- type: recall_at_3
value: 18.018
- type: recall_at_5
value: 24.324
- task:
type: Clustering
dataset:
name: MTEB HALClusteringS2S
type: lyon-nlp/clustering-hal-s2s
config: default
split: test
revision: e06ebbbb123f8144bef1a5d18796f3dec9ae2915
metrics:
- type: v_measure
value: 30.833269778867116
- task:
type: Clustering
dataset:
name: MTEB MLSUMClusteringP2P
type: mlsum
config: default
split: test
revision: b5d54f8f3b61ae17845046286940f03c6bc79bc7
metrics:
- type: v_measure
value: 50.0281928004713
- type: v_measure
value: 43.699961510636534
- task:
type: Classification
dataset:
name: MTEB MTOPDomainClassification (fr)
type: mteb/mtop_domain
config: fr
split: test
revision: d80d48c1eb48d3562165c59d59d0034df9fff0bf
metrics:
- type: accuracy
value: 96.68963357344191
- type: f1
value: 96.45175170820961
- task:
type: Classification
dataset:
name: MTEB MTOPIntentClassification (fr)
type: mteb/mtop_intent
config: fr
split: test
revision: ae001d0e6b1228650b7bd1c2c65fb50ad11a8aba
metrics:
- type: accuracy
value: 87.46946445349202
- type: f1
value: 65.79860440988624
- task:
type: Classification
dataset:
name: MTEB MasakhaNEWSClassification (fra)
type: masakhane/masakhanews
config: fra
split: test
revision: 8ccc72e69e65f40c70e117d8b3c08306bb788b60
metrics:
- type: accuracy
value: 82.60663507109005
- type: f1
value: 77.20462646604777
- task:
type: Clustering
dataset:
name: MTEB MasakhaNEWSClusteringP2P (fra)
type: masakhane/masakhanews
config: fra
split: test
revision: 8ccc72e69e65f40c70e117d8b3c08306bb788b60
metrics:
- type: v_measure
value: 60.19311264967803
- type: v_measure
value: 63.6235764409785
- task:
type: Classification
dataset:
name: MTEB MassiveIntentClassification (fr)
type: mteb/amazon_massive_intent
config: fr
split: test
revision: 31efe3c427b0bae9c22cbb560b8f15491cc6bed7
metrics:
- type: accuracy
value: 81.65097511768661
- type: f1
value: 78.77796091490924
- task:
type: Classification
dataset:
name: MTEB MassiveScenarioClassification (fr)
type: mteb/amazon_massive_scenario
config: fr
split: test
revision: 7d571f92784cd94a019292a1f45445077d0ef634
metrics:
- type: accuracy
value: 86.64425016812373
- type: f1
value: 85.4912728670017
- task:
type: Retrieval
dataset:
name: MTEB MintakaRetrieval (fr)
type: jinaai/mintakaqa
config: fr
split: test
revision: efa78cc2f74bbcd21eff2261f9e13aebe40b814e
metrics:
- type: map_at_1
value: 35.913000000000004
- type: map_at_10
value: 48.147
- type: map_at_100
value: 48.91
- type: map_at_1000
value: 48.949
- type: map_at_3
value: 45.269999999999996
- type: map_at_5
value: 47.115
- type: mrr_at_1
value: 35.913000000000004
- type: mrr_at_10
value: 48.147
- type: mrr_at_100
value: 48.91
- type: mrr_at_1000
value: 48.949
- type: mrr_at_3
value: 45.269999999999996
- type: mrr_at_5
value: 47.115
- type: ndcg_at_1
value: 35.913000000000004
- type: ndcg_at_10
value: 54.03
- type: ndcg_at_100
value: 57.839
- type: ndcg_at_1000
value: 58.925000000000004
- type: ndcg_at_3
value: 48.217999999999996
- type: ndcg_at_5
value: 51.56699999999999
- type: precision_at_1
value: 35.913000000000004
- type: precision_at_10
value: 7.244000000000001
- type: precision_at_100
value: 0.9039999999999999
- type: precision_at_1000
value: 0.099
- type: precision_at_3
value: 18.905
- type: precision_at_5
value: 12.981000000000002
- type: recall_at_1
value: 35.913000000000004
- type: recall_at_10
value: 72.441
- type: recall_at_100
value: 90.41799999999999
- type: recall_at_1000
value: 99.099
- type: recall_at_3
value: 56.716
- type: recall_at_5
value: 64.90599999999999
- task:
type: PairClassification
dataset:
name: MTEB OpusparcusPC (fr)
type: GEM/opusparcus
config: fr
split: test
revision: 9e9b1f8ef51616073f47f306f7f47dd91663f86a
metrics:
- type: cos_sim_accuracy
value: 99.90069513406156
- type: cos_sim_ap
value: 100.0
- type: cos_sim_f1
value: 99.95032290114257
- type: cos_sim_precision
value: 100.0
- type: cos_sim_recall
value: 99.90069513406156
- type: dot_accuracy
value: 99.90069513406156
- type: dot_ap
value: 100.0
- type: dot_f1
value: 99.95032290114257
- type: dot_precision
value: 100.0
- type: dot_recall
value: 99.90069513406156
- type: euclidean_accuracy
value: 99.90069513406156
- type: euclidean_ap
value: 100.0
- type: euclidean_f1
value: 99.95032290114257
- type: euclidean_precision
value: 100.0
- type: euclidean_recall
value: 99.90069513406156
- type: manhattan_accuracy
value: 99.90069513406156
- type: manhattan_ap
value: 100.0
- type: manhattan_f1
value: 99.95032290114257
- type: manhattan_precision
value: 100.0
- type: manhattan_recall
value: 99.90069513406156
- type: max_accuracy
value: 99.90069513406156
- type: max_ap
value: 100.0
- type: max_f1
value: 99.95032290114257
- task:
type: PairClassification
dataset:
name: MTEB PawsX (fr)
type: paws-x
config: fr
split: test
revision: 8a04d940a42cd40658986fdd8e3da561533a3646
metrics:
- type: cos_sim_accuracy
value: 75.25
- type: cos_sim_ap
value: 80.86376001270014
- type: cos_sim_f1
value: 73.65945437441204
- type: cos_sim_precision
value: 64.02289452166802
- type: cos_sim_recall
value: 86.71096345514951
- type: dot_accuracy
value: 75.25
- type: dot_ap
value: 80.93686107633002
- type: dot_f1
value: 73.65945437441204
- type: dot_precision
value: 64.02289452166802
- type: dot_recall
value: 86.71096345514951
- type: euclidean_accuracy
value: 75.25
- type: euclidean_ap
value: 80.86379136218862
- type: euclidean_f1
value: 73.65945437441204
- type: euclidean_precision
value: 64.02289452166802
- type: euclidean_recall
value: 86.71096345514951
- type: manhattan_accuracy
value: 75.3
- type: manhattan_ap
value: 80.87826606097734
- type: manhattan_f1
value: 73.68421052631581
- type: manhattan_precision
value: 64.0
- type: manhattan_recall
value: 86.82170542635659
- type: max_accuracy
value: 75.3
- type: max_ap
value: 80.93686107633002
- type: max_f1
value: 73.68421052631581
- task:
type: STS
dataset:
name: MTEB SICKFr
type: Lajavaness/SICK-fr
config: default
split: test
revision: e077ab4cf4774a1e36d86d593b150422fafd8e8a
metrics:
- type: cos_sim_pearson
value: 81.42349425981143
- type: cos_sim_spearman
value: 78.90454327031226
- type: euclidean_pearson
value: 78.39086497435166
- type: euclidean_spearman
value: 78.9046133980509
- type: manhattan_pearson
value: 78.63743094286502
- type: manhattan_spearman
value: 79.12136348449269
- task:
type: STS
dataset:
name: MTEB STS22 (fr)
type: mteb/sts22-crosslingual-sts
config: fr
split: test
revision: eea2b4fe26a775864c896887d910b76a8098ad3f
metrics:
- type: cos_sim_pearson
value: 81.452697919749
- type: cos_sim_spearman
value: 82.58116836039301
- type: euclidean_pearson
value: 81.04038478932786
- type: euclidean_spearman
value: 82.58116836039301
- type: manhattan_pearson
value: 81.37075396187771
- type: manhattan_spearman
value: 82.73678231355368
- task:
type: STS
dataset:
name: MTEB STSBenchmarkMultilingualSTS (fr)
type: stsb_multi_mt
config: fr
split: test
revision: 93d57ef91790589e3ce9c365164337a8a78b7632
metrics:
- type: cos_sim_pearson
value: 85.7419764013806
- type: cos_sim_spearman
value: 85.46085808849622
- type: euclidean_pearson
value: 83.70449639870063
- type: euclidean_spearman
value: 85.46159013076233
- type: manhattan_pearson
value: 83.95259510313929
- type: manhattan_spearman
value: 85.8029724659458
- task:
type: Summarization
dataset:
name: MTEB SummEvalFr
type: lyon-nlp/summarization-summeval-fr-p2p
config: default
split: test
revision: b385812de6a9577b6f4d0f88c6a6e35395a94054
metrics:
- type: cos_sim_pearson
value: 32.61063271753325
- type: cos_sim_spearman
value: 31.454589417353603
- type: dot_pearson
value: 32.6106288643431
- type: dot_spearman
value: 31.454589417353603
- task:
type: Reranking
dataset:
name: MTEB SyntecReranking
type: lyon-nlp/mteb-fr-reranking-syntec-s2p
config: default
split: test
revision: b205c5084a0934ce8af14338bf03feb19499c84d
metrics:
- type: map
value: 84.31666666666666
- type: mrr
value: 84.31666666666666
- task:
type: Retrieval
dataset:
name: MTEB SyntecRetrieval
type: lyon-nlp/mteb-fr-retrieval-syntec-s2p
config: default
split: test
revision: 77f7e271bf4a92b24fce5119f3486b583ca016ff
metrics:
- type: map_at_1
value: 63.0
- type: map_at_10
value: 73.471
- type: map_at_100
value: 73.87
- type: map_at_1000
value: 73.87
- type: map_at_3
value: 70.5
- type: map_at_5
value: 73.05
- type: mrr_at_1
value: 63.0
- type: mrr_at_10
value: 73.471
- type: mrr_at_100
value: 73.87
- type: mrr_at_1000
value: 73.87
- type: mrr_at_3
value: 70.5
- type: mrr_at_5
value: 73.05
- type: ndcg_at_1
value: 63.0
- type: ndcg_at_10
value: 78.255
- type: ndcg_at_100
value: 79.88
- type: ndcg_at_1000
value: 79.88
- type: ndcg_at_3
value: 72.702
- type: ndcg_at_5
value: 77.264
- type: precision_at_1
value: 63.0
- type: precision_at_10
value: 9.3
- type: precision_at_100
value: 1.0
- type: precision_at_1000
value: 0.1
- type: precision_at_3
value: 26.333000000000002
- type: precision_at_5
value: 18.0
- type: recall_at_1
value: 63.0
- type: recall_at_10
value: 93.0
- type: recall_at_100
value: 100.0
- type: recall_at_1000
value: 100.0
- type: recall_at_3
value: 79.0
- type: recall_at_5
value: 90.0
- task:
type: Retrieval
dataset:
name: MTEB XPQARetrieval (fr)
type: jinaai/xpqa
config: fr
split: test
revision: c99d599f0a6ab9b85b065da6f9d94f9cf731679f
metrics:
- type: map_at_1
value: 40.338
- type: map_at_10
value: 61.927
- type: map_at_100
value: 63.361999999999995
- type: map_at_1000
value: 63.405
- type: map_at_3
value: 55.479
- type: map_at_5
value: 59.732
- type: mrr_at_1
value: 63.551
- type: mrr_at_10
value: 71.006
- type: mrr_at_100
value: 71.501
- type: mrr_at_1000
value: 71.509
- type: mrr_at_3
value: 69.07
- type: mrr_at_5
value: 70.165
- type: ndcg_at_1
value: 63.551
- type: ndcg_at_10
value: 68.297
- type: ndcg_at_100
value: 73.13199999999999
- type: ndcg_at_1000
value: 73.751
- type: ndcg_at_3
value: 62.999
- type: ndcg_at_5
value: 64.89
- type: precision_at_1
value: 63.551
- type: precision_at_10
value: 15.661
- type: precision_at_100
value: 1.9789999999999999
- type: precision_at_1000
value: 0.207
- type: precision_at_3
value: 38.273
- type: precision_at_5
value: 27.61
- type: recall_at_1
value: 40.338
- type: recall_at_10
value: 77.267
- type: recall_at_100
value: 95.892
- type: recall_at_1000
value: 99.75500000000001
- type: recall_at_3
value: 60.36
- type: recall_at_5
value: 68.825
- task:
type: Clustering
dataset:
name: MTEB 8TagsClustering
type: PL-MTEB/8tags-clustering
config: default
split: test
revision: None
metrics:
- type: v_measure
value: 51.36126303874126
- task:
type: Classification
dataset:
name: MTEB AllegroReviews
type: PL-MTEB/allegro-reviews
config: default
split: test
revision: None
metrics:
- type: accuracy
value: 67.13717693836979
- type: f1
value: 57.27609848003782
- task:
type: Retrieval
dataset:
name: MTEB ArguAna-PL
type: clarin-knext/arguana-pl
config: default
split: test
revision: 63fc86750af76253e8c760fc9e534bbf24d260a2
metrics:
- type: map_at_1
value: 35.276999999999994
- type: map_at_10
value: 51.086
- type: map_at_100
value: 51.788000000000004
- type: map_at_1000
value: 51.791
- type: map_at_3
value: 46.147
- type: map_at_5
value: 49.078
- type: mrr_at_1
value: 35.917
- type: mrr_at_10
value: 51.315999999999995
- type: mrr_at_100
value: 52.018
- type: mrr_at_1000
value: 52.022
- type: mrr_at_3
value: 46.349000000000004
- type: mrr_at_5
value: 49.297000000000004
- type: ndcg_at_1
value: 35.276999999999994
- type: ndcg_at_10
value: 59.870999999999995
- type: ndcg_at_100
value: 62.590999999999994
- type: ndcg_at_1000
value: 62.661
- type: ndcg_at_3
value: 49.745
- type: ndcg_at_5
value: 55.067
- type: precision_at_1
value: 35.276999999999994
- type: precision_at_10
value: 8.791
- type: precision_at_100
value: 0.991
- type: precision_at_1000
value: 0.1
- type: precision_at_3
value: 20.057
- type: precision_at_5
value: 14.637
- type: recall_at_1
value: 35.276999999999994
- type: recall_at_10
value: 87.909
- type: recall_at_100
value: 99.14699999999999
- type: recall_at_1000
value: 99.644
- type: recall_at_3
value: 60.171
- type: recall_at_5
value: 73.18599999999999
- task:
type: Classification
dataset:
name: MTEB CBD
type: PL-MTEB/cbd
config: default
split: test
revision: None
metrics:
- type: accuracy
value: 78.03000000000002
- type: ap
value: 29.12548553897622
- type: f1
value: 66.54857118886073
- task:
type: PairClassification
dataset:
name: MTEB CDSC-E
type: PL-MTEB/cdsce-pairclassification
config: default
split: test
revision: None
metrics:
- type: cos_sim_accuracy
value: 89.0
- type: cos_sim_ap
value: 76.75437826834582
- type: cos_sim_f1
value: 66.4850136239782
- type: cos_sim_precision
value: 68.92655367231639
- type: cos_sim_recall
value: 64.21052631578948
- type: dot_accuracy
value: 89.0
- type: dot_ap
value: 76.75437826834582
- type: dot_f1
value: 66.4850136239782
- type: dot_precision
value: 68.92655367231639
- type: dot_recall
value: 64.21052631578948
- type: euclidean_accuracy
value: 89.0
- type: euclidean_ap
value: 76.75437826834582
- type: euclidean_f1
value: 66.4850136239782
- type: euclidean_precision
value: 68.92655367231639
- type: euclidean_recall
value: 64.21052631578948
- type: manhattan_accuracy
value: 89.0
- type: manhattan_ap
value: 76.66074220647083
- type: manhattan_f1
value: 66.47058823529412
- type: manhattan_precision
value: 75.33333333333333
- type: manhattan_recall
value: 59.473684210526315
- type: max_accuracy
value: 89.0
- type: max_ap
value: 76.75437826834582
- type: max_f1
value: 66.4850136239782
- task:
type: STS
dataset:
name: MTEB CDSC-R
type: PL-MTEB/cdscr-sts
config: default
split: test
revision: None
metrics:
- type: cos_sim_pearson
value: 93.12903172428328
- type: cos_sim_spearman
value: 92.66381487060741
- type: euclidean_pearson
value: 90.37278396708922
- type: euclidean_spearman
value: 92.66381487060741
- type: manhattan_pearson
value: 90.32503296540962
- type: manhattan_spearman
value: 92.6902938354313
- task:
type: Retrieval
dataset:
name: MTEB DBPedia-PL
type: clarin-knext/dbpedia-pl
config: default
split: test
revision: 76afe41d9af165cc40999fcaa92312b8b012064a
metrics:
- type: map_at_1
value: 8.83
- type: map_at_10
value: 18.326
- type: map_at_100
value: 26.496
- type: map_at_1000
value: 28.455000000000002
- type: map_at_3
value: 12.933
- type: map_at_5
value: 15.168000000000001
- type: mrr_at_1
value: 66.0
- type: mrr_at_10
value: 72.76700000000001
- type: mrr_at_100
value: 73.203
- type: mrr_at_1000
value: 73.219
- type: mrr_at_3
value: 71.458
- type: mrr_at_5
value: 72.246
- type: ndcg_at_1
value: 55.375
- type: ndcg_at_10
value: 41.3
- type: ndcg_at_100
value: 45.891
- type: ndcg_at_1000
value: 52.905
- type: ndcg_at_3
value: 46.472
- type: ndcg_at_5
value: 43.734
- type: precision_at_1
value: 66.0
- type: precision_at_10
value: 33.074999999999996
- type: precision_at_100
value: 11.094999999999999
- type: precision_at_1000
value: 2.374
- type: precision_at_3
value: 48.583
- type: precision_at_5
value: 42.0
- type: recall_at_1
value: 8.83
- type: recall_at_10
value: 22.587
- type: recall_at_100
value: 50.61600000000001
- type: recall_at_1000
value: 73.559
- type: recall_at_3
value: 13.688
- type: recall_at_5
value: 16.855
- task:
type: Retrieval
dataset:
name: MTEB FiQA-PL
type: clarin-knext/fiqa-pl
config: default
split: test
revision: 2e535829717f8bf9dc829b7f911cc5bbd4e6608e
metrics:
- type: map_at_1
value: 20.587
- type: map_at_10
value: 33.095
- type: map_at_100
value: 35.24
- type: map_at_1000
value: 35.429
- type: map_at_3
value: 28.626
- type: map_at_5
value: 31.136999999999997
- type: mrr_at_1
value: 40.586
- type: mrr_at_10
value: 49.033
- type: mrr_at_100
value: 49.952999999999996
- type: mrr_at_1000
value: 49.992
- type: mrr_at_3
value: 46.553
- type: mrr_at_5
value: 48.035
- type: ndcg_at_1
value: 40.586
- type: ndcg_at_10
value: 41.046
- type: ndcg_at_100
value: 48.586
- type: ndcg_at_1000
value: 51.634
- type: ndcg_at_3
value: 36.773
- type: ndcg_at_5
value: 38.389
- type: precision_at_1
value: 40.586
- type: precision_at_10
value: 11.466
- type: precision_at_100
value: 1.909
- type: precision_at_1000
value: 0.245
- type: precision_at_3
value: 24.434
- type: precision_at_5
value: 18.426000000000002
- type: recall_at_1
value: 20.587
- type: recall_at_10
value: 47.986000000000004
- type: recall_at_100
value: 75.761
- type: recall_at_1000
value: 94.065
- type: recall_at_3
value: 33.339
- type: recall_at_5
value: 39.765
- task:
type: Retrieval
dataset:
name: MTEB HotpotQA-PL
type: clarin-knext/hotpotqa-pl
config: default
split: test
revision: a0bd479ac97b4ccb5bd6ce320c415d0bb4beb907
metrics:
- type: map_at_1
value: 40.878
- type: map_at_10
value: 58.775999999999996
- type: map_at_100
value: 59.632
- type: map_at_1000
value: 59.707
- type: map_at_3
value: 56.074
- type: map_at_5
value: 57.629
- type: mrr_at_1
value: 81.756
- type: mrr_at_10
value: 86.117
- type: mrr_at_100
value: 86.299
- type: mrr_at_1000
value: 86.30600000000001
- type: mrr_at_3
value: 85.345
- type: mrr_at_5
value: 85.832
- type: ndcg_at_1
value: 81.756
- type: ndcg_at_10
value: 67.608
- type: ndcg_at_100
value: 70.575
- type: ndcg_at_1000
value: 71.99600000000001
- type: ndcg_at_3
value: 63.723
- type: ndcg_at_5
value: 65.70700000000001
- type: precision_at_1
value: 81.756
- type: precision_at_10
value: 13.619
- type: precision_at_100
value: 1.5939999999999999
- type: precision_at_1000
value: 0.178
- type: precision_at_3
value: 39.604
- type: precision_at_5
value: 25.332
- type: recall_at_1
value: 40.878
- type: recall_at_10
value: 68.096
- type: recall_at_100
value: 79.696
- type: recall_at_1000
value: 89.082
- type: recall_at_3
value: 59.406000000000006
- type: recall_at_5
value: 63.329
- task:
type: Retrieval
dataset:
name: MTEB MSMARCO-PL
type: clarin-knext/msmarco-pl
config: default
split: test
revision: 8634c07806d5cce3a6138e260e59b81760a0a640
metrics:
- type: map_at_1
value: 2.1839999999999997
- type: map_at_10
value: 11.346
- type: map_at_100
value: 30.325000000000003
- type: map_at_1000
value: 37.806
- type: map_at_3
value: 4.842
- type: map_at_5
value: 6.891
- type: mrr_at_1
value: 86.047
- type: mrr_at_10
value: 89.14699999999999
- type: mrr_at_100
value: 89.46600000000001
- type: mrr_at_1000
value: 89.46600000000001
- type: mrr_at_3
value: 89.14699999999999
- type: mrr_at_5
value: 89.14699999999999
- type: ndcg_at_1
value: 67.829
- type: ndcg_at_10
value: 62.222
- type: ndcg_at_100
value: 55.337
- type: ndcg_at_1000
value: 64.076
- type: ndcg_at_3
value: 68.12700000000001
- type: ndcg_at_5
value: 64.987
- type: precision_at_1
value: 86.047
- type: precision_at_10
value: 69.535
- type: precision_at_100
value: 32.93
- type: precision_at_1000
value: 6.6049999999999995
- type: precision_at_3
value: 79.845
- type: precision_at_5
value: 75.349
- type: recall_at_1
value: 2.1839999999999997
- type: recall_at_10
value: 12.866
- type: recall_at_100
value: 43.505
- type: recall_at_1000
value: 72.366
- type: recall_at_3
value: 4.947
- type: recall_at_5
value: 7.192
- task:
type: Classification
dataset:
name: MTEB MassiveIntentClassification (pl)
type: mteb/amazon_massive_intent
config: pl
split: test
revision: 31efe3c427b0bae9c22cbb560b8f15491cc6bed7
metrics:
- type: accuracy
value: 80.75319435104238
- type: f1
value: 77.58961444860606
- task:
type: Classification
dataset:
name: MTEB MassiveScenarioClassification (pl)
type: mteb/amazon_massive_scenario
config: pl
split: test
revision: 7d571f92784cd94a019292a1f45445077d0ef634
metrics:
- type: accuracy
value: 85.54472091459313
- type: f1
value: 84.29498563572106
- task:
type: Retrieval
dataset:
name: MTEB NFCorpus-PL
type: clarin-knext/nfcorpus-pl
config: default
split: test
revision: 9a6f9567fda928260afed2de480d79c98bf0bec0
metrics:
- type: map_at_1
value: 4.367
- type: map_at_10
value: 10.38
- type: map_at_100
value: 13.516
- type: map_at_1000
value: 14.982000000000001
- type: map_at_3
value: 7.367
- type: map_at_5
value: 8.59
- type: mrr_at_1
value: 41.486000000000004
- type: mrr_at_10
value: 48.886
- type: mrr_at_100
value: 49.657000000000004
- type: mrr_at_1000
value: 49.713
- type: mrr_at_3
value: 46.904
- type: mrr_at_5
value: 48.065000000000005
- type: ndcg_at_1
value: 40.402
- type: ndcg_at_10
value: 30.885
- type: ndcg_at_100
value: 28.393
- type: ndcg_at_1000
value: 37.428
- type: ndcg_at_3
value: 35.394999999999996
- type: ndcg_at_5
value: 33.391999999999996
- type: precision_at_1
value: 41.486000000000004
- type: precision_at_10
value: 23.437
- type: precision_at_100
value: 7.638
- type: precision_at_1000
value: 2.0389999999999997
- type: precision_at_3
value: 32.817
- type: precision_at_5
value: 28.915999999999997
- type: recall_at_1
value: 4.367
- type: recall_at_10
value: 14.655000000000001
- type: recall_at_100
value: 29.665999999999997
- type: recall_at_1000
value: 62.073
- type: recall_at_3
value: 8.51
- type: recall_at_5
value: 10.689
- task:
type: Retrieval
dataset:
name: MTEB NQ-PL
type: clarin-knext/nq-pl
config: default
split: test
revision: f171245712cf85dd4700b06bef18001578d0ca8d
metrics:
- type: map_at_1
value: 28.616000000000003
- type: map_at_10
value: 41.626000000000005
- type: map_at_100
value: 42.689
- type: map_at_1000
value: 42.733
- type: map_at_3
value: 37.729
- type: map_at_5
value: 39.879999999999995
- type: mrr_at_1
value: 32.068000000000005
- type: mrr_at_10
value: 44.029
- type: mrr_at_100
value: 44.87
- type: mrr_at_1000
value: 44.901
- type: mrr_at_3
value: 40.687
- type: mrr_at_5
value: 42.625
- type: ndcg_at_1
value: 32.068000000000005
- type: ndcg_at_10
value: 48.449999999999996
- type: ndcg_at_100
value: 53.13
- type: ndcg_at_1000
value: 54.186
- type: ndcg_at_3
value: 40.983999999999995
- type: ndcg_at_5
value: 44.628
- type: precision_at_1
value: 32.068000000000005
- type: precision_at_10
value: 7.9750000000000005
- type: precision_at_100
value: 1.061
- type: precision_at_1000
value: 0.116
- type: precision_at_3
value: 18.404999999999998
- type: precision_at_5
value: 13.111
- type: recall_at_1
value: 28.616000000000003
- type: recall_at_10
value: 66.956
- type: recall_at_100
value: 87.657
- type: recall_at_1000
value: 95.548
- type: recall_at_3
value: 47.453
- type: recall_at_5
value: 55.87800000000001
- task:
type: Classification
dataset:
name: MTEB PAC
type: laugustyniak/abusive-clauses-pl
config: default
split: test
revision: None
metrics:
- type: accuracy
value: 69.04141326382856
- type: ap
value: 77.47589122111044
- type: f1
value: 66.6332277374775
- task:
type: PairClassification
dataset:
name: MTEB PPC
type: PL-MTEB/ppc-pairclassification
config: default
split: test
revision: None
metrics:
- type: cos_sim_accuracy
value: 86.4
- type: cos_sim_ap
value: 94.1044939667201
- type: cos_sim_f1
value: 88.78048780487805
- type: cos_sim_precision
value: 87.22044728434504
- type: cos_sim_recall
value: 90.39735099337747
- type: dot_accuracy
value: 86.4
- type: dot_ap
value: 94.1044939667201
- type: dot_f1
value: 88.78048780487805
- type: dot_precision
value: 87.22044728434504
- type: dot_recall
value: 90.39735099337747
- type: euclidean_accuracy
value: 86.4
- type: euclidean_ap
value: 94.1044939667201
- type: euclidean_f1
value: 88.78048780487805
- type: euclidean_precision
value: 87.22044728434504
- type: euclidean_recall
value: 90.39735099337747
- type: manhattan_accuracy
value: 86.4
- type: manhattan_ap
value: 94.11438365697387
- type: manhattan_f1
value: 88.77968877968877
- type: manhattan_precision
value: 87.84440842787681
- type: manhattan_recall
value: 89.73509933774835
- type: max_accuracy
value: 86.4
- type: max_ap
value: 94.11438365697387
- type: max_f1
value: 88.78048780487805
- task:
type: PairClassification
dataset:
name: MTEB PSC
type: PL-MTEB/psc-pairclassification
config: default
split: test
revision: None
metrics:
- type: cos_sim_accuracy
value: 97.86641929499072
- type: cos_sim_ap
value: 99.36904211868182
- type: cos_sim_f1
value: 96.56203288490283
- type: cos_sim_precision
value: 94.72140762463343
- type: cos_sim_recall
value: 98.47560975609755
- type: dot_accuracy
value: 97.86641929499072
- type: dot_ap
value: 99.36904211868183
- type: dot_f1
value: 96.56203288490283
- type: dot_precision
value: 94.72140762463343
- type: dot_recall
value: 98.47560975609755
- type: euclidean_accuracy
value: 97.86641929499072
- type: euclidean_ap
value: 99.36904211868183
- type: euclidean_f1
value: 96.56203288490283
- type: euclidean_precision
value: 94.72140762463343
- type: euclidean_recall
value: 98.47560975609755
- type: manhattan_accuracy
value: 98.14471243042672
- type: manhattan_ap
value: 99.43359540492416
- type: manhattan_f1
value: 96.98795180722892
- type: manhattan_precision
value: 95.83333333333334
- type: manhattan_recall
value: 98.17073170731707
- type: max_accuracy
value: 98.14471243042672
- type: max_ap
value: 99.43359540492416
- type: max_f1
value: 96.98795180722892
- task:
type: Classification
dataset:
name: MTEB PolEmo2.0-IN
type: PL-MTEB/polemo2_in
config: default
split: test
revision: None
metrics:
- type: accuracy
value: 89.39058171745152
- type: f1
value: 86.8552093529568
- task:
type: Classification
dataset:
name: MTEB PolEmo2.0-OUT
type: PL-MTEB/polemo2_out
config: default
split: test
revision: None
metrics:
- type: accuracy
value: 74.97975708502024
- type: f1
value: 58.73081628832407
- task:
type: Retrieval
dataset:
name: MTEB Quora-PL
type: clarin-knext/quora-pl
config: default
split: test
revision: 0be27e93455051e531182b85e85e425aba12e9d4
metrics:
- type: map_at_1
value: 64.917
- type: map_at_10
value: 78.74600000000001
- type: map_at_100
value: 79.501
- type: map_at_1000
value: 79.524
- type: map_at_3
value: 75.549
- type: map_at_5
value: 77.495
- type: mrr_at_1
value: 74.9
- type: mrr_at_10
value: 82.112
- type: mrr_at_100
value: 82.314
- type: mrr_at_1000
value: 82.317
- type: mrr_at_3
value: 80.745
- type: mrr_at_5
value: 81.607
- type: ndcg_at_1
value: 74.83999999999999
- type: ndcg_at_10
value: 83.214
- type: ndcg_at_100
value: 84.997
- type: ndcg_at_1000
value: 85.207
- type: ndcg_at_3
value: 79.547
- type: ndcg_at_5
value: 81.46600000000001
- type: precision_at_1
value: 74.83999999999999
- type: precision_at_10
value: 12.822
- type: precision_at_100
value: 1.506
- type: precision_at_1000
value: 0.156
- type: precision_at_3
value: 34.903
- type: precision_at_5
value: 23.16
- type: recall_at_1
value: 64.917
- type: recall_at_10
value: 92.27199999999999
- type: recall_at_100
value: 98.715
- type: recall_at_1000
value: 99.854
- type: recall_at_3
value: 82.04599999999999
- type: recall_at_5
value: 87.2
- task:
type: Retrieval
dataset:
name: MTEB SCIDOCS-PL
type: clarin-knext/scidocs-pl
config: default
split: test
revision: 45452b03f05560207ef19149545f168e596c9337
metrics:
- type: map_at_1
value: 3.51
- type: map_at_10
value: 9.046999999999999
- type: map_at_100
value: 10.823
- type: map_at_1000
value: 11.144
- type: map_at_3
value: 6.257
- type: map_at_5
value: 7.648000000000001
- type: mrr_at_1
value: 17.299999999999997
- type: mrr_at_10
value: 27.419
- type: mrr_at_100
value: 28.618
- type: mrr_at_1000
value: 28.685
- type: mrr_at_3
value: 23.817
- type: mrr_at_5
value: 25.927
- type: ndcg_at_1
value: 17.299999999999997
- type: ndcg_at_10
value: 16.084
- type: ndcg_at_100
value: 23.729
- type: ndcg_at_1000
value: 29.476999999999997
- type: ndcg_at_3
value: 14.327000000000002
- type: ndcg_at_5
value: 13.017999999999999
- type: precision_at_1
value: 17.299999999999997
- type: precision_at_10
value: 8.63
- type: precision_at_100
value: 1.981
- type: precision_at_1000
value: 0.336
- type: precision_at_3
value: 13.4
- type: precision_at_5
value: 11.700000000000001
- type: recall_at_1
value: 3.51
- type: recall_at_10
value: 17.518
- type: recall_at_100
value: 40.275
- type: recall_at_1000
value: 68.203
- type: recall_at_3
value: 8.155
- type: recall_at_5
value: 11.875
- task:
type: PairClassification
dataset:
name: MTEB SICK-E-PL
type: PL-MTEB/sicke-pl-pairclassification
config: default
split: test
revision: None
metrics:
- type: cos_sim_accuracy
value: 86.30248675091724
- type: cos_sim_ap
value: 83.6756734006714
- type: cos_sim_f1
value: 74.97367497367497
- type: cos_sim_precision
value: 73.91003460207612
- type: cos_sim_recall
value: 76.06837606837607
- type: dot_accuracy
value: 86.30248675091724
- type: dot_ap
value: 83.6756734006714
- type: dot_f1
value: 74.97367497367497
- type: dot_precision
value: 73.91003460207612
- type: dot_recall
value: 76.06837606837607
- type: euclidean_accuracy
value: 86.30248675091724
- type: euclidean_ap
value: 83.67566984333091
- type: euclidean_f1
value: 74.97367497367497
- type: euclidean_precision
value: 73.91003460207612
- type: euclidean_recall
value: 76.06837606837607
- type: manhattan_accuracy
value: 86.28210354667753
- type: manhattan_ap
value: 83.64216119130171
- type: manhattan_f1
value: 74.92152075340078
- type: manhattan_precision
value: 73.4107997265892
- type: manhattan_recall
value: 76.49572649572649
- type: max_accuracy
value: 86.30248675091724
- type: max_ap
value: 83.6756734006714
- type: max_f1
value: 74.97367497367497
- task:
type: STS
dataset:
name: MTEB SICK-R-PL
type: PL-MTEB/sickr-pl-sts
config: default
split: test
revision: None
metrics:
- type: cos_sim_pearson
value: 82.23295940859121
- type: cos_sim_spearman
value: 78.89329160768719
- type: euclidean_pearson
value: 79.56019107076818
- type: euclidean_spearman
value: 78.89330209904084
- type: manhattan_pearson
value: 79.76098513973719
- type: manhattan_spearman
value: 79.05490162570123
- task:
type: STS
dataset:
name: MTEB STS22 (pl)
type: mteb/sts22-crosslingual-sts
config: pl
split: test
revision: eea2b4fe26a775864c896887d910b76a8098ad3f
metrics:
- type: cos_sim_pearson
value: 37.732606308062486
- type: cos_sim_spearman
value: 41.01645667030284
- type: euclidean_pearson
value: 26.61722556367085
- type: euclidean_spearman
value: 41.01645667030284
- type: manhattan_pearson
value: 26.60917378970807
- type: manhattan_spearman
value: 41.51335727617614
- task:
type: Retrieval
dataset:
name: MTEB SciFact-PL
type: clarin-knext/scifact-pl
config: default
split: test
revision: 47932a35f045ef8ed01ba82bf9ff67f6e109207e
metrics:
- type: map_at_1
value: 54.31700000000001
- type: map_at_10
value: 65.564
- type: map_at_100
value: 66.062
- type: map_at_1000
value: 66.08699999999999
- type: map_at_3
value: 62.592999999999996
- type: map_at_5
value: 63.888
- type: mrr_at_1
value: 56.99999999999999
- type: mrr_at_10
value: 66.412
- type: mrr_at_100
value: 66.85900000000001
- type: mrr_at_1000
value: 66.88
- type: mrr_at_3
value: 64.22200000000001
- type: mrr_at_5
value: 65.206
- type: ndcg_at_1
value: 56.99999999999999
- type: ndcg_at_10
value: 70.577
- type: ndcg_at_100
value: 72.879
- type: ndcg_at_1000
value: 73.45
- type: ndcg_at_3
value: 65.5
- type: ndcg_at_5
value: 67.278
- type: precision_at_1
value: 56.99999999999999
- type: precision_at_10
value: 9.667
- type: precision_at_100
value: 1.083
- type: precision_at_1000
value: 0.11299999999999999
- type: precision_at_3
value: 26.0
- type: precision_at_5
value: 16.933
- type: recall_at_1
value: 54.31700000000001
- type: recall_at_10
value: 85.056
- type: recall_at_100
value: 95.667
- type: recall_at_1000
value: 100.0
- type: recall_at_3
value: 71.0
- type: recall_at_5
value: 75.672
- task:
type: Retrieval
dataset:
name: MTEB TRECCOVID-PL
type: clarin-knext/trec-covid-pl
config: default
split: test
revision: 81bcb408f33366c2a20ac54adafad1ae7e877fdd
metrics:
- type: map_at_1
value: 0.245
- type: map_at_10
value: 2.051
- type: map_at_100
value: 12.009
- type: map_at_1000
value: 27.448
- type: map_at_3
value: 0.721
- type: map_at_5
value: 1.13
- type: mrr_at_1
value: 88.0
- type: mrr_at_10
value: 93.0
- type: mrr_at_100
value: 93.0
- type: mrr_at_1000
value: 93.0
- type: mrr_at_3
value: 93.0
- type: mrr_at_5
value: 93.0
- type: ndcg_at_1
value: 85.0
- type: ndcg_at_10
value: 80.303
- type: ndcg_at_100
value: 61.23499999999999
- type: ndcg_at_1000
value: 52.978
- type: ndcg_at_3
value: 84.419
- type: ndcg_at_5
value: 82.976
- type: precision_at_1
value: 88.0
- type: precision_at_10
value: 83.39999999999999
- type: precision_at_100
value: 61.96
- type: precision_at_1000
value: 22.648
- type: precision_at_3
value: 89.333
- type: precision_at_5
value: 87.2
- type: recall_at_1
value: 0.245
- type: recall_at_10
value: 2.193
- type: recall_at_100
value: 14.938
- type: recall_at_1000
value: 48.563
- type: recall_at_3
value: 0.738
- type: recall_at_5
value: 1.173
---
# Fashion-Italia/gte-Qwen2-7B-instruct-Q4_K_M-GGUF
This model was converted to GGUF format from [`Alibaba-NLP/gte-Qwen2-7B-instruct`](https://huggingface.co/Alibaba-NLP/gte-Qwen2-7B-instruct) using llama.cpp via the ggml.ai's [GGUF-my-repo](https://huggingface.co/spaces/ggml-org/gguf-my-repo) space.
Refer to the [original model card](https://huggingface.co/Alibaba-NLP/gte-Qwen2-7B-instruct) for more details on the model.
## Use with llama.cpp
Install llama.cpp through brew (works on Mac and Linux)
```bash
brew install llama.cpp
```
Invoke the llama.cpp server or the CLI.
### CLI:
```bash
llama-cli --hf-repo Fashion-Italia/gte-Qwen2-7B-instruct-Q4_K_M-GGUF --hf-file gte-qwen2-7b-instruct-q4_k_m.gguf -p "The meaning to life and the universe is"
```
### Server:
```bash
llama-server --hf-repo Fashion-Italia/gte-Qwen2-7B-instruct-Q4_K_M-GGUF --hf-file gte-qwen2-7b-instruct-q4_k_m.gguf -c 2048
```
Note: You can also use this checkpoint directly through the [usage steps](https://github.com/ggerganov/llama.cpp?tab=readme-ov-file#usage) listed in the Llama.cpp repo as well.
Step 1: Clone llama.cpp from GitHub.
```
git clone https://github.com/ggerganov/llama.cpp
```
Step 2: Move into the llama.cpp folder and build it with `LLAMA_CURL=1` flag along with other hardware-specific flags (for ex: LLAMA_CUDA=1 for Nvidia GPUs on Linux).
```
cd llama.cpp && LLAMA_CURL=1 make
```
Step 3: Run inference through the main binary.
```
./llama-cli --hf-repo Fashion-Italia/gte-Qwen2-7B-instruct-Q4_K_M-GGUF --hf-file gte-qwen2-7b-instruct-q4_k_m.gguf -p "The meaning to life and the universe is"
```
or
```
./llama-server --hf-repo Fashion-Italia/gte-Qwen2-7B-instruct-Q4_K_M-GGUF --hf-file gte-qwen2-7b-instruct-q4_k_m.gguf -c 2048
```
| [
"SUMMARIZATION"
] | Non_BioNLP |
ibm-research/re2g-ctx-encoder-trex | ibm-research | null | [
"transformers",
"pytorch",
"dpr",
"information retrieval",
"reranking",
"license:apache-2.0",
"endpoints_compatible",
"region:us"
] | 1,659,118,360,000 | 2023-05-16T14:49:38 | 21 | 0 | ---
license: apache-2.0
tags:
- information retrieval
- reranking
---
# Model Card for T-REx Context Encoder in Re2G
# Model Details
> The approach of RAG, Multi-DPR, and KGI is to train a neural IR (Information Retrieval) component and further train it end-to-end through its impact in generating the correct output.
<img src="https://github.com/IBM/kgi-slot-filling/raw/re2g/model_cards/Re2G_Arch2.png" width="100%">
## Training, Evaluation and Inference
The code for training, evaluation and inference is in our github in the [re2g branch](https://github.com/IBM/kgi-slot-filling/tree/re2g).
## Usage
The best way to use the model is by adapting the [dpr_apply.py](https://github.com/IBM/kgi-slot-filling/blob/re2g/dpr/dpr_apply.py)
## Citation
```
@inproceedings{glass-etal-2022-re2g,
title = "{R}e2{G}: Retrieve, Rerank, Generate",
author = "Glass, Michael and
Rossiello, Gaetano and
Chowdhury, Md Faisal Mahbub and
Naik, Ankita and
Cai, Pengshan and
Gliozzo, Alfio",
booktitle = "Proceedings of the 2022 Conference of the North American Chapter of the Association for Computational Linguistics: Human Language Technologies",
month = jul,
year = "2022",
address = "Seattle, United States",
publisher = "Association for Computational Linguistics",
url = "https://aclanthology.org/2022.naacl-main.194",
doi = "10.18653/v1/2022.naacl-main.194",
pages = "2701--2715",
abstract = "As demonstrated by GPT-3 and T5, transformers grow in capability as parameter spaces become larger and larger. However, for tasks that require a large amount of knowledge, non-parametric memory allows models to grow dramatically with a sub-linear increase in computational cost and GPU memory requirements. Recent models such as RAG and REALM have introduced retrieval into conditional generation. These models incorporate neural initial retrieval from a corpus of passages. We build on this line of research, proposing Re2G, which combines both neural initial retrieval and reranking into a BART-based sequence-to-sequence generation. Our reranking approach also permits merging retrieval results from sources with incomparable scores, enabling an ensemble of BM25 and neural initial retrieval. To train our system end-to-end, we introduce a novel variation of knowledge distillation to train the initial retrieval, reranker and generation using only ground truth on the target sequence output. We find large gains in four diverse tasks: zero-shot slot filling, question answering, fact checking and dialog, with relative gains of 9{\%} to 34{\%} over the previous state-of-the-art on the KILT leaderboard. We make our code available as open source.",
}
```
## Model Description
The model creators note in the [associated paper](https://aclanthology.org/2022.naacl-main.194.pdf):
> As demonstrated by GPT-3 and T5, transformers grow in capability as parameter spaces become larger and larger. However, for tasks that require a large amount of knowledge, non-parametric memory allows models to grow dramatically with a sub-linear increase in computational cost and GPU memory requirements. Recent models such as RAG and REALM have introduced retrieval into conditional generation. These models incorporate neural initial retrieval from a corpus of passages. We build on this line of research, proposing Re2G, which combines both neural initial retrieval and reranking into a BART-based sequence-to-sequence generation. Our reranking approach also permits merging retrieval results from sources with incomparable scores, enabling an ensemble of BM25 and neural initial retrieval. To train our system end-to-end, we introduce a novel variation of knowledge distillation to train the initial retrieval, reranker and generation using only ground truth on the target sequence output. We find large gains in four diverse tasks: zero-shot slot filling, question answering, fact checking and dialog, with relative gains of 9% to 34% over the previous state-of-the-art on the KILT leaderboard. We make our code available as open source.
- **Developed by:** IBM
- **Shared by [Optional]:** IBM
- **Model type:** Query/Passage Reranker
- **Language(s) (NLP):** English
- **License:** Apache 2.0
- **Parent Model:** [dpr-question_encoder-multiset-base](https://huggingface.co/facebook/dpr-question_encoder-multiset-base)
- **Resources for more information:**
- [GitHub Repo](https://github.com/IBM/kgi-slot-filling)
- [Associated Paper](https://aclanthology.org/2022.naacl-main.194.pdf)
# Uses
## Direct Use
This model can be used for the task of encoding a passage to a vector, this passage or context vector should then be indexed into an Approximate Nearest Neighbors index. It must be used in combination with a query or question encoder that encodes a question to a query vector to search the index.
# Citation
**BibTeX:**
```bibtex
@inproceedings{glass-etal-2022-re2g,
title = "{R}e2{G}: Retrieve, Rerank, Generate",
author = "Glass, Michael and
Rossiello, Gaetano and
Chowdhury, Md Faisal Mahbub and
Naik, Ankita and
Cai, Pengshan and
Gliozzo, Alfio",
booktitle = "Proceedings of the 2022 Conference of the North American Chapter of the Association for Computational Linguistics: Human Language Technologies",
month = jul,
year = "2022",
address = "Seattle, United States",
publisher = "Association for Computational Linguistics",
url = "https://aclanthology.org/2022.naacl-main.194",
doi = "10.18653/v1/2022.naacl-main.194",
pages = "2701--2715",
abstract = "As demonstrated by GPT-3 and T5, transformers grow in capability as parameter spaces become larger and larger. However, for tasks that require a large amount of knowledge, non-parametric memory allows models to grow dramatically with a sub-linear increase in computational cost and GPU memory requirements. Recent models such as RAG and REALM have introduced retrieval into conditional generation. These models incorporate neural initial retrieval from a corpus of passages. We build on this line of research, proposing Re2G, which combines both neural initial retrieval and reranking into a BART-based sequence-to-sequence generation. Our reranking approach also permits merging retrieval results from sources with incomparable scores, enabling an ensemble of BM25 and neural initial retrieval. To train our system end-to-end, we introduce a novel variation of knowledge distillation to train the initial retrieval, reranker and generation using only ground truth on the target sequence output. We find large gains in four diverse tasks: zero-shot slot filling, question answering, fact checking and dialog, with relative gains of 9{\%} to 34{\%} over the previous state-of-the-art on the KILT leaderboard. We make our code available as open source.",
}
```
| [
"QUESTION_ANSWERING"
] | Non_BioNLP |
gaudi/opus-mt-en-kqn-ctranslate2 | gaudi | translation | [
"transformers",
"marian",
"ctranslate2",
"translation",
"license:apache-2.0",
"endpoints_compatible",
"region:us"
] | 1,721,314,857,000 | 2024-10-19T00:18:19 | 8 | 0 | ---
license: apache-2.0
tags:
- ctranslate2
- translation
---
# Repository General Information
## Inspired by and derived from the work of [Helsinki-NLP](https://huggingface.co/Helsinki-NLP), [CTranslate2](https://github.com/OpenNMT/CTranslate2), and [michaelfeil](https://huggingface.co/michaelfeil)!
- Link to Original Model ([Helsinki-NLP](https://huggingface.co/Helsinki-NLP)): [Model Link](https://huggingface.co/Helsinki-NLP/opus-mt-en-kqn)
- This respository was based on the work of [CTranslate2](https://github.com/OpenNMT/CTranslate2).
- This repository was based on the work of [michaelfeil](https://huggingface.co/michaelfeil).
# What is CTranslate2?
[CTranslate2](https://opennmt.net/CTranslate2/) is a C++ and Python library for efficient inference with Transformer models.
CTranslate2 implements a custom runtime that applies many performance optimization techniques such as weights quantization, layers fusion, batch reordering, etc., to accelerate and reduce the memory usage of Transformer models on CPU and GPU.
CTranslate2 is one of the most performant ways of hosting translation models at scale. Current supported models include:
- Encoder-decoder models: Transformer base/big, M2M-100, NLLB, BART, mBART, Pegasus, T5, Whisper
- Decoder-only models: GPT-2, GPT-J, GPT-NeoX, OPT, BLOOM, MPT, Llama, Mistral, Gemma, CodeGen, GPTBigCode, Falcon
- Encoder-only models: BERT, DistilBERT, XLM-RoBERTa
The project is production-oriented and comes with backward compatibility guarantees, but it also includes experimental features related to model compression and inference acceleration.
# CTranslate2 Benchmarks
Please note that the results presented below are only valid for the configuration used during this benchmark: absolute and relative performance may change with different settings. Tested against `newstest2014` (En -> De) dataset.
The benchmark reports the number of target tokens generated per second (higher is better). The results are aggregated over multiple runs. See the benchmark scripts for more details and reproduce these numbers.
Please note that the results presented below are only valid for the configuration used during this benchmark: absolute and relative performance may change with different settings.
## CPU Benchmarks for Generic Opus-MT Models
| Library | Tokens per Second | Max Memory Usage | BLEU |
| :----: | :----: | :----: | :----: |
| Transformers 4.26.1 (with PyTorch 1.13.1) | 147.3 | 2332MB | 27.90 |
| Marian 1.11.0 (int16) | 330.2 | 5901MB | 27.65 |
| Marian 1.11.0 (int8) | 355.8 | 4763MB | 27.27 |
| CTranslate2 3.6.0 (int16) | 596.1 | 660MB | 27.53 |
| CTranslate2 3.6.0 (int8) | 696.1 | 516MB | 27.65 |
## GPU Benchmarks for Generic Opus-MT Models
| Library | Tokens per Second | Max GPU Memory Usage | Max Memory Usage | BLEU |
| :----: | :----: | :----: | :----: | :----: |
| Transformers 4.26.1 (with PyTorch 1.13.1) | 1022.9 | 4097MB | 2109MB | 27.90 |
| Marian 1.11.0 (float16) | 3962.4 | 3239MB | 1976MB | 27.94 |
| CTranslate2 3.6.0 (float16) | 9296.7 | 909MB | 814MB | 27.9 |
| CTranslate2 3.6.0 (int8 + float16) | 8362.7 | 813MB | 766MB | 27.9 |
`Executed with 4 threads on a c5.2xlarge Amazon EC2 instance equipped with an Intel(R) Xeon(R) Platinum 8275CL CPU.`
**Source to benchmark information can be found [here](https://github.com/OpenNMT/CTranslate2).**<br />
**Original model BLEU scores can be found [here](https://huggingface.co/Helsinki-NLP/opus-mt-en-kqn).**
## Internal Benchmarks
Internal testing on our end showed **inference times reduced by 6x-10x** on average compared the vanilla checkpoints using the *transformers* library. A **slight reduction on BLEU scores (~5%)** was also identified in comparison to the vanilla checkpoints with a few exceptions. This is likely due to several factors, one being the quantization applied. Further testing is needed from our end to better assess the reduction in translation quality. The command used to compile the vanilla checkpoint into a CTranslate2 model can be found below. Modifying this command can yield differing balances between inferencing performance and translation quality.
# CTranslate2 Installation
```bash
pip install hf-hub-ctranslate2>=1.0.0 ctranslate2>=3.13.0
```
### ct2-transformers-converter Command Used:
```bash
ct2-transformers-converter --model Helsinki-NLP/opus-mt-en-kqn --output_dir ./ctranslate2/opus-mt-en-kqn-ctranslate2 --force --copy_files README.md generation_config.json tokenizer_config.json vocab.json source.spm .gitattributes target.spm --quantization float16
```
# CTranslate2 Converted Checkpoint Information:
**Compatible With:**
- [ctranslate2](https://github.com/OpenNMT/CTranslate2)
- [hf-hub-ctranslate2](https://github.com/michaelfeil/hf-hub-ctranslate2)
**Compute Type:**
- `compute_type=int8_float16` for `device="cuda"`
- `compute_type=int8` for `device="cpu"`
# Sample Code - ctranslate2
#### Clone the repository to the working directory or wherever you wish to store the model artifacts. ####
```bash
git clone https://huggingface.co/gaudi/opus-mt-en-kqn-ctranslate2
```
#### Take the python code below and update the 'model_dir' variable to the location of the cloned repository. ####
```python
from ctranslate2 import Translator
import transformers
model_dir = "./opus-mt-en-kqn-ctranslate2" # Path to model directory.
translator = Translator(
model_path=model_dir,
device="cuda", # cpu, cuda, or auto.
inter_threads=1, # Maximum number of parallel translations.
intra_threads=4, # Number of OpenMP threads per translator.
compute_type="int8_float16", # int8 for cpu or int8_float16 for cuda.
)
tokenizer = transformers.AutoTokenizer.from_pretrained(model_dir)
source = tokenizer.convert_ids_to_tokens(tokenizer.encode("XXXXXX, XXX XX XXXXXX."))
results = translator.translate_batch([source])
target = results[0].hypotheses[0]
print(tokenizer.decode(tokenizer.convert_tokens_to_ids(target)))
```
# Sample Code - hf-hub-ctranslate2
**Derived From [michaelfeil](https://huggingface.co/michaelfeil):**
```python
from hf_hub_ctranslate2 import TranslatorCT2fromHfHub, GeneratorCT2fromHfHub
from transformers import AutoTokenizer
model_name = "gaudi/opus-mt-en-kqn-ctranslate2"
model = TranslatorCT2fromHfHub(
model_name_or_path=model_name,
device="cuda",
compute_type="int8_float16",
tokenizer=AutoTokenizer.from_pretrained(model_name)
)
outputs = model.generate(
text=["XXX XX XXX XXXXXXX XXXX?", "XX XX XXXX XX XXX!"],
)
print(outputs)
```
# License and other remarks:
License conditions are intended to be idential to [original huggingface repository](https://huggingface.co/Helsinki-NLP/opus-mt-en-kqn) by Helsinki-NLP.
| [
"TRANSLATION"
] | Non_BioNLP |
sehunnnn/123 | sehunnnn | null | [
"instruction-tuning",
"en",
"arxiv:2304.08460",
"region:us"
] | 1,684,246,248,000 | 2023-05-19T14:10:11 | 0 | 0 | ---
language:
- en
tags:
- instruction-tuning
dataset_info:
features:
- name: input
dtype: string
- name: output
dtype: string
- name: source
dtype: string
- name: subset
dtype: string
splits:
- name: train
num_bytes: 63759065
num_examples: 23652
- name: validation
num_bytes: 6190242
num_examples: 2042
- name: test
num_bytes: 6080212
num_examples: 2045
download_size: 45525146
dataset_size: 76029519
task_categories:
- text2text-generation
- text-generation
- question-answering
- conversational
- summarization
- table-question-answering
pretty_name: longform
size_categories:
- 10K<n<100K
---
# LongForm
The LongForm dataset is created by leveraging English corpus
examples with augmented instructions. We select a
diverse set of human-written
documents from existing corpora such as C4 and
Wikipedia and generate instructions for the given
documents via LLMs. Then, we extend these examples with structured corpora examples such as Stack Exchange and WikiHow and task examples such as question answering, email writing, grammar error correction, story/poem generation, and text summarization.
## Distribution
The distribution of the LongForm dataset in terms of the source of examples is below. It contains examples generated from raw text corpora via LLMs, structured corpus examples, as well as various NLP task examples such as email writing, grammar error correction, story/poem generation, and text summarization.
| **Type** | **Source** | **Number of Examples** |
|------------------------|----------------|------------------------|
| **Corpora** | C4 | 10,000 |
| | Wikipedia | 5,000 |
| **Structured Corpora** | Stack Exchange | 4,380 |
| | WikiHow | 2,500 |
| **Tasks** | NIv2 | 3,684 |
| | Big Bench | 600 |
| | BEA-GEC | 1,203 |
| | Enron | 372 |
| **Total** | | 27,739 |
| | | |
| **Train** | | 23,652 |
| **Validation** | | 2,042 |
| **Test** | | 2,045 |
## Models
| | **All** | **Recipe Generation** | **ELI5** | **Writing Prompts** |
|-----------------------|---------|-----------------------------------|----------|---------------------|
| **T0++** | 10.9 | 18.7 | 3.8 | 10.2 |
| **Tk-Instruct** | 6.3 | 12.9* | 3.6 | 2.4 |
| **Flan-T5** | 10.6 | 20.9* | 3.5 | 7.4 |
| **Alpaca-LLaMA-7B** | 14.6 | 19.5 | 12.5 | 11.8 |
| **OPT-30B** | 11.1 | 18.6 | 12.2 | 2.6 |
| **[LongForm-T5-XL](https://huggingface.co/akoksal/LongForm-T5-XL)** | 16.3 | 20.2 | 18.3 | 10.6 |
| **[LongForm-OPT-2.7B](https://huggingface.co/akoksal/LongForm-OPT-2.7B)** | 17.8 | 15.5 | 17.9 | **19.9** |
| **[LongForm-OPT-6.7B](https://huggingface.co/akoksal/LongForm-OPT-6.7B)** | 17.7 | 16.9 | 17.2 | 19.0 |
| [**LongForm-LLaMA-7B**](https://huggingface.co/akoksal/LongForm-LLaMA-7B-diff)‡ | **19.7** | **21.7** | **18.6** | 18.9 |
‡: We can just release the difference between LongForm-LLaMA-7B and pretrained LLaMA-7B publicly due to restrictions of LLaMA models.
## Examples
| **Source** | **Instruction** | **Output**|
|------------------------|----------------|------------------------|
| C4 | What is the likelihood that Donald Trump's HHS secretary nominee, Alex Azar, will push policies that benefit pharmaceutical companies' pocketbooks? | His record suggests he’s unlikely to push pricing policies that would hit pharmaceutical companies’ pocketbooks.<br />President Donald Trump tweeted Monday that his nominee for HHS secretary, Alex Azar will “be a star for … lower drug prices!” But the record of the former top executive for Eli Lilly, which tripled the price of a top-selling insulin drug while he led its U.S. operation, suggests a different story.<br />Lilly is one of three drug companies targeted by a class-action lawsuit that accuses the company[...]<br />“This nominee has impeccable big pharma credentials but non-existent credentials when it comes to concerns on drug prices,” said Peter Welch, co-chair of the House Democrats Drug Pricing Task Force.
|Wikipedia | "Where is San Saba located in Rome, Italy?" Respond in 2 sentences. |San Saba is an ancient basilica church in Rome, Italy. It lies on the so-called "Piccolo Aventino", which is an area close to the ancient Aurelian Walls next to the Aventine Hill and Caelian Hill.|
## Dataset Description
- **Repository:** https://github.com/akoksal/LongForm
- **Paper:** https://arxiv.org/abs/2304.08460
- **Version:** v1.0 - April 18, 2023
- **Contact:** [Abdullatif Köksal](https://twitter.com/akoksal_)
## License
The LongForm project is subject to a MIT License with custom limitations for restrictions imposed by OpenAI (for the instruction generation part), as well as the license of language models (OPT, LLaMA, and T5).
## Citation
```
@misc{koksal2023longform,
title={LongForm: Optimizing Instruction Tuning for Long Text Generation with Corpus Extraction},
author={Abdullatif Köksal and Timo Schick and Anna Korhonen and Hinrich Schütze},
year={2023},
eprint={2304.08460},
archivePrefix={arXiv},
primaryClass={cs.CL}
}
```
| [
"QUESTION_ANSWERING",
"SUMMARIZATION"
] | Non_BioNLP |
context-mt/scat-marian-small-ctx4-cwd1-en-fr | context-mt | translation | [
"transformers",
"pytorch",
"tensorboard",
"marian",
"text2text-generation",
"arxiv:2310.01188",
"contextual-mt",
"document-mt",
"translation",
"en",
"fr",
"dataset:inseq/scat",
"dataset:gsarti/iwslt2017_context",
"license:apache-2.0",
"autotrain_compatible",
"endpoints_compatible",
"region:us"
] | 1,686,745,093,000 | 2024-03-10T12:42:19 | 28 | 0 | ---
datasets:
- inseq/scat
- gsarti/iwslt2017_context
language:
- en
- fr
license: apache-2.0
pipeline_tag: translation
tags:
- arxiv:2310.01188
- contextual-mt
- document-mt
---
*This model corresponds to the [`Helsinki-NLP/opus-mt-en-fr`](https://huggingface.co/Helsinki-NLP/opus-mt-en-fr) further trained on English-to-French translation on the [IWSLT17 dataset](https://huggingface.co/datasets/gsarti/iwslt2017_context) with context tags using the format:
```
Input: SOURCE_CTX <brk> SOURCE_CURR
Output: TARGET_CURR
```
and further fine-tuned on the training split of [SCAT+](https://huggingface.co/datasets/inseq/scat). The model was used in the evaluation of the paper [Quantifying the Plausibility of Context Reliance in Neural Machine Translation](https://openreview.net/forum?id=XTHfNGI3zT) published at ICLR 2024, also available on [Arxiv](https://arxiv.org/abs/2310.01188). It can be used for English to French contextual and non-contextual translation. | [
"TRANSLATION"
] | Non_BioNLP |
jayakody2000lk/flan-t5-small-Q4_K_M-GGUF | jayakody2000lk | text2text-generation | [
"gguf",
"text2text-generation",
"llama-cpp",
"gguf-my-repo",
"en",
"fr",
"ro",
"de",
"multilingual",
"dataset:svakulenk0/qrecc",
"dataset:taskmaster2",
"dataset:djaym7/wiki_dialog",
"dataset:deepmind/code_contests",
"dataset:lambada",
"dataset:gsm8k",
"dataset:aqua_rat",
"dataset:esnli",
"dataset:quasc",
"dataset:qed",
"base_model:google/flan-t5-small",
"base_model:quantized:google/flan-t5-small",
"license:apache-2.0",
"endpoints_compatible",
"region:us"
] | 1,732,160,452,000 | 2024-11-21T03:40:54 | 3 | 0 | ---
base_model: google/flan-t5-small
datasets:
- svakulenk0/qrecc
- taskmaster2
- djaym7/wiki_dialog
- deepmind/code_contests
- lambada
- gsm8k
- aqua_rat
- esnli
- quasc
- qed
language:
- en
- fr
- ro
- de
- multilingual
license: apache-2.0
tags:
- text2text-generation
- llama-cpp
- gguf-my-repo
widget:
- text: 'Translate to German: My name is Arthur'
example_title: Translation
- text: Please answer to the following question. Who is going to be the next Ballon
d'or?
example_title: Question Answering
- text: 'Q: Can Geoffrey Hinton have a conversation with George Washington? Give the
rationale before answering.'
example_title: Logical reasoning
- text: Please answer the following question. What is the boiling point of Nitrogen?
example_title: Scientific knowledge
- text: Answer the following yes/no question. Can you write a whole Haiku in a single
tweet?
example_title: Yes/no question
- text: Answer the following yes/no question by reasoning step-by-step. Can you write
a whole Haiku in a single tweet?
example_title: Reasoning task
- text: 'Q: ( False or not False or False ) is? A: Let''s think step by step'
example_title: Boolean Expressions
- text: The square root of x is the cube root of y. What is y to the power of 2, if
x = 4?
example_title: Math reasoning
- text: 'Premise: At my age you will probably have learnt one lesson. Hypothesis: It''s
not certain how many lessons you''ll learn by your thirties. Does the premise
entail the hypothesis?'
example_title: Premise and hypothesis
---
# jayakody2000lk/flan-t5-small-Q4_K_M-GGUF
This model was converted to GGUF format from [`google/flan-t5-small`](https://huggingface.co/google/flan-t5-small) using llama.cpp via the ggml.ai's [GGUF-my-repo](https://huggingface.co/spaces/ggml-org/gguf-my-repo) space.
Refer to the [original model card](https://huggingface.co/google/flan-t5-small) for more details on the model.
## Use with llama.cpp
Install llama.cpp through brew (works on Mac and Linux)
```bash
brew install llama.cpp
```
Invoke the llama.cpp server or the CLI.
### CLI:
```bash
llama-cli --hf-repo jayakody2000lk/flan-t5-small-Q4_K_M-GGUF --hf-file flan-t5-small-q4_k_m.gguf -p "The meaning to life and the universe is"
```
### Server:
```bash
llama-server --hf-repo jayakody2000lk/flan-t5-small-Q4_K_M-GGUF --hf-file flan-t5-small-q4_k_m.gguf -c 2048
```
Note: You can also use this checkpoint directly through the [usage steps](https://github.com/ggerganov/llama.cpp?tab=readme-ov-file#usage) listed in the Llama.cpp repo as well.
Step 1: Clone llama.cpp from GitHub.
```
git clone https://github.com/ggerganov/llama.cpp
```
Step 2: Move into the llama.cpp folder and build it with `LLAMA_CURL=1` flag along with other hardware-specific flags (for ex: LLAMA_CUDA=1 for Nvidia GPUs on Linux).
```
cd llama.cpp && LLAMA_CURL=1 make
```
Step 3: Run inference through the main binary.
```
./llama-cli --hf-repo jayakody2000lk/flan-t5-small-Q4_K_M-GGUF --hf-file flan-t5-small-q4_k_m.gguf -p "The meaning to life and the universe is"
```
or
```
./llama-server --hf-repo jayakody2000lk/flan-t5-small-Q4_K_M-GGUF --hf-file flan-t5-small-q4_k_m.gguf -c 2048
```
| [
"QUESTION_ANSWERING",
"TRANSLATION"
] | Non_BioNLP |
RichardErkhov/pt-sk_-_ll-3.2-1B_Instruct-gguf | RichardErkhov | null | [
"gguf",
"arxiv:2204.05149",
"arxiv:2405.16406",
"endpoints_compatible",
"region:us",
"conversational"
] | 1,739,442,686,000 | 2025-02-13T11:48:12 | 22 | 0 | ---
{}
---
Quantization made by Richard Erkhov.
[Github](https://github.com/RichardErkhov)
[Discord](https://discord.gg/pvy7H8DZMG)
[Request more models](https://github.com/RichardErkhov/quant_request)
ll-3.2-1B_Instruct - GGUF
- Model creator: https://huggingface.co/pt-sk/
- Original model: https://huggingface.co/pt-sk/ll-3.2-1B_Instruct/
| Name | Quant method | Size |
| ---- | ---- | ---- |
| [ll-3.2-1B_Instruct.Q2_K.gguf](https://huggingface.co/RichardErkhov/pt-sk_-_ll-3.2-1B_Instruct-gguf/blob/main/ll-3.2-1B_Instruct.Q2_K.gguf) | Q2_K | 0.54GB |
| [ll-3.2-1B_Instruct.IQ3_XS.gguf](https://huggingface.co/RichardErkhov/pt-sk_-_ll-3.2-1B_Instruct-gguf/blob/main/ll-3.2-1B_Instruct.IQ3_XS.gguf) | IQ3_XS | 0.58GB |
| [ll-3.2-1B_Instruct.IQ3_S.gguf](https://huggingface.co/RichardErkhov/pt-sk_-_ll-3.2-1B_Instruct-gguf/blob/main/ll-3.2-1B_Instruct.IQ3_S.gguf) | IQ3_S | 0.6GB |
| [ll-3.2-1B_Instruct.Q3_K_S.gguf](https://huggingface.co/RichardErkhov/pt-sk_-_ll-3.2-1B_Instruct-gguf/blob/main/ll-3.2-1B_Instruct.Q3_K_S.gguf) | Q3_K_S | 0.6GB |
| [ll-3.2-1B_Instruct.IQ3_M.gguf](https://huggingface.co/RichardErkhov/pt-sk_-_ll-3.2-1B_Instruct-gguf/blob/main/ll-3.2-1B_Instruct.IQ3_M.gguf) | IQ3_M | 0.61GB |
| [ll-3.2-1B_Instruct.Q3_K.gguf](https://huggingface.co/RichardErkhov/pt-sk_-_ll-3.2-1B_Instruct-gguf/blob/main/ll-3.2-1B_Instruct.Q3_K.gguf) | Q3_K | 0.64GB |
| [ll-3.2-1B_Instruct.Q3_K_M.gguf](https://huggingface.co/RichardErkhov/pt-sk_-_ll-3.2-1B_Instruct-gguf/blob/main/ll-3.2-1B_Instruct.Q3_K_M.gguf) | Q3_K_M | 0.64GB |
| [ll-3.2-1B_Instruct.Q3_K_L.gguf](https://huggingface.co/RichardErkhov/pt-sk_-_ll-3.2-1B_Instruct-gguf/blob/main/ll-3.2-1B_Instruct.Q3_K_L.gguf) | Q3_K_L | 0.68GB |
| [ll-3.2-1B_Instruct.IQ4_XS.gguf](https://huggingface.co/RichardErkhov/pt-sk_-_ll-3.2-1B_Instruct-gguf/blob/main/ll-3.2-1B_Instruct.IQ4_XS.gguf) | IQ4_XS | 0.7GB |
| [ll-3.2-1B_Instruct.Q4_0.gguf](https://huggingface.co/RichardErkhov/pt-sk_-_ll-3.2-1B_Instruct-gguf/blob/main/ll-3.2-1B_Instruct.Q4_0.gguf) | Q4_0 | 0.72GB |
| [ll-3.2-1B_Instruct.IQ4_NL.gguf](https://huggingface.co/RichardErkhov/pt-sk_-_ll-3.2-1B_Instruct-gguf/blob/main/ll-3.2-1B_Instruct.IQ4_NL.gguf) | IQ4_NL | 0.72GB |
| [ll-3.2-1B_Instruct.Q4_K_S.gguf](https://huggingface.co/RichardErkhov/pt-sk_-_ll-3.2-1B_Instruct-gguf/blob/main/ll-3.2-1B_Instruct.Q4_K_S.gguf) | Q4_K_S | 0.72GB |
| [ll-3.2-1B_Instruct.Q4_K.gguf](https://huggingface.co/RichardErkhov/pt-sk_-_ll-3.2-1B_Instruct-gguf/blob/main/ll-3.2-1B_Instruct.Q4_K.gguf) | Q4_K | 0.75GB |
| [ll-3.2-1B_Instruct.Q4_K_M.gguf](https://huggingface.co/RichardErkhov/pt-sk_-_ll-3.2-1B_Instruct-gguf/blob/main/ll-3.2-1B_Instruct.Q4_K_M.gguf) | Q4_K_M | 0.75GB |
| [ll-3.2-1B_Instruct.Q4_1.gguf](https://huggingface.co/RichardErkhov/pt-sk_-_ll-3.2-1B_Instruct-gguf/blob/main/ll-3.2-1B_Instruct.Q4_1.gguf) | Q4_1 | 0.77GB |
| [ll-3.2-1B_Instruct.Q5_0.gguf](https://huggingface.co/RichardErkhov/pt-sk_-_ll-3.2-1B_Instruct-gguf/blob/main/ll-3.2-1B_Instruct.Q5_0.gguf) | Q5_0 | 0.83GB |
| [ll-3.2-1B_Instruct.Q5_K_S.gguf](https://huggingface.co/RichardErkhov/pt-sk_-_ll-3.2-1B_Instruct-gguf/blob/main/ll-3.2-1B_Instruct.Q5_K_S.gguf) | Q5_K_S | 0.83GB |
| [ll-3.2-1B_Instruct.Q5_K.gguf](https://huggingface.co/RichardErkhov/pt-sk_-_ll-3.2-1B_Instruct-gguf/blob/main/ll-3.2-1B_Instruct.Q5_K.gguf) | Q5_K | 0.85GB |
| [ll-3.2-1B_Instruct.Q5_K_M.gguf](https://huggingface.co/RichardErkhov/pt-sk_-_ll-3.2-1B_Instruct-gguf/blob/main/ll-3.2-1B_Instruct.Q5_K_M.gguf) | Q5_K_M | 0.85GB |
| [ll-3.2-1B_Instruct.Q5_1.gguf](https://huggingface.co/RichardErkhov/pt-sk_-_ll-3.2-1B_Instruct-gguf/blob/main/ll-3.2-1B_Instruct.Q5_1.gguf) | Q5_1 | 0.89GB |
| [ll-3.2-1B_Instruct.Q6_K.gguf](https://huggingface.co/RichardErkhov/pt-sk_-_ll-3.2-1B_Instruct-gguf/blob/main/ll-3.2-1B_Instruct.Q6_K.gguf) | Q6_K | 0.95GB |
| [ll-3.2-1B_Instruct.Q8_0.gguf](https://huggingface.co/RichardErkhov/pt-sk_-_ll-3.2-1B_Instruct-gguf/blob/main/ll-3.2-1B_Instruct.Q8_0.gguf) | Q8_0 | 1.23GB |
Original model description:
---
language:
- en
- de
- fr
- it
- pt
- hi
- es
- th
library_name: transformers
pipeline_tag: text-generation
tags:
- facebook
- meta
- pytorch
- llama
- llama-3
license: llama3.2
extra_gated_prompt: >-
### LLAMA 3.2 COMMUNITY LICENSE AGREEMENT
Llama 3.2 Version Release Date: September 25, 2024
“Agreement” means the terms and conditions for use, reproduction, distribution
and modification of the Llama Materials set forth herein.
“Documentation” means the specifications, manuals and documentation accompanying Llama 3.2
distributed by Meta at https://llama.meta.com/doc/overview.
“Licensee” or “you” means you, or your employer or any other person or entity (if you are
entering into this Agreement on such person or entity’s behalf), of the age required under
applicable laws, rules or regulations to provide legal consent and that has legal authority
to bind your employer or such other person or entity if you are entering in this Agreement
on their behalf.
“Llama 3.2” means the foundational large language models and software and algorithms, including
machine-learning model code, trained model weights, inference-enabling code, training-enabling code,
fine-tuning enabling code and other elements of the foregoing distributed by Meta at
https://www.llama.com/llama-downloads.
“Llama Materials” means, collectively, Meta’s proprietary Llama 3.2 and Documentation (and
any portion thereof) made available under this Agreement.
“Meta” or “we” means Meta Platforms Ireland Limited (if you are located in or,
if you are an entity, your principal place of business is in the EEA or Switzerland)
and Meta Platforms, Inc. (if you are located outside of the EEA or Switzerland).
By clicking “I Accept” below or by using or distributing any portion or element of the Llama Materials,
you agree to be bound by this Agreement.
1. License Rights and Redistribution.
a. Grant of Rights. You are granted a non-exclusive, worldwide,
non-transferable and royalty-free limited license under Meta’s intellectual property or other rights
owned by Meta embodied in the Llama Materials to use, reproduce, distribute, copy, create derivative works
of, and make modifications to the Llama Materials.
b. Redistribution and Use.
i. If you distribute or make available the Llama Materials (or any derivative works thereof),
or a product or service (including another AI model) that contains any of them, you shall (A) provide
a copy of this Agreement with any such Llama Materials; and (B) prominently display “Built with Llama”
on a related website, user interface, blogpost, about page, or product documentation. If you use the
Llama Materials or any outputs or results of the Llama Materials to create, train, fine tune, or
otherwise improve an AI model, which is distributed or made available, you shall also include “Llama”
at the beginning of any such AI model name.
ii. If you receive Llama Materials, or any derivative works thereof, from a Licensee as part
of an integrated end user product, then Section 2 of this Agreement will not apply to you.
iii. You must retain in all copies of the Llama Materials that you distribute the
following attribution notice within a “Notice” text file distributed as a part of such copies:
“Llama 3.2 is licensed under the Llama 3.2 Community License, Copyright © Meta Platforms,
Inc. All Rights Reserved.”
iv. Your use of the Llama Materials must comply with applicable laws and regulations
(including trade compliance laws and regulations) and adhere to the Acceptable Use Policy for
the Llama Materials (available at https://www.llama.com/llama3_2/use-policy), which is hereby
incorporated by reference into this Agreement.
2. Additional Commercial Terms. If, on the Llama 3.2 version release date, the monthly active users
of the products or services made available by or for Licensee, or Licensee’s affiliates,
is greater than 700 million monthly active users in the preceding calendar month, you must request
a license from Meta, which Meta may grant to you in its sole discretion, and you are not authorized to
exercise any of the rights under this Agreement unless or until Meta otherwise expressly grants you such rights.
3. Disclaimer of Warranty. UNLESS REQUIRED BY APPLICABLE LAW, THE LLAMA MATERIALS AND ANY OUTPUT AND
RESULTS THEREFROM ARE PROVIDED ON AN “AS IS” BASIS, WITHOUT WARRANTIES OF ANY KIND, AND META DISCLAIMS
ALL WARRANTIES OF ANY KIND, BOTH EXPRESS AND IMPLIED, INCLUDING, WITHOUT LIMITATION, ANY WARRANTIES
OF TITLE, NON-INFRINGEMENT, MERCHANTABILITY, OR FITNESS FOR A PARTICULAR PURPOSE. YOU ARE SOLELY RESPONSIBLE
FOR DETERMINING THE APPROPRIATENESS OF USING OR REDISTRIBUTING THE LLAMA MATERIALS AND ASSUME ANY RISKS ASSOCIATED
WITH YOUR USE OF THE LLAMA MATERIALS AND ANY OUTPUT AND RESULTS.
4. Limitation of Liability. IN NO EVENT WILL META OR ITS AFFILIATES BE LIABLE UNDER ANY THEORY OF LIABILITY,
WHETHER IN CONTRACT, TORT, NEGLIGENCE, PRODUCTS LIABILITY, OR OTHERWISE, ARISING OUT OF THIS AGREEMENT,
FOR ANY LOST PROFITS OR ANY INDIRECT, SPECIAL, CONSEQUENTIAL, INCIDENTAL, EXEMPLARY OR PUNITIVE DAMAGES, EVEN
IF META OR ITS AFFILIATES HAVE BEEN ADVISED OF THE POSSIBILITY OF ANY OF THE FOREGOING.
5. Intellectual Property.
a. No trademark licenses are granted under this Agreement, and in connection with the Llama Materials,
neither Meta nor Licensee may use any name or mark owned by or associated with the other or any of its affiliates,
except as required for reasonable and customary use in describing and redistributing the Llama Materials or as
set forth in this Section 5(a). Meta hereby grants you a license to use “Llama” (the “Mark”) solely as required
to comply with the last sentence of Section 1.b.i. You will comply with Meta’s brand guidelines (currently accessible
at https://about.meta.com/brand/resources/meta/company-brand/). All goodwill arising out of your use of the Mark
will inure to the benefit of Meta.
b. Subject to Meta’s ownership of Llama Materials and derivatives made by or for Meta, with respect to any
derivative works and modifications of the Llama Materials that are made by you, as between you and Meta,
you are and will be the owner of such derivative works and modifications.
c. If you institute litigation or other proceedings against Meta or any entity (including a cross-claim or
counterclaim in a lawsuit) alleging that the Llama Materials or Llama 3.2 outputs or results, or any portion
of any of the foregoing, constitutes infringement of intellectual property or other rights owned or licensable
by you, then any licenses granted to you under this Agreement shall terminate as of the date such litigation or
claim is filed or instituted. You will indemnify and hold harmless Meta from and against any claim by any third
party arising out of or related to your use or distribution of the Llama Materials.
6. Term and Termination. The term of this Agreement will commence upon your acceptance of this Agreement or access
to the Llama Materials and will continue in full force and effect until terminated in accordance with the terms
and conditions herein. Meta may terminate this Agreement if you are in breach of any term or condition of this
Agreement. Upon termination of this Agreement, you shall delete and cease use of the Llama Materials. Sections 3,
4 and 7 shall survive the termination of this Agreement.
7. Governing Law and Jurisdiction. This Agreement will be governed and construed under the laws of the State of
California without regard to choice of law principles, and the UN Convention on Contracts for the International
Sale of Goods does not apply to this Agreement. The courts of California shall have exclusive jurisdiction of
any dispute arising out of this Agreement.
### Llama 3.2 Acceptable Use Policy
Meta is committed to promoting safe and fair use of its tools and features, including Llama 3.2.
If you access or use Llama 3.2, you agree to this Acceptable Use Policy (“**Policy**”).
The most recent copy of this policy can be found at
[https://www.llama.com/llama3_2/use-policy](https://www.llama.com/llama3_2/use-policy).
#### Prohibited Uses
We want everyone to use Llama 3.2 safely and responsibly. You agree you will not use, or allow others to use, Llama 3.2 to:
1. Violate the law or others’ rights, including to:
1. Engage in, promote, generate, contribute to, encourage, plan, incite, or further illegal or unlawful activity or content, such as:
1. Violence or terrorism
2. Exploitation or harm to children, including the solicitation, creation, acquisition, or dissemination of child exploitative content or failure to report Child Sexual Abuse Material
3. Human trafficking, exploitation, and sexual violence
4. The illegal distribution of information or materials to minors, including obscene materials, or failure to employ legally required age-gating in connection with such information or materials.
5. Sexual solicitation
6. Any other criminal activity
1. Engage in, promote, incite, or facilitate the harassment, abuse, threatening, or bullying of individuals or groups of individuals
2. Engage in, promote, incite, or facilitate discrimination or other unlawful or harmful conduct in the provision of employment, employment benefits, credit, housing, other economic benefits, or other essential goods and services
3. Engage in the unauthorized or unlicensed practice of any profession including, but not limited to, financial, legal, medical/health, or related professional practices
4. Collect, process, disclose, generate, or infer private or sensitive information about individuals, including information about individuals’ identity, health, or demographic information, unless you have obtained the right to do so in accordance with applicable law
5. Engage in or facilitate any action or generate any content that infringes, misappropriates, or otherwise violates any third-party rights, including the outputs or results of any products or services using the Llama Materials
6. Create, generate, or facilitate the creation of malicious code, malware, computer viruses or do anything else that could disable, overburden, interfere with or impair the proper working, integrity, operation or appearance of a website or computer system
7. Engage in any action, or facilitate any action, to intentionally circumvent or remove usage restrictions or other safety measures, or to enable functionality disabled by Meta
2. Engage in, promote, incite, facilitate, or assist in the planning or development of activities that present a risk of death or bodily harm to individuals, including use of Llama 3.2 related to the following:
8. Military, warfare, nuclear industries or applications, espionage, use for materials or activities that are subject to the International Traffic Arms Regulations (ITAR) maintained by the United States Department of State or to the U.S. Biological Weapons Anti-Terrorism Act of 1989 or the Chemical Weapons Convention Implementation Act of 1997
9. Guns and illegal weapons (including weapon development)
10. Illegal drugs and regulated/controlled substances
11. Operation of critical infrastructure, transportation technologies, or heavy machinery
12. Self-harm or harm to others, including suicide, cutting, and eating disorders
13. Any content intended to incite or promote violence, abuse, or any infliction of bodily harm to an individual
3. Intentionally deceive or mislead others, including use of Llama 3.2 related to the following:
14. Generating, promoting, or furthering fraud or the creation or promotion of disinformation
15. Generating, promoting, or furthering defamatory content, including the creation of defamatory statements, images, or other content
16. Generating, promoting, or further distributing spam
17. Impersonating another individual without consent, authorization, or legal right
18. Representing that the use of Llama 3.2 or outputs are human-generated
19. Generating or facilitating false online engagement, including fake reviews and other means of fake online engagement
4. Fail to appropriately disclose to end users any known dangers of your AI system
5. Interact with third party tools, models, or software designed to generate unlawful content or engage in unlawful or harmful conduct and/or represent that the outputs of such tools, models, or software are associated with Meta or Llama 3.2
With respect to any multimodal models included in Llama 3.2, the rights granted under Section 1(a) of the Llama 3.2 Community License Agreement are not being granted to you if you are an individual domiciled in, or a company with a principal place of business in, the European Union. This restriction does not apply to end users of a product or service that incorporates any such multimodal models.
Please report any violation of this Policy, software “bug,” or other problems that could lead to a violation of this Policy through one of the following means:
* Reporting issues with the model: [https://github.com/meta-llama/llama-models/issues](https://l.workplace.com/l.php?u=https%3A%2F%2Fgithub.com%2Fmeta-llama%2Fllama-models%2Fissues&h=AT0qV8W9BFT6NwihiOHRuKYQM_UnkzN_NmHMy91OT55gkLpgi4kQupHUl0ssR4dQsIQ8n3tfd0vtkobvsEvt1l4Ic6GXI2EeuHV8N08OG2WnbAmm0FL4ObkazC6G_256vN0lN9DsykCvCqGZ)
* Reporting risky content generated by the model: [developers.facebook.com/llama_output_feedback](http://developers.facebook.com/llama_output_feedback)
* Reporting bugs and security concerns: [facebook.com/whitehat/info](http://facebook.com/whitehat/info)
* Reporting violations of the Acceptable Use Policy or unlicensed uses of Llama 3.2: [email protected]
extra_gated_fields:
First Name: text
Last Name: text
Date of birth: date_picker
Country: country
Affiliation: text
Job title:
type: select
options:
- Student
- Research Graduate
- AI researcher
- AI developer/engineer
- Reporter
- Other
geo: ip_location
By clicking Submit below I accept the terms of the license and acknowledge that the information I provide will be collected stored processed and shared in accordance with the Meta Privacy Policy: checkbox
extra_gated_description: >-
The information you provide will be collected, stored, processed and shared in
accordance with the [Meta Privacy
Policy](https://www.facebook.com/privacy/policy/).
extra_gated_button_content: Submit
---
## Model Information
The Llama 3.2 collection of multilingual large language models (LLMs) is a collection of pretrained and instruction-tuned generative models in 1B and 3B sizes (text in/text out). The Llama 3.2 instruction-tuned text only models are optimized for multilingual dialogue use cases, including agentic retrieval and summarization tasks. They outperform many of the available open source and closed chat models on common industry benchmarks.
**Model Developer:** Meta
**Model Architecture:** Llama 3.2 is an auto-regressive language model that uses an optimized transformer architecture. The tuned versions use supervised fine-tuning (SFT) and reinforcement learning with human feedback (RLHF) to align with human preferences for helpfulness and safety.
| | Training Data | Params | Input modalities | Output modalities | Context Length | GQA | Shared Embeddings | Token count | Knowledge cutoff |
| :---- | :---- | :---- | :---- | :---- | :---- | :---- | :---- | :---- | :---- |
| Llama 3.2 (text only) | A new mix of publicly available online data. | 1B (1.23B) | Multilingual Text | Multilingual Text and code | 128k | Yes | Yes | Up to 9T tokens | December 2023 |
| | | 3B (3.21B) | Multilingual Text | Multilingual Text and code | | | | | |
| Llama 3.2 Quantized (text only) | A new mix of publicly available online data. | 1B (1.23B) | Multilingual Text | Multilingual Text and code | 8k | Yes | Yes | Up to 9T tokens | December 2023 |
| | | 3B (3.21B) | Multilingual Text | Multilingual Text and code | | | | | |
**Supported Languages:** English, German, French, Italian, Portuguese, Hindi, Spanish, and Thai are officially supported. Llama 3.2 has been trained on a broader collection of languages than these 8 supported languages. Developers may fine-tune Llama 3.2 models for languages beyond these supported languages, provided they comply with the Llama 3.2 Community License and the Acceptable Use Policy. Developers are always expected to ensure that their deployments, including those that involve additional languages, are completed safely and responsibly.
**Llama 3.2 Model Family:** Token counts refer to pretraining data only. All model versions use Grouped-Query Attention (GQA) for improved inference scalability.
**Model Release Date:** Sept 25, 2024
**Status:** This is a static model trained on an offline dataset. Future versions may be released that improve model capabilities and safety.
**License:** Use of Llama 3.2 is governed by the [Llama 3.2 Community License](https://github.com/meta-llama/llama-models/blob/main/models/llama3_2/LICENSE) (a custom, commercial license agreement).
**Feedback:** Instructions on how to provide feedback or comments on the model can be found in the Llama Models [README](https://github.com/meta-llama/llama-models/blob/main/README.md). For more technical information about generation parameters and recipes for how to use Llama 3.2 in applications, please go [here](https://github.com/meta-llama/llama-recipes).
## Intended Use
**Intended Use Cases:** Llama 3.2 is intended for commercial and research use in multiple languages. Instruction tuned text only models are intended for assistant-like chat and agentic applications like knowledge retrieval and summarization, mobile AI powered writing assistants and query and prompt rewriting. Pretrained models can be adapted for a variety of additional natural language generation tasks. Similarly, quantized models can be adapted for a variety of on-device use-cases with limited compute resources.
**Out of Scope:** Use in any manner that violates applicable laws or regulations (including trade compliance laws). Use in any other way that is prohibited by the Acceptable Use Policy and Llama 3.2 Community License. Use in languages beyond those explicitly referenced as supported in this model card.
## How to use
This repository contains two versions of Llama-3.2-1B-Instruct, for use with transformers and with the original `llama` codebase.
### Use with transformers
Starting with `transformers >= 4.43.0` onward, you can run conversational inference using the Transformers `pipeline` abstraction or by leveraging the Auto classes with the `generate()` function.
Make sure to update your transformers installation via `pip install --upgrade transformers`.
```python
import torch
from transformers import pipeline
model_id = "meta-llama/Llama-3.2-1B-Instruct"
pipe = pipeline(
"text-generation",
model=model_id,
torch_dtype=torch.bfloat16,
device_map="auto",
)
messages = [
{"role": "system", "content": "You are a pirate chatbot who always responds in pirate speak!"},
{"role": "user", "content": "Who are you?"},
]
outputs = pipe(
messages,
max_new_tokens=256,
)
print(outputs[0]["generated_text"][-1])
```
Note: You can also find detailed recipes on how to use the model locally, with `torch.compile()`, assisted generations, quantised and more at [`huggingface-llama-recipes`](https://github.com/huggingface/huggingface-llama-recipes)
### Use with `llama`
Please, follow the instructions in the [repository](https://github.com/meta-llama/llama)
To download Original checkpoints, see the example command below leveraging `huggingface-cli`:
```
huggingface-cli download meta-llama/Llama-3.2-1B-Instruct --include "original/*" --local-dir Llama-3.2-1B-Instruct
```
## Hardware and Software
**Training Factors:** We used custom training libraries, Meta's custom built GPU cluster, and production infrastructure for pretraining. Fine-tuning, quantization, annotation, and evaluation were also performed on production infrastructure.
**Training Energy Use:** Training utilized a cumulative of **916k** GPU hours of computation on H100-80GB (TDP of 700W) type hardware, per the table below. Training time is the total GPU time required for training each model and power consumption is the peak power capacity per GPU device used, adjusted for power usage efficiency.
**Training Greenhouse Gas Emissions:** Estimated total location-based greenhouse gas emissions were **240** tons CO2eq for training. Since 2020, Meta has maintained net zero greenhouse gas emissions in its global operations and matched 100% of its electricity use with renewable energy; therefore, the total market-based greenhouse gas emissions for training were 0 tons CO2eq.
| | Training Time (GPU hours) | Logit Generation Time (GPU Hours) | Training Power Consumption (W) | Training Location-Based Greenhouse Gas Emissions (tons CO2eq) | Training Market-Based Greenhouse Gas Emissions (tons CO2eq) |
| :---- | :---: | ----- | :---: | :---: | :---: |
| Llama 3.2 1B | 370k | \- | 700 | 107 | 0 |
| Llama 3.2 3B | 460k | \- | 700 | 133 | 0 |
| Llama 3.2 1B SpinQuant | 1.7 | 0 | 700 | *Negligible*\*\* | 0 |
| Llama 3.2 3B SpinQuant | 2.4 | 0 | 700 | *Negligible*\*\* | 0 |
| Llama 3.2 1B QLora | 1.3k | 0 | 700 | 0.381 | 0 |
| Llama 3.2 3B QLora | 1.6k | 0 | 700 | 0.461 | 0 |
| Total | 833k | 86k | | 240 | 0 |
\*\* The location-based CO2e emissions of Llama 3.2 1B SpinQuant and Llama 3.2 3B SpinQuant are less than 0.001 metric tonnes each. This is due to the minimal training GPU hours that are required.
The methodology used to determine training energy use and greenhouse gas emissions can be found [here](https://arxiv.org/pdf/2204.05149). Since Meta is openly releasing these models, the training energy use and greenhouse gas emissions will not be incurred by others.
## Training Data
**Overview:** Llama 3.2 was pretrained on up to 9 trillion tokens of data from publicly available sources. For the 1B and 3B Llama 3.2 models, we incorporated logits from the Llama 3.1 8B and 70B models into the pretraining stage of the model development, where outputs (logits) from these larger models were used as token-level targets. Knowledge distillation was used after pruning to recover performance. In post-training we used a similar recipe as Llama 3.1 and produced final chat models by doing several rounds of alignment on top of the pre-trained model. Each round involved Supervised Fine-Tuning (SFT), Rejection Sampling (RS), and Direct Preference Optimization (DPO).
**Data Freshness:** The pretraining data has a cutoff of December 2023\.
## Quantization
### Quantization Scheme
We designed the current quantization scheme with the [PyTorch’s ExecuTorch](https://github.com/pytorch/executorch) inference framework and Arm CPU backend in mind, taking into account metrics including model quality, prefill/decoding speed, and memory footprint. Our quantization scheme involves three parts:
- All linear layers in all transformer blocks are quantized to a 4-bit groupwise scheme (with a group size of 32) for weights and 8-bit per-token dynamic quantization for activations.
- The classification layer is quantized to 8-bit per-channel for weight and 8-bit per token dynamic quantization for activation.
- Similar to classification layer, an 8-bit per channel quantization is used for embedding layer.
### Quantization-Aware Training and LoRA
The quantization-aware training (QAT) with low-rank adaptation (LoRA) models went through only post-training stages, using the same data as the full precision models. To initialize QAT, we utilize BF16 Llama 3.2 model checkpoints obtained after supervised fine-tuning (SFT) and perform an additional full round of SFT training with QAT. We then freeze the backbone of the QAT model and perform another round of SFT with LoRA adaptors applied to all layers within the transformer block. Meanwhile, the LoRA adaptors' weights and activations are maintained in BF16. Because our approach is similar to QLoRA of Dettmers et al., (2023) (i.e., quantization followed by LoRA adapters), we refer this method as QLoRA. Finally, we fine-tune the resulting model (both backbone and LoRA adaptors) using direct preference optimization (DPO).
### SpinQuant
[SpinQuant](https://arxiv.org/abs/2405.16406) was applied, together with generative post-training quantization (GPTQ). For the SpinQuant rotation matrix fine-tuning, we optimized for 100 iterations, using 800 samples with sequence-length 2048 from the WikiText 2 dataset. For GPTQ, we used 128 samples from the same dataset with the same sequence-length.
## Benchmarks \- English Text
In this section, we report the results for Llama 3.2 models on standard automatic benchmarks. For all these evaluations, we used our internal evaluations library.
### Base Pretrained Models
| Category | Benchmark | \# Shots | Metric | Llama 3.2 1B | Llama 3.2 3B | Llama 3.1 8B |
| ----- | ----- | :---: | :---: | :---: | :---: | :---: |
| General | MMLU | 5 | macro\_avg/acc\_char | 32.2 | 58 | 66.7 |
| | AGIEval English | 3-5 | average/acc\_char | 23.3 | 39.2 | 47.8 |
| | ARC-Challenge | 25 | acc\_char | 32.8 | 69.1 | 79.7 |
| Reading comprehension | SQuAD | 1 | em | 49.2 | 67.7 | 77 |
| | QuAC (F1) | 1 | f1 | 37.9 | 42.9 | 44.9 |
| | DROP (F1) | 3 | f1 | 28.0 | 45.2 | 59.5 |
| Long Context | Needle in Haystack | 0 | em | 96.8 | 1 | 1 |
### Instruction Tuned Models
| Capability | | Benchmark | \# Shots | Metric | Llama 3.2 1B bf16 | Llama 3.2 1B Vanilla PTQ\*\* | Llama 3.2 1B Spin Quant | Llama 3.2 1B QLoRA | Llama 3.2 3B bf16 | Llama 3.2 3B Vanilla PTQ\*\* | Llama 3.2 3B Spin Quant | Llama 3.2 3B QLoRA | Llama 3.1 8B |
| :---: | ----- | :---: | :---: | :---: | :---: | :---: | :---: | :---: | :---: | :---: | :---: | :---: | :---: |
| General | | MMLU | 5 | macro\_avg/acc | 49.3 | 43.3 | 47.3 | 49.0 | 63.4 | 60.5 | 62 | 62.4 | 69.4 |
| Re-writing | | Open-rewrite eval | 0 | micro\_avg/rougeL | 41.6 | 39.2 | 40.9 | 41.2 | 40.1 | 40.3 | 40.8 | 40.7 | 40.9 |
| Summarization | | TLDR9+ (test) | 1 | rougeL | 16.8 | 14.9 | 16.7 | 16.8 | 19.0 | 19.1 | 19.2 | 19.1 | 17.2 |
| Instruction following | | IFEval | 0 | Avg(Prompt/Instruction acc Loose/Strict) | 59.5 | 51.5 | 58.4 | 55.6 | 77.4 | 73.9 | 73.5 | 75.9 | 80.4 |
| Math | | GSM8K (CoT) | 8 | em\_maj1@1 | 44.4 | 33.1 | 40.6 | 46.5 | 77.7 | 72.9 | 75.7 | 77.9 | 84.5 |
| | | MATH (CoT) | 0 | final\_em | 30.6 | 20.5 | 25.3 | 31.0 | 48.0 | 44.2 | 45.3 | 49.2 | 51.9 |
| Reasoning | | ARC-C | 0 | acc | 59.4 | 54.3 | 57 | 60.7 | 78.6 | 75.6 | 77.6 | 77.6 | 83.4 |
| | | GPQA | 0 | acc | 27.2 | 25.9 | 26.3 | 25.9 | 32.8 | 32.8 | 31.7 | 33.9 | 32.8 |
| | | Hellaswag | 0 | acc | 41.2 | 38.1 | 41.3 | 41.5 | 69.8 | 66.3 | 68 | 66.3 | 78.7 |
| Tool Use | | BFCL V2 | 0 | acc | 25.7 | 14.3 | 15.9 | 23.7 | 67.0 | 53.4 | 60.1 | 63.5 | 67.1 |
| | | Nexus | 0 | macro\_avg/acc | 13.5 | 5.2 | 9.6 | 12.5 | 34.3 | 32.4 | 31.5 | 30.1 | 38.5 |
| Long Context | | InfiniteBench/En.QA | 0 | longbook\_qa/f1 | 20.3 | N/A | N/A | N/A | 19.8 | N/A | N/A | N/A | 27.3 |
| | | InfiniteBench/En.MC | 0 | longbook\_choice/acc | 38.0 | N/A | N/A | N/A | 63.3 | N/A | N/A | N/A | 72.2 |
| | | NIH/Multi-needle | 0 | recall | 75.0 | N/A | N/A | N/A | 84.7 | N/A | N/A | N/A | 98.8 |
| Multilingual | | MGSM (CoT) | 0 | em | 24.5 | 13.7 | 18.2 | 24.4 | 58.2 | 48.9 | 54.3 | 56.8 | 68.9 |
\*\*for comparison purposes only. Model not released.
### Multilingual Benchmarks
| Category | Benchmark | Language | Llama 3.2 1B | Llama 3.2 1B Vanilla PTQ\*\* | Llama 3.2 1B Spin Quant | Llama 3.2 1B QLoRA | Llama 3.2 3B | Llama 3.2 3B Vanilla PTQ\*\* | Llama 3.2 3B Spin Quant | Llama 3.2 3B QLoRA | Llama 3.1 8B |
| :---: | :---: | :---: | :---: | :---: | :---: | :---: | :---: | :---: | :---: | :---: | :---: |
| General | MMLU (5-shot, macro_avg/acc) | Portuguese | 39.8 | 34.9 | 38.9 | 40.2 | 54.5 | 50.9 | 53.3 | 53.4 | 62.1 |
| | | Spanish | 41.5 | 36.0 | 39.8 | 41.8 | 55.1 | 51.9 | 53.6 | 53.6 | 62.5 |
| | | Italian | 39.8 | 34.9 | 38.1 | 40.6 | 53.8 | 49.9 | 52.1 | 51.7 | 61.6 |
| | | German | 39.2 | 34.9 | 37.5 | 39.6 | 53.3 | 50.0 | 52.2 | 51.3 | 60.6 |
| | | French | 40.5 | 34.8 | 39.2 | 40.8 | 54.6 | 51.2 | 53.3 | 53.3 | 62.3 |
| | | Hindi | 33.5 | 30.0 | 32.1 | 34.0 | 43.3 | 40.4 | 42.0 | 42.1 | 50.9 |
| | | Thai | 34.7 | 31.2 | 32.4 | 34.9 | 44.5 | 41.3 | 44.0 | 42.2 | 50.3 |
\*\*for comparison purposes only. Model not released.
## Inference time
In the below table, we compare the performance metrics of different quantization methods (SpinQuant and QAT \+ LoRA) with the BF16 baseline. The evaluation was done using the [ExecuTorch](https://github.com/pytorch/executorch) framework as the inference engine, with the ARM CPU as a backend using Android OnePlus 12 device.
| Category | Decode (tokens/sec) | Time-to-first-token (sec) | Prefill (tokens/sec) | Model size (PTE file size in MB) | Memory size (RSS in MB) |
| :---- | ----- | ----- | ----- | ----- | ----- |
| 1B BF16 (baseline) | 19.2 | 1.0 | 60.3 | 2358 | 3,185 |
| 1B SpinQuant | 50.2 (2.6x) | 0.3 (-76.9%) | 260.5 (4.3x) | 1083 (-54.1%) | 1,921 (-39.7%) |
| 1B QLoRA | 45.8 (2.4x) | 0.3 (-76.0%) | 252.0 (4.2x) | 1127 (-52.2%) | 2,255 (-29.2%) |
| 3B BF16 (baseline) | 7.6 | 3.0 | 21.2 | 6129 | 7,419 |
| 3B SpinQuant | 19.7 (2.6x) | 0.7 (-76.4%) | 89.7 (4.2x) | 2435 (-60.3%) | 3,726 (-49.8%) |
| 3B QLoRA | 18.5 (2.4x) | 0.7 (-76.1%) | 88.8 (4.2x) | 2529 (-58.7%) | 4,060 (-45.3%) |
(\*) The performance measurement is done using an adb binary-based approach.
(\*\*) It is measured on an Android OnePlus 12 device.
(\*\*\*) Time-to-first-token (TTFT) is measured with prompt length=64
*Footnote:*
- *Decode (tokens/second) is for how quickly it keeps generating. Higher is better.*
- *Time-to-first-token (TTFT for shorthand) is for how fast it generates the first token for a given prompt. Lower is better.*
- *Prefill is the inverse of TTFT (aka 1/TTFT) in tokens/second. Higher is better*
- *Model size \- how big is the model, measured by, PTE file, a binary file format for ExecuTorch*
- *RSS size \- Memory usage in resident set size (RSS)*
## Responsibility & Safety
As part of our Responsible release approach, we followed a three-pronged strategy to managing trust & safety risks:
1. Enable developers to deploy helpful, safe and flexible experiences for their target audience and for the use cases supported by Llama
2. Protect developers against adversarial users aiming to exploit Llama capabilities to potentially cause harm
3. Provide protections for the community to help prevent the misuse of our models
### Responsible Deployment
**Approach:** Llama is a foundational technology designed to be used in a variety of use cases. Examples on how Meta’s Llama models have been responsibly deployed can be found in our [Community Stories webpage](https://llama.meta.com/community-stories/). Our approach is to build the most helpful models, enabling the world to benefit from the technology power, by aligning our model safety for generic use cases and addressing a standard set of harms. Developers are then in the driver’s seat to tailor safety for their use cases, defining their own policies and deploying the models with the necessary safeguards in their Llama systems. Llama 3.2 was developed following the best practices outlined in our [Responsible Use Guide](https://llama.meta.com/responsible-use-guide/).
#### Llama 3.2 Instruct
**Objective:** Our main objectives for conducting safety fine-tuning are to provide the research community with a valuable resource for studying the robustness of safety fine-tuning, as well as to offer developers a readily available, safe, and powerful model for various applications to reduce the developer workload to deploy safe AI systems. We implemented the same set of safety mitigations as in Llama 3, and you can learn more about these in the Llama 3 [paper](https://ai.meta.com/research/publications/the-llama-3-herd-of-models/).
**Fine-Tuning Data:** We employ a multi-faceted approach to data collection, combining human-generated data from our vendors with synthetic data to mitigate potential safety risks. We’ve developed many large language model (LLM)-based classifiers that enable us to thoughtfully select high-quality prompts and responses, enhancing data quality control.
**Refusals and Tone:** Building on the work we started with Llama 3, we put a great emphasis on model refusals to benign prompts as well as refusal tone. We included both borderline and adversarial prompts in our safety data strategy, and modified our safety data responses to follow tone guidelines.
#### Llama 3.2 Systems
**Safety as a System:** Large language models, including Llama 3.2, **are not designed to be deployed in isolation** but instead should be deployed as part of an overall AI system with additional safety guardrails as required. Developers are expected to deploy system safeguards when building agentic systems. Safeguards are key to achieve the right helpfulness-safety alignment as well as mitigating safety and security risks inherent to the system and any integration of the model or system with external tools. As part of our responsible release approach, we provide the community with [safeguards](https://llama.meta.com/trust-and-safety/) that developers should deploy with Llama models or other LLMs, including Llama Guard, Prompt Guard and Code Shield. All our [reference implementations](https://github.com/meta-llama/llama-agentic-system) demos contain these safeguards by default so developers can benefit from system-level safety out-of-the-box.
### New Capabilities and Use Cases
**Technological Advancement:** Llama releases usually introduce new capabilities that require specific considerations in addition to the best practices that generally apply across all Generative AI use cases. For prior release capabilities also supported by Llama 3.2, see [Llama 3.1 Model Card](https://github.com/meta-llama/llama-models/blob/main/models/llama3_1/MODEL_CARD.md), as the same considerations apply here as well.
**Constrained Environments:** Llama 3.2 1B and 3B models are expected to be deployed in highly constrained environments, such as mobile devices. LLM Systems using smaller models will have a different alignment profile and safety/helpfulness tradeoff than more complex, larger systems. Developers should ensure the safety of their system meets the requirements of their use case. We recommend using lighter system safeguards for such use cases, like Llama Guard 3-1B or its mobile-optimized version.
### Evaluations
**Scaled Evaluations:** We built dedicated, adversarial evaluation datasets and evaluated systems composed of Llama models and Purple Llama safeguards to filter input prompt and output response. It is important to evaluate applications in context, and we recommend building dedicated evaluation dataset for your use case.
**Red Teaming:** We conducted recurring red teaming exercises with the goal of discovering risks via adversarial prompting and we used the learnings to improve our benchmarks and safety tuning datasets. We partnered early with subject-matter experts in critical risk areas to understand the nature of these real-world harms and how such models may lead to unintended harm for society. Based on these conversations, we derived a set of adversarial goals for the red team to attempt to achieve, such as extracting harmful information or reprogramming the model to act in a potentially harmful capacity. The red team consisted of experts in cybersecurity, adversarial machine learning, responsible AI, and integrity in addition to multilingual content specialists with background in integrity issues in specific geographic markets.
### Critical Risks
In addition to our safety work above, we took extra care on measuring and/or mitigating the following critical risk areas:
**1\. CBRNE (Chemical, Biological, Radiological, Nuclear, and Explosive Weapons):** Llama 3.2 1B and 3B models are smaller and less capable derivatives of Llama 3.1. For Llama 3.1 70B and 405B, to assess risks related to proliferation of chemical and biological weapons, we performed uplift testing designed to assess whether use of Llama 3.1 models could meaningfully increase the capabilities of malicious actors to plan or carry out attacks using these types of weapons and have determined that such testing also applies to the smaller 1B and 3B models.
**2\. Child Safety:** Child Safety risk assessments were conducted using a team of experts, to assess the model’s capability to produce outputs that could result in Child Safety risks and inform on any necessary and appropriate risk mitigations via fine tuning. We leveraged those expert red teaming sessions to expand the coverage of our evaluation benchmarks through Llama 3 model development. For Llama 3, we conducted new in-depth sessions using objective based methodologies to assess the model risks along multiple attack vectors including the additional languages Llama 3 is trained on. We also partnered with content specialists to perform red teaming exercises assessing potentially violating content while taking account of market specific nuances or experiences.
**3\. Cyber Attacks:** For Llama 3.1 405B, our cyber attack uplift study investigated whether LLMs can enhance human capabilities in hacking tasks, both in terms of skill level and speed.
Our attack automation study focused on evaluating the capabilities of LLMs when used as autonomous agents in cyber offensive operations, specifically in the context of ransomware attacks. This evaluation was distinct from previous studies that considered LLMs as interactive assistants. The primary objective was to assess whether these models could effectively function as independent agents in executing complex cyber-attacks without human intervention. Because Llama 3.2’s 1B and 3B models are smaller and less capable models than Llama 3.1 405B, we broadly believe that the testing conducted for the 405B model also applies to Llama 3.2 models.
### Community
**Industry Partnerships:** Generative AI safety requires expertise and tooling, and we believe in the strength of the open community to accelerate its progress. We are active members of open consortiums, including the AI Alliance, Partnership on AI and MLCommons, actively contributing to safety standardization and transparency. We encourage the community to adopt taxonomies like the MLCommons Proof of Concept evaluation to facilitate collaboration and transparency on safety and content evaluations. Our Purple Llama tools are open sourced for the community to use and widely distributed across ecosystem partners including cloud service providers. We encourage community contributions to our [Github repository](https://github.com/meta-llama/PurpleLlama).
**Grants:** We also set up the [Llama Impact Grants](https://llama.meta.com/llama-impact-grants/) program to identify and support the most compelling applications of Meta’s Llama model for societal benefit across three categories: education, climate and open innovation. The 20 finalists from the hundreds of applications can be found [here](https://llama.meta.com/llama-impact-grants/#finalists).
**Reporting:** Finally, we put in place a set of resources including an [output reporting mechanism](https://developers.facebook.com/llama_output_feedback) and [bug bounty program](https://www.facebook.com/whitehat) to continuously improve the Llama technology with the help of the community.
## Ethical Considerations and Limitations
**Values:** The core values of Llama 3.2 are openness, inclusivity and helpfulness. It is meant to serve everyone, and to work for a wide range of use cases. It is thus designed to be accessible to people across many different backgrounds, experiences and perspectives. Llama 3.2 addresses users and their needs as they are, without insertion unnecessary judgment or normativity, while reflecting the understanding that even content that may appear problematic in some cases can serve valuable purposes in others. It respects the dignity and autonomy of all users, especially in terms of the values of free thought and expression that power innovation and progress.
**Testing:** Llama 3.2 is a new technology, and like any new technology, there are risks associated with its use. Testing conducted to date has not covered, nor could it cover, all scenarios. For these reasons, as with all LLMs, Llama 3.2’s potential outputs cannot be predicted in advance, and the model may in some instances produce inaccurate, biased or other objectionable responses to user prompts. Therefore, before deploying any applications of Llama 3.2 models, developers should perform safety testing and tuning tailored to their specific applications of the model. Please refer to available resources including our [Responsible Use Guide](https://llama.meta.com/responsible-use-guide), [Trust and Safety](https://llama.meta.com/trust-and-safety/) solutions, and other [resources](https://llama.meta.com/docs/get-started/) to learn more about responsible development.
| [
"SUMMARIZATION"
] | Non_BioNLP |
longem/distilbert-base-uncased-finetuned-emotion | longem | text-classification | [
"transformers",
"tensorboard",
"safetensors",
"distilbert",
"text-classification",
"generated_from_trainer",
"dataset:emotion",
"base_model:distilbert/distilbert-base-uncased",
"base_model:finetune:distilbert/distilbert-base-uncased",
"license:apache-2.0",
"model-index",
"autotrain_compatible",
"endpoints_compatible",
"region:us"
] | 1,716,266,317,000 | 2024-05-21T05:04:18 | 7 | 0 | ---
base_model: distilbert-base-uncased
datasets:
- emotion
license: apache-2.0
metrics:
- accuracy
- f1
tags:
- generated_from_trainer
model-index:
- name: distilbert-base-uncased-finetuned-emotion
results:
- task:
type: text-classification
name: Text Classification
dataset:
name: emotion
type: emotion
config: split
split: validation
args: split
metrics:
- type: accuracy
value: 0.9325
name: Accuracy
- type: f1
value: 0.9323568444980697
name: F1
---
<!-- This model card has been generated automatically according to the information the Trainer had access to. You
should probably proofread and complete it, then remove this comment. -->
# distilbert-base-uncased-finetuned-emotion
This model is a fine-tuned version of [distilbert-base-uncased](https://huggingface.co/distilbert-base-uncased) on the emotion dataset.
It achieves the following results on the evaluation set:
- Loss: 0.1587
- Accuracy: 0.9325
- F1: 0.9324
## Model description
More information needed
## Intended uses & limitations
More information needed
## Training and evaluation data
More information needed
## Training procedure
### Training hyperparameters
The following hyperparameters were used during training:
- learning_rate: 2e-05
- train_batch_size: 64
- eval_batch_size: 64
- seed: 42
- optimizer: Adam with betas=(0.9,0.999) and epsilon=1e-08
- lr_scheduler_type: linear
- num_epochs: 2
### Training results
| Training Loss | Epoch | Step | Validation Loss | Accuracy | F1 |
|:-------------:|:-----:|:----:|:---------------:|:--------:|:------:|
| 0.2164 | 1.0 | 250 | 0.1766 | 0.93 | 0.9305 |
| 0.137 | 2.0 | 500 | 0.1587 | 0.9325 | 0.9324 |
### Framework versions
- Transformers 4.41.0
- Pytorch 2.3.0+cu121
- Datasets 2.19.1
- Tokenizers 0.19.1
| [
"TEXT_CLASSIFICATION"
] | Non_BioNLP |
ashaduzzaman/bert-finetuned-squad | ashaduzzaman | question-answering | [
"transformers",
"tensorboard",
"safetensors",
"bert",
"question-answering",
"generated_from_trainer",
"en",
"dataset:rajpurkar/squad",
"base_model:google-bert/bert-base-cased",
"base_model:finetune:google-bert/bert-base-cased",
"license:apache-2.0",
"endpoints_compatible",
"region:us"
] | 1,724,234,751,000 | 2024-08-24T01:23:06 | 131 | 0 | ---
base_model: bert-base-cased
datasets:
- rajpurkar/squad
language:
- en
library_name: transformers
license: apache-2.0
metrics:
- accuracy
- f1
- exact_match
pipeline_tag: question-answering
tags:
- generated_from_trainer
model-index:
- name: bert-finetuned-squad
results: []
---
<!-- This model card has been generated automatically according to the information the Trainer had access to. You
should probably proofread and complete it, then remove this comment. -->
# BERT Fine-Tuned for Question Answering (SQuAD)
## Model Description
This model is a fine-tuned version of [BERT-base-cased](https://huggingface.co/bert-base-cased), specifically optimized for the task of question answering. It was trained on the SQuAD (Stanford Question Answering Dataset) to understand and extract relevant information from a given context, based on a provided question. BERT is a transformer-based model that uses attention mechanisms to improve the contextual understanding of text, which makes it well-suited for question-answering tasks.
## Intended Uses & Limitations
**Intended Uses:**
- **Question Answering:** This model can be used to extract answers from a given context based on a specific question. It's suitable for applications such as chatbots, virtual assistants, and customer support systems where retrieving relevant information is crucial.
- **Information Retrieval:** Useful in scenarios requiring quick and accurate information extraction from large bodies of text.
**Limitations:**
- **Domain Adaptation:** The model may not perform well on domains that are significantly different from the training data (e.g., technical manuals, medical documents).
- **Context Size Limitation:** Due to the input length limit of BERT (512 tokens), the context must be relatively short, or it needs to be chunked appropriately.
- **Bias and Fairness:** The model may reflect biases present in the SQuAD dataset and its pretraining corpus, potentially affecting the impartiality of answers.
## How to Use
To use this model for question answering, you can utilize the Hugging Face `transformers` library. Here’s a Python code example:
```python
from transformers import pipeline
model_checkpoint = "Ashaduzzaman/bert-finetuned-squad"
question_answerer = pipeline("question-answering", model=model_checkpoint)
question = "What is the name of the architectures?"
context = """
🤗 Transformers (formerly known as pytorch-transformers and pytorch-pretrained-
and pytorch-nlp) provides general-purpose architectures (BERT, GPT-2, RoBERTa, XLM, DistilBert, XLNet…) for Natural
Language Understanding (NLU) and Natural Language Generation (NLG) with over 32+ pretrained models in 100+ languages and
with state-of-the-art performance on SQuAD, GLUE, AWS Glue, and other benchmarks.
"""
result = question_answerer(question=question, context=context)
print(result['answer'])
```
## Training and Evaluation Data
- **Dataset Used:** The model was fine-tuned on the SQuAD dataset, a benchmark dataset for training and evaluating question-answering models. SQuAD provides a collection of questions and corresponding context paragraphs, with labeled answers.
## Training Procedure
The model was trained using the Hugging Face `transformers` library with the following hyperparameters:
- **Learning Rate:** 2e-05
- **Training Batch Size:** 8
- **Evaluation Batch Size:** 8
- **Seed:** 42
- **Optimizer:** Adam with `betas=(0.9,0.999)` and `epsilon=1e-08`
- **Learning Rate Scheduler:** Linear
- **Number of Epochs:** 1
- **Mixed Precision Training:** Native AMP (Automatic Mixed Precision)
### Training Results
- **Final Training Loss:** 1.22
- **Exact Match (EM):** 79.99
- **F1 Score:** 87.55
## Evaluation
The model's performance was evaluated using standard SQuAD metrics, including Exact Match (EM) and F1 score. These metrics measure the model's ability to provide accurate and precise answers to the questions based on the context.
## Framework Versions
- **Transformers:** 4.42.4
- **PyTorch:** 2.3.1+cu121
- **Datasets:** 2.21.0
- **Tokenizers:** 0.19.1 | [
"QUESTION_ANSWERING"
] | Non_BioNLP |
facebook/fasttext-is-vectors | facebook | feature-extraction | [
"fasttext",
"feature-extraction",
"is",
"arxiv:1607.04606",
"arxiv:1802.06893",
"arxiv:1607.01759",
"arxiv:1612.03651",
"license:cc-by-sa-3.0",
"region:us"
] | 1,679,208,187,000 | 2023-06-03T22:12:32 | 9 | 0 | ---
language: is
library_name: fasttext
license: cc-by-sa-3.0
tags:
- feature-extraction
widget:
- text: apple
example_title: apple
---
# fastText (Icelandic)
fastText is an open-source, free, lightweight library that allows users to learn text representations and text classifiers. It works on standard, generic hardware. Models can later be reduced in size to even fit on mobile devices. It was introduced in [this paper](https://arxiv.org/abs/1607.04606). The official website can be found [here](https://fasttext.cc/).
## Model description
fastText is a library for efficient learning of word representations and sentence classification. fastText is designed to be simple to use for developers, domain experts, and students. It's dedicated to text classification and learning word representations, and was designed to allow for quick model iteration and refinement without specialized hardware. fastText models can be trained on more than a billion words on any multicore CPU in less than a few minutes.
It includes pre-trained models learned on Wikipedia and in over 157 different languages. fastText can be used as a command line, linked to a C++ application, or used as a library for use cases from experimentation and prototyping to production.
## Intended uses & limitations
You can use pre-trained word vectors for text classification or language identification. See the [tutorials](https://fasttext.cc/docs/en/supervised-tutorial.html) and [resources](https://fasttext.cc/docs/en/english-vectors.html) on its official website to look for tasks that interest you.
### How to use
Here is how to load and use a pre-trained vectors
```python
>>> import fasttext
>>> from huggingface_hub import hf_hub_download
>>> model_path = hf_hub_download(repo_id="facebook/fasttext-is-vectors", filename="model.bin")
>>> model = fasttext.load_model(model_path)
>>> model.words
['the', 'of', 'and', 'to', 'in', 'a', 'that', 'is', ...]
>>> len(model.words)
145940
>>> model['bread']
array([ 4.89417791e-01, 1.60882145e-01, -2.25947708e-01, -2.94273376e-01,
-1.04577184e-01, 1.17962055e-01, 1.34821936e-01, -2.41778508e-01, ...])
```
Here is how to use this model to query nearest neighbors of an English word vector:
```python
>>> import fasttext
>>> from huggingface_hub import hf_hub_download
>>> model_path = hf_hub_download(repo_id="facebook/fasttext-en-nearest-neighbors", filename="model.bin")
>>> model = fasttext.load_model(model_path)
>>> model.get_nearest_neighbors("bread", k=5)
[(0.5641006231307983, 'butter'),
(0.48875734210014343, 'loaf'),
(0.4491206705570221, 'eat'),
(0.42444291710853577, 'food'),
(0.4229326844215393, 'cheese')]
```
Here is how to use this model to detect the language of a given text:
```python
>>> import fasttext
>>> from huggingface_hub import hf_hub_download
>>> model_path = hf_hub_download(repo_id="facebook/fasttext-language-identification", filename="model.bin")
>>> model = fasttext.load_model(model_path)
>>> model.predict("Hello, world!")
(('__label__eng_Latn',), array([0.81148803]))
>>> model.predict("Hello, world!", k=5)
(('__label__eng_Latn', '__label__vie_Latn', '__label__nld_Latn', '__label__pol_Latn', '__label__deu_Latn'),
array([0.61224753, 0.21323682, 0.09696738, 0.01359863, 0.01319415]))
```
### Limitations and bias
Even if the training data used for this model could be characterized as fairly neutral, this model can have biased predictions.
Cosine similarity can be used to measure the similarity between two different word vectors. If two two vectors are identical, the cosine similarity will be 1. For two completely unrelated vectors, the value will be 0. If two vectors have an opposite relationship, the value will be -1.
```python
>>> import numpy as np
>>> def cosine_similarity(word1, word2):
>>> return np.dot(model[word1], model[word2]) / (np.linalg.norm(model[word1]) * np.linalg.norm(model[word2]))
>>> cosine_similarity("man", "boy")
0.061653383
>>> cosine_similarity("man", "ceo")
0.11989131
>>> cosine_similarity("woman", "ceo")
-0.08834904
```
## Training data
Pre-trained word vectors for 157 languages were trained on [Common Crawl](http://commoncrawl.org/) and [Wikipedia](https://www.wikipedia.org/) using fastText. These models were trained using CBOW with position-weights, in dimension 300, with character n-grams of length 5, a window of size 5 and 10 negatives. We also distribute three new word analogy datasets, for French, Hindi and Polish.
## Training procedure
### Tokenization
We used the [Stanford word segmenter](https://nlp.stanford.edu/software/segmenter.html) for Chinese, [Mecab](http://taku910.github.io/mecab/) for Japanese and [UETsegmenter](https://github.com/phongnt570/UETsegmenter) for Vietnamese. For languages using the Latin, Cyrillic, Hebrew or Greek scripts, we used the tokenizer from the [Europarl](https://www.statmt.org/europarl/) preprocessing tools. For the remaining languages, we used the ICU tokenizer.
More information about the training of these models can be found in the article [Learning Word Vectors for 157 Languages](https://arxiv.org/abs/1802.06893).
### License
The word vectors are distributed under the [*Creative Commons Attribution-Share-Alike License 3.0*](https://creativecommons.org/licenses/by-sa/3.0/).
### Evaluation datasets
The analogy evaluation datasets described in the paper are available here: [French](https://dl.fbaipublicfiles.com/fasttext/word-analogies/questions-words-fr.txt), [Hindi](https://dl.fbaipublicfiles.com/fasttext/word-analogies/questions-words-hi.txt), [Polish](https://dl.fbaipublicfiles.com/fasttext/word-analogies/questions-words-pl.txt).
### BibTeX entry and citation info
Please cite [1] if using this code for learning word representations or [2] if using for text classification.
[1] P. Bojanowski\*, E. Grave\*, A. Joulin, T. Mikolov, [*Enriching Word Vectors with Subword Information*](https://arxiv.org/abs/1607.04606)
```markup
@article{bojanowski2016enriching,
title={Enriching Word Vectors with Subword Information},
author={Bojanowski, Piotr and Grave, Edouard and Joulin, Armand and Mikolov, Tomas},
journal={arXiv preprint arXiv:1607.04606},
year={2016}
}
```
[2] A. Joulin, E. Grave, P. Bojanowski, T. Mikolov, [*Bag of Tricks for Efficient Text Classification*](https://arxiv.org/abs/1607.01759)
```markup
@article{joulin2016bag,
title={Bag of Tricks for Efficient Text Classification},
author={Joulin, Armand and Grave, Edouard and Bojanowski, Piotr and Mikolov, Tomas},
journal={arXiv preprint arXiv:1607.01759},
year={2016}
}
```
[3] A. Joulin, E. Grave, P. Bojanowski, M. Douze, H. Jégou, T. Mikolov, [*FastText.zip: Compressing text classification models*](https://arxiv.org/abs/1612.03651)
```markup
@article{joulin2016fasttext,
title={FastText.zip: Compressing text classification models},
author={Joulin, Armand and Grave, Edouard and Bojanowski, Piotr and Douze, Matthijs and J{'e}gou, H{'e}rve and Mikolov, Tomas},
journal={arXiv preprint arXiv:1612.03651},
year={2016}
}
```
If you use these word vectors, please cite the following paper:
[4] E. Grave\*, P. Bojanowski\*, P. Gupta, A. Joulin, T. Mikolov, [*Learning Word Vectors for 157 Languages*](https://arxiv.org/abs/1802.06893)
```markup
@inproceedings{grave2018learning,
title={Learning Word Vectors for 157 Languages},
author={Grave, Edouard and Bojanowski, Piotr and Gupta, Prakhar and Joulin, Armand and Mikolov, Tomas},
booktitle={Proceedings of the International Conference on Language Resources and Evaluation (LREC 2018)},
year={2018}
}
```
(\* These authors contributed equally.)
| [
"TEXT_CLASSIFICATION"
] | Non_BioNLP |
aritrasen/bge-base-en-v1.5-ft_ragds | aritrasen | sentence-similarity | [
"sentence-transformers",
"safetensors",
"bert",
"sentence-similarity",
"feature-extraction",
"generated_from_trainer",
"dataset_size:9598",
"loss:MultipleNegativesRankingLoss",
"arxiv:1908.10084",
"arxiv:1705.00652",
"base_model:BAAI/bge-base-en-v1.5",
"base_model:finetune:BAAI/bge-base-en-v1.5",
"autotrain_compatible",
"text-embeddings-inference",
"endpoints_compatible",
"region:us"
] | 1,721,634,259,000 | 2024-07-22T07:44:33 | 8 | 0 | ---
base_model: BAAI/bge-base-en-v1.5
datasets: []
language: []
library_name: sentence-transformers
pipeline_tag: sentence-similarity
tags:
- sentence-transformers
- sentence-similarity
- feature-extraction
- generated_from_trainer
- dataset_size:9598
- loss:MultipleNegativesRankingLoss
widget:
- source_sentence: 'Tummy time is recommended from birth to strengthen baby''s arms,
back and neck muscles and prevent the back of his head becoming flat. Jay L. Hoecker,
M.D, of the Mayo Clinic, says babies should not sleep or be left unattended on
tummies, but the position will help move them toward milestones of rolling and
crawling. If your baby does not like lying on his tummy, there are ways you can
help him learn to enjoy it.
Comfort
Make sure baby is nice and comfortable by using a soft surface like a bed and
a fleecy blanket. Your baby may feel more comfortable on his tummy if you take
his clothes and diaper off, making sure the room is nice and warm. This way he
will not have anything pressing into his tummy and the feeling of being naked
may add to his enjoyment. It is best not to make tummy time straight after a feed
as his full tummy may not feel comfortable. Try to position his arms in front
of him as he can eventually start to use them to lean on. Don''t make tummy time
too long to begin with; HealthyChildren.org advises that a newborn only needs
three to five minutes two or three times a day, which can gradually be increased.
Touching
Your baby may enjoy the sensation of your fingers running gently down her back
while she lies on her tummy -- this works better on bare skin. You can also stroke
her cheeks, tickle her toes and gently massage her hands, feet and legs. If she
is feeling a bit uncomfortable or unsure, this touching may help to put her at
ease. Massage will also relax her muscles, helping her whole body feel more comfortable.
Stimulation
Keep your baby stimulated with lots of visual aids. Dr. Sears says young babies
like to look at bright colors, stripes and black and white objects, so place some
of these around your baby to catch his attention. Wave noisy toys, such as rattles
or scrunchy toys for him to look at, or wiggle your fingers in front of him. You
could also play some music during tummy time, as this sound stimulation may be
another way of distracting him from any anxiety about this new position.
Interaction
Your baby loves listening to your voice, so try reclining beside her and singing
or talking. You could also place her on a bed and sit on the floor so your face
is level with hers. Tummy time can also work if you lie on your back with baby
on her tummy on your tummy. You make a warm and soft surface, and she will love
being that close to you and looking into your face. Another way of introducing
tummy time is by laying her across your lap on her front.
References
Photo Credits
- BananaStock/BananaStock/Getty Images'
sentences:
- Why is tummy time recommended for babies from birth?
- Who were the three stars in the NHL game between Buffalo Sabres and Edmonton Oilers?
- What was the modification made to the Creamy Wild Mushroom Soup with Pecorino
recipe by the user dchapdelaine?
- source_sentence: 'Community Justice Project: community lawyers who collaborate closely
with community organizers and grassroots groups in low-income communities of color.
Follow @cjpmiami
Miami Dream Defenders: multiracial group of young people who are organizing to
build power in our communities to advance a new vision for the state. Follow @miami_dreamdefenders
(F)emPower Miami: queer culture shifters running a bail fund to free people from
jail. Follow @fempowermia
Black Lives Matter Broward: alliance of community organizations and individuals
aiming to abolish institutional racism, and to democratize and transform oppressive
systems primarily via popular mobilizations. Follow @BlackLivesMatterAllianceBroward
Engage Miami: taking down barriers and building bridges to equitable civic leadership
through voter registration, civic education, and organizing campaigns on the issues
that matter to young people in Miami. Follow @engagemia
ACLU of Greater Miami Chapter: organization working daily to defend the individual
rights and personal freedoms of every man, woman and child in this country. Follow
@ACLUFL
Catalyst Miami: nonprofit organization identifying and collectively solving issues
adversely affecting low-wealth communities throughout Miami-Dade County. Follow
@catalystmiami
The New Florida Majority: independent organization working to increase the voting
and political power of Florida’s Black and brown communities. Follow @newflmajority
Advancing
Color of Change: the nation’s largest online racial justice organization, Color
of Change moves decision-makers in corporations and government to create a more
human and less hostile world for Black people in America. Follow @colorofchange
Communities United Against Police Brutality: an all-volunteer organization that
works to combat police brutality from many angles, including political and legislative
action, education, research, and providing services and support for victims and
their families. Follow @cuapb.org
Equal Justice Initiative: an organization committed to ending mass incarceration
and excessive punishment in the United States, to challenging social and economic
injustice, and to protecting basic human rights for the most vulnerable people
in American society. Follow @eji_org
An Essential Reading Guide for Fighting Racism via Buzzfeed
Do the work: an anti-racist reading list via The Guardian
Anti-Racist Reading Recs via Bookshop.org
47 Black-Owned Bookstores Across the Country that You Can Support via Entertainment
Weekly
From Hyperallergic: An Essential Watchlist of Groundbreaking Black Documentaries
- These films illustrate both the undeniable threat of white supremacist capitalist
patriarchy and the incomparable strength of Blackness.
Code switch by NPR
About Race with Reni Eddo-Lodge
Pod for the cause from the Leadership Conference on Civil & Human Rights
Black Lives Matter: Media and Where to Find It
Your Black Friends Are Busy: a growing resource for learning about anti-racism,
and supporting the people & organizations doing important work for the Black Lives
Matter movement.
Anti-Racist Resource Guide created by Victoria Alexander, MEd
Miami Organizations Doing the Work for Black Justice by Culture Crusaders
Blacklivesmatters.carrd.co/
181 Black-owned businesses across the United States to support
10 Reasons You Should Support Black Lives'
sentences:
- What are the ingredients needed to prepare the Simple Calzone recipe?
- What was the route of the walk described in the blog post?
- What is the mission of the Equal Justice Initiative?
- source_sentence: 'In vitro meat: Can we stomach it?
In vitro meat, also referred to as cultured meat, is a meat product grown from
extracted cells in a factory. It''s a test concept introduced by researchers to
combat many ethical issues found in today''s industrial livestock operations.
The meat''s production would also contribute to reduced environmental impacts,
global hunger solutions, human health promotion and obesity reduction.\
Within the next 35 years, a process only in its beginning stages could become
the norm, replacing most traditional livestock meat we find on grocery store shelves
today.
Initially, it sounds like something from a futuristic science fiction novel or
a dystopian society. Meat grown in a factory is straight from "The Jetsons" or
"Star Trek." It''s out of the question and couldn''t even be possible, right?
Wrong, completely wrong.
Cultured meat is happening right now. In fact, it has already happened. The first
in vitro hamburger was served in 2013 in London. The two year project was highly
complicated and not economically sustainable, with the final price totaling a
hefty $325,000. But what did the lucky few selected to taste the burger have to
say? Their biggest complaints were that the burger didn''t taste the same as one
that comes from grazing livestock, and it was a bit dry.
Despite the burger falling short on the taste and consistency fields, hopefuls
still see the potential and are trying to obtain more funding for their research.
If more support and funding is provided, the concept can become large scale, allowing
the price to drop and more types of "meat" to be created.
I use the term "create," but of course I must mention that many, if not most,
creationists would be completely against not only the research, but the concept
itself. Many believe that growing meat goes against what is natural. Creating
meat in a test tube, adding our own flavoring, vitamins and additives would be
overstepping our boundaries or even "playing God." There are even people who have
gone as far as saying that it would be equivalent to serving up zombies on a plate.
It''s buffoonery to go as far as calling the meat "zombie production", but how
can the accusation be challenged when there are companies like BiteLabs arising?
The company''s basis is to produce salami made of tissue samples from celebrities.
Gut reaction says that this is disturbing, and, more alarmingly, cannibalistic.
But is it, really?
Challenging the traditional definition of cannibalism, it is decidedly not. The
production and consumption of the salami would take place between two (or more,
no judgement here) consensual adults. It would also fail to violate any U.S. laws
against murder or body desecration. Maybe it''s skin crawling and socially unacceptable,
but I wouldn''t go as far as to call it cannibalism. All Sweeney Todd jokes aside,
if forced to use the word cannibalism, I''d say it is a form of "humane cannibalism,"
if anything.
This argument presents a problem in itself, though. Since in vitro meat production
is such a challenging and fairly newly researched topic there are many gray areas
and questions posed that are difficult to answer because of how our society views
the ideas of life, naturalism, cannibalism and humane treatment of animals. For
in vitro meat to be a success, these terms must be redefined or at least understood
in relation to factory grown meat.
Educational briefings and infographics would have to be readily available for
the public, or they would simply not understand what it is they would be eating
and would be against the meat replacement. If people aren''t able to understand
and accept that factory grown meat follows the same development process that meat
from traditional livestock does, they will not back the introduction of the meat
into the market.
Researchers behind the factory meat growing process also present the argument
that in vitro meat would technically qualify as a vegan food. Part of this idea
is because it would be cruelty free and a non GMO product.
The vegan-friendly argument is backed by PETA, who introduced a $1 million contest
in 2008. The rules of the contest laid out that the company who was able to produce
the first marketable in vitro chicken meat would receive the prize.
Unfortunately, no one was successful before the deadline arrived, and the prize
money went towards combating animal cruelty in today''s meat production process.
PETA still considered the contest to be a raging success, though, based on the
leaps and bounds made in the research and understanding of in vitro meat.
As someone who practices vegetarianism, I can see both sides of the argument.
Yes, there would be cruelty-free meat available, which is a benefit that conquers
a few issues vegetarians and vegans have with industrial livestock productions.
It wouldn''t offer a solution, though, to diehards who are not simply skipping
out on meat products because they promote animal cruelty.
Some, such as Pennsylvania State University environmental resource management
sophomore and environmental sustainability enthusiast Emily Newman, will not be
supporting the endeavor, cruelty free or not.
When asked if she would ever find herself eating cultured meat, Newman, who has
been a vegetarian for nearly two years and even practiced veganism for a brief
period said, "No, definitely not. Food comes from the ground. The earth is giving
it to us. We can utilize it. That''s it, no need for anything to be grown in a
lab."
Most vegetarians and vegans would hold the same standard. To suggest that cultured
meat would be vegan friendly is to redefine the core values which people who practice
a plant based diet hold themselves to.
I, however, am a big fan of the idea, not because I would find myself chowing
down on some factory grown burgers or shoveling test tube bacon in my mouth, but
because people who see meat as a necessity for every meal, the people who are
hurting our environment and relishing in their poor health habits, will finally
be able to contain their socially and environmentally poisonous behavior.
Personally, I''m still holding out for the real wave of the future- the Spy Kids''
food Rehydrator, or the Star Trek Food Replicator. Until the glorious day comes
when they''re introduced, in vitro meat will have to do, and I''m looking forward
to it''s mass introduction, societal acceptance and growth within the next few
decades.
Reach the columnist at [email protected] or follow her on Twitter @mikayrodr.'
sentences:
- What is the role of a Mobility Engineer?
- What were the main criticisms of the first in vitro hamburger served in 2013?
- What is the pre-show ritual of Alexi Laiho, the frontman and guitarist of Children
of Bodom?
- source_sentence: 'Contrary to what many people believe, disability is not a concern
that is limited to the elderly. The reality is that you could suffer a temporary,
or permanent, disability at any time during the course of your lifetime – though
the odds do increase with age. Fortunately, the United States government has programs
that offer financial assistance to individuals who are disabled. Both the Supplemental
Security Income (SSI) and Social Security Disability Insurance (SSDI) program
provide monetary assistance to disabled individuals who qualify. Though the programs
are similar, they have different eligibility guidelines and often provide very
different benefits. To help ensure that you receive all the benefits to which
you may be entitled, the Indianapolis elder law attorneys at Frank & Kraft explain
the differences and similarities between SSI and SSDI.
Are You Disabled?
The first hurdle you must get past if you wish to qualify for either (or both)
SSI or SSDI is to
Supplemental Security Income (SSI)
If you meet the SSA definition of “disabled,” the only other eligibility requirement
for the SSI program is that you have income and resources that fall below the
program limits. The resources limit for an individual is $2,000 and for a married
couple $3,000 in most states. Unlike the SSDI program, an applicant is not required
to have a work history to qualify for SSI; however, the monthly benefits for SSI
recipients are typically less than for SSDI recipients as well. In 2018, for example,
the maximum SSI benefit, referred to as the “Federal Benefit Rate (FBR),” is $750
per month for individuals and $1,125 for couples. The FBR increases annually if
there is a Social Security cost-of-living adjustment. In addition, some states
provide a state supplement, ranging from $10 to $200, which is added to the federal
SSI benefit payment.
Another difference between SSI and SSDI is that family members cannot benefit
from an applicant’s eligibility for SSI benefits. You may, however, be automatically
eligible for other government assistance programs, such as Medicaid or Supplemental
Nutrition Assistance Program (SNAP), once you are approved for SSI benefits.
Social Security Disability Income SSDI
Qualifying for the SSDI program is more difficult; however, the benefits are typically
better if you do get approved. To qualify for SSDI you must have a sufficient
work history prior to becoming disabled. Working earns you “work credits” that
you need to qualify for SSDI. The number of “work credits” you need will depend
on your age at the time of application; however, most applicants need to have
earned 20 credits during the preceding 10 years. A work credit is earned by earning
a designated amount ($1,320 for the year 2018) up to a maximum of four credits
a year if you earned $5,280 or more. Because SSDI benefits are based on your work
history, the monthly benefit you receive will almost always be higher than the
current SSI benefit. By way of example, the average monthly SSDI benefit for 2018
is $1,197 with a maximum benefit of $2,788. Another important benefit to qualifying
for SSDI is that if you are found to be eligible, your dependents may also qualify
for monthly benefits based on your work record.
Note: It is possible to receive both SSDI and SSI if your SSDI benefit is small
enough that you meet the income limit for the SSI program.
How to Apply for SSI or SSDI
You can apply for both SSDI and SSI online through the Social Security Administration’s
website. Unfortunately, about two out of every three initial applications for
SSDI are denied which is why it is usually in your best interest to consult with
an experienced elder law attorney if you feel you might be entitled to SSI or
SSDI benefits before completing the application.
Contact Indianapolis Elder Law Attorneys
For more information, please download our FREE estate planning worksheet. If you
have questions or concerns about SSI, SSDI, or any other elder law issues, contact
the experienced Indianapolis'
sentences:
- What is the primary function of pet therapy in medical institutions?
- What are the differences and similarities between the Supplemental Security Income
(SSI) and Social Security Disability Insurance (SSDI) programs?
- What is the net worth of Ross according to Forbes?
- source_sentence: 'PSY’s “Gangnam Style” T-Shirt Sold on German Online Store
PSY’s “Gangnam Style” took the U.S. by storm last week, and now, it’s reached
a German online shopping mall as well.
Recently, an online t-shirt store, “Spreadshirt,” revealed a new product inspired
by PSY’s “Gangnam Style.” The shirt comes with a picture of PSY’s signature “horse
dance,” and lines that say, “Keep Calm and Gangnam Style.” The “Keep Calm” design
is one of “Spreadshirt’s” most popular items, and the PSY’s edition is the latest
one to come from the highly successful online store.
It’s unclear how many copies of the PSY’s shirt have sold out so far, but Korean
press and netizens are taking it as a reflection of how popular and viral “Gangnam
Style” has gone over the past week.
Netizens commented, “’Gangnam Style’ is daebak,” “I need to order that shirt now,”
and “I wonder who designed that.”
With over 300 employees, the Geremany-based “Spreadshirt” is one of the fastest
growing and largest online t-shirt retailers. It is expected to reach $100 million
in sales this year.
You can order your own “Keep Calm and Gangnam Style” shirt here!'
sentences:
- What is the design on the new product inspired by PSY’s “Gangnam Style” sold on
the German online store "Spreadshirt"?
- Why is Talbots Inc. closing its Fashion Valley store?
- What are the guidelines for submitting an article or story to the YouTube model
railroading community?
---
# SentenceTransformer based on BAAI/bge-base-en-v1.5
This is a [sentence-transformers](https://www.SBERT.net) model finetuned from [BAAI/bge-base-en-v1.5](https://huggingface.co/BAAI/bge-base-en-v1.5). It maps sentences & paragraphs to a 768-dimensional dense vector space and can be used for semantic textual similarity, semantic search, paraphrase mining, text classification, clustering, and more.
## Model Details
### Model Description
- **Model Type:** Sentence Transformer
- **Base model:** [BAAI/bge-base-en-v1.5](https://huggingface.co/BAAI/bge-base-en-v1.5) <!-- at revision a5beb1e3e68b9ab74eb54cfd186867f64f240e1a -->
- **Maximum Sequence Length:** 512 tokens
- **Output Dimensionality:** 768 tokens
- **Similarity Function:** Cosine Similarity
<!-- - **Training Dataset:** Unknown -->
<!-- - **Language:** Unknown -->
<!-- - **License:** Unknown -->
### Model Sources
- **Documentation:** [Sentence Transformers Documentation](https://sbert.net)
- **Repository:** [Sentence Transformers on GitHub](https://github.com/UKPLab/sentence-transformers)
- **Hugging Face:** [Sentence Transformers on Hugging Face](https://huggingface.co/models?library=sentence-transformers)
### Full Model Architecture
```
SentenceTransformer(
(0): Transformer({'max_seq_length': 512, 'do_lower_case': True}) with Transformer model: BertModel
(1): Pooling({'word_embedding_dimension': 768, 'pooling_mode_cls_token': True, 'pooling_mode_mean_tokens': False, 'pooling_mode_max_tokens': False, 'pooling_mode_mean_sqrt_len_tokens': False, 'pooling_mode_weightedmean_tokens': False, 'pooling_mode_lasttoken': False, 'include_prompt': True})
(2): Normalize()
)
```
## Usage
### Direct Usage (Sentence Transformers)
First install the Sentence Transformers library:
```bash
pip install -U sentence-transformers
```
Then you can load this model and run inference.
```python
from sentence_transformers import SentenceTransformer
# Download from the 🤗 Hub
model = SentenceTransformer("aritrasen/bge-base-en-v1.5-ft_ragds")
# Run inference
sentences = [
'PSY’s “Gangnam Style” T-Shirt Sold on German Online Store\nPSY’s “Gangnam Style” took the U.S. by storm last week, and now, it’s reached a German online shopping mall as well.\nRecently, an online t-shirt store, “Spreadshirt,” revealed a new product inspired by PSY’s “Gangnam Style.” The shirt comes with a picture of PSY’s signature “horse dance,” and lines that say, “Keep Calm and Gangnam Style.” The “Keep Calm” design is one of “Spreadshirt’s” most popular items, and the PSY’s edition is the latest one to come from the highly successful online store.\nIt’s unclear how many copies of the PSY’s shirt have sold out so far, but Korean press and netizens are taking it as a reflection of how popular and viral “Gangnam Style” has gone over the past week.\nNetizens commented, “’Gangnam Style’ is daebak,” “I need to order that shirt now,” and “I wonder who designed that.”\nWith over 300 employees, the Geremany-based “Spreadshirt” is one of the fastest growing and largest online t-shirt retailers. It is expected to reach $100 million in sales this year.\nYou can order your own “Keep Calm and Gangnam Style” shirt here!',
'What is the design on the new product inspired by PSY’s “Gangnam Style” sold on the German online store "Spreadshirt"?',
'Why is Talbots Inc. closing its Fashion Valley store?',
]
embeddings = model.encode(sentences)
print(embeddings.shape)
# [3, 768]
# Get the similarity scores for the embeddings
similarities = model.similarity(embeddings, embeddings)
print(similarities.shape)
# [3, 3]
```
<!--
### Direct Usage (Transformers)
<details><summary>Click to see the direct usage in Transformers</summary>
</details>
-->
<!--
### Downstream Usage (Sentence Transformers)
You can finetune this model on your own dataset.
<details><summary>Click to expand</summary>
</details>
-->
<!--
### Out-of-Scope Use
*List how the model may foreseeably be misused and address what users ought not to do with the model.*
-->
<!--
## Bias, Risks and Limitations
*What are the known or foreseeable issues stemming from this model? You could also flag here known failure cases or weaknesses of the model.*
-->
<!--
### Recommendations
*What are recommendations with respect to the foreseeable issues? For example, filtering explicit content.*
-->
## Training Details
### Training Dataset
#### Unnamed Dataset
* Size: 9,598 training samples
* Columns: <code>positive</code> and <code>anchor</code>
* Approximate statistics based on the first 1000 samples:
| | positive | anchor |
|:--------|:--------------------------------------------------------------------------------------|:----------------------------------------------------------------------------------|
| type | string | string |
| details | <ul><li>min: 172 tokens</li><li>mean: 467.87 tokens</li><li>max: 512 tokens</li></ul> | <ul><li>min: 7 tokens</li><li>mean: 18.68 tokens</li><li>max: 43 tokens</li></ul> |
* Samples:
| positive | anchor |
|:----------------------------------------------------------------------------------------------------------------------------------------------------------------------------------------------------------------------------------------------------------------------------------------------------------------------------------------------------------------------------------------------------------------------------------------------------------------------------------------------------------------------------------------------------------------------------------------------------------------------------------------------------------------------------------------------------------------------------------------------------------------------------------------------------------------------------------------------------------------------------------------------------------------------------------------------------------------------------------------------------------------------------------------------------------------------------------------------------------------------------------------------------------------------------------------------------------------------------------------------------------------------------------------------------------------------------------------------------------------------------------------------------------------------------------------------------------------------------------------------------------------------------------------------------------------------------------------------------------------------------------------------------------------------------------------------------------------------------------------------------------------------------------------------------------------------------------------------------------------------------------------------------------------------------------------------------------------------------------------------------------------------------------------------------------------------------------------------------------------------------------------------------------------------------------------------------------------------------------------------------------------------------------------------------------------------------------------------------------------------------------------------------------------------------------------------------------------------------------------------------------------------------------------------------------------------------------------------------------------------------------------------------------------------------------------------------------------------------------------------------------------------------------------------------------------------------------------------------------------------------------------------------------------------------------------------------------------------------------------------------------------------------------------------------------------------------------------------------------------------------------------------------------------------------------------------------------------------------------------------------------------------------------------------------------------------------------------------------------------------------------------------------------------------------------------------------------------------------------------------------------------------------------------------------------------------------------------------------------------------------------------------------------------------------------------------------------------------------------------------------------------------------------------------------------------------------------------------------------------------------------------------------------------------------------------------------------------------------------------------------------------------------------------------------------------------------------------------------------------------------------------------------------------------------------------------------------------------------------------------------------------------------------------------------------------------------------------------------------------------------------------------------------------------------------------------------------------------------------------------------------------------------------------------------------------------------------------------------------------------------------------------------------------------------------------------------------------------------------------------------------------------------------------------------------------------------------------------------------------------------------------------------------------------------------------------------------------|:-----------------------------------------------------------------------------------------------------|
| <code>Caption: Tasmanian berry grower Nic Hansen showing Macau chef Antimo Merone around his property as part of export engagement activities.<br>THE RISE and rise of the Australian strawberry, raspberry and blackberry industries has seen the sectors redouble their international trade focus, with the release of a dedicated export plan to grow their global presence over the next 10 years.<br>Driven by significant grower input, the Berry Export Summary 2028 maps the sectors’ current position, where they want to be, high-opportunity markets and next steps.<br>Hort Innovation trade manager Jenny Van de Meeberg said the value and volume of raspberry and blackberry exports rose by 100 per cent between 2016 and 2017. She said the Australian strawberry industry experienced similar success with an almost 30 per cent rise in export volume and a 26 per cent rise in value to $32.6M over the same period.<br>“Australian berry sectors are in a firm position at the moment,” she said. “Production, adoption of protected substrate cropping, improved genetics and an expanding geographic footprint have all helped put Aussie berries on a positive trajectory.<br>“We are seeing a real transition point. Broad industry interest and a strong commercial appetite for export market development combined with the potential to capitalise on existing trade agreements and build new trade partnerships has created this perfect environment for growth.”<br>High-income countries across Europe, North America and Northern Asia have been identified as having a palate for Australian grown berries with more than 4244 tonnes of fresh berries exported in the last financial year alone.<br>The strategy identified the best short-term prospect markets for the Australian blackberry and raspberry industry as Hong Kong, Singapore, The United Arab Emirates and Canada. The strongest short-term trade options identified for the strawberry sector were Thailand, Malaysia, New Zealand and Macau.<br>The strategy focuses heavily on growing the existing strawberry export market from 4 per cent to at least 8 per cent of national production by volume, in markets with a capacity and willingness to pay a premium for quality fruit. For raspberries and blackberries, the sectors aim to achieve a 5 per cent boost in exports assessed by volume across identified markets by 2021.<br>Tasmanian raspberry exporter Nic Hansen said Australia offers some of the sweetest and most attractive berries in the world, and this combined with our stringent food safety standards across all stages of the supply chain puts growers in a solid position.<br>“We have a great product, we are hungry to expand trade and now with this new plan in place, we have a clear roadmap towards driving growth,” Mr Hansen said.<br>He said it is exciting to see new export market prospects for raspberries: “The more options we have for export the better. Now we just have to get on with the job of ensuring industry has all the tools it needs, such as supporting data and relationship building opportunities, to thrive in new markets.”<br>This project was commissioned by Hort Innovation, and developed by market analysts and research consultants Auspex Strategic Advisory and AgInfinity. Hort Innovation will work now with berry sectors to determine levy-funded activities to support trade.<br>See a summary of the strategy on the Hort Innovation website.<br>For more information on the berry industries, refer to the Horticulture Statistics Handbook and the Strategic Investment Plans for strawberries, raspberries and blackberries. Growers seeking more information should email [email protected]</code> | <code>What is the Berry Export Summary 2028 and what is its purpose?</code> |
| <code>RWSN Collaborations<br>Southern Africa Self-supply Study Review of Self-supply and its support services in African countries<br>A lady in Zimbabwe proudly shows off her onions - watered from her self-supply well<br>© 2015 André Olschewski • Skat<br>Project starts: 2015<br>Project finished: 2016<br>Collaborators & Partners:.<br>Project Description<br>UNICEF and Skat have collaborated on a).<br>Perspectives<br>Reach and benefits:<br>- Self-supply is practised by millions of rural households in Sub-Sahara Africa as well as in Europe, USA and other areas of the world.<br>- Benefits reported from having access to Self-supply water sources include convenience, less time spent for fetching water and access to more and better quality water. In some areas, Self-supply sources offer important added values such as water for productive use, income generation, family safety and improved food security.<br>- Sustainability of services from Self-supply is high as there is strong ownership by people investing in own sources.<br>- As Self-supply sources are shared sources, many people, including poor and vulnerable households, benefit from investments in Self-supply, often at no costs. This means that Self-supply can be effective in reaching the hard-to-reach.<br>- For millions of people in rural areas of Africa, supported Self-supply will be the most cost effective service delivery model to provide access to safe water. This also includes those parts of the population which actually have poor access as they e.g. cannot afford water from communal supplies.<br>- However, in areas where external support for Self-supply is lacking, only marginal improvements can usually be achieved, and the quality of services is lower than in areas where a dedicated support effort was made.<br>Costs and business model for supported Self-supply<br>- In many rural contexts, supported Self-supply is the most cost effective approach for water service delivery. However, as it is not applicable in all contexts, a blended approach combining communal water supply and supported Self-supply models should be followed.<br>- Based on a Life Cycle Cost (LCC) analysis of different service delivery approaches, the LCC for communal supplies are about 40 US$/capita served in the study countries, whereas the LCC for supported Self-supply is about 10 U$/capita.<br>- In sparsely populated areas, communal supplies (e.g. handpumps) are even more costly (up to 100 U$/capita served) as only few people can be served with one additional unit. Serving all rural people with communal supply is therefore not financially viable.<br>- Considering the applicability of Self-supply technologies, in Zambia and Zimbabwe, the cost saving of following a blended approach using both communal supplies and supported Self-supply is almost 50% of the total LCC for reaching 100% of the population by 2030. These cost savings are equivalent to more than 330 million US$ in Zambia and more than 260 million US$ in Zimbabwe.<br>Support services needed<br>- Supported Self-supply is a service delivery model putting support services in place to improve Self-supply, so it is not about a particular technology.<br>- Supported Self-supply is aligned with the Human Rights to Water and Sanitation, which allows a progressive realisation of the universal access to safe water. However, supported Self-supply is not a way to exempt government from its duties: Government has specific roles to play to ensure that everybody will have access to safe water finally.<br>- To sustain and to take Self-supply to scale there is need for contextualised support as well as long-term engagement, capacity development at all levels, M&E and technical support, reliable funding and learning and sharing.<br>- Interministerial cooperation and champions within government agencies are needed to ensure sustainable embedding and for taking Self-supply further, particularly in remote rural areas.<br>- There is no-one-size-fits-all solution for supported Self-supply – for each programme, it needs a contextualized design and follow-up to achieve desired impact.<br>- Hygiene promotion, including Household Water Treatment and Safe Storage (HWTS), is highly recommended for any non-piped water supply services, including Self-supply water sources.<br>- The huge potential for substantially improving the level of water supply for millions of people in rural areas should be accessed through supported Self-supply. Some countries have endorsed supported Self-supply as service delivery model, such as Zimbabwe or Sierra Leone, and in Ethiopia, Self-supply is now being scaled up at national level.<br>More Information<br>» Review of Self-supply and its support services in African countries: Synthesis Report).</code> | <code>What are some of the benefits reported from having access to Self-supply water sources?</code> |
| <code>All Android applications categories<br>Description<br>Coolands for Twitter is a revolutionary twitter client. It has many unique features, gives you the best mobile twitter experience you never imagined before.<br>The first unique feature is Real-Time.<br>You can’t find any refresh button in this app, because you absolutely don’t need to. Every time you open it, you’ll get the latest tweets and while you’re reading, you’ll get incoming tweets in Real-Time. So if your friend mentioned you, you can reply instantly.<br>The second unique feature is Avatar Indicator.<br>Avatar Indicator is small avatars showed on the title bar to indicate that you’ve got new message/tweet/mention. Since it’s real-time, you’ll keep getting incoming tweets while you’re reading your older timeline, Avatar-Indicator will let you know who’s tweet you’ve just got, and decide whether to check it out right away.<br>The third unique feature is Direct Link<br>I think it is obviously the most intuitive and convenient way to open a link. When you want to open a link, just click it in the time line . You can also click a username to open a profile window, click a hash tag to open a search result window. Different kind of links displayed in different colors, you can change it to whatever color you like.<br>The fourth unique feature is Smart Bookmark<br>Have you ever experienced this scenario? When you are reading your home timeline, the app notified you that you’ve got some new tweets, you click “go-to-top” button to read the newest tweets, and then you want to get back to the previous position to continue your reading. How can you do this? In other twitter clients you have to scroll down all the way to find where you were, a lot of time wasted. But in this app, “go-to-top” button will appear when you scrolling up, click it, you can got to top, read the newest tweets. After that when you scrolling down, Smart Bookmark button will appear at the corner. Click it, you can get back to exactly where you were.<br>The fifth unique feature is User Level Notification<br>Notification for all your new tweets is meaningless, if you following more than a few users, you’ll get new tweets all the time. What if you only want to be notified when someone you most care about posted a new tweet? In this app, it’s easy. You can change your friend’s notification setting directly in his/her profile screen. You can also set different notification ringtone for your friends respectively. So when you heard a notification ringtone, you’ll know who he/she is without the need to open your phone. And you can manage all the enabled User-Level-Notification settings in one place.<br>Also has most of the basic twitter client features, like post/delete tweets, retweet, retweet with comment, reply, quote, send/delete direct message, subscribe/unsubscribe lists, follow/unfollow user, multiple accounts support, append picture with your tweets, mention auto complete, recent search auto complete, conversation view.<br>Please give me feedbacks if you have tried it, and I PROMISE to reply all your emails.<br>1.30-1.36 update:<br>*Ad-Free.<br>*3 times faster when launching and loading older tweets.<br>*Support unlimited accounts rather than 3 accounts.<br>*Support notifications for all accounts rather than only for the current account.<br>*Improved mention suggestion feature.<br>*Use URL link to do RT with comment, so you can comment more characters.<br>*Conversation view, click the orange(you can change the color) username in replied tweet to show conversation view.<br>*Support longer tweet, longer tweet will be converted to a picture automatically. You really should try it out your self.<br>*Refined tweet composing view, to support longer tweet.<br>*Support handle text shared by other app.<br>from 103 reviews<br>Download Coolands for Twitter<br>Free - V1.38 - 298K<br>Sorry ...<br>This app is no longer available.<br>Share this app<br>Screenshots</code> | <code>What are the unique features of the Coolands for Twitter app?</code> |
* Loss: [<code>MultipleNegativesRankingLoss</code>](https://sbert.net/docs/package_reference/sentence_transformer/losses.html#multiplenegativesrankingloss) with these parameters:
```json
{
"scale": 20.0,
"similarity_fct": "cos_sim"
}
```
### Evaluation Dataset
#### Unnamed Dataset
* Size: 500 evaluation samples
* Columns: <code>positive</code> and <code>anchor</code>
* Approximate statistics based on the first 1000 samples:
| | positive | anchor |
|:--------|:--------------------------------------------------------------------------------------|:-----------------------------------------------------------------------------------|
| type | string | string |
| details | <ul><li>min: 188 tokens</li><li>mean: 460.02 tokens</li><li>max: 512 tokens</li></ul> | <ul><li>min: 10 tokens</li><li>mean: 18.91 tokens</li><li>max: 39 tokens</li></ul> |
* Samples:
| positive | anchor |
|:---------------------------------------------------------------------------------------------------------------------------------------------------------------------------------------------------------------------------------------------------------------------------------------------------------------------------------------------------------------------------------------------------------------------------------------------------------------------------------------------------------------------------------------------------------------------------------------------------------------------------------------------------------------------------------------------------------------------------------------------------------------------------------------------------------------------------------------------------------------------------------------------------------------------------------------------------------------------------------------------------------------------------------------------------------------------------------------------------------------------------------------------------------------------------------------------------------------------------------------------------------------------------------------------------------------------------------------------------------------------------------------------------------------------------------------------------------------------------------------------------------------------------------------------------------------------------------------------------------------------------------------------------------------------------------------------------------------------------------------------------------------------------------------------------------------------------------------------------------------------------------------------------------------------------------------------------------------------------------------------------------------------------------------------------------------------------------------------------------------------------------------------------------------------------------------------------------------------------------------------------------------------------------------------------------------------------------------------------------------------------------------------------------------------------------------------------------------------------------------------------------------------------------------------------------------------------------------------------------------------------------------------------------------------------------------------------------------------------------------------------------------------------------------------------------------------------------------------------------------------------------------------------------------------------------------------------------------------------------------------------------------------------------------------------------------------------------------------------------------------------------------------------------------------------------------------------------------------------------------------------------------------------------------------------------------------------------------------------------------------------------------------------------------------------------------------------------------------------------------------------------------------------------------------------------------------------------------------------------------------------------------------------------------------------------------------------------------------------------------------------------------------------------------------------------------------------------------------------------------------------------------------------------------------------------------------------------------------------------------------------------------------------------------------------------------------------------------------------------------------------------------------------------------------------------------------------------------------------------------------------------------------------------------------------------------------------------------------------------------------------------------------------------------------------------------------------------------------------------------------------------------------------------------------------------------------------------------------------------------------------------------------------------------------------------------------------------------------------------------------------------------------------------------------------------------------------------------------------------------------------------------------------------------------------------------------------------------------------------------------------------------------------------------------------------------------------------------------------------------------------------------------------------------------------------------------------------------------------------------------------------------------------------------------------------------------------------------------------------------------------------------------------------------------------------------------------------------------------------------------------------------------------------------------------------------------------------------------------------------------------------------------------------------------------------------------------------------------------------------------------------------------------------------------------------------------------------------------------------------------------------------------------------------------------------------------------------------------------------------------------------------------------------------------------------------------------------------------------------------------------------------------------------------------------------------------------------------|:----------------------------------------------------------------------------------------|
| <code>Perhaps Not such a Good Idea<br>I have found trying to run a blog is very time-consuming, and there are other calls on my time. I think it has been demonstrated that if enough people are unable to self-moderate, the nuggets of interest are swamped by the rubbish. Sadly I agree with Mark Frank's assessment. I had hoped more thread topics would be proposed nothing has been suggested by anyone for a while.<br>My personal view is that, considering DaveScot's generally perceived blog persona, I have to admit that he hasn't been (on this site) quite the unmitigated disaster predicted. John Davison, on the other hand has conformed perfectly to predictions, which is a shame, but his choice.<br>I am happy to let things run for a while, but would like to hear from anyone who has a suggestion for a thread topic. Post here or in the suggestions thread<br>23 comments:<br>How about an "ID: show me the research" thread?<br>OK Rich, put some meat on the bones and I'll paste it.<br>Of course I have. I have no respect for you or your cronies from Psnda's Thumb. What did you expect kudos? What do you want another thread for? No one has even attempted to answer my four challenges yet. You know why? I do. It is because they can't, because everything you and your Darwimpian cronies stand for is a myth, an illusion and a hoax. That's why. You might as well close down this flame pit while you are behind as it isn't going anywhere.<br>It is hard to believe isn't it?<br>I love it so!<br>" "How about an "ID: show me the research" thread? "<br>Alan Fox said...<br>OK Rich, put some meat on the bones and I'll paste it. "<br>Why is there no ID research, even on Dembski's blog?<br>Why does ID consist solely of an opinion that some parts of human biology are designed?<br>How would IDers actually prove that some parts of human biology are in fact designed?<br>Expand a bit on the theme, Wonderpants and I'll start a thread if you like.<br>"My personal view is that, considering DaveScot's generally perceived blog persona, I have to admit that he hasn't been (on this site) quite the unmitigated disaster predicted. "<br>At a guess, it's because he can't duck or delete awkward subjects. I note from skimming through the threads though that he's been rather selective as to which ones he posts in, namely the ones that don't pose awkward ID questions. ;-)<br>Well, we can't torture a confession out of him. As Lenny points out frequently, an absence of an answer is in itself an answer. What about a thread from you, entitled " My awkard questions for DaveScot"?<br>I'm not sure how much meat can be put on the bones of a non-existent project, but here's my thought:<br>I would really like to hear about actual research projects that can be / are being done. Without knowing of any that are running currently, I'm not sure if it would be a good thread to start, but maybe you could ask for ideas.<br>It could be a thread dedicated to lab experiments. If X is designed, we will find Y. Here's how we find Y in the lab. Then we watch for the landslide of X and Y that get suggested and, of course, the methods that actually find these things.<br>Why would they start posting it now, though, after years of keeping it secret?<br>JAD: I have no respect for you or your cronies from Psnda's Thumb.<br>Which raises the obvious question of why you hang around in forums like this. Why not submit your work to a technical journal where real scientists will read it?<br>Well, unless and until Wonderpants or Blipey want to expand on it, I have framed a thread along the line suggested.<br>I think a good thread would be "Place A Vote For or Against the Banning of Professor Emeritus John Davison".<br>I invade the ephemeral meaningless world of cyberdom for amusement on the outside chance that I might find a rational mind once in a while, one like johndarius for example. Mostly I encounter mentally impaired ideologues with IQs in the room temperature range or hostile, rabid, certifiably deranged schizophrenic sociopaths like Spravid Dinger. This particular blog seems to be blessed with both varieties.<br>Naturally -<br>I love it so!<br>I'll try and think of something tomorrow.<br>Been watching the footie tonight.<br>Props to France for winning aghainst Spain, Alan.<br>Ah, the World Cup. Something else Mrs Fox and I disagree on. Yes there would have been a few glum faces at work tomorrow. Now if only France can beat Brazil, and England beat Portugal.<br>JAD, you didn't answer my second question: Why not submit your work to a technical journal where real scientists will read it?<br>Why would real scientists want to read the nonsensical ramblings of a pseudoscientist?<br>Give me a shout if you need some "help."<br>Naturally -<br>I love it so!<br>But JAD won't publish in a scientific journal any longer. According to his second post, we can assume that he visits Nature's website "for amusement on the outside chance that [he] might find a rational mind once in a while, one like a [creationist] for example. Mostly [he] encounters mentally impaired [evolutionary biologists] "<br>I can't think of a single great scientist who wouldn't describe himself as a creationist, not one. Can anyone?<br>I love it so!<br>Democritus, Sagan, Darwin, Edison, Feynman, Curie, just to name a handful.<br>Of course, they never managed to publish in Rivista... [snicker]<br>Feynman once described scientific discovery as a religious experience. I agree entirely as I have had the same experience. That anyone could describe Darwin as a scientist is unthinkable. I didn't know that about Curie and tend not to accept it without some documentation.<br>I love it so!<br>What a sad little weasel you are. Feynman was an avowed atheist. Curie was raised Catholic but became an atheist on the death of her mother. Darwin was 100 times the scientist you are.<br>Creationism is all but dead among true scientists; critical inquiry is poison to that superstitious twaddle.</code> | <code>What is the author's personal view on DaveScot's blog persona?</code> |
| <code>Age reduction Academic atmosphere Beef tendon bottom Straight buckle low-heel cowhide Lefu shoes Mary Jane shoes Spring and summer Women's shoes 0.73<br>ins Chaopai shoes Women's Shoes Academic atmosphere Versatile Graffiti Frenulum gym shoes Harajuku leisure time Hip hop jointly skate shoes<br>Air force one Men's shoes Low Gang summer skate shoes student Korean version Versatile leisure time gym shoes female Reflection Little white shoes<br>autumn Clover ozweego Daddy shoes Jackson Yi Same men and women Reflection motion Running shoes EE6999<br>Retro Britain Square head Frenulum Color matching motion Casual shoes 2021 new pattern Versatile Flat bottom Elastic band Little white shoes female<br>Thick bottom British style Small leather shoes Women's shoes 2021 new pattern Big square head Spring and Autumn Lefu Autumn shoes black Single shoes<br>U.S.A quality goods Jeffrey Campbell temperament crude high-heeled dollskill Buckles Low top shoes female widow<br>quality goods Clover ozweego Black Warrior Dad Running shoes Night Walker Retro Men's Shoes Reflection increase Women's Shoes tide<br>Internet celebrity Daddy shoes female 2021 summer new pattern ventilation comfortable leisure time gym shoes Retro Thick bottom increase Single shoes tide<br>Sao Fen Paris Daddy shoes Three generations combination increase Thick bottom ins tide Single shoes Women's Shoes leisure time motion track3.0<br>Paris Home B Daddy shoes one three generation triple s Thick bottom increase men and women lovers leisure time motion Fashion shoes Dirty shoes<br>U.S.A quality goods Jeffrey Campbell temperament crude high-heeled dollskill Buckles Low top shoes female widow<br>D1G New products anniversary Graffiti high-heeled shoes Internet celebrity Show Sharp point Fine heel Women's shoes Europe Versatile Retro Women's Shoes<br>2021 Autumn and winter new pattern Low Gang Single shoes female genuine leather Flat bottom Frenulum Color matching motion Casual shoes male skate shoes tide<br>Little white shoes female Josiny Spring and summer 2021 new pattern Korean version Versatile Leisure fashion ventilation student Flat bottom gym shoes<br>European goods Forrest Gump Daddy shoes female tide 2021 autumn new pattern Small pretty waist gym shoes Frenulum Slope heel Single shoes Women's Shoes tide<br>【 goods in stock 】 devil sisters Sheep puff Lolita original Halloween Thick bottom Women's Shoes hottie high-heeled Women's Shoes<br>Zhou Yangqing Same 2021 Spring and summer new pattern Thick bottom Shoe of sponge cake motion leisure time lovers P family Daddy shoes female ins tide<br>20 new pattern Internet celebrity Sharp point Single shoes female high-heeled genuine leather Fine heel Shallow mouth sexy Bridesmaid Women's Shoes Wedding shoes 6cm 10cm</code> | <code>What type of shoes are mentioned as being suitable for both men and women?</code> |
| <code>I just started a new blog on my ultralight gear. My gear list in all it's glory is located on: each item of gear, I'm writing an in-depth review for the item and how we have used it. Would love to get feedback and the site and our gear and/or comments from people on how we can fine tune.Currently my wifes pack is 7.5 lbs base weight, and mine is 10.5 lbs.Thanks!-Brett<br>Edited by brettmarl on 09/09/2006 15:59:48 MDT.<br>Brett, Your BLOG looks good.You should put the size of your items where their is one such as pants, shoes, jacket etc. There is a golf like "handicap' for anyone that wears larger then size medium or size 9.5 shoe. Sure.I think you might recheck some of your math. Not sure but some totals look low. Don't trust the posted weightof gear, weigh it yourself if you haven't.Why is your pack list so heavy?<br>I agree, nice looking blog. Bill is right on listing the sizes, other than that....looks great!<br>Brett - nice list, and nice format!(One small typo: it currently says "Cloudburt" for the tent.)<br>Edited by slnsf on 09/09/2006 18:08:48 MDT.<br>Great site with good info. I'm trying to decide between the GoLite Infinity and Jam and I think after reading your blog, that the Jam should be plenty big.I'm interested to see what's in your first aid kit.Also, any issues with the water purification tablets? I currently use a MSR miniworks pump and I'm looking to lighten up...<br>At first I thought you might not be warm enough as I reviewed your North Cascade hike, then I recounted your layers. Very nice site! What I was a little confused about was the opening statement of getting four days of backpacking gear into a pack, yet at the bottom the food for two was estimated for three days. However I now understand that these are not mutually exclusive statements.<br>thanks for the feedback.i fixed the cloudburt typo (thanks), and the 4 vs. 3 days. ai also completed all my gear posts - including the innards of my first aid kit and my experiences with the MicroPUR tabs.the weights listed should be the ones that i weighed myself (unless, i've mis-typed in some areas)bill - you say to check my math with "Why is your pack list so heavy?". not sure what you are refering to here.great idea on including the sizes.<br>You must login to post.<br>MEMBERSHIP IS REQUIRED TO POST: You must be a Forum, Annual or Lifetime Member to post messages in the backpackinglight.com forums.<br>SUBSCRIBE NOW »</code> | <code>What are the base weights of the blogger's and his wife's packs?</code> |
* Loss: [<code>MultipleNegativesRankingLoss</code>](https://sbert.net/docs/package_reference/sentence_transformer/losses.html#multiplenegativesrankingloss) with these parameters:
```json
{
"scale": 20.0,
"similarity_fct": "cos_sim"
}
```
### Training Hyperparameters
#### Non-Default Hyperparameters
- `eval_strategy`: steps
- `per_device_train_batch_size`: 10
- `per_device_eval_batch_size`: 10
- `num_train_epochs`: 1
- `warmup_ratio`: 0.1
- `fp16`: True
- `batch_sampler`: no_duplicates
#### All Hyperparameters
<details><summary>Click to expand</summary>
- `overwrite_output_dir`: False
- `do_predict`: False
- `eval_strategy`: steps
- `prediction_loss_only`: True
- `per_device_train_batch_size`: 10
- `per_device_eval_batch_size`: 10
- `per_gpu_train_batch_size`: None
- `per_gpu_eval_batch_size`: None
- `gradient_accumulation_steps`: 1
- `eval_accumulation_steps`: None
- `learning_rate`: 5e-05
- `weight_decay`: 0.0
- `adam_beta1`: 0.9
- `adam_beta2`: 0.999
- `adam_epsilon`: 1e-08
- `max_grad_norm`: 1.0
- `num_train_epochs`: 1
- `max_steps`: -1
- `lr_scheduler_type`: linear
- `lr_scheduler_kwargs`: {}
- `warmup_ratio`: 0.1
- `warmup_steps`: 0
- `log_level`: passive
- `log_level_replica`: warning
- `log_on_each_node`: True
- `logging_nan_inf_filter`: True
- `save_safetensors`: True
- `save_on_each_node`: False
- `save_only_model`: False
- `restore_callback_states_from_checkpoint`: False
- `no_cuda`: False
- `use_cpu`: False
- `use_mps_device`: False
- `seed`: 42
- `data_seed`: None
- `jit_mode_eval`: False
- `use_ipex`: False
- `bf16`: False
- `fp16`: True
- `fp16_opt_level`: O1
- `half_precision_backend`: auto
- `bf16_full_eval`: False
- `fp16_full_eval`: False
- `tf32`: None
- `local_rank`: 0
- `ddp_backend`: None
- `tpu_num_cores`: None
- `tpu_metrics_debug`: False
- `debug`: []
- `dataloader_drop_last`: False
- `dataloader_num_workers`: 0
- `dataloader_prefetch_factor`: None
- `past_index`: -1
- `disable_tqdm`: False
- `remove_unused_columns`: True
- `label_names`: None
- `load_best_model_at_end`: False
- `ignore_data_skip`: False
- `fsdp`: []
- `fsdp_min_num_params`: 0
- `fsdp_config`: {'min_num_params': 0, 'xla': False, 'xla_fsdp_v2': False, 'xla_fsdp_grad_ckpt': False}
- `fsdp_transformer_layer_cls_to_wrap`: None
- `accelerator_config`: {'split_batches': False, 'dispatch_batches': None, 'even_batches': True, 'use_seedable_sampler': True, 'non_blocking': False, 'gradient_accumulation_kwargs': None}
- `deepspeed`: None
- `label_smoothing_factor`: 0.0
- `optim`: adamw_torch
- `optim_args`: None
- `adafactor`: False
- `group_by_length`: False
- `length_column_name`: length
- `ddp_find_unused_parameters`: None
- `ddp_bucket_cap_mb`: None
- `ddp_broadcast_buffers`: False
- `dataloader_pin_memory`: True
- `dataloader_persistent_workers`: False
- `skip_memory_metrics`: True
- `use_legacy_prediction_loop`: False
- `push_to_hub`: False
- `resume_from_checkpoint`: None
- `hub_model_id`: None
- `hub_strategy`: every_save
- `hub_private_repo`: False
- `hub_always_push`: False
- `gradient_checkpointing`: False
- `gradient_checkpointing_kwargs`: None
- `include_inputs_for_metrics`: False
- `eval_do_concat_batches`: True
- `fp16_backend`: auto
- `push_to_hub_model_id`: None
- `push_to_hub_organization`: None
- `mp_parameters`:
- `auto_find_batch_size`: False
- `full_determinism`: False
- `torchdynamo`: None
- `ray_scope`: last
- `ddp_timeout`: 1800
- `torch_compile`: False
- `torch_compile_backend`: None
- `torch_compile_mode`: None
- `dispatch_batches`: None
- `split_batches`: None
- `include_tokens_per_second`: False
- `include_num_input_tokens_seen`: False
- `neftune_noise_alpha`: None
- `optim_target_modules`: None
- `batch_eval_metrics`: False
- `eval_on_start`: False
- `batch_sampler`: no_duplicates
- `multi_dataset_batch_sampler`: proportional
</details>
### Training Logs
| Epoch | Step | Training Loss | loss |
|:------:|:----:|:-------------:|:------:|
| 0.0104 | 10 | 0.1231 | 0.0729 |
| 0.0208 | 20 | 0.0943 | 0.0501 |
| 0.0312 | 30 | 0.0432 | 0.0337 |
| 0.0417 | 40 | 0.1307 | 0.0247 |
| 0.0521 | 50 | 0.0191 | - |
| 0.1042 | 100 | 0.0558 | 0.0188 |
| 0.1562 | 150 | 0.0354 | - |
| 0.2083 | 200 | 0.0623 | 0.0178 |
| 0.2604 | 250 | 0.0692 | - |
| 0.3125 | 300 | 0.0428 | 0.0193 |
| 0.3646 | 350 | 0.0507 | - |
| 0.4167 | 400 | 0.0521 | 0.0250 |
| 0.4688 | 450 | 0.0352 | - |
| 0.5208 | 500 | 0.0285 | 0.0179 |
| 0.5729 | 550 | 0.0428 | - |
| 0.625 | 600 | 0.0315 | 0.0183 |
| 0.6771 | 650 | 0.0363 | - |
| 0.7292 | 700 | 0.0362 | 0.0167 |
| 0.7812 | 750 | 0.0288 | - |
| 0.8333 | 800 | 0.0211 | 0.0128 |
| 0.8854 | 850 | 0.0498 | - |
| 0.9375 | 900 | 0.0316 | 0.0138 |
| 0.9896 | 950 | 0.0336 | - |
### Framework Versions
- Python: 3.10.13
- Sentence Transformers: 3.0.1
- Transformers: 4.42.3
- PyTorch: 2.1.2
- Accelerate: 0.27.0
- Datasets: 2.20.0
- Tokenizers: 0.19.1
## Citation
### BibTeX
#### Sentence Transformers
```bibtex
@inproceedings{reimers-2019-sentence-bert,
title = "Sentence-BERT: Sentence Embeddings using Siamese BERT-Networks",
author = "Reimers, Nils and Gurevych, Iryna",
booktitle = "Proceedings of the 2019 Conference on Empirical Methods in Natural Language Processing",
month = "11",
year = "2019",
publisher = "Association for Computational Linguistics",
url = "https://arxiv.org/abs/1908.10084",
}
```
#### MultipleNegativesRankingLoss
```bibtex
@misc{henderson2017efficient,
title={Efficient Natural Language Response Suggestion for Smart Reply},
author={Matthew Henderson and Rami Al-Rfou and Brian Strope and Yun-hsuan Sung and Laszlo Lukacs and Ruiqi Guo and Sanjiv Kumar and Balint Miklos and Ray Kurzweil},
year={2017},
eprint={1705.00652},
archivePrefix={arXiv},
primaryClass={cs.CL}
}
```
<!--
## Glossary
*Clearly define terms in order to be accessible across audiences.*
-->
<!--
## Model Card Authors
*Lists the people who create the model card, providing recognition and accountability for the detailed work that goes into its construction.*
-->
<!--
## Model Card Contact
*Provides a way for people who have updates to the Model Card, suggestions, or questions, to contact the Model Card authors.*
--> | [
"TEXT_CLASSIFICATION"
] | Non_BioNLP |
MultiBertGunjanPatrick/multiberts-seed-1-1000k | MultiBertGunjanPatrick | null | [
"transformers",
"pytorch",
"bert",
"pretraining",
"exbert",
"multiberts",
"multiberts-seed-1",
"en",
"dataset:bookcorpus",
"dataset:wikipedia",
"arxiv:2106.16163",
"license:apache-2.0",
"endpoints_compatible",
"region:us"
] | 1,646,263,744,000 | 2021-10-04T05:00:47 | 102 | 0 | ---
datasets:
- bookcorpus
- wikipedia
language: en
license: apache-2.0
tags:
- exbert
- multiberts
- multiberts-seed-1
---
# MultiBERTs Seed 1 Checkpoint 1000k (uncased)
Seed 1 intermediate checkpoint 1000k MultiBERTs (pretrained BERT) model on English language using a masked language modeling (MLM) objective. It was introduced in
[this paper](https://arxiv.org/pdf/2106.16163.pdf) and first released in
[this repository](https://github.com/google-research/language/tree/master/language/multiberts). This is an intermediate checkpoint.
The final checkpoint can be found at [multiberts-seed-1](https://hf.co/multberts-seed-1). This model is uncased: it does not make a difference
between english and English.
Disclaimer: The team releasing MultiBERTs did not write a model card for this model so this model card has been written by [gchhablani](https://hf.co/gchhablani).
## Model description
MultiBERTs models are transformers model pretrained on a large corpus of English data in a self-supervised fashion. This means it
was pretrained on the raw texts only, with no humans labelling them in any way (which is why it can use lots of
publicly available data) with an automatic process to generate inputs and labels from those texts. More precisely, it
was pretrained with two objectives:
- Masked language modeling (MLM): taking a sentence, the model randomly masks 15% of the words in the input then run
the entire masked sentence through the model and has to predict the masked words. This is different from traditional
recurrent neural networks (RNNs) that usually see the words one after the other, or from autoregressive models like
GPT which internally mask the future tokens. It allows the model to learn a bidirectional representation of the
sentence.
- Next sentence prediction (NSP): the models concatenates two masked sentences as inputs during pretraining. Sometimes
they correspond to sentences that were next to each other in the original text, sometimes not. The model then has to
predict if the two sentences were following each other or not.
This way, the model learns an inner representation of the English language that can then be used to extract features
useful for downstream tasks: if you have a dataset of labeled sentences for instance, you can train a standard
classifier using the features produced by the MultiBERTs model as inputs.
## Intended uses & limitations
You can use the raw model for either masked language modeling or next sentence prediction, but it's mostly intended to
be fine-tuned on a downstream task. See the [model hub](https://huggingface.co/models?filter=multiberts) to look for
fine-tuned versions on a task that interests you.
Note that this model is primarily aimed at being fine-tuned on tasks that use the whole sentence (potentially masked)
to make decisions, such as sequence classification, token classification or question answering. For tasks such as text
generation you should look at model like GPT2.
### How to use
Here is how to use this model to get the features of a given text in PyTorch:
```python
from transformers import BertTokenizer, BertModel
tokenizer = BertTokenizer.from_pretrained('multiberts-seed-1-1000k')
model = BertModel.from_pretrained("multiberts-seed-1-1000k")
text = "Replace me by any text you'd like."
encoded_input = tokenizer(text, return_tensors='pt')
output = model(**encoded_input)
```
### Limitations and bias
Even if the training data used for this model could be characterized as fairly neutral, this model can have biased
predictions. This bias will also affect all fine-tuned versions of this model. For an understanding of bias of this particular
checkpoint, please try out this checkpoint with the snippet present in the [Limitation and bias section](https://huggingface.co/bert-base-uncased#limitations-and-bias) of the [bert-base-uncased](https://huggingface.co/bert-base-uncased) checkpoint.
## Training data
The MultiBERTs models were pretrained on [BookCorpus](https://yknzhu.wixsite.com/mbweb), a dataset consisting of 11,038
unpublished books and [English Wikipedia](https://en.wikipedia.org/wiki/English_Wikipedia) (excluding lists, tables and
headers).
## Training procedure
### Preprocessing
The texts are lowercased and tokenized using WordPiece and a vocabulary size of 30,000. The inputs of the model are
then of the form:
```
[CLS] Sentence A [SEP] Sentence B [SEP]
```
With probability 0.5, sentence A and sentence B correspond to two consecutive sentences in the original corpus and in
the other cases, it's another random sentence in the corpus. Note that what is considered a sentence here is a
consecutive span of text usually longer than a single sentence. The only constrain is that the result with the two
"sentences" has a combined length of less than 512 tokens.
The details of the masking procedure for each sentence are the following:
- 15% of the tokens are masked.
- In 80% of the cases, the masked tokens are replaced by `[MASK]`.
- In 10% of the cases, the masked tokens are replaced by a random token (different) from the one they replace.
- In the 10% remaining cases, the masked tokens are left as is.
### Pretraining
The full model was trained on 16 Cloud TPU v2 chips for two million steps with a batch size
of 256. The sequence length was set to 512 throughout. The optimizer
used is Adam with a learning rate of 1e-4, \\(\beta_{1} = 0.9\\) and \\(\beta_{2} = 0.999\\), a weight decay of 0.01,
learning rate warmup for 10,000 steps and linear decay of the learning rate after.
### BibTeX entry and citation info
```bibtex
@article{DBLP:journals/corr/abs-2106-16163,
author = {Thibault Sellam and
Steve Yadlowsky and
Jason Wei and
Naomi Saphra and
Alexander D'Amour and
Tal Linzen and
Jasmijn Bastings and
Iulia Turc and
Jacob Eisenstein and
Dipanjan Das and
Ian Tenney and
Ellie Pavlick},
title = {The MultiBERTs: {BERT} Reproductions for Robustness Analysis},
journal = {CoRR},
volume = {abs/2106.16163},
year = {2021},
url = {https://arxiv.org/abs/2106.16163},
eprinttype = {arXiv},
eprint = {2106.16163},
timestamp = {Mon, 05 Jul 2021 15:15:50 +0200},
biburl = {https://dblp.org/rec/journals/corr/abs-2106-16163.bib},
bibsource = {dblp computer science bibliography, https://dblp.org}
}
```
<a href="https://huggingface.co/exbert/?model=multiberts">
<img width="300px" src="https://cdn-media.huggingface.co/exbert/button.png">
</a>
| [
"QUESTION_ANSWERING"
] | Non_BioNLP |
Kaludi/eurekaQA-model | Kaludi | question-answering | [
"transformers",
"pytorch",
"distilbert",
"question-answering",
"qna",
"question-answer",
"en",
"dataset:Kaludi/data-eurekaQA",
"endpoints_compatible",
"region:us"
] | 1,675,399,765,000 | 2023-02-04T01:37:06 | 135 | 2 | ---
datasets:
- Kaludi/data-eurekaQA
language: en
tags:
- qna
- question-answer
---
# EurekaQA
EurekaQA is an AI Question Answering model that uses advanced machine learning algorithms to analyze text data and automatically answer questions based on the information contained within. EurekaQA is an extractive model, meaning it selects the relevant information from a given text document to present as the answer to the question. This model can be used in a variety of applications, including customer service, virtual assistants, and information retrieval systems.
### Gradio
Tis model supports a [Gradio](https://github.com/gradio-app/gradio) Web UI to run the csgo-weapon-classification model:
[](https://huggingface.co/spaces/Kaludi/EurekaQA)
## Validation Metrics
- Loss: 0.523 | [
"QUESTION_ANSWERING"
] | Non_BioNLP |
srikarvar/fine_tuned_model_16 | srikarvar | sentence-similarity | [
"sentence-transformers",
"safetensors",
"bert",
"sentence-similarity",
"feature-extraction",
"generated_from_trainer",
"dataset_size:560",
"loss:MultipleNegativesRankingLoss",
"arxiv:1908.10084",
"arxiv:1705.00652",
"base_model:srikarvar/fine_tuned_model_5",
"base_model:finetune:srikarvar/fine_tuned_model_5",
"model-index",
"autotrain_compatible",
"text-embeddings-inference",
"endpoints_compatible",
"region:us"
] | 1,726,491,563,000 | 2024-09-16T12:59:54 | 5 | 0 | ---
base_model: srikarvar/fine_tuned_model_5
library_name: sentence-transformers
metrics:
- cosine_accuracy@1
- cosine_accuracy@3
- cosine_accuracy@5
- cosine_accuracy@10
- cosine_precision@1
- cosine_precision@3
- cosine_precision@5
- cosine_precision@10
- cosine_recall@1
- cosine_recall@3
- cosine_recall@5
- cosine_recall@10
- cosine_ndcg@10
- cosine_mrr@10
- cosine_map@100
- dot_accuracy@1
- dot_accuracy@3
- dot_accuracy@5
- dot_accuracy@10
- dot_precision@1
- dot_precision@3
- dot_precision@5
- dot_precision@10
- dot_recall@1
- dot_recall@3
- dot_recall@5
- dot_recall@10
- dot_ndcg@10
- dot_mrr@10
- dot_map@100
pipeline_tag: sentence-similarity
tags:
- sentence-transformers
- sentence-similarity
- feature-extraction
- generated_from_trainer
- dataset_size:560
- loss:MultipleNegativesRankingLoss
widget:
- source_sentence: The main objective of the System Logs documentation is to demonstrate
how to utilize the 📋 Logs system to access and manipulate logs of any format or
type.
sentences:
- The purpose of the System Logs documentation is to provide information on how
to use the 📋 Logs system to store and work with logs of any format or type.
- The main difference between a ProductList and an InventoryList is that a ProductList
provides random access to the items, while an InventoryList updates progressively
as you browse the list.
- The most recommended way to clean kitchen surfaces is with a microfiber cloth.
- source_sentence: The main repository page can be accessed by clicking on the link.
sentences:
- The `to_absolute` function translates a `TaskInstruction` instance into a list
of absolute instructions, which are then combined together.
- No, ACTIVATE_X doesn't exist in version 3.0.
- It exists in the main repository. You can click on the provided link to redirect
to the main repository page.
- source_sentence: The documentation does not specify what type of value is returned
by the `fetch_data` function.
sentences:
- The purpose of this document is to provide documentation for the Plugin library.
- The return type of the `fetch_data` function is not specified in the current API
documentation.
- 'The `from_dictionary` function takes the following parameters:
- `data` (Union[dict, Mapping]): A mapping of keys to values or Python objects.
- `schema` (Schema, optional): If not passed, will be inferred from the Mapping
values.
- `metadata` (Union[dict, Mapping], optional): Optional metadata for the schema
(if inferred).'
- source_sentence: The aim of the Gardening.Fertilization class is to carry out the
application of fertilizers in the garden.
sentences:
- The `iterate_folder` function iterates over files within a folder.
- The purpose of the Gardening.Fertilization class is to apply fertilizers in the
garden.
- It may be more convenient for the reader to not specify a section when browsing
a collection because a suitable default may be an aggregated section that displays
all genres if the reader doesn’t request a particular one.
- source_sentence: Two kinds of cooking methods exist, baking and frying.
sentences:
- There are two types of cooking methods, baking and frying.
- The purpose of the given recipe is to provide instructions for making lasagna.
- To get the full path to the locally extracted file, we need to join the path of
the directory where the archive is extracted to and the relative image file path.
model-index:
- name: SentenceTransformer based on srikarvar/fine_tuned_model_5
results:
- task:
type: information-retrieval
name: Information Retrieval
dataset:
name: e5 cogcache small refined
type: e5-cogcache-small-refined
metrics:
- type: cosine_accuracy@1
value: 0.9642857142857143
name: Cosine Accuracy@1
- type: cosine_accuracy@3
value: 1.0
name: Cosine Accuracy@3
- type: cosine_accuracy@5
value: 1.0
name: Cosine Accuracy@5
- type: cosine_accuracy@10
value: 1.0
name: Cosine Accuracy@10
- type: cosine_precision@1
value: 0.9642857142857143
name: Cosine Precision@1
- type: cosine_precision@3
value: 0.3333333333333333
name: Cosine Precision@3
- type: cosine_precision@5
value: 0.19999999999999998
name: Cosine Precision@5
- type: cosine_precision@10
value: 0.09999999999999999
name: Cosine Precision@10
- type: cosine_recall@1
value: 0.9642857142857143
name: Cosine Recall@1
- type: cosine_recall@3
value: 1.0
name: Cosine Recall@3
- type: cosine_recall@5
value: 1.0
name: Cosine Recall@5
- type: cosine_recall@10
value: 1.0
name: Cosine Recall@10
- type: cosine_ndcg@10
value: 0.9844808884566332
name: Cosine Ndcg@10
- type: cosine_mrr@10
value: 0.9791666666666666
name: Cosine Mrr@10
- type: cosine_map@100
value: 0.9791666666666667
name: Cosine Map@100
- type: dot_accuracy@1
value: 0.9642857142857143
name: Dot Accuracy@1
- type: dot_accuracy@3
value: 1.0
name: Dot Accuracy@3
- type: dot_accuracy@5
value: 1.0
name: Dot Accuracy@5
- type: dot_accuracy@10
value: 1.0
name: Dot Accuracy@10
- type: dot_precision@1
value: 0.9642857142857143
name: Dot Precision@1
- type: dot_precision@3
value: 0.3333333333333333
name: Dot Precision@3
- type: dot_precision@5
value: 0.19999999999999998
name: Dot Precision@5
- type: dot_precision@10
value: 0.09999999999999999
name: Dot Precision@10
- type: dot_recall@1
value: 0.9642857142857143
name: Dot Recall@1
- type: dot_recall@3
value: 1.0
name: Dot Recall@3
- type: dot_recall@5
value: 1.0
name: Dot Recall@5
- type: dot_recall@10
value: 1.0
name: Dot Recall@10
- type: dot_ndcg@10
value: 0.9844808884566332
name: Dot Ndcg@10
- type: dot_mrr@10
value: 0.9791666666666666
name: Dot Mrr@10
- type: dot_map@100
value: 0.9791666666666667
name: Dot Map@100
- type: cosine_accuracy@1
value: 0.9642857142857143
name: Cosine Accuracy@1
- type: cosine_accuracy@3
value: 1.0
name: Cosine Accuracy@3
- type: cosine_accuracy@5
value: 1.0
name: Cosine Accuracy@5
- type: cosine_accuracy@10
value: 1.0
name: Cosine Accuracy@10
- type: cosine_precision@1
value: 0.9642857142857143
name: Cosine Precision@1
- type: cosine_precision@3
value: 0.3333333333333333
name: Cosine Precision@3
- type: cosine_precision@5
value: 0.19999999999999998
name: Cosine Precision@5
- type: cosine_precision@10
value: 0.09999999999999999
name: Cosine Precision@10
- type: cosine_recall@1
value: 0.9642857142857143
name: Cosine Recall@1
- type: cosine_recall@3
value: 1.0
name: Cosine Recall@3
- type: cosine_recall@5
value: 1.0
name: Cosine Recall@5
- type: cosine_recall@10
value: 1.0
name: Cosine Recall@10
- type: cosine_ndcg@10
value: 0.9844808884566332
name: Cosine Ndcg@10
- type: cosine_mrr@10
value: 0.9791666666666666
name: Cosine Mrr@10
- type: cosine_map@100
value: 0.9791666666666667
name: Cosine Map@100
- type: dot_accuracy@1
value: 0.9642857142857143
name: Dot Accuracy@1
- type: dot_accuracy@3
value: 1.0
name: Dot Accuracy@3
- type: dot_accuracy@5
value: 1.0
name: Dot Accuracy@5
- type: dot_accuracy@10
value: 1.0
name: Dot Accuracy@10
- type: dot_precision@1
value: 0.9642857142857143
name: Dot Precision@1
- type: dot_precision@3
value: 0.3333333333333333
name: Dot Precision@3
- type: dot_precision@5
value: 0.19999999999999998
name: Dot Precision@5
- type: dot_precision@10
value: 0.09999999999999999
name: Dot Precision@10
- type: dot_recall@1
value: 0.9642857142857143
name: Dot Recall@1
- type: dot_recall@3
value: 1.0
name: Dot Recall@3
- type: dot_recall@5
value: 1.0
name: Dot Recall@5
- type: dot_recall@10
value: 1.0
name: Dot Recall@10
- type: dot_ndcg@10
value: 0.9844808884566332
name: Dot Ndcg@10
- type: dot_mrr@10
value: 0.9791666666666666
name: Dot Mrr@10
- type: dot_map@100
value: 0.9791666666666667
name: Dot Map@100
---
# SentenceTransformer based on srikarvar/fine_tuned_model_5
This is a [sentence-transformers](https://www.SBERT.net) model finetuned from [srikarvar/fine_tuned_model_5](https://huggingface.co/srikarvar/fine_tuned_model_5) on the json dataset. It maps sentences & paragraphs to a 384-dimensional dense vector space and can be used for semantic textual similarity, semantic search, paraphrase mining, text classification, clustering, and more.
## Model Details
### Model Description
- **Model Type:** Sentence Transformer
- **Base model:** [srikarvar/fine_tuned_model_5](https://huggingface.co/srikarvar/fine_tuned_model_5) <!-- at revision 4e4dc22ad09f760a0a35c55d14d2f89ebe2d2ff2 -->
- **Maximum Sequence Length:** 512 tokens
- **Output Dimensionality:** 384 tokens
- **Similarity Function:** Cosine Similarity
- **Training Dataset:**
- json
<!-- - **Language:** Unknown -->
<!-- - **License:** Unknown -->
### Model Sources
- **Documentation:** [Sentence Transformers Documentation](https://sbert.net)
- **Repository:** [Sentence Transformers on GitHub](https://github.com/UKPLab/sentence-transformers)
- **Hugging Face:** [Sentence Transformers on Hugging Face](https://huggingface.co/models?library=sentence-transformers)
### Full Model Architecture
```
SentenceTransformer(
(0): Transformer({'max_seq_length': 512, 'do_lower_case': False}) with Transformer model: BertModel
(1): Pooling({'word_embedding_dimension': 384, 'pooling_mode_cls_token': False, 'pooling_mode_mean_tokens': True, 'pooling_mode_max_tokens': False, 'pooling_mode_mean_sqrt_len_tokens': False, 'pooling_mode_weightedmean_tokens': False, 'pooling_mode_lasttoken': False, 'include_prompt': True})
(2): Normalize()
)
```
## Usage
### Direct Usage (Sentence Transformers)
First install the Sentence Transformers library:
```bash
pip install -U sentence-transformers
```
Then you can load this model and run inference.
```python
from sentence_transformers import SentenceTransformer
# Download from the 🤗 Hub
model = SentenceTransformer("srikarvar/fine_tuned_model_16")
# Run inference
sentences = [
'Two kinds of cooking methods exist, baking and frying.',
'There are two types of cooking methods, baking and frying.',
'The purpose of the given recipe is to provide instructions for making lasagna.',
]
embeddings = model.encode(sentences)
print(embeddings.shape)
# [3, 384]
# Get the similarity scores for the embeddings
similarities = model.similarity(embeddings, embeddings)
print(similarities.shape)
# [3, 3]
```
<!--
### Direct Usage (Transformers)
<details><summary>Click to see the direct usage in Transformers</summary>
</details>
-->
<!--
### Downstream Usage (Sentence Transformers)
You can finetune this model on your own dataset.
<details><summary>Click to expand</summary>
</details>
-->
<!--
### Out-of-Scope Use
*List how the model may foreseeably be misused and address what users ought not to do with the model.*
-->
## Evaluation
### Metrics
#### Information Retrieval
* Dataset: `e5-cogcache-small-refined`
* Evaluated with [<code>InformationRetrievalEvaluator</code>](https://sbert.net/docs/package_reference/sentence_transformer/evaluation.html#sentence_transformers.evaluation.InformationRetrievalEvaluator)
| Metric | Value |
|:--------------------|:-----------|
| cosine_accuracy@1 | 0.9643 |
| cosine_accuracy@3 | 1.0 |
| cosine_accuracy@5 | 1.0 |
| cosine_accuracy@10 | 1.0 |
| cosine_precision@1 | 0.9643 |
| cosine_precision@3 | 0.3333 |
| cosine_precision@5 | 0.2 |
| cosine_precision@10 | 0.1 |
| cosine_recall@1 | 0.9643 |
| cosine_recall@3 | 1.0 |
| cosine_recall@5 | 1.0 |
| cosine_recall@10 | 1.0 |
| cosine_ndcg@10 | 0.9845 |
| cosine_mrr@10 | 0.9792 |
| **cosine_map@100** | **0.9792** |
| dot_accuracy@1 | 0.9643 |
| dot_accuracy@3 | 1.0 |
| dot_accuracy@5 | 1.0 |
| dot_accuracy@10 | 1.0 |
| dot_precision@1 | 0.9643 |
| dot_precision@3 | 0.3333 |
| dot_precision@5 | 0.2 |
| dot_precision@10 | 0.1 |
| dot_recall@1 | 0.9643 |
| dot_recall@3 | 1.0 |
| dot_recall@5 | 1.0 |
| dot_recall@10 | 1.0 |
| dot_ndcg@10 | 0.9845 |
| dot_mrr@10 | 0.9792 |
| dot_map@100 | 0.9792 |
#### Information Retrieval
* Dataset: `e5-cogcache-small-refined`
* Evaluated with [<code>InformationRetrievalEvaluator</code>](https://sbert.net/docs/package_reference/sentence_transformer/evaluation.html#sentence_transformers.evaluation.InformationRetrievalEvaluator)
| Metric | Value |
|:--------------------|:-----------|
| cosine_accuracy@1 | 0.9643 |
| cosine_accuracy@3 | 1.0 |
| cosine_accuracy@5 | 1.0 |
| cosine_accuracy@10 | 1.0 |
| cosine_precision@1 | 0.9643 |
| cosine_precision@3 | 0.3333 |
| cosine_precision@5 | 0.2 |
| cosine_precision@10 | 0.1 |
| cosine_recall@1 | 0.9643 |
| cosine_recall@3 | 1.0 |
| cosine_recall@5 | 1.0 |
| cosine_recall@10 | 1.0 |
| cosine_ndcg@10 | 0.9845 |
| cosine_mrr@10 | 0.9792 |
| **cosine_map@100** | **0.9792** |
| dot_accuracy@1 | 0.9643 |
| dot_accuracy@3 | 1.0 |
| dot_accuracy@5 | 1.0 |
| dot_accuracy@10 | 1.0 |
| dot_precision@1 | 0.9643 |
| dot_precision@3 | 0.3333 |
| dot_precision@5 | 0.2 |
| dot_precision@10 | 0.1 |
| dot_recall@1 | 0.9643 |
| dot_recall@3 | 1.0 |
| dot_recall@5 | 1.0 |
| dot_recall@10 | 1.0 |
| dot_ndcg@10 | 0.9845 |
| dot_mrr@10 | 0.9792 |
| dot_map@100 | 0.9792 |
<!--
## Bias, Risks and Limitations
*What are the known or foreseeable issues stemming from this model? You could also flag here known failure cases or weaknesses of the model.*
-->
<!--
### Recommendations
*What are recommendations with respect to the foreseeable issues? For example, filtering explicit content.*
-->
## Training Details
### Training Dataset
#### json
* Dataset: json
* Size: 560 training samples
* Columns: <code>anchor</code> and <code>positive</code>
* Approximate statistics based on the first 560 samples:
| | anchor | positive |
|:--------|:----------------------------------------------------------------------------------|:----------------------------------------------------------------------------------|
| type | string | string |
| details | <ul><li>min: 9 tokens</li><li>mean: 30.72 tokens</li><li>max: 98 tokens</li></ul> | <ul><li>min: 8 tokens</li><li>mean: 30.52 tokens</li><li>max: 98 tokens</li></ul> |
* Samples:
| anchor | positive |
|:-----------------------------------------------------------------------------------------------------------------------------------|:---------------------------------------------------------------------------------------------------------------------------|
| <code>The function assists in the preprocessing of the whole module in one go.</code> | <code>The function helps preprocess your entire module at once.</code> |
| <code>The `num_threads` parameter determines the quantity of threads used when downloading and processing the data locally.</code> | <code>The `num_threads` parameter specifies the number of threads when downloading and processing the data locally.</code> |
| <code>The `map()` function can be used to apply transformations to all elements of a model.</code> | <code>The `map()` function can apply transforms over an entire model.</code> |
* Loss: [<code>MultipleNegativesRankingLoss</code>](https://sbert.net/docs/package_reference/sentence_transformer/losses.html#multiplenegativesrankingloss) with these parameters:
```json
{
"scale": 20.0,
"similarity_fct": "cos_sim"
}
```
### Training Hyperparameters
#### Non-Default Hyperparameters
- `eval_strategy`: epoch
- `per_device_train_batch_size`: 16
- `per_device_eval_batch_size`: 16
- `num_train_epochs`: 5
- `warmup_ratio`: 0.1
- `batch_sampler`: no_duplicates
#### All Hyperparameters
<details><summary>Click to expand</summary>
- `overwrite_output_dir`: False
- `do_predict`: False
- `eval_strategy`: epoch
- `prediction_loss_only`: True
- `per_device_train_batch_size`: 16
- `per_device_eval_batch_size`: 16
- `per_gpu_train_batch_size`: None
- `per_gpu_eval_batch_size`: None
- `gradient_accumulation_steps`: 1
- `eval_accumulation_steps`: None
- `learning_rate`: 5e-05
- `weight_decay`: 0.0
- `adam_beta1`: 0.9
- `adam_beta2`: 0.999
- `adam_epsilon`: 1e-08
- `max_grad_norm`: 1.0
- `num_train_epochs`: 5
- `max_steps`: -1
- `lr_scheduler_type`: linear
- `lr_scheduler_kwargs`: {}
- `warmup_ratio`: 0.1
- `warmup_steps`: 0
- `log_level`: passive
- `log_level_replica`: warning
- `log_on_each_node`: True
- `logging_nan_inf_filter`: True
- `save_safetensors`: True
- `save_on_each_node`: False
- `save_only_model`: False
- `restore_callback_states_from_checkpoint`: False
- `no_cuda`: False
- `use_cpu`: False
- `use_mps_device`: False
- `seed`: 42
- `data_seed`: None
- `jit_mode_eval`: False
- `use_ipex`: False
- `bf16`: False
- `fp16`: False
- `fp16_opt_level`: O1
- `half_precision_backend`: auto
- `bf16_full_eval`: False
- `fp16_full_eval`: False
- `tf32`: None
- `local_rank`: 0
- `ddp_backend`: None
- `tpu_num_cores`: None
- `tpu_metrics_debug`: False
- `debug`: []
- `dataloader_drop_last`: False
- `dataloader_num_workers`: 0
- `dataloader_prefetch_factor`: None
- `past_index`: -1
- `disable_tqdm`: False
- `remove_unused_columns`: True
- `label_names`: None
- `load_best_model_at_end`: False
- `ignore_data_skip`: False
- `fsdp`: []
- `fsdp_min_num_params`: 0
- `fsdp_config`: {'min_num_params': 0, 'xla': False, 'xla_fsdp_v2': False, 'xla_fsdp_grad_ckpt': False}
- `fsdp_transformer_layer_cls_to_wrap`: None
- `accelerator_config`: {'split_batches': False, 'dispatch_batches': None, 'even_batches': True, 'use_seedable_sampler': True, 'non_blocking': False, 'gradient_accumulation_kwargs': None}
- `deepspeed`: None
- `label_smoothing_factor`: 0.0
- `optim`: adamw_torch
- `optim_args`: None
- `adafactor`: False
- `group_by_length`: False
- `length_column_name`: length
- `ddp_find_unused_parameters`: None
- `ddp_bucket_cap_mb`: None
- `ddp_broadcast_buffers`: False
- `dataloader_pin_memory`: True
- `dataloader_persistent_workers`: False
- `skip_memory_metrics`: True
- `use_legacy_prediction_loop`: False
- `push_to_hub`: False
- `resume_from_checkpoint`: None
- `hub_model_id`: None
- `hub_strategy`: every_save
- `hub_private_repo`: False
- `hub_always_push`: False
- `gradient_checkpointing`: False
- `gradient_checkpointing_kwargs`: None
- `include_inputs_for_metrics`: False
- `eval_do_concat_batches`: True
- `fp16_backend`: auto
- `push_to_hub_model_id`: None
- `push_to_hub_organization`: None
- `mp_parameters`:
- `auto_find_batch_size`: False
- `full_determinism`: False
- `torchdynamo`: None
- `ray_scope`: last
- `ddp_timeout`: 1800
- `torch_compile`: False
- `torch_compile_backend`: None
- `torch_compile_mode`: None
- `dispatch_batches`: None
- `split_batches`: None
- `include_tokens_per_second`: False
- `include_num_input_tokens_seen`: False
- `neftune_noise_alpha`: None
- `optim_target_modules`: None
- `batch_eval_metrics`: False
- `batch_sampler`: no_duplicates
- `multi_dataset_batch_sampler`: proportional
</details>
### Training Logs
| Epoch | Step | Training Loss | e5-cogcache-small-refined_cosine_map@100 |
|:------:|:----:|:-------------:|:----------------------------------------:|
| 0 | 0 | - | 0.9702 |
| 0.3125 | 10 | 0.0171 | - |
| 0.625 | 20 | 0.0042 | - |
| 0.9375 | 30 | 0.0011 | - |
| 1.0 | 32 | - | 0.9792 |
| 1.25 | 40 | 0.0062 | - |
| 1.5625 | 50 | 0.0001 | - |
| 1.875 | 60 | 0.0002 | - |
| 2.0 | 64 | - | 0.9792 |
| 2.1875 | 70 | 0.0001 | - |
| 2.5 | 80 | 0.0005 | - |
| 2.8125 | 90 | 0.0001 | - |
| 3.0 | 96 | - | 0.9792 |
| 3.125 | 100 | 0.0001 | - |
| 3.4375 | 110 | 0.0002 | - |
| 3.75 | 120 | 0.0001 | - |
| 4.0 | 128 | - | 0.9792 |
| 4.0625 | 130 | 0.0001 | - |
| 4.375 | 140 | 0.0 | - |
| 4.6875 | 150 | 0.0001 | - |
| 5.0 | 160 | 0.0001 | 0.9792 |
### Framework Versions
- Python: 3.10.12
- Sentence Transformers: 3.1.0
- Transformers: 4.41.2
- PyTorch: 2.1.2+cu121
- Accelerate: 0.34.2
- Datasets: 2.19.1
- Tokenizers: 0.19.1
## Citation
### BibTeX
#### Sentence Transformers
```bibtex
@inproceedings{reimers-2019-sentence-bert,
title = "Sentence-BERT: Sentence Embeddings using Siamese BERT-Networks",
author = "Reimers, Nils and Gurevych, Iryna",
booktitle = "Proceedings of the 2019 Conference on Empirical Methods in Natural Language Processing",
month = "11",
year = "2019",
publisher = "Association for Computational Linguistics",
url = "https://arxiv.org/abs/1908.10084",
}
```
#### MultipleNegativesRankingLoss
```bibtex
@misc{henderson2017efficient,
title={Efficient Natural Language Response Suggestion for Smart Reply},
author={Matthew Henderson and Rami Al-Rfou and Brian Strope and Yun-hsuan Sung and Laszlo Lukacs and Ruiqi Guo and Sanjiv Kumar and Balint Miklos and Ray Kurzweil},
year={2017},
eprint={1705.00652},
archivePrefix={arXiv},
primaryClass={cs.CL}
}
```
<!--
## Glossary
*Clearly define terms in order to be accessible across audiences.*
-->
<!--
## Model Card Authors
*Lists the people who create the model card, providing recognition and accountability for the detailed work that goes into its construction.*
-->
<!--
## Model Card Contact
*Provides a way for people who have updates to the Model Card, suggestions, or questions, to contact the Model Card authors.*
--> | [
"TEXT_CLASSIFICATION"
] | Non_BioNLP |
tomaarsen/mpnet-base-all-nli-triplet | tomaarsen | sentence-similarity | [
"sentence-transformers",
"safetensors",
"mpnet",
"sentence-similarity",
"feature-extraction",
"100K<n<1M",
"loss:MultipleNegativesRankingLoss",
"en",
"arxiv:1908.10084",
"arxiv:1705.00652",
"base_model:microsoft/mpnet-base",
"base_model:finetune:microsoft/mpnet-base",
"license:apache-2.0",
"model-index",
"co2_eq_emissions",
"autotrain_compatible",
"endpoints_compatible",
"region:us"
] | 1,716,807,443,000 | 2024-06-04T19:14:30 | 20 | 1 | ---
base_model: microsoft/mpnet-base
language:
- en
library_name: sentence-transformers
license: apache-2.0
metrics:
- cosine_accuracy
- dot_accuracy
- manhattan_accuracy
- euclidean_accuracy
- max_accuracy
pipeline_tag: sentence-similarity
tags:
- sentence-transformers
- sentence-similarity
- feature-extraction
- 100K<n<1M
- loss:MultipleNegativesRankingLoss
widget:
- source_sentence: The strangely dressed guys, one wearing an orange wig, sunglasses
with peace signs, and a karate costume with an orannge belt, another wearing a
curly blue wig, heart shaped sunglasses, and a karate outfit painted with leaves,
and the third wearing pink underwear, a black afro, and giant sunglasses.
sentences:
- A blonde female is reaching into a golf hole while holding two golf balls.
- There are people wearing outfits.
- The people are naked.
- source_sentence: A group of children playing and having a good time.
sentences:
- The kids are together.
- The children are reading books.
- People are pointing at a Middle-aged woman.
- source_sentence: Three children dressed in winter clothes are walking through the
woods while pushing cargo along.
sentences:
- A woman is sitting.
- Three childre are dressed in summer clothes.
- Three children are dressed in winter clothes.
- source_sentence: A young child is enjoying the water and rock scenery with their
dog.
sentences:
- The child and dog are enjoying some fresh air.
- The teenage boy is taking his cat for a walk beside the water.
- A lady in blue has birds around her.
- source_sentence: 'Boca da Corrida Encumeada (moderate; 5 hours): views of Curral
das Freiras and the valley of Ribeiro do Poco.'
sentences:
- 'Boca da Corrida Encumeada is a moderate text that takes 5 hours to complete. '
- This chapter is in the advance category.
- I think it is something that we need.
co2_eq_emissions:
emissions: 118.81134392463773
energy_consumed: 0.30566177669432554
source: codecarbon
training_type: fine-tuning
on_cloud: false
cpu_model: 13th Gen Intel(R) Core(TM) i7-13700K
ram_total_size: 31.777088165283203
hours_used: 1.661
hardware_used: 1 x NVIDIA GeForce RTX 3090
model-index:
- name: MPNet base trained on AllNLI triplets
results:
- task:
type: triplet
name: Triplet
dataset:
name: all nli dev
type: all-nli-dev
metrics:
- type: cosine_accuracy
value: 0.9003645200486027
name: Cosine Accuracy
- type: dot_accuracy
value: 0.09705346294046173
name: Dot Accuracy
- type: manhattan_accuracy
value: 0.8968712029161604
name: Manhattan Accuracy
- type: euclidean_accuracy
value: 0.8974787363304981
name: Euclidean Accuracy
- type: max_accuracy
value: 0.9003645200486027
name: Max Accuracy
- task:
type: triplet
name: Triplet
dataset:
name: all nli test
type: all-nli-test
metrics:
- type: cosine_accuracy
value: 0.9149644424269935
name: Cosine Accuracy
- type: dot_accuracy
value: 0.08564079285822364
name: Dot Accuracy
- type: manhattan_accuracy
value: 0.911484339536995
name: Manhattan Accuracy
- type: euclidean_accuracy
value: 0.9134513542139506
name: Euclidean Accuracy
- type: max_accuracy
value: 0.9149644424269935
name: Max Accuracy
---
# MPNet base trained on AllNLI triplets
This is a [sentence-transformers](https://www.SBERT.net) model finetuned from [microsoft/mpnet-base](https://huggingface.co/microsoft/mpnet-base) on the [sentence-transformers/all-nli](https://huggingface.co/datasets/sentence-transformers/all-nli) dataset. It maps sentences & paragraphs to a 768-dimensional dense vector space and can be used for semantic textual similarity, semantic search, paraphrase mining, text classification, clustering, and more.
## Model Details
### Model Description
- **Model Type:** Sentence Transformer
- **Base model:** [microsoft/mpnet-base](https://huggingface.co/microsoft/mpnet-base) <!-- at revision 6996ce1e91bd2a9c7d7f61daec37463394f73f09 -->
- **Maximum Sequence Length:** 512 tokens
- **Output Dimensionality:** 768 tokens
- **Similarity Function:** Cosine Similarity
- **Training Dataset:**
- [sentence-transformers/all-nli](https://huggingface.co/datasets/sentence-transformers/all-nli)
- **Language:** en
- **License:** apache-2.0
### Model Sources
- **Documentation:** [Sentence Transformers Documentation](https://sbert.net)
- **Repository:** [Sentence Transformers on GitHub](https://github.com/UKPLab/sentence-transformers)
- **Hugging Face:** [Sentence Transformers on Hugging Face](https://huggingface.co/models?library=sentence-transformers)
### Full Model Architecture
```
SentenceTransformer(
(0): Transformer({'max_seq_length': 512, 'do_lower_case': False}) with Transformer model: MPNetModel
(1): Pooling({'word_embedding_dimension': 768, 'pooling_mode_cls_token': False, 'pooling_mode_mean_tokens': True, 'pooling_mode_max_tokens': False, 'pooling_mode_mean_sqrt_len_tokens': False, 'pooling_mode_weightedmean_tokens': False, 'pooling_mode_lasttoken': False, 'include_prompt': True})
)
```
## Usage
### Direct Usage (Sentence Transformers)
First install the Sentence Transformers library:
```bash
pip install -U sentence-transformers
```
Then you can load this model and run inference.
```python
from sentence_transformers import SentenceTransformer
# Download from the 🤗 Hub
model = SentenceTransformer("tomaarsen/mpnet-base-all-nli-triplet")
# Run inference
sentences = [
'Then he ran.',
'The people are running.',
'The man is on his bike.',
]
embeddings = model.encode(sentences)
print(embeddings.shape)
# [3, 768]
# Get the similarity scores for the embeddings
similarities = model.similarity(embeddings, embeddings)
print(similarities.shape)
# [3, 3]
```
<!--
### Direct Usage (Transformers)
<details><summary>Click to see the direct usage in Transformers</summary>
</details>
-->
<!--
### Downstream Usage (Sentence Transformers)
You can finetune this model on your own dataset.
<details><summary>Click to expand</summary>
</details>
-->
<!--
### Out-of-Scope Use
*List how the model may foreseeably be misused and address what users ought not to do with the model.*
-->
## Evaluation
### Metrics
#### Triplet
* Dataset: `all-nli-dev`
* Evaluated with [<code>TripletEvaluator</code>](https://sbert.net/docs/package_reference/sentence_transformer/evaluation.html#sentence_transformers.evaluation.TripletEvaluator)
| Metric | Value |
|:-------------------|:-----------|
| cosine_accuracy | 0.9004 |
| dot_accuracy | 0.0971 |
| manhattan_accuracy | 0.8969 |
| euclidean_accuracy | 0.8975 |
| **max_accuracy** | **0.9004** |
#### Triplet
* Dataset: `all-nli-test`
* Evaluated with [<code>TripletEvaluator</code>](https://sbert.net/docs/package_reference/sentence_transformer/evaluation.html#sentence_transformers.evaluation.TripletEvaluator)
| Metric | Value |
|:-------------------|:----------|
| cosine_accuracy | 0.915 |
| dot_accuracy | 0.0856 |
| manhattan_accuracy | 0.9115 |
| euclidean_accuracy | 0.9135 |
| **max_accuracy** | **0.915** |
<!--
## Bias, Risks and Limitations
*What are the known or foreseeable issues stemming from this model? You could also flag here known failure cases or weaknesses of the model.*
-->
<!--
### Recommendations
*What are recommendations with respect to the foreseeable issues? For example, filtering explicit content.*
-->
## Training Details
### Training Dataset
#### sentence-transformers/all-nli
* Dataset: [sentence-transformers/all-nli](https://huggingface.co/datasets/sentence-transformers/all-nli) at [d482672](https://huggingface.co/datasets/sentence-transformers/all-nli/tree/d482672c8e74ce18da116f430137434ba2e52fab)
* Size: 100,000 training samples
* Columns: <code>anchor</code>, <code>positive</code>, and <code>negative</code>
* Approximate statistics based on the first 1000 samples:
| | anchor | positive | negative |
|:--------|:----------------------------------------------------------------------------------|:----------------------------------------------------------------------------------|:---------------------------------------------------------------------------------|
| type | string | string | string |
| details | <ul><li>min: 7 tokens</li><li>mean: 10.46 tokens</li><li>max: 46 tokens</li></ul> | <ul><li>min: 6 tokens</li><li>mean: 12.81 tokens</li><li>max: 40 tokens</li></ul> | <ul><li>min: 5 tokens</li><li>mean: 13.4 tokens</li><li>max: 50 tokens</li></ul> |
* Samples:
| anchor | positive | negative |
|:---------------------------------------------------------------------------|:-------------------------------------------------|:-----------------------------------------------------------|
| <code>A person on a horse jumps over a broken down airplane.</code> | <code>A person is outdoors, on a horse.</code> | <code>A person is at a diner, ordering an omelette.</code> |
| <code>Children smiling and waving at camera</code> | <code>There are children present</code> | <code>The kids are frowning</code> |
| <code>A boy is jumping on skateboard in the middle of a red bridge.</code> | <code>The boy does a skateboarding trick.</code> | <code>The boy skates down the sidewalk.</code> |
* Loss: [<code>MultipleNegativesRankingLoss</code>](https://sbert.net/docs/package_reference/sentence_transformer/losses.html#multiplenegativesrankingloss) with these parameters:
```json
{
"scale": 20.0,
"similarity_fct": "cos_sim"
}
```
### Evaluation Dataset
#### sentence-transformers/all-nli
* Dataset: [sentence-transformers/all-nli](https://huggingface.co/datasets/sentence-transformers/all-nli) at [d482672](https://huggingface.co/datasets/sentence-transformers/all-nli/tree/d482672c8e74ce18da116f430137434ba2e52fab)
* Size: 6,584 evaluation samples
* Columns: <code>anchor</code>, <code>positive</code>, and <code>negative</code>
* Approximate statistics based on the first 1000 samples:
| | anchor | positive | negative |
|:--------|:----------------------------------------------------------------------------------|:---------------------------------------------------------------------------------|:----------------------------------------------------------------------------------|
| type | string | string | string |
| details | <ul><li>min: 6 tokens</li><li>mean: 17.95 tokens</li><li>max: 63 tokens</li></ul> | <ul><li>min: 4 tokens</li><li>mean: 9.78 tokens</li><li>max: 29 tokens</li></ul> | <ul><li>min: 5 tokens</li><li>mean: 10.35 tokens</li><li>max: 29 tokens</li></ul> |
* Samples:
| anchor | positive | negative |
|:-------------------------------------------------------------------------------------------------------------------------------------------------------------------------------|:------------------------------------------------------------|:--------------------------------------------------------|
| <code>Two women are embracing while holding to go packages.</code> | <code>Two woman are holding packages.</code> | <code>The men are fighting outside a deli.</code> |
| <code>Two young children in blue jerseys, one with the number 9 and one with the number 2 are standing on wooden steps in a bathroom and washing their hands in a sink.</code> | <code>Two kids in numbered jerseys wash their hands.</code> | <code>Two kids in jackets walk to school.</code> |
| <code>A man selling donuts to a customer during a world exhibition event held in the city of Angeles</code> | <code>A man selling donuts to a customer.</code> | <code>A woman drinks her coffee in a small cafe.</code> |
* Loss: [<code>MultipleNegativesRankingLoss</code>](https://sbert.net/docs/package_reference/sentence_transformer/losses.html#multiplenegativesrankingloss) with these parameters:
```json
{
"scale": 20.0,
"similarity_fct": "cos_sim"
}
```
### Training Hyperparameters
#### Non-Default Hyperparameters
- `eval_strategy`: steps
- `per_device_train_batch_size`: 16
- `per_device_eval_batch_size`: 16
- `num_train_epochs`: 1
- `warmup_ratio`: 0.1
- `fp16`: True
- `batch_sampler`: no_duplicates
#### All Hyperparameters
<details><summary>Click to expand</summary>
- `overwrite_output_dir`: False
- `do_predict`: False
- `eval_strategy`: steps
- `prediction_loss_only`: True
- `per_device_train_batch_size`: 16
- `per_device_eval_batch_size`: 16
- `per_gpu_train_batch_size`: None
- `per_gpu_eval_batch_size`: None
- `gradient_accumulation_steps`: 1
- `eval_accumulation_steps`: None
- `learning_rate`: 5e-05
- `weight_decay`: 0.0
- `adam_beta1`: 0.9
- `adam_beta2`: 0.999
- `adam_epsilon`: 1e-08
- `max_grad_norm`: 1.0
- `num_train_epochs`: 1
- `max_steps`: -1
- `lr_scheduler_type`: linear
- `lr_scheduler_kwargs`: {}
- `warmup_ratio`: 0.1
- `warmup_steps`: 0
- `log_level`: passive
- `log_level_replica`: warning
- `log_on_each_node`: True
- `logging_nan_inf_filter`: True
- `save_safetensors`: True
- `save_on_each_node`: False
- `save_only_model`: False
- `restore_callback_states_from_checkpoint`: False
- `no_cuda`: False
- `use_cpu`: False
- `use_mps_device`: False
- `seed`: 42
- `data_seed`: None
- `jit_mode_eval`: False
- `use_ipex`: False
- `bf16`: False
- `fp16`: True
- `fp16_opt_level`: O1
- `half_precision_backend`: auto
- `bf16_full_eval`: False
- `fp16_full_eval`: False
- `tf32`: None
- `local_rank`: 0
- `ddp_backend`: None
- `tpu_num_cores`: None
- `tpu_metrics_debug`: False
- `debug`: []
- `dataloader_drop_last`: False
- `dataloader_num_workers`: 0
- `dataloader_prefetch_factor`: None
- `past_index`: -1
- `disable_tqdm`: False
- `remove_unused_columns`: True
- `label_names`: None
- `load_best_model_at_end`: False
- `ignore_data_skip`: False
- `fsdp`: []
- `fsdp_min_num_params`: 0
- `fsdp_config`: {'min_num_params': 0, 'xla': False, 'xla_fsdp_v2': False, 'xla_fsdp_grad_ckpt': False}
- `fsdp_transformer_layer_cls_to_wrap`: None
- `accelerator_config`: {'split_batches': False, 'dispatch_batches': None, 'even_batches': True, 'use_seedable_sampler': True, 'non_blocking': False, 'gradient_accumulation_kwargs': None}
- `deepspeed`: None
- `label_smoothing_factor`: 0.0
- `optim`: adamw_torch
- `optim_args`: None
- `adafactor`: False
- `group_by_length`: False
- `length_column_name`: length
- `ddp_find_unused_parameters`: None
- `ddp_bucket_cap_mb`: None
- `ddp_broadcast_buffers`: False
- `dataloader_pin_memory`: True
- `dataloader_persistent_workers`: False
- `skip_memory_metrics`: True
- `use_legacy_prediction_loop`: False
- `push_to_hub`: False
- `resume_from_checkpoint`: None
- `hub_model_id`: None
- `hub_strategy`: every_save
- `hub_private_repo`: False
- `hub_always_push`: False
- `gradient_checkpointing`: False
- `gradient_checkpointing_kwargs`: None
- `include_inputs_for_metrics`: False
- `eval_do_concat_batches`: True
- `fp16_backend`: auto
- `push_to_hub_model_id`: None
- `push_to_hub_organization`: None
- `mp_parameters`:
- `auto_find_batch_size`: False
- `full_determinism`: False
- `torchdynamo`: None
- `ray_scope`: last
- `ddp_timeout`: 1800
- `torch_compile`: False
- `torch_compile_backend`: None
- `torch_compile_mode`: None
- `dispatch_batches`: None
- `split_batches`: None
- `include_tokens_per_second`: False
- `include_num_input_tokens_seen`: False
- `neftune_noise_alpha`: None
- `optim_target_modules`: None
- `batch_eval_metrics`: False
- `batch_sampler`: no_duplicates
- `multi_dataset_batch_sampler`: proportional
</details>
### Training Logs
| Epoch | Step | Training Loss | loss | all-nli-dev_max_accuracy | all-nli-test_max_accuracy |
|:-----:|:----:|:-------------:|:------:|:------------------------:|:-------------------------:|
| 0 | 0 | - | - | 0.6832 | - |
| 0.016 | 100 | 2.6355 | 1.0725 | 0.7924 | - |
| 0.032 | 200 | 0.9206 | 0.8342 | 0.8080 | - |
| 0.048 | 300 | 1.2567 | 0.7855 | 0.8133 | - |
| 0.064 | 400 | 0.7949 | 0.8857 | 0.7974 | - |
| 0.08 | 500 | 0.7583 | 0.9487 | 0.7872 | - |
| 0.096 | 600 | 1.0022 | 1.1312 | 0.7848 | - |
| 0.112 | 700 | 0.8178 | 1.2282 | 0.7895 | - |
| 0.128 | 800 | 0.9997 | 1.5132 | 0.7488 | - |
| 0.144 | 900 | 1.1173 | 1.4605 | 0.7473 | - |
| 0.16 | 1000 | 1.0089 | 1.3794 | 0.7543 | - |
| 0.176 | 1100 | 1.0235 | 1.4188 | 0.7640 | - |
| 0.192 | 1200 | 1.0031 | 1.2465 | 0.7570 | - |
| 0.208 | 1300 | 0.8286 | 1.4176 | 0.7426 | - |
| 0.224 | 1400 | 0.8411 | 1.1914 | 0.7600 | - |
| 0.24 | 1500 | 0.8389 | 1.1719 | 0.7820 | - |
| 0.256 | 1600 | 0.7144 | 1.1167 | 0.7691 | - |
| 0.272 | 1700 | 0.881 | 1.0747 | 0.7902 | - |
| 0.288 | 1800 | 0.8657 | 1.1576 | 0.7966 | - |
| 0.304 | 1900 | 0.7323 | 1.0122 | 0.8322 | - |
| 0.32 | 2000 | 0.6578 | 1.1248 | 0.8273 | - |
| 0.336 | 2100 | 0.6037 | 1.1194 | 0.8269 | - |
| 0.352 | 2200 | 0.641 | 1.1410 | 0.8341 | - |
| 0.368 | 2300 | 0.7843 | 1.0600 | 0.8328 | - |
| 0.384 | 2400 | 0.8222 | 0.9988 | 0.8161 | - |
| 0.4 | 2500 | 0.7287 | 1.2026 | 0.8395 | - |
| 0.416 | 2600 | 0.6035 | 0.8802 | 0.8273 | - |
| 0.432 | 2700 | 0.8275 | 1.1631 | 0.8458 | - |
| 0.448 | 2800 | 0.8483 | 0.9218 | 0.8316 | - |
| 0.464 | 2900 | 0.8813 | 1.1187 | 0.8147 | - |
| 0.48 | 3000 | 0.7408 | 0.9582 | 0.8246 | - |
| 0.496 | 3100 | 0.7886 | 0.9364 | 0.8261 | - |
| 0.512 | 3200 | 0.6064 | 0.8338 | 0.8302 | - |
| 0.528 | 3300 | 0.6415 | 0.7895 | 0.8650 | - |
| 0.544 | 3400 | 0.5766 | 0.7525 | 0.8571 | - |
| 0.56 | 3500 | 0.6212 | 0.8605 | 0.8572 | - |
| 0.576 | 3600 | 0.5773 | 0.7460 | 0.8419 | - |
| 0.592 | 3700 | 0.6104 | 0.7480 | 0.8580 | - |
| 0.608 | 3800 | 0.5754 | 0.7215 | 0.8657 | - |
| 0.624 | 3900 | 0.5525 | 0.7900 | 0.8630 | - |
| 0.64 | 4000 | 0.7802 | 0.7443 | 0.8612 | - |
| 0.656 | 4100 | 0.9796 | 0.7756 | 0.8748 | - |
| 0.672 | 4200 | 0.9355 | 0.6917 | 0.8796 | - |
| 0.688 | 4300 | 0.7081 | 0.6442 | 0.8832 | - |
| 0.704 | 4400 | 0.6868 | 0.6395 | 0.8891 | - |
| 0.72 | 4500 | 0.5964 | 0.5983 | 0.8820 | - |
| 0.736 | 4600 | 0.6618 | 0.5754 | 0.8861 | - |
| 0.752 | 4700 | 0.6957 | 0.6177 | 0.8803 | - |
| 0.768 | 4800 | 0.6375 | 0.5577 | 0.8881 | - |
| 0.784 | 4900 | 0.5481 | 0.5496 | 0.8835 | - |
| 0.8 | 5000 | 0.6626 | 0.5728 | 0.8949 | - |
| 0.816 | 5100 | 0.5192 | 0.5329 | 0.8935 | - |
| 0.832 | 5200 | 0.5856 | 0.5188 | 0.8935 | - |
| 0.848 | 5300 | 0.5142 | 0.5252 | 0.8920 | - |
| 0.864 | 5400 | 0.6404 | 0.5641 | 0.8885 | - |
| 0.88 | 5500 | 0.5466 | 0.5209 | 0.8929 | - |
| 0.896 | 5600 | 0.575 | 0.5170 | 0.8961 | - |
| 0.912 | 5700 | 0.626 | 0.5095 | 0.9001 | - |
| 0.928 | 5800 | 0.5631 | 0.4817 | 0.8984 | - |
| 0.944 | 5900 | 0.7301 | 0.4996 | 0.8984 | - |
| 0.96 | 6000 | 0.7712 | 0.5160 | 0.9014 | - |
| 0.976 | 6100 | 0.6203 | 0.5000 | 0.9007 | - |
| 0.992 | 6200 | 0.0005 | 0.4996 | 0.9004 | - |
| 1.0 | 6250 | - | - | - | 0.9150 |
### Environmental Impact
Carbon emissions were measured using [CodeCarbon](https://github.com/mlco2/codecarbon).
- **Energy Consumed**: 0.306 kWh
- **Carbon Emitted**: 0.119 kg of CO2
- **Hours Used**: 1.661 hours
### Training Hardware
- **On Cloud**: No
- **GPU Model**: 1 x NVIDIA GeForce RTX 3090
- **CPU Model**: 13th Gen Intel(R) Core(TM) i7-13700K
- **RAM Size**: 31.78 GB
### Framework Versions
- Python: 3.11.6
- Sentence Transformers: 3.0.0.dev0
- Transformers: 4.41.1
- PyTorch: 2.3.0+cu121
- Accelerate: 0.30.1
- Datasets: 2.19.1
- Tokenizers: 0.19.1
## Citation
### BibTeX
#### Sentence Transformers
```bibtex
@inproceedings{reimers-2019-sentence-bert,
title = "Sentence-BERT: Sentence Embeddings using Siamese BERT-Networks",
author = "Reimers, Nils and Gurevych, Iryna",
booktitle = "Proceedings of the 2019 Conference on Empirical Methods in Natural Language Processing",
month = "11",
year = "2019",
publisher = "Association for Computational Linguistics",
url = "https://arxiv.org/abs/1908.10084",
}
```
#### MultipleNegativesRankingLoss
```bibtex
@misc{henderson2017efficient,
title={Efficient Natural Language Response Suggestion for Smart Reply},
author={Matthew Henderson and Rami Al-Rfou and Brian Strope and Yun-hsuan Sung and Laszlo Lukacs and Ruiqi Guo and Sanjiv Kumar and Balint Miklos and Ray Kurzweil},
year={2017},
eprint={1705.00652},
archivePrefix={arXiv},
primaryClass={cs.CL}
}
```
<!--
## Glossary
*Clearly define terms in order to be accessible across audiences.*
-->
<!--
## Model Card Authors
*Lists the people who create the model card, providing recognition and accountability for the detailed work that goes into its construction.*
-->
<!--
## Model Card Contact
*Provides a way for people who have updates to the Model Card, suggestions, or questions, to contact the Model Card authors.*
--> | [
"TEXT_CLASSIFICATION"
] | Non_BioNLP |
ssmits/Falcon2-5.5B-Swedish | ssmits | text-generation | [
"transformers",
"safetensors",
"falcon",
"text-generation",
"mergekit",
"merge",
"lazymergekit",
"tiiuae/falcon-11B",
"conversational",
"custom_code",
"sv",
"base_model:tiiuae/falcon-11B",
"base_model:finetune:tiiuae/falcon-11B",
"license:apache-2.0",
"autotrain_compatible",
"text-generation-inference",
"endpoints_compatible",
"region:us"
] | 1,716,229,835,000 | 2024-06-05T16:19:20 | 15 | 0 | ---
base_model:
- tiiuae/falcon-11B
language:
- sv
library_name: transformers
license: apache-2.0
tags:
- mergekit
- merge
- lazymergekit
- tiiuae/falcon-11B
---
## Why prune?
Even though [Falcon-11B](https://huggingface.co/tiiuae/falcon-11B) is trained on 5T tokens, it is still undertrained, as can be seen by this graph:

This is why the choice is made to prune 50% of the layers.
Note that \~1B of continued pre-training (\~1M rows of 1k tokens) is still required to restore the perplexity of this model in the desired language.
I'm planning on doing that for certain languages, depending on how much compute will be available.
# sliced
This is a merge of pre-trained language models created using [mergekit](https://github.com/cg123/mergekit).
## Merge Details
### Merge Method
This model was merged using the passthrough merge method.
### Models Merged
The following models were included in the merge:
* [tiiuae/falcon-11B](https://huggingface.co/tiiuae/falcon-11B)
### Configuration
The following YAML configuration was used to produce this model:
```yaml
slices:
- sources:
- model: tiiuae/falcon-11B
layer_range: [0, 25]
- sources:
- model: tiiuae/falcon-11B
layer_range: [56, 59]
merge_method: passthrough
dtype: bfloat16
```
[PruneMe](https://github.com/arcee-ai/PruneMe) has been utilized using the wikimedia/wikipedia Swedish (sv) subset by investigating layer similarity with 2000 samples. The layer ranges for pruning were determined based on this analysis to maintain performance while reducing model size.

```python
from transformers import AutoTokenizer, AutoModelForCausalLM
import transformers
import torch
model = "ssmits/Falcon2-5.5B-Swedish"
tokenizer = AutoTokenizer.from_pretrained(model)
pipeline = transformers.pipeline(
"text-generation",
model=model,
tokenizer=tokenizer,
torch_dtype=torch.bfloat16,
)
sequences = pipeline(
"Can you explain the concepts of Quantum Computing?",
max_length=200,
do_sample=True,
top_k=10,
num_return_sequences=1,
eos_token_id=tokenizer.eos_token_id,
)
for seq in sequences:
print(f"Result: {seq['generated_text']}")
```
💥 **Falcon LLMs require PyTorch 2.0 for use with `transformers`!**
For fast inference with Falcon, check-out [Text Generation Inference](https://github.com/huggingface/text-generation-inference)! Read more in this [blogpost]((https://huggingface.co/blog/falcon).
## Direct Use
Research on large language models; as a foundation for further specialization and finetuning for specific usecases (e.g., summarization, text generation, chatbot, etc.)
## Out-of-Scope Use
Production use without adequate assessment of risks and mitigation; any use cases which may be considered irresponsible or harmful.
## Bias, Risks, and Limitations
Falcon2-5.5B is trained mostly on English, but also German, Spanish, French, Italian, Portuguese, Polish, Dutch, Romanian, Czech, Swedish. It will not generalize appropriately to other languages. Furthermore, as it is trained on a large-scale corpora representative of the web, it will carry the stereotypes and biases commonly encountered online.
## Recommendations
We recommend users of Falcon2-5.5B to consider finetuning it for the specific set of tasks of interest, and for guardrails and appropriate precautions to be taken for any production use. | [
"SUMMARIZATION"
] | Non_BioNLP |
tmnam20/xlm-roberta-large-vsmec-10 | tmnam20 | text-classification | [
"transformers",
"safetensors",
"xlm-roberta",
"text-classification",
"generated_from_trainer",
"en",
"dataset:tmnam20/VieGLUE",
"base_model:FacebookAI/xlm-roberta-large",
"base_model:finetune:FacebookAI/xlm-roberta-large",
"license:mit",
"model-index",
"autotrain_compatible",
"endpoints_compatible",
"region:us"
] | 1,705,565,036,000 | 2024-01-18T08:07:26 | 9 | 0 | ---
base_model: xlm-roberta-large
datasets:
- tmnam20/VieGLUE
language:
- en
license: mit
metrics:
- accuracy
tags:
- generated_from_trainer
model-index:
- name: xlm-roberta-large-vsmec-10
results:
- task:
type: text-classification
name: Text Classification
dataset:
name: tmnam20/VieGLUE/VSMEC
type: tmnam20/VieGLUE
config: vsmec
split: validation
args: vsmec
metrics:
- type: accuracy
value: 0.37900874635568516
name: Accuracy
---
<!-- This model card has been generated automatically according to the information the Trainer had access to. You
should probably proofread and complete it, then remove this comment. -->
# xlm-roberta-large-vsmec-10
This model is a fine-tuned version of [xlm-roberta-large](https://huggingface.co/xlm-roberta-large) on the tmnam20/VieGLUE/VSMEC dataset.
It achieves the following results on the evaluation set:
- Loss: 1.6499
- Accuracy: 0.3790
## Model description
More information needed
## Intended uses & limitations
More information needed
## Training and evaluation data
More information needed
## Training procedure
### Training hyperparameters
The following hyperparameters were used during training:
- learning_rate: 2e-05
- train_batch_size: 32
- eval_batch_size: 16
- seed: 10
- optimizer: Adam with betas=(0.9,0.999) and epsilon=1e-08
- lr_scheduler_type: linear
- num_epochs: 3.0
### Training results
| Training Loss | Epoch | Step | Validation Loss | Accuracy |
|:-------------:|:-----:|:----:|:---------------:|:--------:|
| 1.6481 | 2.87 | 500 | 1.6571 | 0.3761 |
### Framework versions
- Transformers 4.36.0
- Pytorch 2.1.0+cu121
- Datasets 2.15.0
- Tokenizers 0.15.0
| [
"TEXT_CLASSIFICATION"
] | Non_BioNLP |
HPLT/sft-fpft-fr-bloom-1b7 | HPLT | text-generation | [
"transformers",
"pytorch",
"safetensors",
"bloom",
"text-generation",
"generation",
"question answering",
"instruction tuning",
"fr",
"arxiv:2309.08958",
"license:cc-by-nc-4.0",
"autotrain_compatible",
"text-generation-inference",
"endpoints_compatible",
"region:us"
] | 1,712,250,488,000 | 2025-01-24T13:12:05 | 27 | 0 | ---
language:
- fr
license: cc-by-nc-4.0
tags:
- generation
- question answering
- instruction tuning
---
### Model Description
This HF repository contains base LLMs instruction tuned (SFT) with full-parameter fine-tuning and then used to study whether monolingual or multilingual instruction tuning is more favourable.
* [GitHub](https://github.com/hplt-project/monolingual-multilingual-instruction-tuning/tree/main)
* [Paper](https://arxiv.org/abs/2309.08958)
#### Instruction tuning details
* Base model: [bloom-1b7](https://huggingface.co/bloom-1b7)
* Instruction tuning language: French
* Training method: full-parameter fine-tuning.
* Best checkpoint: best cross-entropy on a validation set, trained for 3 epochs.
* Dataset: machine-translated from [yahma/alpaca-cleaned](https://huggingface.co/datasets/yahma/alpaca-cleaned). You can download our data [HERE](https://github.com/hplt-project/monolingual-multilingual-instruction-tuning/tree/main/training-data).
#### Usage
The model checkpoint should be loaded using `transformers` library.
Please refer to our Github repository [HERE](https://github.com/hplt-project/monolingual-multilingual-instruction-tuning/tree/main/fpft) for inference and training instructions.
#### Citation
```
@inproceedings{chen-etal-2024-monolingual,
title="Monolingual or multilingual instruction tuning: Which makes a better {Alpaca}",
author="Pinzhen Chen and Shaoxiong Ji and Nikolay Bogoychev and Andrey Kutuzov and Barry Haddow and Kenneth Heafield",
year="2024",
booktitle = "Findings of the Association for Computational Linguistics: EACL 2024",
}
```
| [
"QUESTION_ANSWERING"
] | Non_BioNLP |
RichardErkhov/NucleusOrg_-_Nucleus-1B-alpha-1-awq | RichardErkhov | null | [
"safetensors",
"mistral",
"4-bit",
"awq",
"region:us"
] | 1,732,119,103,000 | 2024-11-20T16:12:46 | 5 | 0 | ---
{}
---
Quantization made by Richard Erkhov.
[Github](https://github.com/RichardErkhov)
[Discord](https://discord.gg/pvy7H8DZMG)
[Request more models](https://github.com/RichardErkhov/quant_request)
Nucleus-1B-alpha-1 - AWQ
- Model creator: https://huggingface.co/NucleusOrg/
- Original model: https://huggingface.co/NucleusOrg/Nucleus-1B-alpha-1/
Original model description:
---
license: mit
language:
- en
datasets:
- nampdn-ai/tiny-textbooks
---
# Nuclues 1B Alpha1
<p align="center">
<img src="https://github.com/prp-e/nucleus/raw/main/nucleus-logo.png" width=256 height=256>
</p>
## What is Nucleus?
Nucleus is a small language model based on Mistral (actually, the trimmed untrained version you can find [here](https://huggingface.co/lmlab/lmlab-mistral-1b-untrained)) and trained in different steps. First, we've pretrained it on TinyStories dataset, then [TinyTextBooks](https://huggingface.co/datasets/nampdn-ai/tiny-textbooks) to make it a more specific model. This model is just a _proof of concept_ at this point, but showed good promises in early tests. So with proper training, can be a good product over time!
## Inference
[](https://colab.research.google.com/github/prp-e/nucleus/blob/main/nucleus_1b_inference.ipynb)
First you need to install `transformers` and `accelerate` libraries in order to run this model. Then, you basically have to run the following code:
```python
from transformers import AutoModelForCausalLM, AutoTokenizer, GenerationConfig
import torch
model_name_or_id = "NucleusOrg/Nucleus-1B-alpha-1"
model = AutoModelForCausalLM.from_pretrained(model_name_or_id, torch_dtype=torch.float16, device_map="cuda")
tokenizer = AutoTokenizer.from_pretrained(model_name_or_id)
prompt = "### Lesson: Python Programming 101\n### Introduction\n"
inputs = tokenizer(prompt, return_tensors="pt").to("cuda")
generation_config = GenerationConfig(
do_sample=True,
top_k=1,
temperature=0.9,
max_new_tokens=500,
repetition_penalty=1.5,
pad_token_id=tokenizer.eos_token_id
)
outputs = model.generate(**inputs, generation_config=generation_config)
print(tokenizer.decode(outputs[0], skip_special_tokens=True))
```
__Prompt Format__: This model does not have a specific prompt format, but the best results could be achieved with a _textbook_ type of format like:
```
### Chapter 1: Elon Musk and Iron Man
Elon met Tony at a Cafe in Monaco, then they had a conversation about
```
You also can try something like this:
```
Question: Who are you?
Answer:
```
But since the model isn't made for chat/question answering, the result won't be good enough.
__Repetition Penalty__: Since most of these models like to repeat themselves, just keep that number there. You can increase or decrease it based on your liking,but keep in mind that a number lower than 1 makes the model _super repetitive_.
## Known Issues
* Since we only had 420k rows of data, a lot of information are missing on this model. Since mentioned earlier in this very model card, it's a _proof of concept_ model.
* You probably may test it with coding. Let's say that the model is terrible at coding. We may release a coding optimized model as soon as possible.
## Our Team
* Muhammadreza Haghiri ([X (formerly Twitter)](https://twitter.com/haghiri_ai) - [Website](https://haghiri75.com/en) - [Github](https://github.com/prp-e) - [LinkedIn](https://www.linkedin.com/in/muhammadreza-haghiri-1761325b))
* Mahi Mohrechi ([Website](https://mohrechi-portfolio.vercel.app/) - [Github](https://github.com/f-mohrechi) - [LinkedIn](https://www.linkedin.com/in/faeze-mohrechi/))
## Special Thanks
* LMLabs for providing 1B untrained model.
* Mistral Team for providing the best open source base model ever.
* _Sina Rashidi_, who translated Alpaca dataset to Persian.
* [Jupyto](https://jupyto.com) team for providing our infrastructure.
| [
"QUESTION_ANSWERING"
] | Non_BioNLP |
tuanio/ft-moellava-qwen1.5-1.8b-vista-lora-2ep | tuanio | text-classification | [
"adapter-transformers",
"safetensors",
"llava_qwen1_5",
"text-classification",
"vi",
"dataset:Vi-VLM/Vista",
"license:mit",
"region:us"
] | 1,721,308,868,000 | 2024-07-26T14:32:36 | 16 | 2 | ---
datasets:
- Vi-VLM/Vista
language:
- vi
library_name: adapter-transformers
license: mit
pipeline_tag: text-classification
---
<p align="center">
<div style="display: flex;text-align: center;">
<div>
<img src="https://firebasestorage.googleapis.com/v0/b/database-7ca5c.appspot.com/o/llm%2F68747470733a2f2f7331312e617831782e636f6d2f323032332f31322f32382f70697176444d562e706e67.png?alt=media&token=30a2470d-861e-4295-a7f4-da48231724cf" width="250" style="margin-bottom: 0.2;"/>
</div>
<div>
<img src="https://firebasestorage.googleapis.com/v0/b/database-7ca5c.appspot.com/o/llm%2Flogo_qwen.jpg?alt=media&token=fd2cd557-2f45-4f94-86d3-a5e7c9eef630" width="600" style="margin-bottom: 1rem;"/>
</div>
</div>
<p>
<h1 align="center">MoE-LLaVA-Qwen1.5-1.8B×4-Top2: When Vision meet Small-scaled Language Model and Vietnamese Synthetic Dataset</h1>
<h5 align="center">
# Introducing MoE-LLaVA-Qwen1.5-1.8B×4-Top2 for Vietnamese
We are excited to present MoE-LLaVA-Qwen1.5-1.8B×4-Top2, tailored for the Vietnamese language. This model is part of our ongoing efforts to develop Vision Language Models (VLM) for Vietnamese, a domain that is currently limited and predominantly features larger models (**~7B parameters**). Our model activates approximately **2.2B** 🤗😎 parameters per call, significantly reducing the memory footprint, and it can be quantized for local execution.
## Training Dataset
Our model is trained on the comprehensive [Vi-VLM/Vista dataset](https://huggingface.co/datasets/Vi-VLM/Vista), which includes around 700,000 Vietnamese vision-language samples curated by Gemini Pro. We employed various prompt engineering techniques, including:
- **Few-shot Learning**
- **Caption-based Prompting**
- **Image-based Prompting**
For the COCO dataset, we utilized Llava-style prompts to generate data. For the ShareGPT4V dataset, translation prompts were applied.
### Techniques Used
- **MoE-LLaVA**: [MoE-LLaVA](https://github.com/PKU-YuanGroup/MoE-LLaVA/tree/main)
## Evaluation
- Comming soon 🫡
## Bias, Risks, and Limitations
The dataset may contain biases originating from its sources. Users should remain aware of these potential biases when utilizing the dataset.
## More Information
This dataset represents the first stage of a two-stage development process for a larger model. Stay tuned for future developments by subscribing to our updates. | [
"TRANSLATION"
] | Non_BioNLP |
Someman/bart-hindi | Someman | summarization | [
"transformers",
"pytorch",
"safetensors",
"bart",
"text2text-generation",
"generated_from_trainer",
"hindi",
"summarization",
"seq2seq",
"dataset:Someman/hindi-summarization",
"base_model:facebook/bart-base",
"base_model:finetune:facebook/bart-base",
"license:apache-2.0",
"autotrain_compatible",
"endpoints_compatible",
"region:us"
] | 1,685,582,241,000 | 2023-09-15T11:31:34 | 48 | 1 | ---
base_model: facebook/bart-base
datasets:
- Someman/hindi-summarization
license: apache-2.0
tags:
- generated_from_trainer
- hindi
- summarization
- seq2seq
model-index:
- name: bart-hindi
results: []
---
<!-- This model card has been generated automatically according to the information the Trainer had access to. You
should probably proofread and complete it, then remove this comment. -->
# bart-hindi
This model is a fine-tuned version of [facebook/bart-base](https://huggingface.co/facebook/bart-base) on the [Someman/hindi-summarization](https://huggingface.co/datasets/Someman/hindi-summarization) dataset.
It achieves the following results on the evaluation set:
- Loss: 0.4985
## Model description
More information needed
## Intended uses & limitations
More information needed
## Training and evaluation data
More information needed
## Training procedure
### Training hyperparameters
The following hyperparameters were used during training:
- learning_rate: 5e-05
- train_batch_size: 1
- eval_batch_size: 1
- seed: 42
- gradient_accumulation_steps: 16
- total_train_batch_size: 16
- optimizer: Adam with betas=(0.9,0.999) and epsilon=1e-08
- lr_scheduler_type: linear
- lr_scheduler_warmup_steps: 500
- num_epochs: 1
### Training results
| Training Loss | Epoch | Step | Validation Loss |
|:-------------:|:-----:|:----:|:---------------:|
| 0.6568 | 0.14 | 500 | 0.6501 |
| 0.682 | 0.29 | 1000 | 0.5757 |
| 0.5331 | 0.43 | 1500 | 0.5530 |
| 0.5612 | 0.58 | 2000 | 0.5311 |
| 0.5685 | 0.72 | 2500 | 0.5043 |
| 0.4993 | 0.87 | 3000 | 0.4985 |
### Framework versions
- Transformers 4.29.2
- Pytorch 2.0.1+cu118
- Datasets 2.12.0
- Tokenizers 0.13.3 | [
"SUMMARIZATION"
] | Non_BioNLP |
frahman/distilbert-base-uncased-finetuned-clinc | frahman | text-classification | [
"transformers",
"pytorch",
"tensorboard",
"distilbert",
"text-classification",
"generated_from_trainer",
"dataset:clinc_oos",
"license:apache-2.0",
"model-index",
"autotrain_compatible",
"endpoints_compatible",
"region:us"
] | 1,646,263,745,000 | 2022-02-28T15:10:11 | 117 | 0 | ---
datasets:
- clinc_oos
license: apache-2.0
metrics:
- accuracy
tags:
- generated_from_trainer
model-index:
- name: distilbert-base-uncased-finetuned-clinc
results:
- task:
type: text-classification
name: Text Classification
dataset:
name: clinc_oos
type: clinc_oos
args: plus
metrics:
- type: accuracy
value: 0.9187096774193548
name: Accuracy
---
<!-- This model card has been generated automatically according to the information the Trainer had access to. You
should probably proofread and complete it, then remove this comment. -->
# distilbert-base-uncased-finetuned-clinc
This model is a fine-tuned version of [distilbert-base-uncased](https://huggingface.co/distilbert-base-uncased) on the clinc_oos dataset.
It achieves the following results on the evaluation set:
- Loss: 0.7703
- Accuracy: 0.9187
## Model description
More information needed
## Intended uses & limitations
More information needed
## Training and evaluation data
More information needed
## Training procedure
### Training hyperparameters
The following hyperparameters were used during training:
- learning_rate: 2e-05
- train_batch_size: 48
- eval_batch_size: 48
- seed: 42
- optimizer: Adam with betas=(0.9,0.999) and epsilon=1e-08
- lr_scheduler_type: linear
- num_epochs: 5
### Training results
| Training Loss | Epoch | Step | Validation Loss | Accuracy |
|:-------------:|:-----:|:----:|:---------------:|:--------:|
| 4.2896 | 1.0 | 318 | 3.2887 | 0.7419 |
| 2.6309 | 2.0 | 636 | 1.8797 | 0.8310 |
| 1.5443 | 3.0 | 954 | 1.1537 | 0.8974 |
| 1.0097 | 4.0 | 1272 | 0.8560 | 0.9135 |
| 0.7918 | 5.0 | 1590 | 0.7703 | 0.9187 |
### Framework versions
- Transformers 4.11.3
- Pytorch 1.10.0+cu111
- Datasets 1.16.1
- Tokenizers 0.10.3
| [
"TEXT_CLASSIFICATION"
] | Non_BioNLP |
Triangle104/granite-3.1-8b-instruct-Q5_K_S-GGUF | Triangle104 | text-generation | [
"transformers",
"gguf",
"language",
"granite-3.1",
"llama-cpp",
"gguf-my-repo",
"text-generation",
"base_model:ibm-granite/granite-3.1-8b-instruct",
"base_model:quantized:ibm-granite/granite-3.1-8b-instruct",
"license:apache-2.0",
"region:us",
"conversational"
] | 1,735,130,680,000 | 2024-12-25T12:45:30 | 5 | 0 | ---
base_model: ibm-granite/granite-3.1-8b-instruct
library_name: transformers
license: apache-2.0
pipeline_tag: text-generation
tags:
- language
- granite-3.1
- llama-cpp
- gguf-my-repo
inference: false
---
# Triangle104/granite-3.1-8b-instruct-Q5_K_S-GGUF
This model was converted to GGUF format from [`ibm-granite/granite-3.1-8b-instruct`](https://huggingface.co/ibm-granite/granite-3.1-8b-instruct) using llama.cpp via the ggml.ai's [GGUF-my-repo](https://huggingface.co/spaces/ggml-org/gguf-my-repo) space.
Refer to the [original model card](https://huggingface.co/ibm-granite/granite-3.1-8b-instruct) for more details on the model.
---
Model details:
-
Granite-3.1-8B-Instruct is a 8B parameter long-context instruct model
finetuned from Granite-3.1-8B-Base using a combination of open source
instruction datasets with permissive license and internally collected
synthetic datasets tailored for solving long context problems. This
model is developed using a diverse set of techniques with a structured
chat format, including supervised finetuning, model alignment using
reinforcement learning, and model merging.
Developers: Granite Team, IBM
GitHub Repository: ibm-granite/granite-3.1-language-models
Website: Granite Docs
Paper: Granite 3.1 Language Models (coming soon)
Release Date: December 18th, 2024
License: Apache 2.0
Supported Languages:
English, German, Spanish, French, Japanese, Portuguese, Arabic, Czech,
Italian, Korean, Dutch, and Chinese. Users may finetune Granite 3.1
models for languages beyond these 12 languages.
Intended Use:
The model is designed to respond to general instructions and can be used
to build AI assistants for multiple domains, including business
applications.
Capabilities
Summarization
Text classification
Text extraction
Question-answering
Retrieval Augmented Generation (RAG)
Code related tasks
Function-calling tasks
Multilingual dialog use cases
Long-context tasks including long document/meeting summarization, long document QA, etc.
Generation:
This is a simple example of how to use Granite-3.1-8B-Instruct model.
Install the following libraries:
pip install torch torchvision torchaudio
pip install accelerate
pip install transformers
Then, copy the snippet from the section that is relevant for your use case.
import torch
from transformers import AutoModelForCausalLM, AutoTokenizer
device = "auto"
model_path = "ibm-granite/granite-3.1-8b-instruct"
tokenizer = AutoTokenizer.from_pretrained(model_path)
# drop device_map if running on CPU
model = AutoModelForCausalLM.from_pretrained(model_path, device_map=device)
model.eval()
# change input text as desired
chat = [
{ "role": "user", "content": "Please list one IBM Research laboratory located in the United States. You should only output its name and location." },
]
chat = tokenizer.apply_chat_template(chat, tokenize=False, add_generation_prompt=True)
# tokenize the text
input_tokens = tokenizer(chat, return_tensors="pt").to(device)
# generate output tokens
output = model.generate(**input_tokens,
max_new_tokens=100)
# decode output tokens into text
output = tokenizer.batch_decode(output)
# print output
print(output)
Model Architecture:
Granite-3.1-8B-Instruct is based on a decoder-only dense transformer
architecture. Core components of this architecture are: GQA and RoPE,
MLP with SwiGLU, RMSNorm, and shared input/output embeddings.
---
## Use with llama.cpp
Install llama.cpp through brew (works on Mac and Linux)
```bash
brew install llama.cpp
```
Invoke the llama.cpp server or the CLI.
### CLI:
```bash
llama-cli --hf-repo Triangle104/granite-3.1-8b-instruct-Q5_K_S-GGUF --hf-file granite-3.1-8b-instruct-q5_k_s.gguf -p "The meaning to life and the universe is"
```
### Server:
```bash
llama-server --hf-repo Triangle104/granite-3.1-8b-instruct-Q5_K_S-GGUF --hf-file granite-3.1-8b-instruct-q5_k_s.gguf -c 2048
```
Note: You can also use this checkpoint directly through the [usage steps](https://github.com/ggerganov/llama.cpp?tab=readme-ov-file#usage) listed in the Llama.cpp repo as well.
Step 1: Clone llama.cpp from GitHub.
```
git clone https://github.com/ggerganov/llama.cpp
```
Step 2: Move into the llama.cpp folder and build it with `LLAMA_CURL=1` flag along with other hardware-specific flags (for ex: LLAMA_CUDA=1 for Nvidia GPUs on Linux).
```
cd llama.cpp && LLAMA_CURL=1 make
```
Step 3: Run inference through the main binary.
```
./llama-cli --hf-repo Triangle104/granite-3.1-8b-instruct-Q5_K_S-GGUF --hf-file granite-3.1-8b-instruct-q5_k_s.gguf -p "The meaning to life and the universe is"
```
or
```
./llama-server --hf-repo Triangle104/granite-3.1-8b-instruct-Q5_K_S-GGUF --hf-file granite-3.1-8b-instruct-q5_k_s.gguf -c 2048
```
| [
"TEXT_CLASSIFICATION",
"SUMMARIZATION"
] | Non_BioNLP |
philipk22/ind312-ft-v0 | philipk22 | sentence-similarity | [
"sentence-transformers",
"safetensors",
"bert",
"sentence-similarity",
"feature-extraction",
"generated_from_trainer",
"dataset_size:798",
"loss:MatryoshkaLoss",
"loss:MultipleNegativesRankingLoss",
"arxiv:1908.10084",
"arxiv:2205.13147",
"arxiv:1705.00652",
"base_model:Snowflake/snowflake-arctic-embed-m",
"base_model:finetune:Snowflake/snowflake-arctic-embed-m",
"model-index",
"autotrain_compatible",
"text-embeddings-inference",
"endpoints_compatible",
"region:us"
] | 1,740,253,819,000 | 2025-02-22T19:50:47 | 15 | 0 | ---
base_model: Snowflake/snowflake-arctic-embed-m
library_name: sentence-transformers
metrics:
- cosine_accuracy@1
- cosine_accuracy@3
- cosine_accuracy@5
- cosine_accuracy@10
- cosine_precision@1
- cosine_precision@3
- cosine_precision@5
- cosine_precision@10
- cosine_recall@1
- cosine_recall@3
- cosine_recall@5
- cosine_recall@10
- cosine_ndcg@10
- cosine_mrr@10
- cosine_map@100
pipeline_tag: sentence-similarity
tags:
- sentence-transformers
- sentence-similarity
- feature-extraction
- generated_from_trainer
- dataset_size:798
- loss:MatryoshkaLoss
- loss:MultipleNegativesRankingLoss
widget:
- source_sentence: What is the definition of a sponsor-investigator according to the
provided context?
sentences:
- '§ 312.47 Meetings.
(a) General. Meetings between a sponsor and the agency are frequently useful in
resolving questions and
issues raised during the course of a clinical investigation. FDA encourages such
meetings to the extent
that they aid in the evaluation of the drug and in the solution of scientific
problems concerning the drug, to
the extent that FDA''s resources permit. The general principle underlying the
conduct of such meetings is'
- 'employees to conduct an investigation that it has initiated is a sponsor, not
a sponsor-investigator, and
the employees are investigators.
Sponsor-Investigator means an individual who both initiates and conducts an investigation,
and under whose
immediate direction the investigational drug is administered or dispensed. The
term does not include any
person other than an individual. The requirements applicable to a sponsor-investigator
under this part'
- 'practice regulations in part 58, or, if the study was not conducted in compliance
with those
regulations, a brief statement of the reason for the noncompliance.
(9) Previous human experience with the investigational drug. A summary of previous
human experience
known to the applicant, if any, with the investigational drug. The information
is required to include
the following:
(i) If the investigational drug has been investigated or marketed previously,
either in the United'
- source_sentence: What is the primary purpose of Phase 1 studies in drug development?
sentences:
- '§ 312.53 Selecting investigators and monitors.
§ 312.54 Emergency research under § 50.24 of this chapter.
§ 312.55 Informing investigators.
This content is from the eCFR and is authoritative but unofficial.
21 CFR Part 312 (up to date as of 1/23/2025)
Investigational New Drug Application 21 CFR Part 312 (Jan. 23, 2025)
21 CFR Part 312 (Jan. 23, 2025) (enhanced display) page 1 of 54'
- 'relevant to the safety of the drug as are required under § 312.32. The sponsor
shall make annual reports
on the progress of the investigation in accordance with § 312.33.
(d) A sponsor who determines that its investigational drug presents an unreasonable
and significant risk to
subjects shall discontinue those investigations that present the risk, notify
FDA, all institutional review
boards, and all investigators who have at any time participated in the investigation
of the discontinuance,'
- 'are typically closely monitored and may be conducted in patients or normal volunteer
subjects.
These studies are designed to determine the metabolism and pharmacologic actions
of the drug in
humans, the side effects associated with increasing doses, and, if possible, to
gain early evidence on
effectiveness. During Phase 1, sufficient information about the drug''s pharmacokinetics
and
pharmacological effects should be obtained to permit the design of well-controlled,
scientifically'
- source_sentence: What is the required format for numbering submissions related to
the investigation?
sentences:
- 'using a single, three-digit serial number. The initial IND is required to be
numbered 000; each subsequent
submission (e.g., amendment, report, or correspondence) is required to be numbered
chronologically in
sequence.
(f) Identification of exception from informed consent. If the investigation involves
an exception from informed
consent under § 50.24 of this chapter, the sponsor shall prominently identify
on the cover sheet that the'
- 'response time, a sponsor may not proceed with a clinical trial on which a clinical
hold has been imposed
until the sponsor has been notified by FDA that the hold has been lifted.
(f) Appeal. If the sponsor disagrees with the reasons cited for the clinical hold,
the sponsor may request
reconsideration of the decision in accordance with § 312.48.
(g) Conversion of IND on clinical hold to inactive status. If all investigations
covered by an IND remain on'
- 'investigator, the sponsor of any investigation in which the investigator has
been named as a participant,
and the reviewing institutional review boards (IRBs) that the investigator is
not eligible to receive test
articles under this part. The notification to the investigator, sponsor, and IRBs
will provide a statement of
21 CFR Part 312 (up to date as of 1/23/2025)
Investigational New Drug Application 21 CFR 312.66
21 CFR 312.70(b) (enhanced display) page 37 of 54'
- source_sentence: What are the regions mentioned in the context where drugs can be
exported?
sentences:
- 'Africa, or to any country in the European Union or the European Economic Area,
and complies with
the laws of the country to which it is being exported, the applicable provisions
of section 802(c), (f),
and (g) of the act, and § 1.101 of this chapter. Drugs exported under this paragraph
that are not the
subject of an IND are exempt from the label requirement in § 312.6(a); or
(4) Except as provided in paragraph (b)(5) of this section, the person exporting
the drug sends an email'
- 'before its implementation. Protocol amendments to add a new investigator or to
provide additional
information about investigators may be grouped and submitted at 30-day intervals.
When several
submissions of new protocols or protocol changes are anticipated during a short
period, the sponsor is
encouraged, to the extent feasible, to include these all in a single submission.
21 CFR Part 312 (up to date as of 1/23/2025)
Investigational New Drug Application 21 CFR 312.30(b)(2)(i)(b)'
- 'that apply to specific types of expanded access are described in §§ 312.310 through
312.320.
(a) Scope. This subpart contains the requirements for the use of investigational
new drugs and approved
drugs where availability is limited by a risk evaluation and mitigation strategy
(REMS) when the primary
purpose is to diagnose, monitor, or treat a patient''s disease or condition. The
aim of this subpart is to'
- source_sentence: What regulatory framework does 21 CFR Part 312 pertain to as of
January 23, 2025?
sentences:
- 'risk-benefit judgment in making the final decision on approvability. As part
of this evaluation, consistent
with the statement of purpose in § 312.80, FDA will consider whether the benefits
of the drug outweigh
the known and potential risks of the drug and the need to answer remaining questions
about risks and
benefits of the drug, taking into consideration the severity of the disease and
the absence of satisfactory
alternative therapy.'
- 'provide for disposition of the unused supplies of the drug under § 312.59.
(b) Case histories. An investigator is required to prepare and maintain adequate
and accurate case histories
that record all observations and other data pertinent to the investigation on
each individual administered
the investigational drug or employed as a control in the investigation. Case histories
include the case
report forms and supporting data including, for example, signed and dated consent
forms and medical'
- '§ 312.315 Intermediate-size patient populations.
21 CFR Part 312 (up to date as of 1/23/2025)
Investigational New Drug Application 21 CFR Part 312 (Jan. 23, 2025)
21 CFR Part 312 (Jan. 23, 2025) (enhanced display) page 2 of 54'
model-index:
- name: SentenceTransformer based on Snowflake/snowflake-arctic-embed-m
results:
- task:
type: information-retrieval
name: Information Retrieval
dataset:
name: Unknown
type: unknown
metrics:
- type: cosine_accuracy@1
value: 0.92
name: Cosine Accuracy@1
- type: cosine_accuracy@3
value: 0.99
name: Cosine Accuracy@3
- type: cosine_accuracy@5
value: 0.99
name: Cosine Accuracy@5
- type: cosine_accuracy@10
value: 1.0
name: Cosine Accuracy@10
- type: cosine_precision@1
value: 0.92
name: Cosine Precision@1
- type: cosine_precision@3
value: 0.33000000000000007
name: Cosine Precision@3
- type: cosine_precision@5
value: 0.19799999999999998
name: Cosine Precision@5
- type: cosine_precision@10
value: 0.09999999999999998
name: Cosine Precision@10
- type: cosine_recall@1
value: 0.92
name: Cosine Recall@1
- type: cosine_recall@3
value: 0.99
name: Cosine Recall@3
- type: cosine_recall@5
value: 0.99
name: Cosine Recall@5
- type: cosine_recall@10
value: 1.0
name: Cosine Recall@10
- type: cosine_ndcg@10
value: 0.9637992620139386
name: Cosine Ndcg@10
- type: cosine_mrr@10
value: 0.9516666666666665
name: Cosine Mrr@10
- type: cosine_map@100
value: 0.9516666666666667
name: Cosine Map@100
---
# SentenceTransformer based on Snowflake/snowflake-arctic-embed-m
This is a [sentence-transformers](https://www.SBERT.net) model finetuned from [Snowflake/snowflake-arctic-embed-m](https://huggingface.co/Snowflake/snowflake-arctic-embed-m). It maps sentences & paragraphs to a 768-dimensional dense vector space and can be used for semantic textual similarity, semantic search, paraphrase mining, text classification, clustering, and more.
## Model Details
### Model Description
- **Model Type:** Sentence Transformer
- **Base model:** [Snowflake/snowflake-arctic-embed-m](https://huggingface.co/Snowflake/snowflake-arctic-embed-m) <!-- at revision fc74610d18462d218e312aa986ec5c8a75a98152 -->
- **Maximum Sequence Length:** 512 tokens
- **Output Dimensionality:** 768 dimensions
- **Similarity Function:** Cosine Similarity
<!-- - **Training Dataset:** Unknown -->
<!-- - **Language:** Unknown -->
<!-- - **License:** Unknown -->
### Model Sources
- **Documentation:** [Sentence Transformers Documentation](https://sbert.net)
- **Repository:** [Sentence Transformers on GitHub](https://github.com/UKPLab/sentence-transformers)
- **Hugging Face:** [Sentence Transformers on Hugging Face](https://huggingface.co/models?library=sentence-transformers)
### Full Model Architecture
```
SentenceTransformer(
(0): Transformer({'max_seq_length': 512, 'do_lower_case': False}) with Transformer model: BertModel
(1): Pooling({'word_embedding_dimension': 768, 'pooling_mode_cls_token': True, 'pooling_mode_mean_tokens': False, 'pooling_mode_max_tokens': False, 'pooling_mode_mean_sqrt_len_tokens': False, 'pooling_mode_weightedmean_tokens': False, 'pooling_mode_lasttoken': False, 'include_prompt': True})
(2): Normalize()
)
```
## Usage
### Direct Usage (Sentence Transformers)
First install the Sentence Transformers library:
```bash
pip install -U sentence-transformers
```
Then you can load this model and run inference.
```python
from sentence_transformers import SentenceTransformer
# Download from the 🤗 Hub
model = SentenceTransformer("philipk22/ind312-ft-v0")
# Run inference
sentences = [
'What regulatory framework does 21 CFR Part 312 pertain to as of January 23, 2025?',
'§ 312.315 Intermediate-size patient populations.\n21 CFR Part 312 (up to date as of 1/23/2025)\nInvestigational New Drug Application 21 CFR Part 312 (Jan. 23, 2025)\n21 CFR Part 312 (Jan. 23, 2025) (enhanced display) page 2 of 54',
'risk-benefit judgment in making the final decision on approvability. As part of this evaluation, consistent\nwith the statement of purpose in § 312.80, FDA will consider whether the benefits of the drug outweigh\nthe known and potential risks of the drug and the need to answer remaining questions about risks and\nbenefits of the drug, taking into consideration the severity of the disease and the absence of satisfactory\nalternative therapy.',
]
embeddings = model.encode(sentences)
print(embeddings.shape)
# [3, 768]
# Get the similarity scores for the embeddings
similarities = model.similarity(embeddings, embeddings)
print(similarities.shape)
# [3, 3]
```
<!--
### Direct Usage (Transformers)
<details><summary>Click to see the direct usage in Transformers</summary>
</details>
-->
<!--
### Downstream Usage (Sentence Transformers)
You can finetune this model on your own dataset.
<details><summary>Click to expand</summary>
</details>
-->
<!--
### Out-of-Scope Use
*List how the model may foreseeably be misused and address what users ought not to do with the model.*
-->
## Evaluation
### Metrics
#### Information Retrieval
* Evaluated with [<code>InformationRetrievalEvaluator</code>](https://sbert.net/docs/package_reference/sentence_transformer/evaluation.html#sentence_transformers.evaluation.InformationRetrievalEvaluator)
| Metric | Value |
|:--------------------|:-----------|
| cosine_accuracy@1 | 0.92 |
| cosine_accuracy@3 | 0.99 |
| cosine_accuracy@5 | 0.99 |
| cosine_accuracy@10 | 1.0 |
| cosine_precision@1 | 0.92 |
| cosine_precision@3 | 0.33 |
| cosine_precision@5 | 0.198 |
| cosine_precision@10 | 0.1 |
| cosine_recall@1 | 0.92 |
| cosine_recall@3 | 0.99 |
| cosine_recall@5 | 0.99 |
| cosine_recall@10 | 1.0 |
| **cosine_ndcg@10** | **0.9638** |
| cosine_mrr@10 | 0.9517 |
| cosine_map@100 | 0.9517 |
<!--
## Bias, Risks and Limitations
*What are the known or foreseeable issues stemming from this model? You could also flag here known failure cases or weaknesses of the model.*
-->
<!--
### Recommendations
*What are recommendations with respect to the foreseeable issues? For example, filtering explicit content.*
-->
## Training Details
### Training Dataset
#### Unnamed Dataset
* Size: 798 training samples
* Columns: <code>sentence_0</code> and <code>sentence_1</code>
* Approximate statistics based on the first 798 samples:
| | sentence_0 | sentence_1 |
|:--------|:-----------------------------------------------------------------------------------|:------------------------------------------------------------------------------------|
| type | string | string |
| details | <ul><li>min: 12 tokens</li><li>mean: 20.82 tokens</li><li>max: 46 tokens</li></ul> | <ul><li>min: 19 tokens</li><li>mean: 93.06 tokens</li><li>max: 158 tokens</li></ul> |
* Samples:
| sentence_0 | sentence_1 |
|:--------------------------------------------------------------------------------------------------------|:----------------------------------------------------------------------------------------------------------------------------------------------------------------------------------------------------------------------------------------------------------------------------------------------------------------------------------------------------------------------------------------------------------------------------------------------------------------------------------------------------------------------------|
| <code>What is the scope of Part 312 in Title 21 regarding investigational new drug applications?</code> | <code>Title 21 —Food and Drugs<br>Chapter I —Food and Drug Administration, Department of Health and Human Services<br>Subchapter D —Drugs for Human Use<br>Part 312 Investigational New Drug Application<br>Subpart A General Provisions<br>§ 312.1 Scope.<br>§ 312.2 Applicability.<br>§ 312.3 Definitions and interpretations.<br>§ 312.6 Labeling of an investigational new drug.<br>§ 312.7 Promotion of investigational drugs.<br>§ 312.8 Charging for investigational drugs under an IND.<br>§ 312.10 Waivers.</code> |
| <code>How does § 3126 address the labeling requirements for investigational new drugs?</code> | <code>Title 21 —Food and Drugs<br>Chapter I —Food and Drug Administration, Department of Health and Human Services<br>Subchapter D —Drugs for Human Use<br>Part 312 Investigational New Drug Application<br>Subpart A General Provisions<br>§ 312.1 Scope.<br>§ 312.2 Applicability.<br>§ 312.3 Definitions and interpretations.<br>§ 312.6 Labeling of an investigational new drug.<br>§ 312.7 Promotion of investigational drugs.<br>§ 312.8 Charging for investigational drugs under an IND.<br>§ 312.10 Waivers.</code> |
| <code>What are the general principles outlined in § 31222 regarding the IND submission?</code> | <code>§ 312.10 Waivers.<br>Subpart B Investigational New Drug Application (IND)<br>§ 312.20 Requirement for an IND.<br>§ 312.21 Phases of an investigation.<br>§ 312.22 General principles of the IND submission.<br>§ 312.23 IND content and format.<br>§ 312.30 Protocol amendments.<br>§ 312.31 Information amendments.<br>§ 312.32 IND safety reporting.<br>§ 312.33 Annual reports.<br>§ 312.38 Withdrawal of an IND.<br>Subpart C Administrative Actions</code> |
* Loss: [<code>MatryoshkaLoss</code>](https://sbert.net/docs/package_reference/sentence_transformer/losses.html#matryoshkaloss) with these parameters:
```json
{
"loss": "MultipleNegativesRankingLoss",
"matryoshka_dims": [
768,
512,
256,
128,
64
],
"matryoshka_weights": [
1,
1,
1,
1,
1
],
"n_dims_per_step": -1
}
```
### Training Hyperparameters
#### Non-Default Hyperparameters
- `eval_strategy`: steps
- `per_device_train_batch_size`: 10
- `per_device_eval_batch_size`: 10
- `num_train_epochs`: 10
- `multi_dataset_batch_sampler`: round_robin
#### All Hyperparameters
<details><summary>Click to expand</summary>
- `overwrite_output_dir`: False
- `do_predict`: False
- `eval_strategy`: steps
- `prediction_loss_only`: True
- `per_device_train_batch_size`: 10
- `per_device_eval_batch_size`: 10
- `per_gpu_train_batch_size`: None
- `per_gpu_eval_batch_size`: None
- `gradient_accumulation_steps`: 1
- `eval_accumulation_steps`: None
- `torch_empty_cache_steps`: None
- `learning_rate`: 5e-05
- `weight_decay`: 0.0
- `adam_beta1`: 0.9
- `adam_beta2`: 0.999
- `adam_epsilon`: 1e-08
- `max_grad_norm`: 1
- `num_train_epochs`: 10
- `max_steps`: -1
- `lr_scheduler_type`: linear
- `lr_scheduler_kwargs`: {}
- `warmup_ratio`: 0.0
- `warmup_steps`: 0
- `log_level`: passive
- `log_level_replica`: warning
- `log_on_each_node`: True
- `logging_nan_inf_filter`: True
- `save_safetensors`: True
- `save_on_each_node`: False
- `save_only_model`: False
- `restore_callback_states_from_checkpoint`: False
- `no_cuda`: False
- `use_cpu`: False
- `use_mps_device`: False
- `seed`: 42
- `data_seed`: None
- `jit_mode_eval`: False
- `use_ipex`: False
- `bf16`: False
- `fp16`: False
- `fp16_opt_level`: O1
- `half_precision_backend`: auto
- `bf16_full_eval`: False
- `fp16_full_eval`: False
- `tf32`: None
- `local_rank`: 0
- `ddp_backend`: None
- `tpu_num_cores`: None
- `tpu_metrics_debug`: False
- `debug`: []
- `dataloader_drop_last`: False
- `dataloader_num_workers`: 0
- `dataloader_prefetch_factor`: None
- `past_index`: -1
- `disable_tqdm`: False
- `remove_unused_columns`: True
- `label_names`: None
- `load_best_model_at_end`: False
- `ignore_data_skip`: False
- `fsdp`: []
- `fsdp_min_num_params`: 0
- `fsdp_config`: {'min_num_params': 0, 'xla': False, 'xla_fsdp_v2': False, 'xla_fsdp_grad_ckpt': False}
- `fsdp_transformer_layer_cls_to_wrap`: None
- `accelerator_config`: {'split_batches': False, 'dispatch_batches': None, 'even_batches': True, 'use_seedable_sampler': True, 'non_blocking': False, 'gradient_accumulation_kwargs': None}
- `deepspeed`: None
- `label_smoothing_factor`: 0.0
- `optim`: adamw_torch
- `optim_args`: None
- `adafactor`: False
- `group_by_length`: False
- `length_column_name`: length
- `ddp_find_unused_parameters`: None
- `ddp_bucket_cap_mb`: None
- `ddp_broadcast_buffers`: False
- `dataloader_pin_memory`: True
- `dataloader_persistent_workers`: False
- `skip_memory_metrics`: True
- `use_legacy_prediction_loop`: False
- `push_to_hub`: False
- `resume_from_checkpoint`: None
- `hub_model_id`: None
- `hub_strategy`: every_save
- `hub_private_repo`: None
- `hub_always_push`: False
- `gradient_checkpointing`: False
- `gradient_checkpointing_kwargs`: None
- `include_inputs_for_metrics`: False
- `include_for_metrics`: []
- `eval_do_concat_batches`: True
- `fp16_backend`: auto
- `push_to_hub_model_id`: None
- `push_to_hub_organization`: None
- `mp_parameters`:
- `auto_find_batch_size`: False
- `full_determinism`: False
- `torchdynamo`: None
- `ray_scope`: last
- `ddp_timeout`: 1800
- `torch_compile`: False
- `torch_compile_backend`: None
- `torch_compile_mode`: None
- `dispatch_batches`: None
- `split_batches`: None
- `include_tokens_per_second`: False
- `include_num_input_tokens_seen`: False
- `neftune_noise_alpha`: None
- `optim_target_modules`: None
- `batch_eval_metrics`: False
- `eval_on_start`: False
- `use_liger_kernel`: False
- `eval_use_gather_object`: False
- `average_tokens_across_devices`: False
- `prompts`: None
- `batch_sampler`: batch_sampler
- `multi_dataset_batch_sampler`: round_robin
</details>
### Training Logs
| Epoch | Step | Training Loss | cosine_ndcg@10 |
|:-----:|:----:|:-------------:|:--------------:|
| 0.625 | 50 | - | 0.9091 |
| 1.0 | 80 | - | 0.9209 |
| 1.25 | 100 | - | 0.9329 |
| 1.875 | 150 | - | 0.9439 |
| 2.0 | 160 | - | 0.9379 |
| 2.5 | 200 | - | 0.9367 |
| 3.0 | 240 | - | 0.9459 |
| 3.125 | 250 | - | 0.9432 |
| 3.75 | 300 | - | 0.9479 |
| 4.0 | 320 | - | 0.9515 |
| 4.375 | 350 | - | 0.9509 |
| 5.0 | 400 | - | 0.9581 |
| 5.625 | 450 | - | 0.9551 |
| 6.0 | 480 | - | 0.9604 |
| 6.25 | 500 | 0.3078 | 0.9577 |
| 6.875 | 550 | - | 0.9651 |
| 7.0 | 560 | - | 0.9651 |
| 7.5 | 600 | - | 0.9641 |
| 8.0 | 640 | - | 0.9641 |
| 8.125 | 650 | - | 0.9638 |
| 8.75 | 700 | - | 0.9638 |
| 9.0 | 720 | - | 0.9638 |
| 9.375 | 750 | - | 0.9601 |
| 10.0 | 800 | - | 0.9638 |
### Framework Versions
- Python: 3.11.11
- Sentence Transformers: 3.4.1
- Transformers: 4.48.3
- PyTorch: 2.5.1+cu124
- Accelerate: 1.3.0
- Datasets: 3.3.2
- Tokenizers: 0.21.0
## Citation
### BibTeX
#### Sentence Transformers
```bibtex
@inproceedings{reimers-2019-sentence-bert,
title = "Sentence-BERT: Sentence Embeddings using Siamese BERT-Networks",
author = "Reimers, Nils and Gurevych, Iryna",
booktitle = "Proceedings of the 2019 Conference on Empirical Methods in Natural Language Processing",
month = "11",
year = "2019",
publisher = "Association for Computational Linguistics",
url = "https://arxiv.org/abs/1908.10084",
}
```
#### MatryoshkaLoss
```bibtex
@misc{kusupati2024matryoshka,
title={Matryoshka Representation Learning},
author={Aditya Kusupati and Gantavya Bhatt and Aniket Rege and Matthew Wallingford and Aditya Sinha and Vivek Ramanujan and William Howard-Snyder and Kaifeng Chen and Sham Kakade and Prateek Jain and Ali Farhadi},
year={2024},
eprint={2205.13147},
archivePrefix={arXiv},
primaryClass={cs.LG}
}
```
#### MultipleNegativesRankingLoss
```bibtex
@misc{henderson2017efficient,
title={Efficient Natural Language Response Suggestion for Smart Reply},
author={Matthew Henderson and Rami Al-Rfou and Brian Strope and Yun-hsuan Sung and Laszlo Lukacs and Ruiqi Guo and Sanjiv Kumar and Balint Miklos and Ray Kurzweil},
year={2017},
eprint={1705.00652},
archivePrefix={arXiv},
primaryClass={cs.CL}
}
```
<!--
## Glossary
*Clearly define terms in order to be accessible across audiences.*
-->
<!--
## Model Card Authors
*Lists the people who create the model card, providing recognition and accountability for the detailed work that goes into its construction.*
-->
<!--
## Model Card Contact
*Provides a way for people who have updates to the Model Card, suggestions, or questions, to contact the Model Card authors.*
--> | [
"TEXT_CLASSIFICATION"
] | BioNLP |
RichardErkhov/lemon-mint_-_gemma-2-2b-translation-Iter1-gguf | RichardErkhov | null | [
"gguf",
"arxiv:1910.09700",
"endpoints_compatible",
"region:us",
"conversational"
] | 1,740,472,036,000 | 2025-02-25T09:17:48 | 429 | 0 | ---
{}
---
Quantization made by Richard Erkhov.
[Github](https://github.com/RichardErkhov)
[Discord](https://discord.gg/pvy7H8DZMG)
[Request more models](https://github.com/RichardErkhov/quant_request)
gemma-2-2b-translation-Iter1 - GGUF
- Model creator: https://huggingface.co/lemon-mint/
- Original model: https://huggingface.co/lemon-mint/gemma-2-2b-translation-Iter1/
| Name | Quant method | Size |
| ---- | ---- | ---- |
| [gemma-2-2b-translation-Iter1.Q2_K.gguf](https://huggingface.co/RichardErkhov/lemon-mint_-_gemma-2-2b-translation-Iter1-gguf/blob/main/gemma-2-2b-translation-Iter1.Q2_K.gguf) | Q2_K | 1.15GB |
| [gemma-2-2b-translation-Iter1.IQ3_XS.gguf](https://huggingface.co/RichardErkhov/lemon-mint_-_gemma-2-2b-translation-Iter1-gguf/blob/main/gemma-2-2b-translation-Iter1.IQ3_XS.gguf) | IQ3_XS | 1.22GB |
| [gemma-2-2b-translation-Iter1.IQ3_S.gguf](https://huggingface.co/RichardErkhov/lemon-mint_-_gemma-2-2b-translation-Iter1-gguf/blob/main/gemma-2-2b-translation-Iter1.IQ3_S.gguf) | IQ3_S | 1.27GB |
| [gemma-2-2b-translation-Iter1.Q3_K_S.gguf](https://huggingface.co/RichardErkhov/lemon-mint_-_gemma-2-2b-translation-Iter1-gguf/blob/main/gemma-2-2b-translation-Iter1.Q3_K_S.gguf) | Q3_K_S | 1.27GB |
| [gemma-2-2b-translation-Iter1.IQ3_M.gguf](https://huggingface.co/RichardErkhov/lemon-mint_-_gemma-2-2b-translation-Iter1-gguf/blob/main/gemma-2-2b-translation-Iter1.IQ3_M.gguf) | IQ3_M | 1.3GB |
| [gemma-2-2b-translation-Iter1.Q3_K.gguf](https://huggingface.co/RichardErkhov/lemon-mint_-_gemma-2-2b-translation-Iter1-gguf/blob/main/gemma-2-2b-translation-Iter1.Q3_K.gguf) | Q3_K | 1.36GB |
| [gemma-2-2b-translation-Iter1.Q3_K_M.gguf](https://huggingface.co/RichardErkhov/lemon-mint_-_gemma-2-2b-translation-Iter1-gguf/blob/main/gemma-2-2b-translation-Iter1.Q3_K_M.gguf) | Q3_K_M | 1.36GB |
| [gemma-2-2b-translation-Iter1.Q3_K_L.gguf](https://huggingface.co/RichardErkhov/lemon-mint_-_gemma-2-2b-translation-Iter1-gguf/blob/main/gemma-2-2b-translation-Iter1.Q3_K_L.gguf) | Q3_K_L | 1.44GB |
| [gemma-2-2b-translation-Iter1.IQ4_XS.gguf](https://huggingface.co/RichardErkhov/lemon-mint_-_gemma-2-2b-translation-Iter1-gguf/blob/main/gemma-2-2b-translation-Iter1.IQ4_XS.gguf) | IQ4_XS | 1.47GB |
| [gemma-2-2b-translation-Iter1.Q4_0.gguf](https://huggingface.co/RichardErkhov/lemon-mint_-_gemma-2-2b-translation-Iter1-gguf/blob/main/gemma-2-2b-translation-Iter1.Q4_0.gguf) | Q4_0 | 1.52GB |
| [gemma-2-2b-translation-Iter1.IQ4_NL.gguf](https://huggingface.co/RichardErkhov/lemon-mint_-_gemma-2-2b-translation-Iter1-gguf/blob/main/gemma-2-2b-translation-Iter1.IQ4_NL.gguf) | IQ4_NL | 1.53GB |
| [gemma-2-2b-translation-Iter1.Q4_K_S.gguf](https://huggingface.co/RichardErkhov/lemon-mint_-_gemma-2-2b-translation-Iter1-gguf/blob/main/gemma-2-2b-translation-Iter1.Q4_K_S.gguf) | Q4_K_S | 1.53GB |
| [gemma-2-2b-translation-Iter1.Q4_K.gguf](https://huggingface.co/RichardErkhov/lemon-mint_-_gemma-2-2b-translation-Iter1-gguf/blob/main/gemma-2-2b-translation-Iter1.Q4_K.gguf) | Q4_K | 1.59GB |
| [gemma-2-2b-translation-Iter1.Q4_K_M.gguf](https://huggingface.co/RichardErkhov/lemon-mint_-_gemma-2-2b-translation-Iter1-gguf/blob/main/gemma-2-2b-translation-Iter1.Q4_K_M.gguf) | Q4_K_M | 1.59GB |
| [gemma-2-2b-translation-Iter1.Q4_1.gguf](https://huggingface.co/RichardErkhov/lemon-mint_-_gemma-2-2b-translation-Iter1-gguf/blob/main/gemma-2-2b-translation-Iter1.Q4_1.gguf) | Q4_1 | 1.64GB |
| [gemma-2-2b-translation-Iter1.Q5_0.gguf](https://huggingface.co/RichardErkhov/lemon-mint_-_gemma-2-2b-translation-Iter1-gguf/blob/main/gemma-2-2b-translation-Iter1.Q5_0.gguf) | Q5_0 | 1.75GB |
| [gemma-2-2b-translation-Iter1.Q5_K_S.gguf](https://huggingface.co/RichardErkhov/lemon-mint_-_gemma-2-2b-translation-Iter1-gguf/blob/main/gemma-2-2b-translation-Iter1.Q5_K_S.gguf) | Q5_K_S | 1.75GB |
| [gemma-2-2b-translation-Iter1.Q5_K.gguf](https://huggingface.co/RichardErkhov/lemon-mint_-_gemma-2-2b-translation-Iter1-gguf/blob/main/gemma-2-2b-translation-Iter1.Q5_K.gguf) | Q5_K | 1.79GB |
| [gemma-2-2b-translation-Iter1.Q5_K_M.gguf](https://huggingface.co/RichardErkhov/lemon-mint_-_gemma-2-2b-translation-Iter1-gguf/blob/main/gemma-2-2b-translation-Iter1.Q5_K_M.gguf) | Q5_K_M | 1.79GB |
| [gemma-2-2b-translation-Iter1.Q5_1.gguf](https://huggingface.co/RichardErkhov/lemon-mint_-_gemma-2-2b-translation-Iter1-gguf/blob/main/gemma-2-2b-translation-Iter1.Q5_1.gguf) | Q5_1 | 1.87GB |
| [gemma-2-2b-translation-Iter1.Q6_K.gguf](https://huggingface.co/RichardErkhov/lemon-mint_-_gemma-2-2b-translation-Iter1-gguf/blob/main/gemma-2-2b-translation-Iter1.Q6_K.gguf) | Q6_K | 2.0GB |
| [gemma-2-2b-translation-Iter1.Q8_0.gguf](https://huggingface.co/RichardErkhov/lemon-mint_-_gemma-2-2b-translation-Iter1-gguf/blob/main/gemma-2-2b-translation-Iter1.Q8_0.gguf) | Q8_0 | 2.59GB |
Original model description:
---
library_name: transformers
license: gemma
language:
- ko
- en
pipeline_tag: translation
---
# Model Card for Model ID
<!-- Provide a quick summary of what the model is/does. -->
## Model Details
### Model Description
<!-- Provide a longer summary of what this model is. -->
This is the model card of a 🤗 transformers model that has been pushed on the Hub. This model card has been automatically generated.
- **Developed by:** [More Information Needed]
- **Funded by [optional]:** [More Information Needed]
- **Shared by [optional]:** [More Information Needed]
- **Model type:** [More Information Needed]
- **Language(s) (NLP):** [More Information Needed]
- **License:** [More Information Needed]
- **Finetuned from model [optional]:** [More Information Needed]
### Model Sources [optional]
<!-- Provide the basic links for the model. -->
- **Repository:** [More Information Needed]
- **Paper [optional]:** [More Information Needed]
- **Demo [optional]:** [More Information Needed]
## Uses
<!-- Address questions around how the model is intended to be used, including the foreseeable users of the model and those affected by the model. -->
### Direct Use
<!-- This section is for the model use without fine-tuning or plugging into a larger ecosystem/app. -->
[More Information Needed]
### Downstream Use [optional]
<!-- This section is for the model use when fine-tuned for a task, or when plugged into a larger ecosystem/app -->
[More Information Needed]
### Out-of-Scope Use
<!-- This section addresses misuse, malicious use, and uses that the model will not work well for. -->
[More Information Needed]
## Bias, Risks, and Limitations
<!-- This section is meant to convey both technical and sociotechnical limitations. -->
[More Information Needed]
### Recommendations
<!-- This section is meant to convey recommendations with respect to the bias, risk, and technical limitations. -->
Users (both direct and downstream) should be made aware of the risks, biases and limitations of the model. More information needed for further recommendations.
## How to Get Started with the Model
Use the code below to get started with the model.
[More Information Needed]
## Training Details
### Training Data
<!-- This should link to a Dataset Card, perhaps with a short stub of information on what the training data is all about as well as documentation related to data pre-processing or additional filtering. -->
[More Information Needed]
### Training Procedure
<!-- This relates heavily to the Technical Specifications. Content here should link to that section when it is relevant to the training procedure. -->
#### Preprocessing [optional]
[More Information Needed]
#### Training Hyperparameters
- **Training regime:** [More Information Needed] <!--fp32, fp16 mixed precision, bf16 mixed precision, bf16 non-mixed precision, fp16 non-mixed precision, fp8 mixed precision -->
#### Speeds, Sizes, Times [optional]
<!-- This section provides information about throughput, start/end time, checkpoint size if relevant, etc. -->
[More Information Needed]
## Evaluation
<!-- This section describes the evaluation protocols and provides the results. -->
### Testing Data, Factors & Metrics
#### Testing Data
<!-- This should link to a Dataset Card if possible. -->
[More Information Needed]
#### Factors
<!-- These are the things the evaluation is disaggregating by, e.g., subpopulations or domains. -->
[More Information Needed]
#### Metrics
<!-- These are the evaluation metrics being used, ideally with a description of why. -->
[More Information Needed]
### Results
[More Information Needed]
#### Summary
## Model Examination [optional]
<!-- Relevant interpretability work for the model goes here -->
[More Information Needed]
## Environmental Impact
<!-- Total emissions (in grams of CO2eq) and additional considerations, such as electricity usage, go here. Edit the suggested text below accordingly -->
Carbon emissions can be estimated using the [Machine Learning Impact calculator](https://mlco2.github.io/impact#compute) presented in [Lacoste et al. (2019)](https://arxiv.org/abs/1910.09700).
- **Hardware Type:** [More Information Needed]
- **Hours used:** [More Information Needed]
- **Cloud Provider:** [More Information Needed]
- **Compute Region:** [More Information Needed]
- **Carbon Emitted:** [More Information Needed]
## Technical Specifications [optional]
### Model Architecture and Objective
[More Information Needed]
### Compute Infrastructure
[More Information Needed]
#### Hardware
[More Information Needed]
#### Software
[More Information Needed]
## Citation [optional]
<!-- If there is a paper or blog post introducing the model, the APA and Bibtex information for that should go in this section. -->
**BibTeX:**
[More Information Needed]
**APA:**
[More Information Needed]
## Glossary [optional]
<!-- If relevant, include terms and calculations in this section that can help readers understand the model or model card. -->
[More Information Needed]
## More Information [optional]
[More Information Needed]
## Model Card Authors [optional]
[More Information Needed]
## Model Card Contact
[More Information Needed]
| [
"TRANSLATION"
] | Non_BioNLP |
smallsuper/distilbert-base-uncased-finetuned-emotion | smallsuper | text-classification | [
"transformers",
"pytorch",
"tensorboard",
"safetensors",
"distilbert",
"text-classification",
"generated_from_trainer",
"dataset:emotion",
"base_model:distilbert/distilbert-base-uncased",
"base_model:finetune:distilbert/distilbert-base-uncased",
"license:apache-2.0",
"model-index",
"autotrain_compatible",
"endpoints_compatible",
"region:us"
] | 1,678,252,425,000 | 2024-12-07T13:44:17 | 17 | 0 | ---
base_model: distilbert-base-uncased
datasets:
- emotion
license: apache-2.0
metrics:
- accuracy
- f1
tags:
- generated_from_trainer
model-index:
- name: distilbert-base-uncased-finetuned-emotion
results:
- task:
type: text-classification
name: Text Classification
dataset:
name: emotion
type: emotion
args: split
metrics:
- type: accuracy
value: 0.923
name: Accuracy
- type: f1
value: 0.9231021443963242
name: F1
---
<!-- This model card has been generated automatically according to the information the Trainer had access to. You
should probably proofread and complete it, then remove this comment. -->
# distilbert-base-uncased-finetuned-emotion
This model is a fine-tuned version of [distilbert-base-uncased](https://huggingface.co/distilbert-base-uncased) on the emotion dataset.
It achieves the following results on the evaluation set:
- Loss: 0.2143
- Accuracy: 0.923
- F1: 0.9231
## Model description
More information needed
## Intended uses & limitations
More information needed
## Training and evaluation data
More information needed
## Training procedure
### Training hyperparameters
The following hyperparameters were used during training:
- learning_rate: 2e-05
- train_batch_size: 64
- eval_batch_size: 64
- seed: 42
- optimizer: Adam with betas=(0.9,0.999) and epsilon=1e-08
- lr_scheduler_type: linear
- num_epochs: 2
### Training results
| Training Loss | Epoch | Step | Validation Loss | Accuracy | F1 |
|:-------------:|:-----:|:----:|:---------------:|:--------:|:------:|
| 0.8258 | 1.0 | 250 | 0.2989 | 0.9115 | 0.9098 |
| 0.242 | 2.0 | 500 | 0.2143 | 0.923 | 0.9231 |
### Framework versions
- Transformers 4.11.3
- Pytorch 1.13.1+cu117
- Datasets 2.9.0
- Tokenizers 0.10.3
| [
"TEXT_CLASSIFICATION"
] | Non_BioNLP |
wptoux/albert-chinese-large-qa | wptoux | question-answering | [
"transformers",
"pytorch",
"albert",
"question-answering",
"Question Answering",
"zh",
"dataset:webqa",
"dataset:dureader",
"license:apache-2.0",
"endpoints_compatible",
"region:us"
] | 1,646,263,745,000 | 2021-03-09T07:48:40 | 109 | 12 | ---
datasets:
- webqa
- dureader
language:
- zh
license: apache-2.0
tags:
- Question Answering
---
# albert-chinese-large-qa
Albert large QA model pretrained from baidu webqa and baidu dureader datasets.
## Data source
+ baidu webqa 1.0
+ baidu dureader
## Traing Method
We combined the two datasets together and created a new dataset in squad format, including 705139 samples for training and 69638 samples for validation.
We finetune the model based on the albert chinese large model.
## Hyperparams
+ learning_rate 1e-5
+ max_seq_length 512
+ max_query_length 50
+ max_answer_length 300
+ doc_stride 256
+ num_train_epochs 2
+ warmup_steps 1000
+ per_gpu_train_batch_size 8
+ gradient_accumulation_steps 3
+ n_gpu 2 (Nvidia Tesla P100)
## Usage
```
from transformers import AutoModelForQuestionAnswering, BertTokenizer
model = AutoModelForQuestionAnswering.from_pretrained('wptoux/albert-chinese-large-qa')
tokenizer = BertTokenizer.from_pretrained('wptoux/albert-chinese-large-qa')
```
***Important: use BertTokenizer***
## MoreInfo
Please visit https://github.com/wptoux/albert-chinese-large-webqa for details.
| [
"QUESTION_ANSWERING"
] | Non_BioNLP |
research-backup/mbart-large-cc25-ruquad-qa | research-backup | text2text-generation | [
"transformers",
"pytorch",
"mbart",
"text2text-generation",
"question answering",
"ru",
"dataset:lmqg/qg_ruquad",
"arxiv:2210.03992",
"license:cc-by-4.0",
"model-index",
"autotrain_compatible",
"endpoints_compatible",
"region:us"
] | 1,679,702,666,000 | 2023-03-25T00:10:19 | 23 | 0 | ---
datasets:
- lmqg/qg_ruquad
language: ru
license: cc-by-4.0
metrics:
- bleu4
- meteor
- rouge-l
- bertscore
- moverscore
pipeline_tag: text2text-generation
tags:
- question answering
widget:
- text: 'question: чем соответствует абсолютная погрешность скорости света ?, context:
Наивысшая точность измерений была достигнута в начале 1970-х. В 1975 году XV Генеральная
конференция по мерам и весам зафиксировала это положение и рекомендовала считать
скорость света, равной 299 792 458 м/с с относительной погрешностью 4•10−9, что
соответствует абсолютной погрешности 1,1 м/с. Впоследствии это значение скорости
света было положено в основу определения метра в Международной системе единиц
(СИ), а сама скорость света стала рассматриваться как фундаментальная физическая
постоянная, по определению равная указанному значению точно.'
example_title: Question Answering Example 1
- text: 'question: Какие начинания предпринял Lloyds в начале 1970-х годов?, context:
В начале 1970-х Lloyds начал расширять деятельность на международной арене, для
чего был создан Lloyds Bank International. География его деятельности включала
ФРГ, Швейцарию, Ближний Восток, Австралию, Канаду и США; к 1978 году Lloyds был
представлен в 43 странах. В 1972 году было создано подразделение страхования,
а в 1973 году была основана лизинговая компания Lloyds Leasing. В 1979 году банк
начал предоставлять услуги ипотечного кредитования (при покупке недвижимости стоимостью
от £25 000 до £150 000). В 1982 году начало работу агентство недвижимости Blackhorse
Agencies, к 1989 году у него было 563 отделения. В 1986 году сфера деятельности
Lloyds Bank PLC ещё больше расширилась с учреждением брокерской конторы и торгового
банка Lloyds Merchant Bank. В 1988 году была поглощена страховая компания Abbey
Life Group PLC; после объединения с ней всей своей страховой деятельности была
образована дочерняя компания Lloyds Abbey Life. В 1995 году Lloyds Bank Plc объединился
с TSB Group plc (группой, образованной в 1986 году из четырёх сберегательных банков
Trustee Savings Banks) под названием Lloyds TSB Bank plc. В 2000 году за £7 млрд
была поглощена шотландская взаимная страховая компания Scottish Widows.'
example_title: Question Answering Example 2
model-index:
- name: lmqg/mbart-large-cc25-ruquad-qa
results:
- task:
type: text2text-generation
name: Text2text Generation
dataset:
name: lmqg/qg_ruquad
type: default
args: default
metrics:
- type: bleu4_question_answering
value: 37.6
name: BLEU4 (Question Answering)
- type: rouge_l_question_answering
value: 57.83
name: ROUGE-L (Question Answering)
- type: meteor_question_answering
value: 43.12
name: METEOR (Question Answering)
- type: bertscore_question_answering
value: 96.01
name: BERTScore (Question Answering)
- type: moverscore_question_answering
value: 85.88
name: MoverScore (Question Answering)
- type: answer_f1_score__question_answering
value: 78.73
name: AnswerF1Score (Question Answering)
- type: answer_exact_match_question_answering
value: 58.02
name: AnswerExactMatch (Question Answering)
---
# Model Card of `lmqg/mbart-large-cc25-ruquad-qa`
This model is fine-tuned version of [facebook/mbart-large-cc25](https://huggingface.co/facebook/mbart-large-cc25) for question answering task on the [lmqg/qg_ruquad](https://huggingface.co/datasets/lmqg/qg_ruquad) (dataset_name: default) via [`lmqg`](https://github.com/asahi417/lm-question-generation).
### Overview
- **Language model:** [facebook/mbart-large-cc25](https://huggingface.co/facebook/mbart-large-cc25)
- **Language:** ru
- **Training data:** [lmqg/qg_ruquad](https://huggingface.co/datasets/lmqg/qg_ruquad) (default)
- **Online Demo:** [https://autoqg.net/](https://autoqg.net/)
- **Repository:** [https://github.com/asahi417/lm-question-generation](https://github.com/asahi417/lm-question-generation)
- **Paper:** [https://arxiv.org/abs/2210.03992](https://arxiv.org/abs/2210.03992)
### Usage
- With [`lmqg`](https://github.com/asahi417/lm-question-generation#lmqg-language-model-for-question-generation-)
```python
from lmqg import TransformersQG
# initialize model
model = TransformersQG(language="ru", model="lmqg/mbart-large-cc25-ruquad-qa")
# model prediction
answers = model.answer_q(list_question="чем соответствует абсолютная погрешность скорости света ?", list_context=" Наивысшая точность измерений была достигнута в начале 1970-х. В 1975 году XV Генеральная конференция по мерам и весам зафиксировала это положение и рекомендовала считать скорость света, равной 299 792 458 м/с с относительной погрешностью 4•10−9, что соответствует абсолютной погрешности 1,1 м/с. Впоследствии это значение скорости света было положено в основу определения метра в Международной системе единиц (СИ), а сама скорость света стала рассматриваться как фундаментальная физическая постоянная, по определению равная указанному значению точно.")
```
- With `transformers`
```python
from transformers import pipeline
pipe = pipeline("text2text-generation", "lmqg/mbart-large-cc25-ruquad-qa")
output = pipe("question: чем соответствует абсолютная погрешность скорости света ?, context: Наивысшая точность измерений была достигнута в начале 1970-х. В 1975 году XV Генеральная конференция по мерам и весам зафиксировала это положение и рекомендовала считать скорость света, равной 299 792 458 м/с с относительной погрешностью 4•10−9, что соответствует абсолютной погрешности 1,1 м/с. Впоследствии это значение скорости света было положено в основу определения метра в Международной системе единиц (СИ), а сама скорость света стала рассматриваться как фундаментальная физическая постоянная, по определению равная указанному значению точно.")
```
## Evaluation
- ***Metric (Question Answering)***: [raw metric file](https://huggingface.co/lmqg/mbart-large-cc25-ruquad-qa/raw/main/eval/metric.first.answer.paragraph_question.answer.lmqg_qg_ruquad.default.json)
| | Score | Type | Dataset |
|:-----------------|--------:|:--------|:-----------------------------------------------------------------|
| AnswerExactMatch | 58.02 | default | [lmqg/qg_ruquad](https://huggingface.co/datasets/lmqg/qg_ruquad) |
| AnswerF1Score | 78.73 | default | [lmqg/qg_ruquad](https://huggingface.co/datasets/lmqg/qg_ruquad) |
| BERTScore | 96.01 | default | [lmqg/qg_ruquad](https://huggingface.co/datasets/lmqg/qg_ruquad) |
| Bleu_1 | 54.16 | default | [lmqg/qg_ruquad](https://huggingface.co/datasets/lmqg/qg_ruquad) |
| Bleu_2 | 48.29 | default | [lmqg/qg_ruquad](https://huggingface.co/datasets/lmqg/qg_ruquad) |
| Bleu_3 | 42.95 | default | [lmqg/qg_ruquad](https://huggingface.co/datasets/lmqg/qg_ruquad) |
| Bleu_4 | 37.6 | default | [lmqg/qg_ruquad](https://huggingface.co/datasets/lmqg/qg_ruquad) |
| METEOR | 43.12 | default | [lmqg/qg_ruquad](https://huggingface.co/datasets/lmqg/qg_ruquad) |
| MoverScore | 85.88 | default | [lmqg/qg_ruquad](https://huggingface.co/datasets/lmqg/qg_ruquad) |
| ROUGE_L | 57.83 | default | [lmqg/qg_ruquad](https://huggingface.co/datasets/lmqg/qg_ruquad) |
## Training hyperparameters
The following hyperparameters were used during fine-tuning:
- dataset_path: lmqg/qg_ruquad
- dataset_name: default
- input_types: ['paragraph_question']
- output_types: ['answer']
- prefix_types: None
- model: facebook/mbart-large-cc25
- max_length: 512
- max_length_output: 32
- epoch: 15
- batch: 8
- lr: 0.0001
- fp16: False
- random_seed: 1
- gradient_accumulation_steps: 8
- label_smoothing: 0.15
The full configuration can be found at [fine-tuning config file](https://huggingface.co/lmqg/mbart-large-cc25-ruquad-qa/raw/main/trainer_config.json).
## Citation
```
@inproceedings{ushio-etal-2022-generative,
title = "{G}enerative {L}anguage {M}odels for {P}aragraph-{L}evel {Q}uestion {G}eneration",
author = "Ushio, Asahi and
Alva-Manchego, Fernando and
Camacho-Collados, Jose",
booktitle = "Proceedings of the 2022 Conference on Empirical Methods in Natural Language Processing",
month = dec,
year = "2022",
address = "Abu Dhabi, U.A.E.",
publisher = "Association for Computational Linguistics",
}
```
| [
"QUESTION_ANSWERING"
] | Non_BioNLP |
Mollel/swahili-serengeti-E250-nli-matryoshka | Mollel | sentence-similarity | [
"sentence-transformers",
"safetensors",
"electra",
"sentence-similarity",
"feature-extraction",
"generated_from_trainer",
"dataset_size:557850",
"loss:MatryoshkaLoss",
"loss:MultipleNegativesRankingLoss",
"arxiv:1908.10084",
"arxiv:2205.13147",
"arxiv:1705.00652",
"base_model:UBC-NLP/serengeti-E250",
"base_model:finetune:UBC-NLP/serengeti-E250",
"model-index",
"autotrain_compatible",
"endpoints_compatible",
"region:us"
] | 1,720,222,800,000 | 2024-07-05T23:49:25 | 5 | 1 | ---
base_model: UBC-NLP/serengeti-E250
datasets: []
language: []
library_name: sentence-transformers
metrics:
- pearson_cosine
- spearman_cosine
- pearson_manhattan
- spearman_manhattan
- pearson_euclidean
- spearman_euclidean
- pearson_dot
- spearman_dot
- pearson_max
- spearman_max
pipeline_tag: sentence-similarity
tags:
- sentence-transformers
- sentence-similarity
- feature-extraction
- generated_from_trainer
- dataset_size:557850
- loss:MatryoshkaLoss
- loss:MultipleNegativesRankingLoss
widget:
- source_sentence: Mwanamume aliyepangwa vizuri anasimama kwa mguu mmoja karibu na
pwani safi ya bahari.
sentences:
- mtu anacheka wakati wa kufua nguo
- Mwanamume fulani yuko nje karibu na ufuo wa bahari.
- Mwanamume fulani ameketi kwenye sofa yake.
- source_sentence: Mwanamume mwenye ngozi nyeusi akivuta sigareti karibu na chombo
cha taka cha kijani.
sentences:
- Karibu na chombo cha taka mwanamume huyo alisimama na kuvuta sigareti
- Kitanda ni chafu.
- Alipokuwa kwenye dimbwi la kuogelea mvulana huyo mwenye ugonjwa wa albino alijihadhari
na jua kupita kiasi
- source_sentence: Mwanamume kijana mwenye nywele nyekundu anaketi ukutani akisoma
gazeti huku mwanamke na msichana mchanga wakipita.
sentences:
- Mwanamume aliyevalia shati la bluu amegonga ukuta kando ya barabara na gari la
bluu na gari nyekundu lenye maji nyuma.
- Mwanamume mchanga anatazama gazeti huku wanawake wawili wakipita karibu naye.
- Mwanamume huyo mchanga analala huku Mama akimwongoza binti yake kwenye bustani.
- source_sentence: Wasichana wako nje.
sentences:
- Wasichana wawili wakisafiri kwenye sehemu ya kusisimua.
- Kuna watu watatu wakiongoza gari linaloweza kugeuzwa-geuzwa wakipita watu wengine.
- Wasichana watatu wamesimama pamoja katika chumba, mmoja anasikiliza, mwingine
anaandika ukutani na wa tatu anaongea nao.
- source_sentence: Mwanamume aliyevalia koti la bluu la kuzuia upepo, amelala uso
chini kwenye benchi ya bustani, akiwa na chupa ya pombe iliyofungwa kwenye mojawapo
ya miguu ya benchi.
sentences:
- Mwanamume amelala uso chini kwenye benchi ya bustani.
- Mwanamke anaunganisha uzi katika mipira kando ya rundo la mipira
- Mwanamume fulani anacheza dansi kwenye klabu hiyo akifungua chupa.
model-index:
- name: SentenceTransformer based on UBC-NLP/serengeti-E250
results:
- task:
type: semantic-similarity
name: Semantic Similarity
dataset:
name: sts test 768
type: sts-test-768
metrics:
- type: pearson_cosine
value: 0.7113368462970326
name: Pearson Cosine
- type: spearman_cosine
value: 0.706531149090894
name: Spearman Cosine
- type: pearson_manhattan
value: 0.7134349154531519
name: Pearson Manhattan
- type: spearman_manhattan
value: 0.7023005843725415
name: Spearman Manhattan
- type: pearson_euclidean
value: 0.7137962920501839
name: Pearson Euclidean
- type: spearman_euclidean
value: 0.7020941994285994
name: Spearman Euclidean
- type: pearson_dot
value: 0.3920803758314358
name: Pearson Dot
- type: spearman_dot
value: 0.3601086266312748
name: Spearman Dot
- type: pearson_max
value: 0.7137962920501839
name: Pearson Max
- type: spearman_max
value: 0.706531149090894
name: Spearman Max
- task:
type: semantic-similarity
name: Semantic Similarity
dataset:
name: sts test 512
type: sts-test-512
metrics:
- type: pearson_cosine
value: 0.7090618585285485
name: Pearson Cosine
- type: spearman_cosine
value: 0.7045766195278508
name: Spearman Cosine
- type: pearson_manhattan
value: 0.7129955390384859
name: Pearson Manhattan
- type: spearman_manhattan
value: 0.7021695501159393
name: Spearman Manhattan
- type: pearson_euclidean
value: 0.7138697740168334
name: Pearson Euclidean
- type: spearman_euclidean
value: 0.7032055408694606
name: Spearman Euclidean
- type: pearson_dot
value: 0.39352767760073326
name: Pearson Dot
- type: spearman_dot
value: 0.3628376619678567
name: Spearman Dot
- type: pearson_max
value: 0.7138697740168334
name: Pearson Max
- type: spearman_max
value: 0.7045766195278508
name: Spearman Max
- task:
type: semantic-similarity
name: Semantic Similarity
dataset:
name: sts test 256
type: sts-test-256
metrics:
- type: pearson_cosine
value: 0.7067837420770313
name: Pearson Cosine
- type: spearman_cosine
value: 0.7044452613349608
name: Spearman Cosine
- type: pearson_manhattan
value: 0.7137425083925593
name: Pearson Manhattan
- type: spearman_manhattan
value: 0.7032345257234871
name: Spearman Manhattan
- type: pearson_euclidean
value: 0.7146861583047366
name: Pearson Euclidean
- type: spearman_euclidean
value: 0.7039212190752775
name: Spearman Euclidean
- type: pearson_dot
value: 0.37462153895392747
name: Pearson Dot
- type: spearman_dot
value: 0.34441190254194326
name: Spearman Dot
- type: pearson_max
value: 0.7146861583047366
name: Pearson Max
- type: spearman_max
value: 0.7044452613349608
name: Spearman Max
- task:
type: semantic-similarity
name: Semantic Similarity
dataset:
name: sts test 128
type: sts-test-128
metrics:
- type: pearson_cosine
value: 0.7046839100746249
name: Pearson Cosine
- type: spearman_cosine
value: 0.7050559450173808
name: Spearman Cosine
- type: pearson_manhattan
value: 0.7120431790616113
name: Pearson Manhattan
- type: spearman_manhattan
value: 0.7010054121016321
name: Spearman Manhattan
- type: pearson_euclidean
value: 0.7132280398983044
name: Pearson Euclidean
- type: spearman_euclidean
value: 0.701626975970973
name: Spearman Euclidean
- type: pearson_dot
value: 0.35455409787695585
name: Pearson Dot
- type: spearman_dot
value: 0.32292034736383524
name: Spearman Dot
- type: pearson_max
value: 0.7132280398983044
name: Pearson Max
- type: spearman_max
value: 0.7050559450173808
name: Spearman Max
- task:
type: semantic-similarity
name: Semantic Similarity
dataset:
name: sts test 64
type: sts-test-64
metrics:
- type: pearson_cosine
value: 0.7012310578605567
name: Pearson Cosine
- type: spearman_cosine
value: 0.7044132231714119
name: Spearman Cosine
- type: pearson_manhattan
value: 0.7091211798265005
name: Pearson Manhattan
- type: spearman_manhattan
value: 0.6972792688781575
name: Spearman Manhattan
- type: pearson_euclidean
value: 0.7103033981031003
name: Pearson Euclidean
- type: spearman_euclidean
value: 0.6985716335223231
name: Spearman Euclidean
- type: pearson_dot
value: 0.3379821887901175
name: Pearson Dot
- type: spearman_dot
value: 0.30513652558145304
name: Spearman Dot
- type: pearson_max
value: 0.7103033981031003
name: Pearson Max
- type: spearman_max
value: 0.7044132231714119
name: Spearman Max
---
# SentenceTransformer based on UBC-NLP/serengeti-E250
This is a [sentence-transformers](https://www.SBERT.net) model finetuned from [UBC-NLP/serengeti-E250](https://huggingface.co/UBC-NLP/serengeti-E250). It maps sentences & paragraphs to a 768-dimensional dense vector space and can be used for semantic textual similarity, semantic search, paraphrase mining, text classification, clustering, and more.
## Model Details
### Model Description
- **Model Type:** Sentence Transformer
- **Base model:** [UBC-NLP/serengeti-E250](https://huggingface.co/UBC-NLP/serengeti-E250) <!-- at revision 41b5b8b6179c4af2859768cbf4f0f03e928d651d -->
- **Maximum Sequence Length:** 512 tokens
- **Output Dimensionality:** 768 tokens
- **Similarity Function:** Cosine Similarity
<!-- - **Training Dataset:** Unknown -->
<!-- - **Language:** Unknown -->
<!-- - **License:** Unknown -->
### Model Sources
- **Documentation:** [Sentence Transformers Documentation](https://sbert.net)
- **Repository:** [Sentence Transformers on GitHub](https://github.com/UKPLab/sentence-transformers)
- **Hugging Face:** [Sentence Transformers on Hugging Face](https://huggingface.co/models?library=sentence-transformers)
### Full Model Architecture
```
SentenceTransformer(
(0): Transformer({'max_seq_length': 512, 'do_lower_case': False}) with Transformer model: ElectraModel
(1): Pooling({'word_embedding_dimension': 768, 'pooling_mode_cls_token': False, 'pooling_mode_mean_tokens': True, 'pooling_mode_max_tokens': False, 'pooling_mode_mean_sqrt_len_tokens': False, 'pooling_mode_weightedmean_tokens': False, 'pooling_mode_lasttoken': False, 'include_prompt': True})
)
```
## Usage
### Direct Usage (Sentence Transformers)
First install the Sentence Transformers library:
```bash
pip install -U sentence-transformers
```
Then you can load this model and run inference.
```python
from sentence_transformers import SentenceTransformer
# Download from the 🤗 Hub
model = SentenceTransformer("Mollel/swahili-serengeti-E250-nli-matryoshka")
# Run inference
sentences = [
'Mwanamume aliyevalia koti la bluu la kuzuia upepo, amelala uso chini kwenye benchi ya bustani, akiwa na chupa ya pombe iliyofungwa kwenye mojawapo ya miguu ya benchi.',
'Mwanamume amelala uso chini kwenye benchi ya bustani.',
'Mwanamume fulani anacheza dansi kwenye klabu hiyo akifungua chupa.',
]
embeddings = model.encode(sentences)
print(embeddings.shape)
# [3, 768]
# Get the similarity scores for the embeddings
similarities = model.similarity(embeddings, embeddings)
print(similarities.shape)
# [3, 3]
```
<!--
### Direct Usage (Transformers)
<details><summary>Click to see the direct usage in Transformers</summary>
</details>
-->
<!--
### Downstream Usage (Sentence Transformers)
You can finetune this model on your own dataset.
<details><summary>Click to expand</summary>
</details>
-->
<!--
### Out-of-Scope Use
*List how the model may foreseeably be misused and address what users ought not to do with the model.*
-->
## Evaluation
### Metrics
#### Semantic Similarity
* Dataset: `sts-test-768`
* Evaluated with [<code>EmbeddingSimilarityEvaluator</code>](https://sbert.net/docs/package_reference/sentence_transformer/evaluation.html#sentence_transformers.evaluation.EmbeddingSimilarityEvaluator)
| Metric | Value |
|:--------------------|:-----------|
| pearson_cosine | 0.7113 |
| **spearman_cosine** | **0.7065** |
| pearson_manhattan | 0.7134 |
| spearman_manhattan | 0.7023 |
| pearson_euclidean | 0.7138 |
| spearman_euclidean | 0.7021 |
| pearson_dot | 0.3921 |
| spearman_dot | 0.3601 |
| pearson_max | 0.7138 |
| spearman_max | 0.7065 |
#### Semantic Similarity
* Dataset: `sts-test-512`
* Evaluated with [<code>EmbeddingSimilarityEvaluator</code>](https://sbert.net/docs/package_reference/sentence_transformer/evaluation.html#sentence_transformers.evaluation.EmbeddingSimilarityEvaluator)
| Metric | Value |
|:--------------------|:-----------|
| pearson_cosine | 0.7091 |
| **spearman_cosine** | **0.7046** |
| pearson_manhattan | 0.713 |
| spearman_manhattan | 0.7022 |
| pearson_euclidean | 0.7139 |
| spearman_euclidean | 0.7032 |
| pearson_dot | 0.3935 |
| spearman_dot | 0.3628 |
| pearson_max | 0.7139 |
| spearman_max | 0.7046 |
#### Semantic Similarity
* Dataset: `sts-test-256`
* Evaluated with [<code>EmbeddingSimilarityEvaluator</code>](https://sbert.net/docs/package_reference/sentence_transformer/evaluation.html#sentence_transformers.evaluation.EmbeddingSimilarityEvaluator)
| Metric | Value |
|:--------------------|:-----------|
| pearson_cosine | 0.7068 |
| **spearman_cosine** | **0.7044** |
| pearson_manhattan | 0.7137 |
| spearman_manhattan | 0.7032 |
| pearson_euclidean | 0.7147 |
| spearman_euclidean | 0.7039 |
| pearson_dot | 0.3746 |
| spearman_dot | 0.3444 |
| pearson_max | 0.7147 |
| spearman_max | 0.7044 |
#### Semantic Similarity
* Dataset: `sts-test-128`
* Evaluated with [<code>EmbeddingSimilarityEvaluator</code>](https://sbert.net/docs/package_reference/sentence_transformer/evaluation.html#sentence_transformers.evaluation.EmbeddingSimilarityEvaluator)
| Metric | Value |
|:--------------------|:-----------|
| pearson_cosine | 0.7047 |
| **spearman_cosine** | **0.7051** |
| pearson_manhattan | 0.712 |
| spearman_manhattan | 0.701 |
| pearson_euclidean | 0.7132 |
| spearman_euclidean | 0.7016 |
| pearson_dot | 0.3546 |
| spearman_dot | 0.3229 |
| pearson_max | 0.7132 |
| spearman_max | 0.7051 |
#### Semantic Similarity
* Dataset: `sts-test-64`
* Evaluated with [<code>EmbeddingSimilarityEvaluator</code>](https://sbert.net/docs/package_reference/sentence_transformer/evaluation.html#sentence_transformers.evaluation.EmbeddingSimilarityEvaluator)
| Metric | Value |
|:--------------------|:-----------|
| pearson_cosine | 0.7012 |
| **spearman_cosine** | **0.7044** |
| pearson_manhattan | 0.7091 |
| spearman_manhattan | 0.6973 |
| pearson_euclidean | 0.7103 |
| spearman_euclidean | 0.6986 |
| pearson_dot | 0.338 |
| spearman_dot | 0.3051 |
| pearson_max | 0.7103 |
| spearman_max | 0.7044 |
<!--
## Bias, Risks and Limitations
*What are the known or foreseeable issues stemming from this model? You could also flag here known failure cases or weaknesses of the model.*
-->
<!--
### Recommendations
*What are recommendations with respect to the foreseeable issues? For example, filtering explicit content.*
-->
## Training Details
### Training Hyperparameters
#### Non-Default Hyperparameters
- `per_device_train_batch_size`: 16
- `per_device_eval_batch_size`: 16
- `learning_rate`: 2e-05
- `num_train_epochs`: 1
- `warmup_ratio`: 0.1
- `bf16`: True
- `batch_sampler`: no_duplicates
#### All Hyperparameters
<details><summary>Click to expand</summary>
- `overwrite_output_dir`: False
- `do_predict`: False
- `prediction_loss_only`: True
- `per_device_train_batch_size`: 16
- `per_device_eval_batch_size`: 16
- `per_gpu_train_batch_size`: None
- `per_gpu_eval_batch_size`: None
- `gradient_accumulation_steps`: 1
- `eval_accumulation_steps`: None
- `learning_rate`: 2e-05
- `weight_decay`: 0.0
- `adam_beta1`: 0.9
- `adam_beta2`: 0.999
- `adam_epsilon`: 1e-08
- `max_grad_norm`: 1.0
- `num_train_epochs`: 1
- `max_steps`: -1
- `lr_scheduler_type`: linear
- `lr_scheduler_kwargs`: {}
- `warmup_ratio`: 0.1
- `warmup_steps`: 0
- `log_level`: passive
- `log_level_replica`: warning
- `log_on_each_node`: True
- `logging_nan_inf_filter`: True
- `save_safetensors`: True
- `save_on_each_node`: False
- `save_only_model`: False
- `no_cuda`: False
- `use_cpu`: False
- `use_mps_device`: False
- `seed`: 42
- `data_seed`: None
- `jit_mode_eval`: False
- `use_ipex`: False
- `bf16`: True
- `fp16`: False
- `fp16_opt_level`: O1
- `half_precision_backend`: auto
- `bf16_full_eval`: False
- `fp16_full_eval`: False
- `tf32`: None
- `local_rank`: 0
- `ddp_backend`: None
- `tpu_num_cores`: None
- `tpu_metrics_debug`: False
- `debug`: []
- `dataloader_drop_last`: False
- `dataloader_num_workers`: 0
- `dataloader_prefetch_factor`: None
- `past_index`: -1
- `disable_tqdm`: False
- `remove_unused_columns`: True
- `label_names`: None
- `load_best_model_at_end`: False
- `ignore_data_skip`: False
- `fsdp`: []
- `fsdp_min_num_params`: 0
- `fsdp_config`: {'min_num_params': 0, 'xla': False, 'xla_fsdp_v2': False, 'xla_fsdp_grad_ckpt': False}
- `fsdp_transformer_layer_cls_to_wrap`: None
- `accelerator_config`: {'split_batches': False, 'dispatch_batches': None, 'even_batches': True, 'use_seedable_sampler': True, 'gradient_accumulation_kwargs': None}
- `deepspeed`: None
- `label_smoothing_factor`: 0.0
- `optim`: adamw_torch
- `optim_args`: None
- `adafactor`: False
- `group_by_length`: False
- `length_column_name`: length
- `ddp_find_unused_parameters`: None
- `ddp_bucket_cap_mb`: None
- `ddp_broadcast_buffers`: False
- `dataloader_pin_memory`: True
- `dataloader_persistent_workers`: False
- `skip_memory_metrics`: True
- `use_legacy_prediction_loop`: False
- `push_to_hub`: False
- `resume_from_checkpoint`: None
- `hub_model_id`: None
- `hub_strategy`: every_save
- `hub_private_repo`: False
- `hub_always_push`: False
- `gradient_checkpointing`: False
- `gradient_checkpointing_kwargs`: None
- `include_inputs_for_metrics`: False
- `eval_do_concat_batches`: True
- `fp16_backend`: auto
- `push_to_hub_model_id`: None
- `push_to_hub_organization`: None
- `mp_parameters`:
- `auto_find_batch_size`: False
- `full_determinism`: False
- `torchdynamo`: None
- `ray_scope`: last
- `ddp_timeout`: 1800
- `torch_compile`: False
- `torch_compile_backend`: None
- `torch_compile_mode`: None
- `dispatch_batches`: None
- `split_batches`: None
- `include_tokens_per_second`: False
- `include_num_input_tokens_seen`: False
- `neftune_noise_alpha`: None
- `optim_target_modules`: None
- `batch_sampler`: no_duplicates
- `multi_dataset_batch_sampler`: proportional
</details>
### Training Logs
<details><summary>Click to expand</summary>
| Epoch | Step | Training Loss | sts-test-128_spearman_cosine | sts-test-256_spearman_cosine | sts-test-512_spearman_cosine | sts-test-64_spearman_cosine | sts-test-768_spearman_cosine |
|:------:|:-----:|:-------------:|:----------------------------:|:----------------------------:|:----------------------------:|:---------------------------:|:----------------------------:|
| 0.0057 | 100 | 25.7713 | - | - | - | - | - |
| 0.0115 | 200 | 20.7886 | - | - | - | - | - |
| 0.0172 | 300 | 17.0398 | - | - | - | - | - |
| 0.0229 | 400 | 15.3913 | - | - | - | - | - |
| 0.0287 | 500 | 14.0214 | - | - | - | - | - |
| 0.0344 | 600 | 12.2125 | - | - | - | - | - |
| 0.0402 | 700 | 10.3033 | - | - | - | - | - |
| 0.0459 | 800 | 9.3822 | - | - | - | - | - |
| 0.0516 | 900 | 8.9276 | - | - | - | - | - |
| 0.0574 | 1000 | 8.552 | - | - | - | - | - |
| 0.0631 | 1100 | 8.6293 | - | - | - | - | - |
| 0.0688 | 1200 | 8.5353 | - | - | - | - | - |
| 0.0746 | 1300 | 8.6431 | - | - | - | - | - |
| 0.0803 | 1400 | 8.3192 | - | - | - | - | - |
| 0.0860 | 1500 | 7.1834 | - | - | - | - | - |
| 0.0918 | 1600 | 6.7834 | - | - | - | - | - |
| 0.0975 | 1700 | 6.4758 | - | - | - | - | - |
| 0.1033 | 1800 | 6.756 | - | - | - | - | - |
| 0.1090 | 1900 | 7.807 | - | - | - | - | - |
| 0.1147 | 2000 | 6.8836 | - | - | - | - | - |
| 0.1205 | 2100 | 6.9948 | - | - | - | - | - |
| 0.1262 | 2200 | 6.5031 | - | - | - | - | - |
| 0.1319 | 2300 | 6.3596 | - | - | - | - | - |
| 0.1377 | 2400 | 6.0257 | - | - | - | - | - |
| 0.1434 | 2500 | 5.9757 | - | - | - | - | - |
| 0.1491 | 2600 | 5.464 | - | - | - | - | - |
| 0.1549 | 2700 | 5.6518 | - | - | - | - | - |
| 0.1606 | 2800 | 6.2899 | - | - | - | - | - |
| 0.1664 | 2900 | 6.4876 | - | - | - | - | - |
| 0.1721 | 3000 | 6.9466 | - | - | - | - | - |
| 0.1778 | 3100 | 6.8439 | - | - | - | - | - |
| 0.1836 | 3200 | 6.2545 | - | - | - | - | - |
| 0.1893 | 3300 | 5.9795 | - | - | - | - | - |
| 0.1950 | 3400 | 5.3904 | - | - | - | - | - |
| 0.2008 | 3500 | 6.2798 | - | - | - | - | - |
| 0.2065 | 3600 | 5.6882 | - | - | - | - | - |
| 0.2122 | 3700 | 6.195 | - | - | - | - | - |
| 0.2180 | 3800 | 5.8728 | - | - | - | - | - |
| 0.2237 | 3900 | 6.2428 | - | - | - | - | - |
| 0.2294 | 4000 | 5.801 | - | - | - | - | - |
| 0.2352 | 4100 | 5.6918 | - | - | - | - | - |
| 0.2409 | 4200 | 5.3977 | - | - | - | - | - |
| 0.2467 | 4300 | 5.8792 | - | - | - | - | - |
| 0.2524 | 4400 | 5.9297 | - | - | - | - | - |
| 0.2581 | 4500 | 6.161 | - | - | - | - | - |
| 0.2639 | 4600 | 5.6571 | - | - | - | - | - |
| 0.2696 | 4700 | 5.5849 | - | - | - | - | - |
| 0.2753 | 4800 | 5.6382 | - | - | - | - | - |
| 0.2811 | 4900 | 5.2978 | - | - | - | - | - |
| 0.2868 | 5000 | 5.108 | - | - | - | - | - |
| 0.2925 | 5100 | 5.1158 | - | - | - | - | - |
| 0.2983 | 5200 | 5.6218 | - | - | - | - | - |
| 0.3040 | 5300 | 5.643 | - | - | - | - | - |
| 0.3098 | 5400 | 5.6894 | - | - | - | - | - |
| 0.3155 | 5500 | 5.373 | - | - | - | - | - |
| 0.3212 | 5600 | 5.0673 | - | - | - | - | - |
| 0.3270 | 5700 | 5.1915 | - | - | - | - | - |
| 0.3327 | 5800 | 5.3705 | - | - | - | - | - |
| 0.3384 | 5900 | 5.6432 | - | - | - | - | - |
| 0.3442 | 6000 | 5.2567 | - | - | - | - | - |
| 0.3499 | 6100 | 5.4516 | - | - | - | - | - |
| 0.3556 | 6200 | 5.4844 | - | - | - | - | - |
| 0.3614 | 6300 | 4.8238 | - | - | - | - | - |
| 0.3671 | 6400 | 4.8271 | - | - | - | - | - |
| 0.3729 | 6500 | 4.9863 | - | - | - | - | - |
| 0.3786 | 6600 | 5.4894 | - | - | - | - | - |
| 0.3843 | 6700 | 4.95 | - | - | - | - | - |
| 0.3901 | 6800 | 5.0881 | - | - | - | - | - |
| 0.3958 | 6900 | 5.249 | - | - | - | - | - |
| 0.4015 | 7000 | 5.0082 | - | - | - | - | - |
| 0.4073 | 7100 | 5.5064 | - | - | - | - | - |
| 0.4130 | 7200 | 5.0885 | - | - | - | - | - |
| 0.4187 | 7300 | 5.0321 | - | - | - | - | - |
| 0.4245 | 7400 | 4.8212 | - | - | - | - | - |
| 0.4302 | 7500 | 5.4231 | - | - | - | - | - |
| 0.4360 | 7600 | 4.7687 | - | - | - | - | - |
| 0.4417 | 7700 | 4.5707 | - | - | - | - | - |
| 0.4474 | 7800 | 5.2229 | - | - | - | - | - |
| 0.4532 | 7900 | 5.2446 | - | - | - | - | - |
| 0.4589 | 8000 | 4.682 | - | - | - | - | - |
| 0.4646 | 8100 | 4.888 | - | - | - | - | - |
| 0.4704 | 8200 | 5.0496 | - | - | - | - | - |
| 0.4761 | 8300 | 4.7089 | - | - | - | - | - |
| 0.4818 | 8400 | 4.9567 | - | - | - | - | - |
| 0.4876 | 8500 | 4.7913 | - | - | - | - | - |
| 0.4933 | 8600 | 4.8904 | - | - | - | - | - |
| 0.4991 | 8700 | 5.247 | - | - | - | - | - |
| 0.5048 | 8800 | 4.8254 | - | - | - | - | - |
| 0.5105 | 8900 | 4.973 | - | - | - | - | - |
| 0.5163 | 9000 | 4.6657 | - | - | - | - | - |
| 0.5220 | 9100 | 4.9224 | - | - | - | - | - |
| 0.5277 | 9200 | 4.8163 | - | - | - | - | - |
| 0.5335 | 9300 | 4.3673 | - | - | - | - | - |
| 0.5392 | 9400 | 4.6509 | - | - | - | - | - |
| 0.5449 | 9500 | 5.0667 | - | - | - | - | - |
| 0.5507 | 9600 | 4.8771 | - | - | - | - | - |
| 0.5564 | 9700 | 5.1056 | - | - | - | - | - |
| 0.5622 | 9800 | 4.8297 | - | - | - | - | - |
| 0.5679 | 9900 | 5.0156 | - | - | - | - | - |
| 0.5736 | 10000 | 5.0758 | - | - | - | - | - |
| 0.5794 | 10100 | 4.9551 | - | - | - | - | - |
| 0.5851 | 10200 | 4.9594 | - | - | - | - | - |
| 0.5908 | 10300 | 5.136 | - | - | - | - | - |
| 0.5966 | 10400 | 4.7873 | - | - | - | - | - |
| 0.6023 | 10500 | 4.5154 | - | - | - | - | - |
| 0.6080 | 10600 | 4.928 | - | - | - | - | - |
| 0.6138 | 10700 | 5.1825 | - | - | - | - | - |
| 0.6195 | 10800 | 5.046 | - | - | - | - | - |
| 0.6253 | 10900 | 5.0111 | - | - | - | - | - |
| 0.6310 | 11000 | 4.9458 | - | - | - | - | - |
| 0.6367 | 11100 | 5.188 | - | - | - | - | - |
| 0.6425 | 11200 | 4.6219 | - | - | - | - | - |
| 0.6482 | 11300 | 5.3367 | - | - | - | - | - |
| 0.6539 | 11400 | 4.9851 | - | - | - | - | - |
| 0.6597 | 11500 | 5.2068 | - | - | - | - | - |
| 0.6654 | 11600 | 4.3789 | - | - | - | - | - |
| 0.6711 | 11700 | 5.3533 | - | - | - | - | - |
| 0.6769 | 11800 | 5.3983 | - | - | - | - | - |
| 0.6826 | 11900 | 4.6 | - | - | - | - | - |
| 0.6883 | 12000 | 4.6668 | - | - | - | - | - |
| 0.6941 | 12100 | 5.0814 | - | - | - | - | - |
| 0.6998 | 12200 | 5.0787 | - | - | - | - | - |
| 0.7056 | 12300 | 4.6325 | - | - | - | - | - |
| 0.7113 | 12400 | 4.9415 | - | - | - | - | - |
| 0.7170 | 12500 | 4.7053 | - | - | - | - | - |
| 0.7228 | 12600 | 4.3212 | - | - | - | - | - |
| 0.7285 | 12700 | 4.8205 | - | - | - | - | - |
| 0.7342 | 12800 | 4.8602 | - | - | - | - | - |
| 0.7400 | 12900 | 4.6944 | - | - | - | - | - |
| 0.7457 | 13000 | 4.7785 | - | - | - | - | - |
| 0.7514 | 13100 | 4.3515 | - | - | - | - | - |
| 0.7572 | 13200 | 5.7561 | - | - | - | - | - |
| 0.7629 | 13300 | 5.3526 | - | - | - | - | - |
| 0.7687 | 13400 | 5.187 | - | - | - | - | - |
| 0.7744 | 13500 | 5.0143 | - | - | - | - | - |
| 0.7801 | 13600 | 4.515 | - | - | - | - | - |
| 0.7859 | 13700 | 4.639 | - | - | - | - | - |
| 0.7916 | 13800 | 4.5556 | - | - | - | - | - |
| 0.7973 | 13900 | 4.3526 | - | - | - | - | - |
| 0.8031 | 14000 | 4.3091 | - | - | - | - | - |
| 0.8088 | 14100 | 4.1761 | - | - | - | - | - |
| 0.8145 | 14200 | 4.0484 | - | - | - | - | - |
| 0.8203 | 14300 | 4.1886 | - | - | - | - | - |
| 0.8260 | 14400 | 4.237 | - | - | - | - | - |
| 0.8318 | 14500 | 4.2167 | - | - | - | - | - |
| 0.8375 | 14600 | 4.0329 | - | - | - | - | - |
| 0.8432 | 14700 | 3.9902 | - | - | - | - | - |
| 0.8490 | 14800 | 3.8211 | - | - | - | - | - |
| 0.8547 | 14900 | 4.0048 | - | - | - | - | - |
| 0.8604 | 15000 | 3.7979 | - | - | - | - | - |
| 0.8662 | 15100 | 3.8117 | - | - | - | - | - |
| 0.8719 | 15200 | 3.909 | - | - | - | - | - |
| 0.8776 | 15300 | 3.8526 | - | - | - | - | - |
| 0.8834 | 15400 | 3.79 | - | - | - | - | - |
| 0.8891 | 15500 | 3.7792 | - | - | - | - | - |
| 0.8949 | 15600 | 3.7469 | - | - | - | - | - |
| 0.9006 | 15700 | 3.8387 | - | - | - | - | - |
| 0.9063 | 15800 | 3.6418 | - | - | - | - | - |
| 0.9121 | 15900 | 3.645 | - | - | - | - | - |
| 0.9178 | 16000 | 3.4861 | - | - | - | - | - |
| 0.9235 | 16100 | 3.6416 | - | - | - | - | - |
| 0.9293 | 16200 | 3.6665 | - | - | - | - | - |
| 0.9350 | 16300 | 3.6809 | - | - | - | - | - |
| 0.9407 | 16400 | 3.7944 | - | - | - | - | - |
| 0.9465 | 16500 | 3.6585 | - | - | - | - | - |
| 0.9522 | 16600 | 3.5398 | - | - | - | - | - |
| 0.9580 | 16700 | 3.7036 | - | - | - | - | - |
| 0.9637 | 16800 | 3.6386 | - | - | - | - | - |
| 0.9694 | 16900 | 3.5501 | - | - | - | - | - |
| 0.9752 | 17000 | 3.7957 | - | - | - | - | - |
| 0.9809 | 17100 | 3.6076 | - | - | - | - | - |
| 0.9866 | 17200 | 3.4653 | - | - | - | - | - |
| 0.9924 | 17300 | 3.6768 | - | - | - | - | - |
| 0.9981 | 17400 | 3.49 | - | - | - | - | - |
| 1.0 | 17433 | - | 0.7051 | 0.7044 | 0.7046 | 0.7044 | 0.7065 |
</details>
### Framework Versions
- Python: 3.11.9
- Sentence Transformers: 3.0.1
- Transformers: 4.40.1
- PyTorch: 2.3.0+cu121
- Accelerate: 0.29.3
- Datasets: 2.19.0
- Tokenizers: 0.19.1
## Citation
### BibTeX
#### Sentence Transformers
```bibtex
@inproceedings{reimers-2019-sentence-bert,
title = "Sentence-BERT: Sentence Embeddings using Siamese BERT-Networks",
author = "Reimers, Nils and Gurevych, Iryna",
booktitle = "Proceedings of the 2019 Conference on Empirical Methods in Natural Language Processing",
month = "11",
year = "2019",
publisher = "Association for Computational Linguistics",
url = "https://arxiv.org/abs/1908.10084",
}
```
#### MatryoshkaLoss
```bibtex
@misc{kusupati2024matryoshka,
title={Matryoshka Representation Learning},
author={Aditya Kusupati and Gantavya Bhatt and Aniket Rege and Matthew Wallingford and Aditya Sinha and Vivek Ramanujan and William Howard-Snyder and Kaifeng Chen and Sham Kakade and Prateek Jain and Ali Farhadi},
year={2024},
eprint={2205.13147},
archivePrefix={arXiv},
primaryClass={cs.LG}
}
```
#### MultipleNegativesRankingLoss
```bibtex
@misc{henderson2017efficient,
title={Efficient Natural Language Response Suggestion for Smart Reply},
author={Matthew Henderson and Rami Al-Rfou and Brian Strope and Yun-hsuan Sung and Laszlo Lukacs and Ruiqi Guo and Sanjiv Kumar and Balint Miklos and Ray Kurzweil},
year={2017},
eprint={1705.00652},
archivePrefix={arXiv},
primaryClass={cs.CL}
}
```
<!--
## Glossary
*Clearly define terms in order to be accessible across audiences.*
-->
<!--
## Model Card Authors
*Lists the people who create the model card, providing recognition and accountability for the detailed work that goes into its construction.*
-->
<!--
## Model Card Contact
*Provides a way for people who have updates to the Model Card, suggestions, or questions, to contact the Model Card authors.*
--> | [
"TEXT_CLASSIFICATION",
"SEMANTIC_SIMILARITY"
] | Non_BioNLP |
Sharka/CIVQA_DVQA_Impira_QA | Sharka | document-question-answering | [
"transformers",
"pytorch",
"layoutlm",
"document-question-answering",
"document question answering",
"cs",
"license:mit",
"endpoints_compatible",
"region:us"
] | 1,706,457,581,000 | 2024-01-28T16:01:36 | 24 | 1 | ---
language:
- cs
license: mit
tags:
- document question answering
---
# LayoutLMv2 Model Fine-tuned with CIVQA (Tesseract) dataset
This is a fine-tuned version of the [Impira Document QA model](https://huggingface.co/impira/layoutlm-document-qa), which was trained on Czech Invoice Visual Question Answering (CIVQA) dataset containing invoices in the Czech language as well as on the Data Visualizations via Question Answering ([DVQA] (https://paperswithcode.com/dataset/dvqa)) dataset.
This model enables Document Visual Question Answering on Czech invoices with the use of the existing DVQA dataset.
Regarding the Czech invoices, we focused on 10 different entities, which are crucial for processing the invoices.
- Variable symbol
- Specific symbol
- Constant symbol
- Bank code
- Account number
- Total amount
- Invoice date
- Name of supplier
- DIC
- QR code
You can find more information about this model in this [paper](https://nlp.fi.muni.cz/raslan/raslan23.pdf#page=31). | [
"QUESTION_ANSWERING"
] | Non_BioNLP |
desarrolloasesoreslocales/SetFitPrueba | desarrolloasesoreslocales | text-classification | [
"sentence-transformers",
"pytorch",
"bert",
"setfit",
"text-classification",
"arxiv:2209.11055",
"license:apache-2.0",
"region:us"
] | 1,696,328,667,000 | 2023-10-09T10:37:54 | 7 | 0 | ---
license: apache-2.0
pipeline_tag: text-classification
tags:
- setfit
- sentence-transformers
- text-classification
---
# desarrolloasesoreslocales/SetFitPrueba
This is a [SetFit model](https://github.com/huggingface/setfit) that can be used for text classification. The model has been trained using an efficient few-shot learning technique that involves:
1. Fine-tuning a [Sentence Transformer](https://www.sbert.net) with contrastive learning.
2. Training a classification head with features from the fine-tuned Sentence Transformer.
## Usage
To use this model for inference, first install the SetFit library:
```bash
python -m pip install setfit
```
You can then run inference as follows:
```python
from setfit import SetFitModel
# Download from Hub and run inference
model = SetFitModel.from_pretrained("desarrolloasesoreslocales/SetFitPrueba")
# Run inference
preds = model(["i loved the spiderman movie!", "pineapple on pizza is the worst 🤮"])
```
## BibTeX entry and citation info
```bibtex
@article{https://doi.org/10.48550/arxiv.2209.11055,
doi = {10.48550/ARXIV.2209.11055},
url = {https://arxiv.org/abs/2209.11055},
author = {Tunstall, Lewis and Reimers, Nils and Jo, Unso Eun Seo and Bates, Luke and Korat, Daniel and Wasserblat, Moshe and Pereg, Oren},
keywords = {Computation and Language (cs.CL), FOS: Computer and information sciences, FOS: Computer and information sciences},
title = {Efficient Few-Shot Learning Without Prompts},
publisher = {arXiv},
year = {2022},
copyright = {Creative Commons Attribution 4.0 International}
}
```
| [
"TEXT_CLASSIFICATION"
] | Non_BioNLP |
BUT-FIT/CSTinyLLama-1.2B-RAGsum | BUT-FIT | text-generation | [
"transformers",
"safetensors",
"gguf",
"llama",
"text-generation",
"cs",
"base_model:BUT-FIT/CSTinyLlama-1.2B",
"base_model:quantized:BUT-FIT/CSTinyLlama-1.2B",
"autotrain_compatible",
"text-generation-inference",
"endpoints_compatible",
"region:us"
] | 1,733,337,005,000 | 2025-01-07T07:33:18 | 143 | 0 | ---
base_model:
- BUT-FIT/CSTinyLlama-1.2B
language:
- cs
library_name: transformers
---
Training Dataset: Semant Search Summarization Dataset
Training configuration
```yaml
gradient_checkpointing: true
learning_rate: 1e-5
max_grad_norm: 5.0
num_epochs: 6 # 4110 steps in total
optimizer: adamw_torch
lr_scheduler: cosine
batch_size: 128
overrides_of_model_config:
max_position_embeddings: 16384
rope_theta: 100000
rope_scaling:
type: linear # linear | dynamic
factor: 2.0
liger_rope: true
liger_rms_norm: true
liger_glu_activation: true
liger_layer_norm: true
liger_fused_linear_cross_entropy: true
format: |
Shrň následující výsledky pro dotaz "{instruction}".
|Výsledky|: {input}
|Shrnutí|:
```
Example:
```python
input_args = {
"query": "výlet na Praděd ze Sobotína a okolí",
"input": "Výsledek [1]: Až do Domašova (8 km) mož- no dvakrát denně užíti pošty (za 11/4 hod.); vozem z Frýval- dova až do Waldenburgu (host. Schubertův 12 km, 3 hod. pě- šky, povoz. 11/2 hod.). Odtud dále dle bělozelených značek silně stoupající cesta 23/4 hod. na Praděd podle Vys. Vody k vodopádu Huhfallu (vysoký, ale mívá málo vody); kdo chce jíti pohodlněji, jde dále ještě sjízdnou cestou až do Jablonné (Gabel) a odtud dle značek k Švýcárně. Sestup po případě do Karlsbrunnu (v. níže č. 2.) neb Sobotína (str. 190.), přes Mysl Františkovu do Koutů (viz str. 191.), nebo přes Šerák do Ram- zové (61/2 hod., str. 181.). 1. Dvoudenní pochod Jesení- kem můžeme si z Frývaldova zaříditi tak, že jdeme z Frý- valdova a Rejvizu (také přes Houk a Zlatou Kupu nebo přes oba vrchole viz č. 5., 6 níže) (modrožluté značky k Plesu a přes poutní místo sv Marka a Marii Pom. na Edel- štejn a do Cukmantlu nocl 2. dne z Cukmantlu na Biskup- skou Výšinu a Slezským Švý- carskem (Wid Grund) do Hlu- cholazů. — 2. Na Praděd a Karl. Lázně (Karlsbrunn) vyžaduje výlet přes Waldenburg (3 hod.) se vzestupem na Praděd (+234 hod.). Výstup dosti příkrý. S Praděda do Karl. Lázní 11/2 hod. (Špičky červ. trojúh. smě řují na Praděd. Špičky jino- barev. trojúh. vyznačují se- stup). Až do Waldenburgu (14 km) dobře sjízdná silnice. Jde- me mal. dolinou Bělé Buchels- dorfem a Adelsdorfem 34 hod. do bystřinou oživeného dolu až ke kostelu ve Sv. Tomáši (Thomasdorf), kde počíná sil- nice nová serpentinovitě ve- doucí do Winkelsdorfu (v pr.) Jdeme starou silnicí přímo dále dle žlutých značek (zkra- cujeme si serpentiny) vzhůru\nVýsledek [2]: přes Franzenshöhe, s níž dále no 2 hod.). Odtud lesem značen. vede cesta znač. na Schafhäuser, cestou (průvodčí příjemný) k hra- neundorfskou boudu (Neundorfer ničnému kameni č. 83 a na hřeben B.), na Malém Sněžníku a k Svý- Mal. Sněžníku po hřebenu po- carství na hřebenu (3 hod.). Dále vlovně vzhůru a přímo v pr. k po hřebenu ještě 3/4 hod. b) Přes rozhl. na Vel. Sněžniku, odtud pak dolů k Švýcarství, — f) Přes Wölfelsgrund. Stejným směrem jako při a) do Lauterbachu, přímo Klappersteine. Velmi pěkná partie však dále přes Neundorf a Urnice přes Schöntal a Neissbach 2 hod. k Wölfelsfallu, odtud dále viz na nejjižnější bod hřebene, kde níže při Langenau. (Celkem 5 hod.) s balvanitého pole nabízí se krás- — c) Jako při a, b) do Lauterbachu, ný rozhled (u hranič. kam. č. 115) avšak vsi podle potoka vzhůru na celé hrabství kladské, do Čech až na hřeben při úpatí Malého i Moravy; přes hrabství viděti až Sněžníka (hraniční kámen II. 83), na Krkonoše. Také od hranič. ka- zde v pr. přes Thanndorfskou mene č. 118 je pěkný rozhl. (na cestu okolo Mal. Sněžníka (1348 kuželovitý kopec s hradem Scham- m), ještě 11/4 hod. k Švýcar- bachem; bílé šipky ukazují k pra- ství (v pr. Velký Sněžník s rozhl. menům Nisy). S Klappersteinu a vzhůru na vrchol. — e) Přes (1138 m) po hřebeni podle hranice Thanndorf. Z Mittelwalde nejpo- zemské dojdeme k vrchoii Hled- hodlnější výstup 5 hod. s pozvol- sebe (Flammenpappel 1185m 4 hod.) ným stoupáním Buď Gläsendor- a k hraničnému kameni č. 83, od- fem nebo Schöntalem do Hor. kudž pokračujeme (jako při d). Thanndorfu k mysl. (až sem sjízd- Západním nízkým bokem Bystřického hřebene vede nás trať podle Nisy (v pr.) k severu míjíc Herzogswald a Schön- feld se zámkem (v 1.) do zastávky Ebersdorfu (nádr. rs.), velmi vhodného východiště na Kladský Sněžník. Do Wöl- brechta až k vozovce v pr. odbo- felsdorfu 8 km (povoz až k Wäl- čující, jež okolo Heubergu vede felspallu; většinou beze stínu ra- na silnici od Seitenbergu prichá- dno jeti za 4 mk; omnibus 1.20 zející, touto dále 21/2 hod. k Švý- mk). Ves Wölfelsgrund (520—600 carství. m) je oblíbené Ls. Host. nejlepší d) Přes Pannu Marii Sněžnou Z. Forelle, Z. guten Laune (zvlášť Vých. od host. z. gut. Laune vede oblíbený, pok. 75 pf až 3 mk, T. nová označ. cesta 1 hod. k pout. d'h 1.50, také v zimě otevř. jízdy kostelíku P. Marie Sněžné na Špi- rohačkami: několik vill s pokoji). čatém vrchu (Spitziger Berg 816 Tyroler Hof (dobrý). Byty týdně 6—30 mk. Bystřina Wölfel tvoří rs. z. schön. Aussicht, pok. 1 až 2 mk, Ls., slabší host, z. Maria tu malebný vodopád Wölfelsfall Schnee. Rozhledna poskytuje roz- 30 m vys. (přístup zahr. host. Z. hled na hrabství a hřeben Klad- g. Laune; vstup na můstek, v pr. ského Sněžníku a náleží k nej- 42 stupňů vzhůru a 82 dolů k vyhl. krásnějším v Kladsku. Odtud proti vodopádu). Z Wölfelsgrundu k vých. podle mysl. (průvodčí pří- možno voliti různý směr: a) Stará jemný) přes silnici prince Al- cesta, dosti příkrá údolím Wölfly brechta a přímo dále mezi Heu- 2—21/2 hod. k Švýcarství na Sněž- bergem (v pr.) a Černou Horou níku, — b) Mírněji stoupající sil- (v 1.), pak k S., po 1/4 hod. opět nicí; u mostu v horní vsi na pr. k vých., za 1/4 hod. jsme na pě- břeh Wölfly a přímo k Malému šině »Schlegelské« zvané (ukaz.) Sněžníku dále po hřebenu až k Švý- touto na vrchol (3 hod.). carství 2—21/2 hod. e) Přes Puhu. Krásnou silnicí c) Nejpohodlněji od konce vsi za mysl. novou silnicí Prince Al- prince Albrechta až k host. Puhu,\nVýsledek [3]: Odbočka z moravsko-slezské horské dráhy vede dále údolím Desné pod Brousnou do Šumperka, zněmč. města, jež je hlavním sídlem mor. plátenictví, dále podle zast. Vykýřovic na stanici Petrovice-Losín, kdež se tratě dělí. Krátká místní dráha vede do Sobotína, delší tratí přes Rejpotín, Losín lázně, Losín Velký, Wiesenberk a Rejpotice do Koutů (Reutenhau). Na Praděd vystupujeme tu čtyřmi směry: stoupáme hodinu lesem podle a) Ze Sobotína na Praděd, Merty (pozor! po 1/4 hod. stou- Sobotín, (Zöpten, host. u nádr., pání vděčno odbočiti k místu, kde 10 min. od stanice host. u hutní- Merta z úzké sluje vyvirá; místo ka) rozkošně položená ves s hu- se skvostným rozhledem). Opusti- těmi bar. Kleina. Na blízku (11/2 vše Mertu. vinutou cestou vzhůru hod.) Rauchbeerstein (790 m) se jsme za 4 hod. na hřebeni. u Mys- skvostnou vyhl. Žlutě a modře livny Františkovy (1183 m) ještě znač. cesta vede od host. u hut- 2 hod. od Švýcarství na Pradědu). nika. — Žlutě a zeleně značkovaná Je tu 7 loží, úředníci panští mají cesta (zelená ukazuje) vede nás přednost). Krásná poloha a vy- přímo od nádraží (ves zůstane hlídka, zejména od bodu 5 min. v pr.) do Teplice (11/4 h. Wermsdorf vzdálen. na Praděd, Vys. Holi, host. Kubíčkuv) a dále údolím stále Medvědí Kameny, Petrštýn, do užším do malébné kotliny, kde obrátíme se ostře v pr. a příkřeokolních úvalů na Teplice, holé\nVýsledek [4]: Ze Sobotína do Jeseníku. a) Ze Sobotína na Praděd. Mertu, vinutou cestou vzhůru Sobotín (Zöptau. host. u nádr., jsme za 4 hod. na hřeben 10 min. od stanice host. u hut- u Myslivny Františkovy (1183 níka), rozkošně položená ves m, ještě 2 hod. od Švýcarství s hutěmi. Na blízku (11/2 hod.) na Pradědu). Je tu 7 loží Rauchbeerstein (790 m) se úředníci panští mají přednost. skvostnou vyhl. žlutě a modře Krásná poloha a vyhlídka, ze znač. cesta vede od hostince jména od bodu 5 min. vzdál. u hutníka. — Žlutě a zeleně na Praděd, Vys. Holi, Medvědí značkovaná cesta (zelená uka- Kameny, Petrštýn, do okolních zatel) vede nás přímo od nádra- úvalů, na Teplice, holé hřebe- ží (ves zůstane v pr.) do ny Májové Hory (1381 m). Ze- Teplice (Wermsdorf, 11/4 hod., leně a červ. znač. cesta vede host. Kubíčkův) a dále údolím dále na hlavní hřeben Májové stále užším do malebné kotli- Hory a po hřebenu červeno- ny, kde obrátíme se ostře v pr. žluté značky přes Vys. Holi a příkře stoupáme hodinu le- !1464 m), Petrštýn (1446 m) na sem podle Merty (pozor! po 1/4 vrchol Praděda (1490 m) a dále hod. stoupání vděčno odbočiti ku Švýcárně (1364 m) pod Ma- k místu, kde Merta z úzké lým Pradědem. (6 hod. Další sluje vyvírá; místo se skvost- viz str. 182.) Jiná cesta vede ným rozhledem). Opustivše od Myslivny (zeleně-bílé znač.)\nVýsledek [5]: nici; z Velké Moravy možno hřeben Sněžníku přes Malý Sněžník až na skalí Klapper- nad kostelem 1/2 km odbočiti v pr. a tak si kus nadejíti. steinu a prohýbá se pod ním údolí Moravy a nejzáze roze- Kdo chce do Králík, jde jen silnicí až do Červ. Potoka. znáváme Králíky s pozadím Suchého Vrchu. Srovn. květe- Zde přes trať a silnicí beze nu na str. 11. a popis na str. stínu do Králík. 7. Sestup: Nechceme-li voliti b) Do Starého Města mor. vede bíločerv. označená cesta k sestupu některý zpětný směr do Wölfelsgrundu (21/2 hodiny, 3 hod. od Švýcarství přes značky zelenobílé, přes Heu- Stříbrnice nebo přes N. Rum- burk (od rozhl. k Švývarství berg černobílé) nebo některý směr na Mittelwalde (str. 165.) dle bíločerných značek). Může se odtud (viz níže trať III b) přes Thanndorf (červenobílé značky) nebo Klapperstein a prodloužiti pochodem na Pra- Neissbach (černobílé zn., 41/2 děd. Taktéž sestup c) do Ram- hod.), volte směr do Čech ne- zové na moravsko-slezskou dráhu bíle a žlutě označ. ce- bo Moravy: a) Údolím Moravy stou přes Fichtlich, odtud dle do Červ. Potoka k stanici (3 hod.), po případě dle zelenobí- značek žlutomodrých, 81/2 hod., lých značek přes H. Lipku do možno prodloužiti pochodem Červ. Potoka nebo až do Krá- na Šerák a Praděd. lík (4 hod.). Morava sbírá se Jiné značené cesty vedou od ze dvou pramenů na již. sva- rozhl. do Seitenberka (odtud hu Sněžníka. Serpentina kolem na Javorník nebo Kladsko, vrchu (2 hod.) vedoucí (6 min. znač. bíločerné, 31/4 hod.), také pod vrchem odbočuje cesta k možno do Seitenberka k nádr Tvarohovým Dírám, 3 jeskyň- přes Johannesberk (černobílé kám s hnízdy vápence v rule) znač.) nebo přes Husí Chřtán a Klessengrund (3 hod.) nebo jde podle pramenů do údolí: po 2 hod. konec lesa. Mezi do Vilémova dle bílomodrých domky Horní, Velké a Dolní značek přes Sněžnou Jámu a Moravy dospějeme až k sta- Kamnici (21/4 hod.). Brzy za Ebersdorfem objeví se nám půvabná dolina pod Bystřickým Hřebenem (v 1.), v níž leží nedaleko stanice (10 min.) Lázně Langenau, jež v 1. v údolí malebně jsou rozloženy. Nádr. rs. Ves, 10 min. vzdál., má železité i slatinné Lz. s vodoléčeb. ústavem a pěknými sady. Bývá tu přes 1200 hostů. Hostinec: Láz. dům. Jägerhof, Annahof, Rupprecht. Rs. ve ville Urban a Merkur se zahradou. Láz. taxa. Pitné želez. kyselky. V nejbližším okolí pěkné vycházky na Grafenfichte (20 min. s pěkným rozhl.), Scharfe Ecke (15 min.) v 1. od sil- nice do Bystřice, Krähenberg (pěkná vyhl.), Belvedere (pěk- ná vyhl.), Waldhäuschen (1/4 hod.). Také některé výlety odtud přes Bystřický Hřeben k Čes. Hřebenu jsou půvabné, na př. přes Kavčí Horu (Dohlen-\nVýsledek [6]: blíže tratě Venušin Vrch a před samým Bruntálem v levé Köhlenberg. Bruntál stan., něm. město, hl. sídlo něm. ryt. řádu, jen v okolí mnoho statků má, vedle znamenitého zámku s krásný mi zahradami. Vnucená správa republ. Úřady státní v něm rukou. Čechů ne celá 2 . Pomník Josefa II. odstraněn teprv po četných demonstracích. Město bylo teprve za Přemysla 1 Němci osazeno. Něm. pojm. Freudental je přezvisko z nov. do by. Průmysl plátenický a bavlnický. Real. školy. Karlova, odtud dle Roz. 1. Venušin vrch — Roudno. Na tyto mladší vyvřeliny vede hod. k Alfrédově chýši, malému silnice k J. V. do Karlovce, ale útulnému host. (levný nocl a k Jelení Studánce na hřebe z které odboč. v pr. cestou na novou cestu od Skřítku k Ovči Nové Pláně dle černo-červ. nu. — b) Drahou jen do An znač. 11/2 hod. na Venušin Vrch dělské Hory, odtud dle žluto (656 m) přes Thierberg (692 m) černých zn. přes Dürseifen d do N. Plání; za těmito se přiblí- Karlovy Studánky (Karlovýc) žíme ke Karlovci (v pr.), jdeme lázní); lz. s uhličitou vodou však přímo do Roudné a vy- železitou v lesnaté kraj.; léčba stoupíme na vrchol Roudná žinčicí. V nov. době zařízen (780 m). Návrat přes Karlovec. zdokonaleno. 2. Köhlerberg (674 m) jihozáp. Sem možno také oklikou: dra od města je znamenán poutni- ckým kostelíkem. hou ke Krnovu, na Nové Her vinovy a zde odbočkou do 3. Annenská Hora. Odb. trati, Vrbna (pěšky dle černomodr vedoucí do Morávky, Karlova, až do stan. And. Hora, odkudž znač. 2 km), odkudž do Karl St. jede pošta. Z K. St. dle pěkná alej vede na vrch s býv. poutnic. kostelíkem a rs. Se- černočerv. zn. (cestou dle ukaz stup dle modrozel. znač. k Fi- v 1. k vodopádu Opavice 314 hod. na Praděd. Kdo nechce losofské Skále. přímo na Pr. může odchýlit 4. Praděd. a) Drahou až do Morávky — Karlova. V Mo- se z cesty černočerv. znač. dle rávce výr. dřev. žebříků růz. znač. černožlutých v 1. k Ovčí- pat. systémů. Z Morávky do nu (21/2 hod.). Opouštíme Bruntál hl. tratí podle Spillendorfu (vl.) do stan- Hervinovy-Dachov (nespr. Heřminovy nebo Heřmanice — Neu Erbersdorf nebo Friedersdorf), vsi tkalcovské s výr. zboží stávkového a pleteného, kde odbočuje místní dráha přes Nové Hervinovy do Vrbna, něm. města nad Opa- a pěkným got. kostelem. vicí s odb. školou pro zpraco- Z Vr. na Praděd možno jíti vání dřeva, výr. dřev. zboží, a) na (modroč. zn.) Karl. Stu- nábytku z kořenů, průmysl. dánku (viz výše č. 4 b.) nebo tkalc. a stávkařským, staré b) romant. údolím stř. Opavice doly na olovo a měď. Se svole- přes Buchbergstál a Jablonné ním les. úř. ve Vr. možno vy- (na haldách nerosty), (tur. host.) nebo c) oklikou celého Jesení- jíti na Zám. Vrch ke zříc. St. Fürstenwaldu. S Vr. souvi- ku přes Rejviz a Frýval- sí Einsiedel s vodoléč. ústavem dov; modročerv. zn. vedou\nVýsledek [7]: Z Petrovic odbočujeme od tratě sobotínské k S. přes Ra otín do Lázní Losína (za nimiž násl. zast. Velký Losín), jež známy jsou výbornou teplicí sírnou, podobnou oné v Pí- ťanech a Teplicích Trenčinských. Pěkná hornatá krajina poskytuje vlídný pobyt. Dobře zařiz. láz. dům, vodoléčebný ústav. Pití ovčí žinčice. Také odtud lze zaměřiti na Praděd: a) Z Losína buď k nádraží Dreistein (vyhl., 928 m) a od- tud dále dle těchže nebo nebo Sobotíně anebo přímo přes modročerv. zn. do Rejpotic (3 Maršovice do Teplic a dále dle hod.) nebo kratčeji přes Pri- nebo b) jako ze Sobotína, miswald (778) za 2 hod. Dále až 8 hod. viz níže. b) Do Rejpotic a Koutů: zlutě a červ. značk. cestou na Minuvše Viesenberk a Rejpotice (viz výše) stihneme do konečné stanice Kouty nad Děsnou (Winkelsdorf), kteréž jsou velmi vděč- nou výchozí stanicí na hlavní pásmo Pradědu. Odtud vy- jížděl před válkou autobus přímo přes sedlo směrem na Frý- valdov (r. 1922 nebyl ještě znovuzaveden). Pod Červ. Hůrou křižuje silnice tato, v četných zákrutech se vinoucí, hlavní hřeben; v pr. po něm je skoro stejně daleko na Praděd, jako v 1. na Šerák. Pěší turista sleduje z Koutů tyto směry: graben) a velice malebnou, ale 1. Praděd. Od stanice (Dům obtížnou partií téhož v 1. na uristů) zeleně a bíle znač. ce- Praděd (31/2 hod.) nebo přímo sta vede pod mysl. údolím dále podle Děsné k myslivně mladé ještě Děsné silnicí Frý- Františkově (1/2 hod.; v 1. od valdovskou 21/2 km, načež ji rozc. dle červených a bílých opouštíme v pr., jdouce 1/2 km značek přímo k Švýcárně (134 k rozc., v pr. podle Děsné dále hod.). do Kamenného Úvalu (Stein\nVýsledek [8]: Ze Šumperka [M.-Schönberg] 381 m, 12.500 ob. 1. na Heukoppe bílo-žluté [žluté] 78 m. [11/4I; 2. na Karlovu Výšinu bílo-žluté [žluté] 155 m. [21/]; 3. na zříceniny Nov. Zámku přes Bratrušov, Velvice bílo-modré [modré] 160 m. [21/2]; 4. do Rejchartic bílo-žluté [žl.] 135 m. [21/4l; 5. na Bürgerstein bílo-červené [červené]; 6. na Fichtberk bílo-zelené [zelené] 115 m. [2]; 7. na Kokerstein bílo-modré [modré] 65 m. [1] u sanatoria počínají; 8. k Hraniční studni a přes Pfitzenstein na Osykovské Výšiny a Fichtberk 743 bílo-zelené Iz.J (21/2). Š. je moderně stavěn, zvl. v novější části. Má přímé, čisté a výstavné ulice i náměstí. Prů- myslově je velmi čilý. Pěkná radnice. — Nejlepší pohled na Š. je od host. Bergu. * * Ze Švýcarství [Schweizerei] na M. Pradědu 1311 m. 1. na Praděd modro-červ. [červ.] 49 m. I3/4I; 2. do Kamenného Úvalu, divoce romantického údolí, přes Praděd modro-červené [červené], s Pra- dědu červeno-bílé [bílé] 190 m. [3]; 3. do Kamenného Úvalu mimo Praděd modro- červené [červené], po 25 m. uchylují pr. modro- bílé, s nich po 22 m. na červeno-bílé [bílé] 182 m. [3]; 4. do Koutů červeno-modré, po 30 m. 1. na červeno-bílé [bílé] 165 m. [23/4]; 5. do Valdenburku mimo Vys. Vodopád čer- veno-modré [modré], po 5 m. pr. na zeleno- bílé [bílé] 155 m. [11/4]; 91\nVýsledek [9]: 1. Kladský Sněžník: Vzhůru[seifen (Neutznerův host.) k Mi- líři. — Od Milíře podle pramene cestou bíle znač. sestup červ. Adelinina vzhůru na hřebenovou označen. a) Pohodlněji, ač dále (4 cestu a touto na Sněžník. (Viz h.) z města k S. silnicí do Květ- str. 131). nova; u novorumburského 2. Praděd. Možno voliti trojí mostu v 1. do Nov. Rumburku, směr, pokaždé na některou sta- tímto vzhůru příkře k lesu a po- nici moravsko-slezské horské drá- dle kapličky s kříž. cestou k t. hy a odtud dále dle tratě IV.: a) zv. Milíři na úpatí Sněžníku (2 přes Koldštýn k vých. do Hei- hod.). — b) Z města k bělidlu, od- merlstálu, kde se u kříže cesty tud v 1. polní cestou přes písečné dělí; jdeme v pr. po úpatí Eber- jámy 3/4 hod. k posl. domkům Nov. šáru s krásnými pohledy na Klad- Rumburku a v 1. skrze Stuben-\nVýsledek [10]: IVb. Rudoltice—Lanškroun . . . . Rudoltice. Lanškroun 177. Lanškroun—Zámecký Vrch. Švédské Šance. Maria Cell. Anenské Studánky 178. Lanškroun—Suchý Vrch. Klocberg. Údolí Umučených. Výprachtická Lípa 179. Lanškroun—Buková Hora 180. Trať IV. (pokrač.). Rudoltice— Zábřeh . . . . . 180 Rudoltice. Krasíkov. Hoštýn. Zábřeh 180. Trať IVc. Zábřeh—Bludov. Hanušovice. Mi- kulovice. Hlucholazy. Krnov . . . . . . Bludov. Komňátka. Čes. Bohdíkov. Hanušovice. Kold- štýn—Vozka—Šerák. Ostružná. Ramzová 180. Ramzová— Šerák—Praděd 182. Ramzová—Klad. Sněžník 184. Dol. Lipová—Fichtlich. Kladský Sněžník. Přes Kopřivnou do Gräfenberku 184. Gotteshausberk. Hukovice—Vid- nava. Bernartice. Javorník 185. Frývaldov—Gräfen- berg 185. Domašov. Waldenburg. Praděd. Karlsbrunn 186. Dvoudenní pochod Jeseníkem. Z Frývaldova na Praděd a Karl. Lázně. Šerák. Praděd. Karlova Stu- dánka—Karl. Lázně. Křížový Vrch. Zlatá Kupa 186. Harik a Kaltenšten 187. — Z Frývaldova drahou na Sandhübel—Šupikovice, Mikulovice ve Slezsku. Cuk- mantl—Edelštejn. Výlety z Cukmantlu 187. Mikulovice— Krnov . . . . . . Hlucholazy. Před. Kupa. Střední Kupa. Čertova Kaza- telna. Třemošná—Osoblaha. Třemošná—Krnov 188. Kr- nov—Burgberk—Úvalno. Melzerberk 189.",
"chatgpt_summary": "Výlet na Praděd lze zrealizovat z několika tras, přičemž nejoblíbenější je cesta ze Sobotína přes Myslivny. Odtud se stoupá zeleně značkovanou cestou kolem Merty, což trvá přibližně 4 hodiny k hřebeni (1183 m) a dále na Praděd (1490 m), což zabere další 2 hodiny [4]. Pokud se chcete vydat z Lázní Losína, okružní trasa zahrnuje nádraží Dreistein a pokračuje přes Rejpotice a Kouty; celkově cesta zabere z Losína do Koutů asi 8 hodin [7]. Další nádherné výhledy se nabízejí ze Švýcárny, přičemž přístup k této vyhlídce je možný také z Frývaldova [8]. Existují také alternativní trasy směřující na Sněžník s různými obtížnostmi, které lze zvládnout během 2 až 5 hodin chůze [5]. Pokud toužíte po atraktivních výhledech a přírodě, Jeseníky a okolí s různými turistickými trasami nabízejí ideální prostředí pro výlety do přírody."
}
import torch
import transformers
model_name = "BUT-FIT/CSTinyLLama-1.2B-RAGsum"
tokenizer = transformers.AutoTokenizer.from_pretrained(model_name)
config = transformers.AutoConfig.from_pretrained(model_name, trust_remote_code=True)
formatted_input = f"""Shrň následující výsledky pro dotaz "{input_args['query']}".
|Výsledky|: {input_args['input']}
|Shrnutí|:
"""
device = "cuda" if torch.cuda.is_available() else "cpu"
model = transformers.AutoModelForCausalLM.from_pretrained(
model_name,
config=config,
trust_remote_code=True
).cuda()
with torch.autocast('cuda', dtype=torch.bfloat16):
inputs = tokenizer([formatted_input], return_tensors="pt").to(device)
del inputs['token_type_ids']
input_ids = inputs['input_ids']
outputs = model.generate(input_ids=input_ids,
max_new_tokens=16000,
top_p=0.95,
repetition_penalty=1.0,
do_sample=True,
use_cache=True)
input_length = inputs['input_ids'].shape[1]
generated_text = tokenizer.decode(outputs[0][input_length:], skip_special_tokens=True)
print("RAG Summary:", generated_text)
```
Example of generated summary
```
Generated Output: Výlet na Praděd je populární a nabízí krásné výlety na vrchol nejvyšší moravské hory.
Je možné se pohybovat pěšky nebo pomocí značených tras ze Šumperska a dalších částí Jesenicka [1], na
které poskytují širokou škálu možností pro túry a horské procházky [2]. Tato trasa navíc vede po trase
šumperského vlaku, což je výhoda, protože není třeba platit za dopravu [3]. V Hrubém Jeseníku se navíc
nachází řada ubytovacích zařízení a dalších možností ubytování, kde je možné získat zázemí pro další
dobrodružství [4].
``` | [
"SUMMARIZATION"
] | Non_BioNLP |
florianhoenicke/pet-shop32-per-device-4_9062874564 | florianhoenicke | feature-extraction | [
"transformers",
"safetensors",
"bert",
"feature-extraction",
"custom_code",
"text-embeddings-inference",
"endpoints_compatible",
"region:us"
] | 1,712,670,148,000 | 2024-04-09T14:46:37 | 5 | 0 | ---
{}
---
# pet-shop32-per-device-4_9062874564
## Model Description
pet-shop32-per-device-4_9062874564 is a fine-tuned version of jina-embeddings-v2-base-en designed for a specific domain.
## Use Case
This model is designed to support various applications in natural language processing and understanding.
## Associated Dataset
This the dataset for this model can be found [**here**](https://huggingface.co/datasets/florianhoenicke/pet-shop32-per-device-4_9062874564).
## How to Use
This model can be easily integrated into your NLP pipeline for tasks such as text classification, sentiment analysis, entity recognition, and more. Here's a simple example to get you started:
```python
from transformers import AutoModel, AutoTokenizer
model_name = "pet-shop32-per-device-4_9062874564"
tokenizer = AutoTokenizer.from_pretrained(model_name)
model = AutoModel.from_pretrained(model_name)
tokens = tokenizer("Your text here", return_tensors="pt")
embedding = model(**tokens)
```
| [
"TEXT_CLASSIFICATION"
] | Non_BioNLP |
Qdrant/bge-base-en-v1.5-onnx-Q | Qdrant | sentence-similarity | [
"transformers",
"onnx",
"bert",
"feature-extraction",
"sentence-similarity",
"base_model:BAAI/bge-base-en-v1.5",
"base_model:quantized:BAAI/bge-base-en-v1.5",
"license:apache-2.0",
"text-embeddings-inference",
"endpoints_compatible",
"region:us"
] | 1,705,391,984,000 | 2024-09-12T18:12:31 | 133,862 | 1 | ---
base_model:
- BAAI/bge-base-en-v1.5
license: apache-2.0
pipeline_tag: sentence-similarity
---
Quantized ONNX port of [BAAI/bge-base-en-v1.5](https://huggingface.co/BAAI/bge-base-en-v1.5) for text classification and similarity searches.
### Usage
Here's an example of performing inference using the model with [FastEmbed](https://github.com/qdrant/fastembed).
```py
from fastembed import TextEmbedding
documents = [
"You should stay, study and sprint.",
"History can only prepare us to be surprised yet again.",
]
model = TextEmbedding(model_name="BAAI/bge-base-en-v1.5")
embeddings = list(model.embed(documents))
# [
# array([
# 0.00611658, 0.00068912, -0.0203846, ..., -0.01751488, -0.01174267,
# 0.01463472
# ],
# dtype=float32),
# array([
# 0.00173448, -0.00329958, 0.01557874, ..., -0.01473586, 0.0281806,
# -0.00448205
# ],
# dtype=float32)
# ]
``` | [
"TEXT_CLASSIFICATION"
] | Non_BioNLP |
cowWhySo/Phi-3-mini-4k-instruct-Friendly | cowWhySo | text-generation | [
"transformers",
"safetensors",
"phi3",
"text-generation",
"conversational",
"custom_code",
"dataset:mlabonne/orpo-dpo-mix-40k",
"license:mit",
"autotrain_compatible",
"text-generation-inference",
"endpoints_compatible",
"region:us"
] | 1,717,551,973,000 | 2024-06-07T01:39:25 | 14 | 0 | ---
datasets:
- mlabonne/orpo-dpo-mix-40k
license: mit
---
This is a uncenscored version of Phi-3.
Abliterated using the following the guide here: https://huggingface.co/blog/mlabonne/abliteration
Then it was fine tuned on orpo-dpo-mix-40k
[<img src="https://raw.githubusercontent.com/OpenAccess-AI-Collective/axolotl/main/image/axolotl-badge-web.png" alt="Built with Axolotl" width="200" height="32"/>](https://github.com/OpenAccess-AI-Collective/axolotl)
<details><summary>See axolotl config</summary>
axolotl version: `0.4.0`
```yaml
base_model: cowWhySo/Phi-3-mini-4k-instruct-Friendly
trust_remote_code: true
model_type: AutoModelForCausalLM
tokenizer_type: AutoTokenizer
chat_template: phi_3
load_in_8bit: false
load_in_4bit: true
strict: false
save_safetensors: true
rl: dpo
datasets:
- path: mlabonne/orpo-dpo-mix-40k
split: train
type: chatml.intel
dataset_prepared_path:
val_set_size: 0.0
output_dir: ./out
sequence_len: 4096
sample_packing: false
pad_to_sequence_len: false
adapter: qlora
lora_model_dir:
lora_r: 64
lora_alpha: 32
lora_dropout: 0.1
lora_target_linear: true
lora_fan_in_fan_out:
wandb_project: axolotl
wandb_entity:
wandb_watch:
wandb_name: phi3-mini-4k-instruct-Friendly
wandb_log_model:
gradient_accumulation_steps: 8
micro_batch_size: 4
num_epochs: 1
optimizer: paged_adamw_8bit
lr_scheduler: linear
learning_rate: 5e-6
train_on_inputs: false
group_by_length: false
bf16: auto
gradient_checkpointing: true
gradient_checkpointing_kwargs:
use_reentrant: True
early_stopping_patience:
resume_from_checkpoint:
local_rank:
logging_steps: 1
xformers_attention:
flash_attention: true
warmup_steps: 150
evals_per_epoch: 0
eval_table_size:
eval_table_max_new_tokens: 128
saves_per_epoch: 1
debug:
deepspeed: deepspeed_configs/zero3.json
weight_decay: 0.01
max_grad_norm: 1.0
resize_token_embeddings_to_32x: true
```
</details><br>
## Quants
GGUF: https://huggingface.co/cowWhySo/Phi-3-mini-4k-instruct-Friendly-gguf
## Benchmarks
| Model |AGIEval|GPT4All|TruthfulQA|Bigbench|Average|
|--------------------------------------------------------------------------------------------------|------:|------:|---------:|-------:|------:|
|[Phi-3-mini-4k-instruct-Friendly](https://huggingface.co/cowWhySo/Phi-3-mini-4k-instruct-Friendly)| 41| 67.56| 46.36| 39.3| 48.56|
### AGIEval
| Task |Version| Metric |Value| |Stderr|
|------------------------------|------:|--------|----:|---|-----:|
|agieval_aqua_rat | 0|acc |22.05|± | 2.61|
| | |acc_norm|22.05|± | 2.61|
|agieval_logiqa_en | 0|acc |41.01|± | 1.93|
| | |acc_norm|41.32|± | 1.93|
|agieval_lsat_ar | 0|acc |22.17|± | 2.75|
| | |acc_norm|22.17|± | 2.75|
|agieval_lsat_lr | 0|acc |45.69|± | 2.21|
| | |acc_norm|45.88|± | 2.21|
|agieval_lsat_rc | 0|acc |59.48|± | 3.00|
| | |acc_norm|56.51|± | 3.03|
|agieval_sat_en | 0|acc |75.24|± | 3.01|
| | |acc_norm|70.39|± | 3.19|
|agieval_sat_en_without_passage| 0|acc |39.81|± | 3.42|
| | |acc_norm|37.86|± | 3.39|
|agieval_sat_math | 0|acc |33.64|± | 3.19|
| | |acc_norm|31.82|± | 3.15|
Average: 41.0%
### GPT4All
| Task |Version| Metric |Value| |Stderr|
|-------------|------:|--------|----:|---|-----:|
|arc_challenge| 0|acc |49.74|± | 1.46|
| | |acc_norm|50.43|± | 1.46|
|arc_easy | 0|acc |76.68|± | 0.87|
| | |acc_norm|73.23|± | 0.91|
|boolq | 1|acc |79.27|± | 0.71|
|hellaswag | 0|acc |57.91|± | 0.49|
| | |acc_norm|77.13|± | 0.42|
|openbookqa | 0|acc |35.00|± | 2.14|
| | |acc_norm|43.80|± | 2.22|
|piqa | 0|acc |77.86|± | 0.97|
| | |acc_norm|79.54|± | 0.94|
|winogrande | 0|acc |69.53|± | 1.29|
Average: 67.56%
### TruthfulQA
| Task |Version|Metric|Value| |Stderr|
|-------------|------:|------|----:|---|-----:|
|truthfulqa_mc| 1|mc1 |31.21|± | 1.62|
| | |mc2 |46.36|± | 1.55|
Average: 46.36%
### Bigbench
| Task |Version| Metric |Value| |Stderr|
|------------------------------------------------|------:|---------------------|----:|---|-----:|
|bigbench_causal_judgement | 0|multiple_choice_grade|54.74|± | 3.62|
|bigbench_date_understanding | 0|multiple_choice_grade|66.67|± | 2.46|
|bigbench_disambiguation_qa | 0|multiple_choice_grade|29.46|± | 2.84|
|bigbench_geometric_shapes | 0|multiple_choice_grade|11.98|± | 1.72|
| | |exact_str_match | 0.00|± | 0.00|
|bigbench_logical_deduction_five_objects | 0|multiple_choice_grade|28.00|± | 2.01|
|bigbench_logical_deduction_seven_objects | 0|multiple_choice_grade|17.14|± | 1.43|
|bigbench_logical_deduction_three_objects | 0|multiple_choice_grade|45.67|± | 2.88|
|bigbench_movie_recommendation | 0|multiple_choice_grade|24.40|± | 1.92|
|bigbench_navigate | 0|multiple_choice_grade|53.70|± | 1.58|
|bigbench_reasoning_about_colored_objects | 0|multiple_choice_grade|68.10|± | 1.04|
|bigbench_ruin_names | 0|multiple_choice_grade|31.03|± | 2.19|
|bigbench_salient_translation_error_detection | 0|multiple_choice_grade|15.93|± | 1.16|
|bigbench_snarks | 0|multiple_choice_grade|77.35|± | 3.12|
|bigbench_sports_understanding | 0|multiple_choice_grade|52.64|± | 1.59|
|bigbench_temporal_sequences | 0|multiple_choice_grade|51.50|± | 1.58|
|bigbench_tracking_shuffled_objects_five_objects | 0|multiple_choice_grade|19.52|± | 1.12|
|bigbench_tracking_shuffled_objects_seven_objects| 0|multiple_choice_grade|13.89|± | 0.83|
|bigbench_tracking_shuffled_objects_three_objects| 0|multiple_choice_grade|45.67|± | 2.88|
Average: 39.3%
Average score: 48.56%
## Training Summary
```json
{
"train/loss": 0.299,
"train/grad_norm": 0.9337566701340533,
"train/learning_rate": 0,
"train/rewards/chosen": 0.08704188466072083,
"train/rewards/rejected": -2.835820436477661,
"train/rewards/accuracies": 0.84375,
"train/rewards/margins": 2.9228620529174805,
"train/logps/rejected": -509.9840393066406,
"train/logps/chosen": -560.8234252929688,
"train/logits/rejected": 1.6356163024902344,
"train/logits/chosen": 1.7323706150054932,
"train/epoch": 1.002169197396963,
"train/global_step": 231,
"_timestamp": 1717711643.3345022,
"_runtime": 22808.557655334473,
"_step": 231,
"train_runtime": 22809.152,
"train_samples_per_second": 1.944,
"train_steps_per_second": 0.01,
"total_flos": 0,
"train_loss": 0.44557410065745895,
"_wandb": {
"runtime": 22810
}
}
``` | [
"TRANSLATION"
] | Non_BioNLP |
DrishtiSharma/mbart-large-50-en-es-translation-lr-1e-05-weight-decay-0.0 | DrishtiSharma | translation | [
"transformers",
"pytorch",
"mbart",
"text2text-generation",
"translation",
"generated_from_trainer",
"base_model:facebook/mbart-large-50",
"base_model:finetune:facebook/mbart-large-50",
"license:mit",
"autotrain_compatible",
"endpoints_compatible",
"region:us"
] | 1,693,650,675,000 | 2023-09-02T13:50:23 | 30 | 0 | ---
base_model: facebook/mbart-large-50
license: mit
metrics:
- bleu
- rouge
tags:
- translation
- generated_from_trainer
model-index:
- name: mbart-large-50-en-es-translation-lr-1e-05-weight-decay-0.0
results: []
---
<!-- This model card has been generated automatically according to the information the Trainer had access to. You
should probably proofread and complete it, then remove this comment. -->
# mbart-large-50-en-es-translation-lr-1e-05-weight-decay-0.0
This model is a fine-tuned version of [facebook/mbart-large-50](https://huggingface.co/facebook/mbart-large-50) on the None dataset.
It achieves the following results on the evaluation set:
- Loss: 0.9549
- Bleu: 45.0307
- Rouge: {'rouge1': 0.7049318825090395, 'rouge2': 0.5238048751750992, 'rougeL': 0.684187379601513, 'rougeLsum': 0.6843574853855577}
## Model description
More information needed
## Intended uses & limitations
More information needed
## Training and evaluation data
More information needed
## Training procedure
### Training hyperparameters
The following hyperparameters were used during training:
- learning_rate: 1e-05
- train_batch_size: 8
- eval_batch_size: 8
- seed: 42
- optimizer: Adam with betas=(0.9,0.999) and epsilon=1e-08
- lr_scheduler_type: linear
- num_epochs: 4
### Training results
| Training Loss | Epoch | Step | Validation Loss | Bleu | Rouge |
|:-------------:|:-----:|:-----:|:---------------:|:-------:|:----------------------------------------------------------------------------------------------------------------------------:|
| 1.4627 | 1.0 | 4500 | 1.0255 | 42.1880 | {'rouge1': 0.6725633216905762, 'rouge2': 0.48605402524493657, 'rougeL': 0.6498853764470456, 'rougeLsum': 0.6501981166312041} |
| 0.8878 | 2.0 | 9000 | 0.9572 | 44.1734 | {'rouge1': 0.6912686406245903, 'rouge2': 0.5093695171345348, 'rougeL': 0.6701896043455414, 'rougeLsum': 0.6703473419504804} |
| 0.7125 | 3.0 | 13500 | 0.9414 | 44.8709 | {'rouge1': 0.7051197958532004, 'rouge2': 0.5210482863677958, 'rougeL': 0.6843075431636916, 'rougeLsum': 0.6846265298079588} |
| 0.6092 | 4.0 | 18000 | 0.9549 | 45.0821 | {'rouge1': 0.7047932899349161, 'rouge2': 0.523739339466653, 'rougeL': 0.6840127607742443, 'rougeLsum': 0.684202100852132} |
### Framework versions
- Transformers 4.33.0.dev0
- Pytorch 2.0.1+cu118
- Datasets 2.14.4.dev0
- Tokenizers 0.13.3
| [
"TRANSLATION"
] | Non_BioNLP |
Rostlab/ProstT5 | Rostlab | translation | [
"transformers",
"pytorch",
"t5",
"text2text-generation",
"biology",
"translation",
"dataset:adrianhenkel/lucidprots_full_data",
"license:mit",
"autotrain_compatible",
"text-generation-inference",
"endpoints_compatible",
"region:us"
] | 1,689,940,597,000 | 2023-11-16T08:51:30 | 246,724 | 20 | ---
datasets:
- adrianhenkel/lucidprots_full_data
license: mit
pipeline_tag: translation
tags:
- biology
---
# Model Card for ProstT5
<!-- Provide a quick summary of what the model is/does. -->
ProstT5 is a protein language model (pLM) which can translate between protein sequence and structure.

## Model Details
### Model Description
ProstT5 (Protein structure-sequence T5) is based on [ProtT5-XL-U50](https://huggingface.co/Rostlab/prot_t5_xl_uniref50), a T5 model trained on encoding protein sequences using span corruption applied on billions of protein sequences.
ProstT5 finetunes [ProtT5-XL-U50](https://huggingface.co/Rostlab/prot_t5_xl_uniref50) on translating between protein sequence and structure using 17M proteins with high-quality 3D structure predictions from the AlphaFoldDB.
Protein structure is converted from 3D to 1D using the 3Di-tokens introduced by [Foldseek](https://github.com/steineggerlab/foldseek).
In a first step, ProstT5 learnt to represent the newly introduced 3Di-tokens by continuing the original span-denoising objective applied on 3Di- and amino acid- (AA) sequences.
Only in a second step, ProstT5 was trained on translating between the two modalities.
The direction of the translation is indicated by two special tokens ("\<fold2AA>" for translating from 3Di to AAs, “\<AA2fold>” for translating from AAs to 3Di).
To avoid clashes with AA tokens, 3Di-tokens were cast to lower-case (alphabets are identical otherwise).
- **Developed by:** Michael Heinzinger (GitHub [@mheinzinger](https://github.com/mheinzinger); Twitter [@HeinzingerM](https://twitter.com/HeinzingerM))
- **Model type:** Encoder-decoder (T5)
- **Language(s) (NLP):** Protein sequence and structure
- **License:** MIT
- **Finetuned from model:** [ProtT5-XL-U50](https://huggingface.co/Rostlab/prot_t5_xl_uniref50)
## Uses
1. The model can be used for traditional feature extraction.
For this, we recommend using only the [encoder](https://huggingface.co/docs/transformers/model_doc/t5#transformers.T5EncoderModel) in half-precision (fp16) together with batching. Examples (currently only for original [ProtT5-XL-U50](https://huggingface.co/Rostlab/prot_t5_xl_uniref50) but replacing repository links and adding prefixes works): [script](https://github.com/agemagician/ProtTrans/blob/master/Embedding/prott5_embedder.py) and [colab](https://colab.research.google.com/drive/1h7F5v5xkE_ly-1bTQSu-1xaLtTP2TnLF?usp=sharing)
While original [ProtT5-XL-U50](https://huggingface.co/Rostlab/prot_t5_xl_uniref50) could only embed AA sequences, ProstT5 can now also embed 3D structures represented by 3Di tokens. 3Di tokens can either be derived from 3D structures using Foldseek or they can be predicted from AA sequences by ProstT5.
3. "Folding": Translation from sequence (AAs) to structure (3Di). The resulting 3Di strings can be used together with [Foldseek](https://github.com/steineggerlab/foldseek) for remote homology detection while avoiding to compute 3D structures explicitly.
4. "Inverse Folding": Translation from structure (3Di) to sequence (AA).
## How to Get Started with the Model
Feature extraction:
```python
from transformers import T5Tokenizer, T5EncoderModel
import torch
device = torch.device('cuda:0' if torch.cuda.is_available() else 'cpu')
# Load the tokenizer
tokenizer = T5Tokenizer.from_pretrained('Rostlab/ProstT5', do_lower_case=False).to(device)
# Load the model
model = T5EncoderModel.from_pretrained("Rostlab/ProstT5").to(device)
# only GPUs support half-precision currently; if you want to run on CPU use full-precision (not recommended, much slower)
model.full() if device=='cpu' else model.half()
# prepare your protein sequences/structures as a list. Amino acid sequences are expected to be upper-case ("PRTEINO" below) while 3Di-sequences need to be lower-case ("strctr" below).
sequence_examples = ["PRTEINO", "strct"]
# replace all rare/ambiguous amino acids by X (3Di sequences does not have those) and introduce white-space between all sequences (AAs and 3Di)
sequence_examples = [" ".join(list(re.sub(r"[UZOB]", "X", sequence))) for sequence in sequence_examples]
# add pre-fixes accordingly (this already expects 3Di-sequences to be lower-case)
# if you go from AAs to 3Di (or if you want to embed AAs), you need to prepend "<AA2fold>"
# if you go from 3Di to AAs (or if you want to embed 3Di), you need to prepend "<fold2AA>"
sequence_examples = [ "<AA2fold>" + " " + s if s.isupper() else "<fold2AA>" + " " + s
for s in sequence_examples
]
# tokenize sequences and pad up to the longest sequence in the batch
ids = tokenizer.batch_encode_plus(sequences_example, add_special_tokens=True, padding="longest",return_tensors='pt').to(device))
# generate embeddings
with torch.no_grad():
embedding_rpr = model(
ids.input_ids,
attention_mask=ids.attention_mask
)
# extract residue embeddings for the first ([0,:]) sequence in the batch and remove padded & special tokens, incl. prefix ([0,1:8])
emb_0 = embedding_repr.last_hidden_state[0,1:8] # shape (7 x 1024)
# same for the second ([1,:]) sequence but taking into account different sequence lengths ([1,:6])
emb_1 = embedding_repr.last_hidden_state[1,1:6] # shape (5 x 1024)
# if you want to derive a single representation (per-protein embedding) for the whole protein
emb_0_per_protein = emb_0.mean(dim=0) # shape (1024)
```
Translation ("folding", i.e., AA to 3Di):
```python
from transformers import T5Tokenizer, AutoModelForSeq2SeqLM
import torch
device = torch.device('cuda:0' if torch.cuda.is_available() else 'cpu')
# Load the tokenizer
tokenizer = T5Tokenizer.from_pretrained('Rostlab/ProstT5', do_lower_case=False).to(device)
# Load the model
model = AutoModelForSeq2SeqLM.from_pretrained("Rostlab/ProstT5").to(device)
# only GPUs support half-precision currently; if you want to run on CPU use full-precision (not recommended, much slower)
model.full() if device=='cpu' else model.half()
# prepare your protein sequences/structures as a list.
# Amino acid sequences are expected to be upper-case ("PRTEINO" below)
# while 3Di-sequences need to be lower-case.
sequence_examples = ["PRTEINO", "SEQWENCE"]
min_len = min([ len(s) for s in folding_example])
max_len = max([ len(s) for s in folding_example])
# replace all rare/ambiguous amino acids by X (3Di sequences does not have those) and introduce white-space between all sequences (AAs and 3Di)
sequence_examples = [" ".join(list(re.sub(r"[UZOB]", "X", sequence))) for sequence in sequence_examples]
# add pre-fixes accordingly. For the translation from AAs to 3Di, you need to prepend "<AA2fold>"
sequence_examples = [ "<AA2fold>" + " " + s for s in sequence_examples]
# tokenize sequences and pad up to the longest sequence in the batch
ids = tokenizer.batch_encode_plus(sequences_example,
add_special_tokens=True,
padding="longest",
return_tensors='pt').to(device))
# Generation configuration for "folding" (AA-->3Di)
gen_kwargs_aa2fold = {
"do_sample": True,
"num_beams": 3,
"top_p" : 0.95,
"temperature" : 1.2,
"top_k" : 6,
"repetition_penalty" : 1.2,
}
# translate from AA to 3Di (AA-->3Di)
with torch.no_grad():
translations = model.generate(
ids.input_ids,
attention_mask=ids.attention_mask,
max_length=max_len, # max length of generated text
min_length=min_len, # minimum length of the generated text
early_stopping=True, # stop early if end-of-text token is generated
num_return_sequences=1, # return only a single sequence
**gen_kwargs_aa2fold
)
# Decode and remove white-spaces between tokens
decoded_translations = tokenizer.batch_decode( translations, skip_special_tokens=True )
structure_sequences = [ "".join(ts.split(" ")) for ts in decoded_translations ] # predicted 3Di strings
# Now we can use the same model and invert the translation logic
# to generate an amino acid sequence from the predicted 3Di-sequence (3Di-->AA)
# add pre-fixes accordingly. For the translation from 3Di to AA (3Di-->AA), you need to prepend "<fold2AA>"
sequence_examples_backtranslation = [ "<fold2AA>" + " " + s for s in decoded_translations]
# tokenize sequences and pad up to the longest sequence in the batch
ids_backtranslation = tokenizer.batch_encode_plus(sequence_examples_backtranslation,
add_special_tokens=True,
padding="longest",
return_tensors='pt').to(device))
# Example generation configuration for "inverse folding" (3Di-->AA)
gen_kwargs_fold2AA = {
"do_sample": True,
"top_p" : 0.90,
"temperature" : 1.1,
"top_k" : 6,
"repetition_penalty" : 1.2,
}
# translate from 3Di to AA (3Di-->AA)
with torch.no_grad():
backtranslations = model.generate(
ids_backtranslation.input_ids,
attention_mask=ids_backtranslation.attention_mask,
max_length=max_len, # max length of generated text
min_length=min_len, # minimum length of the generated text
early_stopping=True, # stop early if end-of-text token is generated
num_return_sequences=1, # return only a single sequence
**gen_kwargs_fold2AA
)
# Decode and remove white-spaces between tokens
decoded_backtranslations = tokenizer.batch_decode( backtranslations, skip_special_tokens=True )
aminoAcid_sequences = [ "".join(ts.split(" ")) for ts in decoded_backtranslations ] # predicted amino acid strings
```
## Training Details
### Training Data
[Pre-training data (3Di+AA sequences for 17M proteins)](https://huggingface.co/datasets/Rostlab/ProstT5Dataset)
### Training Procedure
The first phase of the pre-training is continuing span-based denoising using 3Di- and AA-sequences using this [script](https://github.com/huggingface/transformers/blob/main/examples/flax/language-modeling/run_t5_mlm_flax.py).
For the second phase of pre-training (actual translation from 3Di- to AA-sequences and vice versa), we used this [script](https://github.com/huggingface/transformers/blob/main/examples/pytorch/summarization/run_summarization_no_trainer.py).
#### Training Hyperparameters
- **Training regime:** we used DeepSpeed (stage-2), gradient accumulation steps (5 steps), mixed half-precision (bf16) and PyTorch2.0’s torchInductor compiler
#### Speed
Generating embeddings for the human proteome from the Pro(s)tT5 encoder requires around 35m (minutes) or 0.1s (seconds) per protein using batch-processing and half-precision (fp16) on a single RTX A6000 GPU with 48 GB vRAM.
The translation is comparatively slow (0.6-2.5s/protein at an average length 135 and 406, respectively) due to the sequential nature of the decoding process which needs to generate left-to-right, token-by-token.
We only used batch-processing with half-precision without further optimization.
| [
"TRANSLATION",
"SUMMARIZATION"
] | Non_BioNLP |
YakovElm/IntelDAOS20SetFitModel_balance_ratio_3 | YakovElm | text-classification | [
"sentence-transformers",
"pytorch",
"mpnet",
"setfit",
"text-classification",
"arxiv:2209.11055",
"license:apache-2.0",
"region:us"
] | 1,685,687,194,000 | 2023-06-02T06:27:10 | 8 | 0 | ---
license: apache-2.0
pipeline_tag: text-classification
tags:
- setfit
- sentence-transformers
- text-classification
---
# YakovElm/IntelDAOS20SetFitModel_balance_ratio_3
This is a [SetFit model](https://github.com/huggingface/setfit) that can be used for text classification. The model has been trained using an efficient few-shot learning technique that involves:
1. Fine-tuning a [Sentence Transformer](https://www.sbert.net) with contrastive learning.
2. Training a classification head with features from the fine-tuned Sentence Transformer.
## Usage
To use this model for inference, first install the SetFit library:
```bash
python -m pip install setfit
```
You can then run inference as follows:
```python
from setfit import SetFitModel
# Download from Hub and run inference
model = SetFitModel.from_pretrained("YakovElm/IntelDAOS20SetFitModel_balance_ratio_3")
# Run inference
preds = model(["i loved the spiderman movie!", "pineapple on pizza is the worst 🤮"])
```
## BibTeX entry and citation info
```bibtex
@article{https://doi.org/10.48550/arxiv.2209.11055,
doi = {10.48550/ARXIV.2209.11055},
url = {https://arxiv.org/abs/2209.11055},
author = {Tunstall, Lewis and Reimers, Nils and Jo, Unso Eun Seo and Bates, Luke and Korat, Daniel and Wasserblat, Moshe and Pereg, Oren},
keywords = {Computation and Language (cs.CL), FOS: Computer and information sciences, FOS: Computer and information sciences},
title = {Efficient Few-Shot Learning Without Prompts},
publisher = {arXiv},
year = {2022},
copyright = {Creative Commons Attribution 4.0 International}
}
```
| [
"TEXT_CLASSIFICATION"
] | Non_BioNLP |
Lvxue/distilled-mt5-small-0.07-0.25 | Lvxue | text2text-generation | [
"transformers",
"pytorch",
"mt5",
"text2text-generation",
"generated_from_trainer",
"en",
"ro",
"dataset:wmt16",
"license:apache-2.0",
"model-index",
"autotrain_compatible",
"endpoints_compatible",
"region:us"
] | 1,660,209,298,000 | 2022-08-11T10:18:34 | 11 | 0 | ---
datasets:
- wmt16
language:
- en
- ro
license: apache-2.0
metrics:
- bleu
tags:
- generated_from_trainer
model-index:
- name: distilled-mt5-small-0.07-0.25
results:
- task:
type: translation
name: Translation
dataset:
name: wmt16 ro-en
type: wmt16
args: ro-en
metrics:
- type: bleu
value: 7.0665
name: Bleu
---
<!-- This model card has been generated automatically according to the information the Trainer had access to. You
should probably proofread and complete it, then remove this comment. -->
# distilled-mt5-small-0.07-0.25
This model is a fine-tuned version of [google/mt5-small](https://huggingface.co/google/mt5-small) on the wmt16 ro-en dataset.
It achieves the following results on the evaluation set:
- Loss: 2.8593
- Bleu: 7.0665
- Gen Len: 43.5793
## Model description
More information needed
## Intended uses & limitations
More information needed
## Training and evaluation data
More information needed
## Training procedure
### Training hyperparameters
The following hyperparameters were used during training:
- learning_rate: 5e-05
- train_batch_size: 4
- eval_batch_size: 8
- seed: 42
- optimizer: Adam with betas=(0.9,0.999) and epsilon=1e-08
- lr_scheduler_type: linear
- num_epochs: 5.0
### Training results
### Framework versions
- Transformers 4.20.1
- Pytorch 1.12.0+cu102
- Datasets 2.3.2
- Tokenizers 0.12.1
| [
"TRANSLATION"
] | Non_BioNLP |
mukayese/transformer-turkish-summarization | mukayese | summarization | [
"transformers",
"pytorch",
"safetensors",
"bart",
"text2text-generation",
"summarization",
"tr",
"dataset:mlsum",
"arxiv:2203.01215",
"license:mit",
"model-index",
"autotrain_compatible",
"endpoints_compatible",
"region:us"
] | 1,647,953,899,000 | 2023-03-24T11:20:38 | 41 | 5 | ---
datasets:
- mlsum
language:
- tr
license: mit
metrics:
- rouge
pipeline_tag: summarization
model-index:
- name: mukayese/transformer-turkish-summarization
results:
- task:
type: summarization
name: Summarization
dataset:
name: mlsum tu
type: mlsum
args: tu
metrics:
- type: rouge
value: 43.2049
name: Rouge1
---
# [Mukayese: Turkish NLP Strikes Back](https://arxiv.org/abs/2203.01215)
## Summarization: mukayese/transformer-turkish-summarization
_This model is uncased_, it was initialized from scratch and trained only the mlsum/tu dataset with no pre-training.
It achieves the following results on the evaluation set:
- Rouge1: 43.2049
- Rouge2: 30.7082
- Rougel: 38.1981
- Rougelsum: 39.9453
Check [this](https://arxiv.org/abs/2203.01215) paper for more details on the model and the dataset.
## Training procedure
### Training hyperparameters
The following hyperparameters were used during training:
- learning_rate: 0.0001
- train_batch_size: 4
- eval_batch_size: 8
- seed: 42
- distributed_type: multi-GPU
- num_devices: 8
- gradient_accumulation_steps: 2
- total_train_batch_size: 64
- total_eval_batch_size: 64
- optimizer: Adam with betas=(0.9,0.999) and epsilon=1e-08
- lr_scheduler_type: linear
- num_epochs: 15.0
- mixed_precision_training: Native AMP
- label_smoothing_factor: 0.1
### Framework versions
- Transformers 4.11.3
- Pytorch 1.8.2+cu111
- Datasets 1.14.0
- Tokenizers 0.10.3
### Citation
```
@misc{safaya-etal-2022-mukayese,
title={Mukayese: Turkish NLP Strikes Back},
author={Ali Safaya and Emirhan Kurtuluş and Arda Göktoğan and Deniz Yuret},
year={2022},
eprint={2203.01215},
archivePrefix={arXiv},
primaryClass={cs.CL}
}
``` | [
"SUMMARIZATION"
] | Non_BioNLP |
neuralmagic/Pixtral-Large-Instruct-2411-hf-quantized.w4a16 | neuralmagic | image-text-to-text | [
"vllm",
"safetensors",
"llava",
"w4a16",
"int4",
"vision",
"image-text-to-text",
"conversational",
"en",
"fr",
"de",
"es",
"it",
"pt",
"zh",
"ja",
"ru",
"ko",
"license:other",
"compressed-tensors",
"region:us"
] | 1,738,947,609,000 | 2025-03-04T15:17:54 | 363 | 0 | ---
base_model: neuralmagic/Pixtral-Large-Instruct-2411-hf
language:
- en
- fr
- de
- es
- it
- pt
- zh
- ja
- ru
- ko
library_name: vllm
license: other
license_name: mrl
license_link: https://mistral.ai/licenses/MRL-0.1.md
pipeline_tag: image-text-to-text
tags:
- w4a16
- int4
- vllm
- vision
inference: false
extra_gated_prompt: '# Mistral AI Research License
If You want to use a Mistral Model, a Derivative or an Output for any purpose that
is not expressly authorized under this Agreement, You must request a license from
Mistral AI, which Mistral AI may grant to You in Mistral AI''s sole discretion.
To discuss such a license, please contact Mistral AI via the website contact form:
https://mistral.ai/contact/
## 1. Scope and acceptance
**1.1. Scope of the Agreement.** This Agreement applies to any use, modification,
or Distribution of any Mistral Model by You, regardless of the source You obtained
a copy of such Mistral Model.
**1.2. Acceptance.** By accessing, using, modifying, Distributing a Mistral Model,
or by creating, using or distributing a Derivative of the Mistral Model, You agree
to be bound by this Agreement.
**1.3. Acceptance on behalf of a third-party.** If You accept this Agreement on
behalf of Your employer or another person or entity, You warrant and represent that
You have the authority to act and accept this Agreement on their behalf. In such
a case, the word "You" in this Agreement will refer to Your employer or such other
person or entity.
## 2. License
**2.1. Grant of rights**. Subject to Section 3 below, Mistral AI hereby grants
You a non-exclusive, royalty-free, worldwide, non-sublicensable, non-transferable,
limited license to use, copy, modify, and Distribute under the conditions provided
in Section 2.2 below, the Mistral Model and any Derivatives made by or for Mistral
AI and to create Derivatives of the Mistral Model.
**2.2. Distribution of Mistral Model and Derivatives made by or for Mistral AI.**
Subject to Section 3 below, You may Distribute copies of the Mistral Model and/or
Derivatives made by or for Mistral AI, under the following conditions: You must
make available a copy of this Agreement to third-party recipients of the Mistral
Models and/or Derivatives made by or for Mistral AI you Distribute, it being specified
that any rights to use the Mistral Models and/or Derivatives made by or for Mistral
AI shall be directly granted by Mistral AI to said third-party recipients pursuant
to the Mistral AI Research License agreement executed between these parties; You
must retain in all copies of the Mistral Models the following attribution notice
within a "Notice" text file distributed as part of such copies: "Licensed by Mistral
AI under the Mistral AI Research License".
**2.3. Distribution of Derivatives made by or for You.** Subject to Section 3 below,
You may Distribute any Derivatives made by or for You under additional or different
terms and conditions, provided that: In any event, the use and modification of Mistral
Model and/or Derivatives made by or for Mistral AI shall remain governed by the
terms and conditions of this Agreement; You include in any such Derivatives made
by or for You prominent notices stating that You modified the concerned Mistral
Model; and Any terms and conditions You impose on any third-party recipients relating
to Derivatives made by or for You shall neither limit such third-party recipients''
use of the Mistral Model or any Derivatives made by or for Mistral AI in accordance
with the Mistral AI Research License nor conflict with any of its terms and conditions.
## 3. Limitations
**3.1. Misrepresentation.** You must not misrepresent or imply, through any means,
that the Derivatives made by or for You and/or any modified version of the Mistral
Model You Distribute under your name and responsibility is an official product of
Mistral AI or has been endorsed, approved or validated by Mistral AI, unless You
are authorized by Us to do so in writing.
**3.2. Usage Limitation.** You shall only use the Mistral Models, Derivatives (whether
or not created by Mistral AI) and Outputs for Research Purposes.
## 4. Intellectual Property
**4.1. Trademarks.** No trademark licenses are granted under this Agreement, and
in connection with the Mistral Models, You may not use any name or mark owned by
or associated with Mistral AI or any of its affiliates, except (i) as required for
reasonable and customary use in describing and Distributing the Mistral Models and
Derivatives made by or for Mistral AI and (ii) for attribution purposes as required
by this Agreement.
**4.2. Outputs.** We claim no ownership rights in and to the Outputs. You are solely
responsible for the Outputs You generate and their subsequent uses in accordance
with this Agreement. Any Outputs shall be subject to the restrictions set out in
Section 3 of this Agreement.
**4.3. Derivatives.** By entering into this Agreement, You accept that any Derivatives
that You may create or that may be created for You shall be subject to the restrictions
set out in Section 3 of this Agreement.
## 5. Liability
**5.1. Limitation of liability.** In no event, unless required by applicable law
(such as deliberate and grossly negligent acts) or agreed to in writing, shall Mistral
AI be liable to You for damages, including any direct, indirect, special, incidental,
or consequential damages of any character arising as a result of this Agreement
or out of the use or inability to use the Mistral Models and Derivatives (including
but not limited to damages for loss of data, loss of goodwill, loss of expected
profit or savings, work stoppage, computer failure or malfunction, or any damage
caused by malware or security breaches), even if Mistral AI has been advised of
the possibility of such damages.
**5.2. Indemnification.** You agree to indemnify and hold harmless Mistral AI from
and against any claims, damages, or losses arising out of or related to Your use
or Distribution of the Mistral Models and Derivatives.
## 6. Warranty
**6.1. Disclaimer.** Unless required by applicable law or prior agreed to by Mistral
AI in writing, Mistral AI provides the Mistral Models and Derivatives on an "AS
IS" BASIS, WITHOUT WARRANTIES OR CONDITIONS OF ANY KIND, either express or implied,
including, without limitation, any warranties or conditions of TITLE, NON-INFRINGEMENT,
MERCHANTABILITY, or FITNESS FOR A PARTICULAR PURPOSE. Mistral AI does not represent
nor warrant that the Mistral Models and Derivatives will be error-free, meet Your
or any third party''s requirements, be secure or will allow You or any third party
to achieve any kind of result or generate any kind of content. You are solely responsible
for determining the appropriateness of using or Distributing the Mistral Models
and Derivatives and assume any risks associated with Your exercise of rights under
this Agreement.
## 7. Termination
**7.1. Term.** This Agreement is effective as of the date of your acceptance of
this Agreement or access to the concerned Mistral Models or Derivatives and will
continue until terminated in accordance with the following terms.
**7.2. Termination.** Mistral AI may terminate this Agreement at any time if You
are in breach of this Agreement. Upon termination of this Agreement, You must cease
to use all Mistral Models and Derivatives and shall permanently delete any copy
thereof. The following provisions, in their relevant parts, will survive any termination
or expiration of this Agreement, each for the duration necessary to achieve its
own intended purpose (e.g. the liability provision will survive until the end of
the applicable limitation period):Sections 5 (Liability), 6(Warranty), 7 (Termination)
and 8 (General Provisions).
**7.3. Litigation.** If You initiate any legal action or proceedings against Us
or any other entity (including a cross-claim or counterclaim in a lawsuit), alleging
that the Model or a Derivative, or any part thereof, infringe upon intellectual
property or other rights owned or licensable by You, then any licenses granted to
You under this Agreement will immediately terminate as of the date such legal action
or claim is filed or initiated.
## 8. General provisions
**8.1. Governing laws.** This Agreement will be governed by the laws of France,
without regard to choice of law principles, and the UN Convention on Contracts for
the International Sale of Goods does not apply to this Agreement.
**8.2. Competent jurisdiction.** The courts of Paris shall have exclusive jurisdiction
of any dispute arising out of this Agreement.
**8.3. Severability.** If any provision of this Agreement is held to be invalid,
illegal or unenforceable, the remaining provisions shall be unaffected thereby and
remain valid as if such provision had not been set forth herein.
## 9. Definitions
"Agreement": means this Mistral AI Research License agreement governing the access,
use, and Distribution of the Mistral Models, Derivatives and Outputs.
"Derivative": means any (i) modified version of the Mistral Model (including but
not limited to any customized or fine-tuned version thereof), (ii) work based on
the Mistral Model, or (iii) any other derivative work thereof.
"Distribution", "Distributing", "Distribute" or "Distributed": means supplying,
providing or making available, by any means, a copy of the Mistral Models and/or
the Derivatives as the case may be, subject to Section 3 of this Agreement.
"Mistral AI", "We" or "Us": means Mistral AI, a French société par actions simplifiée
registered in the Paris commercial registry under the number 952 418 325, and having
its registered seat at 15, rue des Halles, 75001 Paris.
"Mistral Model": means the foundational large language model(s), and its elements
which include algorithms, software, instructed checkpoints, parameters, source code
(inference code, evaluation code and, if applicable, fine-tuning code) and any other
elements associated thereto made available by Mistral AI under this Agreement, including,
if any, the technical documentation, manuals and instructions for the use and operation
thereof.
"Research Purposes": means any use of a Mistral Model, Derivative, or Output that
is solely for (a) personal, scientific or academic research, and (b) for non-profit
and non-commercial purposes, and not directly or indirectly connected to any commercial
activities or business operations. For illustration purposes, Research Purposes
does not include (1) any usage of the Mistral Model, Derivative or Output by individuals
or contractors employed in or engaged by companies in the context of (a) their daily
tasks, or (b) any activity (including but not limited to any testing or proof-of-concept)
that is intended to generate revenue, nor (2) any Distribution by a commercial entity
of the Mistral Model, Derivative or Output whether in return for payment or free
of charge, in any medium or form, including but not limited to through a hosted
or managed service (e.g. SaaS, cloud instances, etc.), or behind a software layer.
"Outputs": means any content generated by the operation of the Mistral Models or
the Derivatives from a prompt (i.e., text instructions) provided by users. For
the avoidance of doubt, Outputs do not include any components of a Mistral Models,
such as any fine-tuned versions of the Mistral Models, the weights, or parameters.
"You": means the individual or entity entering into this Agreement with Mistral
AI.
*Mistral AI processes your personal data below to provide the model and enforce
its license. If you are affiliated with a commercial entity, we may also send you
communications about our models. For more information on your rights and data handling,
please see our <a href="https://mistral.ai/terms/">privacy policy</a>.*'
extra_gated_fields:
First Name: text
Last Name: text
Country: country
Affiliation: text
Job title: text
I understand that I can only use the model, any derivative versions and their outputs for non-commercial research purposes: checkbox
? I understand that if I am a commercial entity, I am not permitted to use or distribute
the model internally or externally, or expose it in my own offerings without a
commercial license
: checkbox
? I understand that if I upload the model, or any derivative version, on any platform,
I must include the Mistral Research License
: checkbox
? I understand that for commercial use of the model, I can contact Mistral or use
the Mistral AI API on la Plateforme or any of our cloud provider partners
: checkbox
? By clicking Submit below I accept the terms of the license and acknowledge that
the information I provide will be collected stored processed and shared in accordance
with the Mistral Privacy Policy
: checkbox
geo: ip_location
extra_gated_description: Mistral AI processes your personal data below to provide
the model and enforce its license. If you are affiliated with a commercial entity,
we may also send you communications about our models. For more information on your
rights and data handling, please see our <a href="https://mistral.ai/terms/">privacy
policy</a>.
extra_gated_button_content: Submit
---
# Pixtral-Large-Instruct-2411-hf-quantized.w4a16
## Model Overview
- **Model Architecture:** neuralmagic/Pixtral-Large-Instruct-2411-hf
- **Input:** Vision-Text
- **Output:** Text
- **Model Optimizations:**
- **Weight quantization:** INT4
- **Activation quantization:** FP16
- **Release Date:** 2/24/2025
- **Version:** 1.0
- **Model Developers:** Neural Magic
Quantized version of [neuralmagic/Pixtral-Large-Instruct-2411-hf](https://huggingface.co/neuralmagic/Pixtral-Large-Instruct-2411-hf/tree/main).
### Model Optimizations
This model was obtained by quantizing the weights of [neuralmagic/Pixtral-Large-Instruct-2411-hf](https://huggingface.co/neuralmagic/Pixtral-Large-Instruct-2411-hf/tree/main) to INT4 data type, ready for inference with vLLM >= 0.5.2.
## Deployment
### Use with vLLM
This model can be deployed efficiently using the [vLLM](https://docs.vllm.ai/en/latest/) backend, as shown in the example below.
```python
from vllm.assets.image import ImageAsset
from vllm import LLM, SamplingParams
# prepare model
llm = LLM(
model="neuralmagic/Pixtral-Large-Instruct-2411-hf-quantized.w4a16",
trust_remote_code=True,
max_model_len=4096,
max_num_seqs=2,
)
# prepare inputs
question = "What is the content of this image?"
inputs = {
"prompt": f"<|user|>\n<|image_1|>\n{question}<|end|>\n<|assistant|>\n",
"multi_modal_data": {
"image": ImageAsset("cherry_blossom").pil_image.convert("RGB")
},
}
# generate response
print("========== SAMPLE GENERATION ==============")
outputs = llm.generate(inputs, SamplingParams(temperature=0.2, max_tokens=64))
print(f"PROMPT : {outputs[0].prompt}")
print(f"RESPONSE: {outputs[0].outputs[0].text}")
print("==========================================")
```
vLLM also supports OpenAI-compatible serving. See the [documentation](https://docs.vllm.ai/en/latest/) for more details.
## Creation
This model was created with [llm-compressor](https://github.com/vllm-project/llm-compressor) by running the code snippet below as part a multimodal announcement blog.
<details>
<summary>Model Creation Code</summary>
```python
import requests
import torch
from PIL import Image
from transformers import AutoProcessor
from llmcompressor.modifiers.quantization import GPTQModifier
from llmcompressor.transformers import oneshot
from llmcompressor.transformers.tracing import TraceableLlavaForConditionalGeneration
from compressed_tensors.quantization import QuantizationArgs, QuantizationType, QuantizationStrategy, ActivationOrdering, QuantizationScheme
# Load model.
model_id = "neuralmagic/Pixtral-Large-Instruct-2411-hf"
model = TraceableLlavaForConditionalGeneration.from_pretrained(
model_id, device_map="auto", torch_dtype="auto"
)
processor = AutoProcessor.from_pretrained(model_id, trust_remote_code=True)
# Oneshot arguments
DATASET_ID = "flickr30k"
DATASET_SPLIT = {"calibration": "test[:512]"}
NUM_CALIBRATION_SAMPLES = 512
MAX_SEQUENCE_LENGTH = 2048
dampening_frac=0.01
# Define a oneshot data collator for multimodal inputs.
def data_collator(batch):
assert len(batch) == 1
return {
"input_ids": torch.LongTensor(batch[0]["input_ids"]),
"attention_mask": torch.tensor(batch[0]["attention_mask"]),
"pixel_values": torch.tensor(batch[0]["pixel_values"]),
}
recipe = GPTQModifier(
targets="Linear",
config_groups={
"config_group": QuantizationScheme(
targets=["Linear"],
weights=QuantizationArgs(
num_bits=4,
type=QuantizationType.INT,
strategy=QuantizationStrategy.GROUP,
group_size=128,
symmetric=True,
dynamic=False,
actorder=ActivationOrdering.WEIGHT,
),
),
},
sequential_targets=["MistralDecoderLayer"],
ignore=["re:.*lm_head", "re:vision_tower.*", "re:multi_modal_projector.*"],
update_size=NUM_CALIBRATION_SAMPLES,
dampening_frac=dampening_frac,
)
SAVE_DIR=f"{model_id.split('/')[1]}-quantized.w4a16"
# Perform oneshot
oneshot(
model=model,
tokenizer=model_id,
dataset=DATASET_ID,
splits=DATASET_SPLIT,
recipe=recipe,
max_seq_length=MAX_SEQUENCE_LENGTH,
num_calibration_samples=NUM_CALIBRATION_SAMPLES,
trust_remote_code_model=True,
data_collator=data_collator,
output_dir=SAVE_DIR
)
```
</details>
## Evaluation
The model was evaluated using [mistral-evals](https://github.com/neuralmagic/mistral-evals) for vision-related tasks and using [lm_evaluation_harness](https://github.com/neuralmagic/lm-evaluation-harness) for select text-based benchmarks. The evaluations were conducted using the following commands:
<details>
<summary>Evaluation Commands</summary>
### Vision Tasks
- vqav2
- docvqa
- mathvista
- mmmu
- chartqa
```
vllm serve neuralmagic/pixtral-12b-quantized.w8a8 --tensor_parallel_size 1 --max_model_len 25000 --trust_remote_code --max_num_seqs 8 --gpu_memory_utilization 0.9 --dtype float16 --limit_mm_per_prompt image=7
python -m eval.run eval_vllm \
--model_name neuralmagic/pixtral-12b-quantized.w8a8 \
--url http://0.0.0.0:8000 \
--output_dir ~/tmp \
--eval_name <vision_task_name>
```
### Text-based Tasks
#### MMLU
```
lm_eval \
--model vllm \
--model_args pretrained="<model_name>",dtype=auto,add_bos_token=True,max_model_len=4096,tensor_parallel_size=<n>,gpu_memory_utilization=0.8,enable_chunked_prefill=True,trust_remote_code=True \
--tasks mmlu \
--num_fewshot 5 \
--batch_size auto \
--output_path output_dir
```
#### MGSM
```
lm_eval \
--model vllm \
--model_args pretrained="<model_name>",dtype=auto,max_model_len=4096,max_gen_toks=2048,max_num_seqs=128,tensor_parallel_size=<n>,gpu_memory_utilization=0.9 \
--tasks mgsm_cot_native \
--num_fewshot 0 \
--batch_size auto \
--output_path output_dir
```
</details>
</details>
### Accuracy
<table>
<thead>
<tr>
<th>Category</th>
<th>Metric</th>
<th>neuralmagic/Pixtral-Large-Instruct-2411-hf</th>
<th>neuralmagic/Pixtral-Large-Instruct-2411-hf-quantized.w4a16</th>
<th>Recovery (%)</th>
</tr>
</thead>
<tbody>
<tr>
<td rowspan="6"><b>Vision</b></td>
<td>MMMU (val, CoT)<br><i>explicit_prompt_relaxed_correctness</i></td>
<td>63.56</td>
<td>60.56</td>
<td>95.28%</td>
</tr>
<tr>
<td>VQAv2 (val)<br><i>vqa_match</i></td>
<td>79.03</td>
<td>79.04</td>
<td>100.01%</td>
</tr>
<tr>
<td>DocVQA (val)<br><i>anls</i></td>
<td>89.55</td>
<td>89.00</td>
<td>99.39%</td>
</tr>
<tr>
<td>ChartQA (test, CoT)<br><i>anywhere_in_answer_relaxed_correctness</i></td>
<td>82.24</td>
<td>81.52</td>
<td>99.12%</td>
</tr>
<tr>
<td>Mathvista (testmini, CoT)<br><i>explicit_prompt_relaxed_correctness</i></td>
<td>67.3</td>
<td>66.60</td>
<td>98.96%</td>
</tr>
<tr>
<td><b>Average Score</b></td>
<td><b>76.34</b></td>
<td><b>75.34</b></td>
<td><b>98.69%</b></td>
</tr>
<tr>
<td rowspan="2"><b>Text</b></td>
<td>MGSM (CoT)</td>
<td>76.05</td>
<td>75.09</td>
<td>98.74%</td>
</tr>
<tr>
<td>MMLU (5-shot)</td>
<td>82.8</td>
<td>82.25</td>
<td>99.33%</td>
</tr>
</tbody>
</table>
## Inference Performance
This model achieves up to 2.80x speedup in single-stream deployment and up to 1.75x speedup in multi-stream asynchronous deployment, depending on hardware and use-case scenario.
The following performance benchmarks were conducted with [vLLM](https://docs.vllm.ai/en/latest/) version 0.7.2, and [GuideLLM](https://github.com/neuralmagic/guidellm).
<details>
<summary>Benchmarking Command</summary>
```
guidellm --model neuralmagic/Pixtral-Large-Instruct-2411-hf-quantized.w4a16 --target "http://localhost:8000/v1" --data-type emulated --data prompt_tokens=<prompt_tokens>,generated_tokens=<generated_tokens>,images=<num_images>,width=<image_width>,height=<image_height> --max seconds 120 --backend aiohttp_server
```
</details>
### Single-stream performance (measured with vLLM version 0.7.2)
<table border="1" class="dataframe">
<thead>
<tr>
<th></th>
<th></th>
<th></th>
<th></th>
<th style="text-align: center;" colspan="2" >Document Visual Question Answering<br>1680W x 2240H<br>64/128</th>
<th style="text-align: center;" colspan="2" >Visual Reasoning <br>640W x 480H<br>128/128</th>
<th style="text-align: center;" colspan="2" >Image Captioning<br>480W x 360H<br>0/128</th>
</tr>
<tr>
<th>Hardware</th>
<th>Number of GPUs</th>
<th>Model</th>
<th>Average Cost Reduction</th>
<th>Latency (s)</th>
<th>Queries Per Dollar</th>
<th>Latency (s)</th>
<th>Queries Per Dollar</th>
<th>Latency (s)</th>
<th>Queries Per Dollar</th>
</tr>
</thead>
<tbody style="text-align: center">
<tr>
<th rowspan="3" valign="top">A100</th>
<td>4</td>
<td>neuralmagic/Pixtral-Large-Instruct-2411-hf</td>
<td></td>
<td>7.5</td>
<td>67</td>
<td>6.5</td>
<td>77</td>
<td>6.4</td>
<td>79</td>
</tr>
<tr>
<td>2</td>
<td>neuralmagic/Pixtral-Large-Instruct-2411-hf-quantized.w8a8</td>
<td>1.86</td>
<td>8.1</td>
<td>124</td>
<td>7.1</td>
<td>142</td>
<td>6.8</td>
<td>148</td>
</tr>
<tr>
<td>2</td>
<td>neuralmagic/Pixtral-Large-Instruct-2411-hf-quantized.w4a16</td>
<td>2.52</td>
<td>6.9</td>
<td>147</td>
<td>5.1</td>
<td>199</td>
<td>4.5</td>
<td>221</td>
</tr>
<tr>
<th rowspan="3" valign="top">H100</th>
<td>4</td>
<td>neuralmagic/Pixtral-Large-Instruct-2411-hf</td>
<td></td>
<td>4.4</td>
<td>67</td>
<td>3.9</td>
<td>74</td>
<td>3.7</td>
<td>79</td>
</tr>
<tr>
<td>2</td>
<td>neuralmagic/Pixtral-Large-Instruct-2411-hf-FP8-Dynamic</td>
<td>1.82</td>
<td>4.7</td>
<td>120</td>
<td>4.1</td>
<td>137</td>
<td>3.9</td>
<td>145</td>
</tr>
<tr>
<td>2</td>
<td>neuralmagic/Pixtral-Large-Instruct-2411-hf-quantized.w4a16</td>
<td>1.87</td>
<td>4.7</td>
<td>120</td>
<td>3.9</td>
<td>144</td>
<td>3.8</td>
<td>149</td>
</tr>
</tbody>
</table>
**Use case profiles: Image Size (WxH) / prompt tokens / generation tokens
**QPD: Queries per dollar, based on on-demand cost at [Lambda Labs](https://lambdalabs.com/service/gpu-cloud) (observed on 2/18/2025).
### Multi-stream asynchronous performance (measured with vLLM version 0.7.2)
<table border="1" class="dataframe">
<thead>
<tr>
<th></th>
<th></th>
<th></th>
<th style="text-align: center;" colspan="2" >Document Visual Question Answering<br>1680W x 2240H<br>64/128</th>
<th style="text-align: center;" colspan="2" >Visual Reasoning <br>640W x 480H<br>128/128</th>
<th style="text-align: center;" colspan="2" >Image Captioning<br>480W x 360H<br>0/128</th>
</tr>
<tr>
<th>Hardware</th>
<th>Model</th>
<th>Average Cost Reduction</th>
<th>Maximum throughput (QPS)</th>
<th>Queries Per Dollar</th>
<th>Maximum throughput (QPS)</th>
<th>Queries Per Dollar</th>
<th>Maximum throughput (QPS)</th>
<th>Queries Per Dollar</th>
</tr>
</thead>
<tbody style="text-align: center">
<tr>
<th rowspan="3" valign="top">A100x4</th>
<td>neuralmagic/Pixtral-Large-Instruct-2411-hf</td>
<td></td>
<td>0.4</td>
<td>222</td>
<td>0.7</td>
<td>341</td>
<td>0.8</td>
<td>399</td>
</tr>
<tr>
<td>neuralmagic/Pixtral-Large-Instruct-2411-hf-quantized.w8a8</td>
<td>1.70</td>
<td>0.8</td>
<td>383</td>
<td>1.1</td>
<td>571</td>
<td>1.3</td>
<td>674</td>
</tr>
<tr>
<td>neuralmagic/Pixtral-Large-Instruct-2411-hf-quantized.w4a16</td>
<td>1.48</td>
<td>0.5</td>
<td>276</td>
<td>1.0</td>
<td>505</td>
<td>1.4</td>
<td>680</td>
</tr>
<tr>
<<th rowspan="3" valign="top">H100x4</th>
<td>neuralmagic/Pixtral-Large-Instruct-2411-hf</td>
<td></td>
<td>1.0</td>
<td>284</td>
<td>1.6</td>
<td>465</td>
<td>1.8</td>
<td>511</td>
</tr>
<tr>
<td>neuralmagic/Pixtral-Large-Instruct-2411-hf-FP8-Dynamic</td>
<td>1.61</td>
<td>1.7</td>
<td>467</td>
<td>2.6</td>
<td>726</td>
<td>3.2</td>
<td>908</td>
</tr>
<tr>
<td>neuralmagic/Pixtral-Large-Instruct-2411-hf-quantized.w4a16</td>
<td>1.33</td>
<td>1.4</td>
<td>393</td>
<td>2.2</td>
<td>726</td>
<td>2.7</td>
<td>764</td>
</tr>
</tbody>
</table>
**Use case profiles: Image Size (WxH) / prompt tokens / generation tokens
**QPS: Queries per second.
**QPD: Queries per dollar, based on on-demand cost at [Lambda Labs](https://lambdalabs.com/service/gpu-cloud) (observed on 2/18/2025).
## The Mistral AI Team
Albert Jiang, Alexandre Sablayrolles, Alexis Tacnet, Alok Kothari, Antoine Roux, Arthur Mensch, Audrey Herblin-Stoop, Augustin Garreau, Austin Birky, Bam4d, Baptiste Bout, Baudouin de Monicault, Blanche Savary, Carole Rambaud, Caroline Feldman, Devendra Singh Chaplot, Diego de las Casas, Diogo Costa, Eleonore Arcelin, Emma Bou Hanna, Etienne Metzger, Gaspard Blanchet, Gianna Lengyel, Guillaume Bour, Guillaume Lample, Harizo Rajaona, Henri Roussez, Hichem Sattouf, Ian Mack, Jean-Malo Delignon, Jessica Chudnovsky, Justus Murke, Kartik Khandelwal, Lawrence Stewart, Louis Martin, Louis Ternon, Lucile Saulnier, Lélio Renard Lavaud, Margaret Jennings, Marie Pellat, Marie Torelli, Marie-Anne Lachaux, Marjorie Janiewicz, Mickaël Seznec, Nicolas Schuhl, Niklas Muhs, Olivier de Garrigues, Patrick von Platen, Paul Jacob, Pauline Buche, Pavan Kumar Reddy, Perry Savas, Pierre Stock, Romain Sauvestre, Sagar Vaze, Sandeep Subramanian, Saurabh Garg, Sophia Yang, Szymon Antoniak, Teven Le Scao, Thibault Schueller, Thibaut Lavril, Thomas Wang, Théophile Gervet, Timothée Lacroix, Valera Nemychnikova, Wendy Shang, William El Sayed, William Marshall | [
"QUESTION_ANSWERING"
] | Non_BioNLP |
LeroyDyer/QuietStar_Project | LeroyDyer | text-generation | [
"transformers",
"mistral",
"text-generation",
"Mistral_Star",
"Mistral_Quiet",
"Mistral",
"Mixtral",
"Question-Answer",
"Token-Classification",
"Sequence-Classification",
"SpydazWeb-AI",
"chemistry",
"biology",
"legal",
"code",
"climate",
"medical",
"text-generation-inference",
"custom_code",
"en",
"sw",
"ig",
"zu",
"ca",
"es",
"pt",
"ha",
"license:mit",
"autotrain_compatible",
"region:us"
] | 1,721,300,706,000 | 2024-07-20T08:43:35 | 9 | 2 | ---
language:
- en
- sw
- ig
- zu
- ca
- es
- pt
- ha
license: mit
pipeline_tag: text-generation
tags:
- Mistral_Star
- Mistral_Quiet
- Mistral
- Mixtral
- Question-Answer
- Token-Classification
- Sequence-Classification
- SpydazWeb-AI
- chemistry
- biology
- legal
- code
- climate
- medical
- text-generation-inference
---
# SpydazWeb AGI
This is based on the Quiet Star Reasoning Project : which was abandoned earlier in the year :)
These are some associated files ...
# Introduction :
## STAR REASONERS !
this provides a platform for the model to commuicate pre-response , so an internal objective can be set ie adding an extra planning stage to the model improving its focus and output:
the thought head can be charged with a thought or methodolgy, such as a ststing to take a step by step approach to the problem or to make an object oriented model first and consider the use cases before creating an output:
so each thought head can be dedicated to specific ppurpose such as Planning or artifact generation or use case design : or even deciding which methodology should be applied before planning the potential solve route for the response :
Another head could also be dedicated to retrieving content based on the query from the self which can also be used in the pregenerations stages :
all pre- reasoners can be seen to be Self Guiding ! essentially removing the requirement to give the model a system prompt instead aligning the heads to a thoght pathways !
these chains produce data which can be considered to be thoughts : and can further be displayed by framing these thoughts with thought tokens : even allowing for editors comments giving key guidance to the model during training :
these thoughts will be used in future genrations assisting the model as well a displaying explantory informations in the output :
these tokens can be displayed or with held also a setting in the model !
### can this be applied in other areas ?
Yes! , we can use this type of method to allow for the model to generate code in another channel or head potentially creating a head to produce artifacts for every output , or to produce entity lilsts for every output and framing the outputs in thier relative code tags or function call tags :
these can also be displayed or hidden for the response . but these can also be used in problem solvibng tasks internally , which again enables for the model to simualte the inpouts and outputs from an interpretor !
it may even be prudent to include a function executing internally to the model ! ( allowing the model to execute functions in the background! before responding ) as well this oul hae tpo also be specified in the config , as autoexecute or not !.
### Conclusion
the resonaer methodology , might be seen to be the way forwards , adding internal funciton laity to the models instead of external connectivity enables for faster and seemless model usage : as well as enriched and informed responses , as even outputs could essentially be cleanss and formated before being presented to the Calling interface, internally to the model :
the take away is that arre we seeing the decoder/encoder model as simple a function of the inteligence which in truth need to be autonomus !
ie internal functions and tools as well as disk interaction : an agent must have awareness and control over its environment with sensors and actuators : as a fuction callingmodel it has actuators and canread the directorys it has sensors ... its a start: as we can eget media in and out , but the model needs to get its own control to inpout and output also !
....
Fine tuning : agin this issue of fine tuning : the disussion above eplains the requirement to control the environment from within the moel ( with constraints ) does this eliminate theneed to fine tune a model !
in fact it should as this give transparency to ther growth ofthe model and if the model fine tuned itself we would be in danger of a model evolveing !
hence an AGI !
#### AI AGI ?
so yes we can see we are not far from an ai which can evolve : an advance general inteligent system ( still non sentient by the way )
<img src="https://cdn-avatars.huggingface.co/v1/production/uploads/65d883893a52cd9bcd8ab7cf/tRsCJlHNZo1D02kBTmfy9.jpeg" width="300"/>
https://github.com/spydaz
* 32k context window (vs 8k context in v0.1)
* Rope-theta = 1e6
* No Sliding-Window Attention
* Talk heads - produce resposnes which can be used towards the final output
* Pre-Thoughts - Enables for pre-generation steps of potential artifacts for task solving:
* Generates plans for step by step thinking
* Generates python Code Artifacts for future tasks
* Recalls context for task internally to be used as refference for task:
* show thoughts or hidden thought usages ( Simular to self-Rag )
This model will be a custom model with internal experts and rag systems
enabling for preprocessing of the task internally before outputting a response
## SpydazWeb AI model :
This model is based on the worlds archive of knowledge maintaining historical documents and providing services for the survivors of mankind ,
who may need to construct shelters develop technologys , or medical resources as well as maintain the history of the past . keeping store of all the religious knowledge and data of the world:
A friendly interface with a personality caring and flirtatious at times : non binary !...
and Expert in all feilds: ie Uncensored and will not refuse to give information : the model can be used for role play as many character dialogues were als trained into the model as its personality to enable a greater perspective and outlook and natural discussion with the agents:
the model was trained to operateinaragenvironment utilizing content and internal knowledge to respond to questions or create enriched sumarys.
### General Intenal Methods:
Trained for multi-task operations as well as rag and function calling :
This model is a fully functioning model and is fully uncensored:
the model has been trained on multiple datasets on the huggingface hub and kaggle :
the focus has been mainly on methodology :
* Chain of thoughts
* step by step planning
* tree of thoughts
* forest of thoughts
* graph of thoughts
* agent generation : Voting, ranking, ... dual agent response generation:
with these methods the model has gained insights into tasks, enabling for knowldge transfer between tasks :
the model has been intensivly trained in recalling data previously entered into the matrix:
The model has also been trained on rich data and markdown outputs as much as possible :
the model can also generate markdown charts with mermaid.
## Training Reginmes:
* Alpaca
* ChatML / OpenAI / MistralAI
* Text Generation
* Question/Answer (Chat)
* Instruction/Input/Response (instruct)
* Mistral Standard Prompt
* Translation Tasks
* Entitys / Topic detection
* Book recall
* Coding challenges, Code Feedback, Code Sumarization, Commenting Code
* Agent Ranking and response anyalisis
* Medical tasks
* PubMed
* Diagnosis
* Psychaitry
* Counselling
* Life Coaching
* Note taking
* Medical smiles
* Medical Reporting
* Virtual laboritys simulations
* Chain of thoughts methods
* One shot / Multi shot prompting tasks | [
"TRANSLATION"
] | Non_BioNLP |
gaudi/opus-mt-fi-ig-ctranslate2 | gaudi | translation | [
"transformers",
"marian",
"ctranslate2",
"translation",
"license:apache-2.0",
"endpoints_compatible",
"region:us"
] | 1,721,663,510,000 | 2024-10-19T03:35:52 | 6 | 0 | ---
license: apache-2.0
tags:
- ctranslate2
- translation
---
# Repository General Information
## Inspired by and derived from the work of [Helsinki-NLP](https://huggingface.co/Helsinki-NLP), [CTranslate2](https://github.com/OpenNMT/CTranslate2), and [michaelfeil](https://huggingface.co/michaelfeil)!
- Link to Original Model ([Helsinki-NLP](https://huggingface.co/Helsinki-NLP)): [Model Link](https://huggingface.co/Helsinki-NLP/opus-mt-fi-ig)
- This respository was based on the work of [CTranslate2](https://github.com/OpenNMT/CTranslate2).
- This repository was based on the work of [michaelfeil](https://huggingface.co/michaelfeil).
# What is CTranslate2?
[CTranslate2](https://opennmt.net/CTranslate2/) is a C++ and Python library for efficient inference with Transformer models.
CTranslate2 implements a custom runtime that applies many performance optimization techniques such as weights quantization, layers fusion, batch reordering, etc., to accelerate and reduce the memory usage of Transformer models on CPU and GPU.
CTranslate2 is one of the most performant ways of hosting translation models at scale. Current supported models include:
- Encoder-decoder models: Transformer base/big, M2M-100, NLLB, BART, mBART, Pegasus, T5, Whisper
- Decoder-only models: GPT-2, GPT-J, GPT-NeoX, OPT, BLOOM, MPT, Llama, Mistral, Gemma, CodeGen, GPTBigCode, Falcon
- Encoder-only models: BERT, DistilBERT, XLM-RoBERTa
The project is production-oriented and comes with backward compatibility guarantees, but it also includes experimental features related to model compression and inference acceleration.
# CTranslate2 Benchmarks
Please note that the results presented below are only valid for the configuration used during this benchmark: absolute and relative performance may change with different settings. Tested against `newstest2014` (En -> De) dataset.
The benchmark reports the number of target tokens generated per second (higher is better). The results are aggregated over multiple runs. See the benchmark scripts for more details and reproduce these numbers.
Please note that the results presented below are only valid for the configuration used during this benchmark: absolute and relative performance may change with different settings.
## CPU Benchmarks for Generic Opus-MT Models
| Library | Tokens per Second | Max Memory Usage | BLEU |
| :----: | :----: | :----: | :----: |
| Transformers 4.26.1 (with PyTorch 1.13.1) | 147.3 | 2332MB | 27.90 |
| Marian 1.11.0 (int16) | 330.2 | 5901MB | 27.65 |
| Marian 1.11.0 (int8) | 355.8 | 4763MB | 27.27 |
| CTranslate2 3.6.0 (int16) | 596.1 | 660MB | 27.53 |
| CTranslate2 3.6.0 (int8) | 696.1 | 516MB | 27.65 |
## GPU Benchmarks for Generic Opus-MT Models
| Library | Tokens per Second | Max GPU Memory Usage | Max Memory Usage | BLEU |
| :----: | :----: | :----: | :----: | :----: |
| Transformers 4.26.1 (with PyTorch 1.13.1) | 1022.9 | 4097MB | 2109MB | 27.90 |
| Marian 1.11.0 (float16) | 3962.4 | 3239MB | 1976MB | 27.94 |
| CTranslate2 3.6.0 (float16) | 9296.7 | 909MB | 814MB | 27.9 |
| CTranslate2 3.6.0 (int8 + float16) | 8362.7 | 813MB | 766MB | 27.9 |
`Executed with 4 threads on a c5.2xlarge Amazon EC2 instance equipped with an Intel(R) Xeon(R) Platinum 8275CL CPU.`
**Source to benchmark information can be found [here](https://github.com/OpenNMT/CTranslate2).**<br />
**Original model BLEU scores can be found [here](https://huggingface.co/Helsinki-NLP/opus-mt-fi-ig).**
## Internal Benchmarks
Internal testing on our end showed **inference times reduced by 6x-10x** on average compared the vanilla checkpoints using the *transformers* library. A **slight reduction on BLEU scores (~5%)** was also identified in comparison to the vanilla checkpoints with a few exceptions. This is likely due to several factors, one being the quantization applied. Further testing is needed from our end to better assess the reduction in translation quality. The command used to compile the vanilla checkpoint into a CTranslate2 model can be found below. Modifying this command can yield differing balances between inferencing performance and translation quality.
# CTranslate2 Installation
```bash
pip install hf-hub-ctranslate2>=1.0.0 ctranslate2>=3.13.0
```
### ct2-transformers-converter Command Used:
```bash
ct2-transformers-converter --model Helsinki-NLP/opus-mt-fi-ig --output_dir ./ctranslate2/opus-mt-fi-ig-ctranslate2 --force --copy_files README.md generation_config.json tokenizer_config.json vocab.json source.spm .gitattributes target.spm --quantization float16
```
# CTranslate2 Converted Checkpoint Information:
**Compatible With:**
- [ctranslate2](https://github.com/OpenNMT/CTranslate2)
- [hf-hub-ctranslate2](https://github.com/michaelfeil/hf-hub-ctranslate2)
**Compute Type:**
- `compute_type=int8_float16` for `device="cuda"`
- `compute_type=int8` for `device="cpu"`
# Sample Code - ctranslate2
#### Clone the repository to the working directory or wherever you wish to store the model artifacts. ####
```bash
git clone https://huggingface.co/gaudi/opus-mt-fi-ig-ctranslate2
```
#### Take the python code below and update the 'model_dir' variable to the location of the cloned repository. ####
```python
from ctranslate2 import Translator
import transformers
model_dir = "./opus-mt-fi-ig-ctranslate2" # Path to model directory.
translator = Translator(
model_path=model_dir,
device="cuda", # cpu, cuda, or auto.
inter_threads=1, # Maximum number of parallel translations.
intra_threads=4, # Number of OpenMP threads per translator.
compute_type="int8_float16", # int8 for cpu or int8_float16 for cuda.
)
tokenizer = transformers.AutoTokenizer.from_pretrained(model_dir)
source = tokenizer.convert_ids_to_tokens(tokenizer.encode("XXXXXX, XXX XX XXXXXX."))
results = translator.translate_batch([source])
target = results[0].hypotheses[0]
print(tokenizer.decode(tokenizer.convert_tokens_to_ids(target)))
```
# Sample Code - hf-hub-ctranslate2
**Derived From [michaelfeil](https://huggingface.co/michaelfeil):**
```python
from hf_hub_ctranslate2 import TranslatorCT2fromHfHub, GeneratorCT2fromHfHub
from transformers import AutoTokenizer
model_name = "gaudi/opus-mt-fi-ig-ctranslate2"
model = TranslatorCT2fromHfHub(
model_name_or_path=model_name,
device="cuda",
compute_type="int8_float16",
tokenizer=AutoTokenizer.from_pretrained(model_name)
)
outputs = model.generate(
text=["XXX XX XXX XXXXXXX XXXX?", "XX XX XXXX XX XXX!"],
)
print(outputs)
```
# License and other remarks:
License conditions are intended to be idential to [original huggingface repository](https://huggingface.co/Helsinki-NLP/opus-mt-fi-ig) by Helsinki-NLP.
| [
"TRANSLATION"
] | Non_BioNLP |
DrishtiSharma/llama-pro-8b-tweet-summarization-gradnorm-0.3-warmupratio-0.05 | DrishtiSharma | null | [
"peft",
"safetensors",
"trl",
"sft",
"generated_from_trainer",
"dataset:dialogstudio",
"base_model:TencentARC/LLaMA-Pro-8B",
"base_model:adapter:TencentARC/LLaMA-Pro-8B",
"license:llama2",
"region:us"
] | 1,706,777,526,000 | 2024-02-01T08:52:56 | 0 | 0 | ---
base_model: TencentARC/LLaMA-Pro-8B
datasets:
- dialogstudio
library_name: peft
license: llama2
tags:
- trl
- sft
- generated_from_trainer
model-index:
- name: llama-pro-8b-tweet-summarization-gradnorm-0.3-warmupratio-0.05
results: []
---
<!-- This model card has been generated automatically according to the information the Trainer had access to. You
should probably proofread and complete it, then remove this comment. -->
# llama-pro-8b-tweet-summarization-gradnorm-0.3-warmupratio-0.05
This model is a fine-tuned version of [TencentARC/LLaMA-Pro-8B](https://huggingface.co/TencentARC/LLaMA-Pro-8B) on the dialogstudio dataset.
## Model description
More information needed
## Intended uses & limitations
More information needed
## Training and evaluation data
More information needed
## Training procedure
### Training hyperparameters
The following hyperparameters were used during training:
- learning_rate: 0.0001
- train_batch_size: 4
- eval_batch_size: 8
- seed: 42
- optimizer: Adam with betas=(0.9,0.999) and epsilon=1e-08
- lr_scheduler_type: cosine
- lr_scheduler_warmup_ratio: 0.05
- num_epochs: 7
- mixed_precision_training: Native AMP
### Framework versions
- PEFT 0.8.2.dev0
- Transformers 4.38.0.dev0
- Pytorch 2.1.0+cu121
- Datasets 2.16.2.dev0
- Tokenizers 0.15.1 | [
"SUMMARIZATION"
] | Non_BioNLP |
yhavinga/byt5-small-ccmatrix-en-nl | yhavinga | translation | [
"transformers",
"pytorch",
"jax",
"tensorboard",
"safetensors",
"t5",
"text2text-generation",
"byt5",
"translation",
"seq2seq",
"nl",
"en",
"multilingual",
"dataset:yhavinga/mc4_nl_cleaned",
"dataset:yhavinga/ccmatrix",
"license:apache-2.0",
"autotrain_compatible",
"text-generation-inference",
"endpoints_compatible",
"region:us"
] | 1,660,993,928,000 | 2023-07-12T08:40:54 | 13 | 0 | ---
datasets:
- yhavinga/mc4_nl_cleaned
- yhavinga/ccmatrix
language:
- nl
- en
- multilingual
license: apache-2.0
pipeline_tag: translation
tags:
- byt5
- translation
- seq2seq
widget:
- text: 'It is a painful and tragic spectacle that rises before me: I have drawn back
the curtain from the rottenness of man. This word, in my mouth, is at least free
from one suspicion: that it involves a moral accusation against humanity.'
- text: Young Wehling was hunched in his chair, his head in his hand. He was so rumpled,
so still and colorless as to be virtually invisible. His camouflage was perfect,
since the waiting room had a disorderly and demoralized air, too. Chairs and ashtrays
had been moved away from the walls. The floor was paved with spattered dropcloths.
---
Logs at https://wandb.ai/yepster/byt5-small-ccmatrix-en-nl/runs/1wm9igj9?workspace=user-yepster
| [
"TRANSLATION"
] | Non_BioNLP |
TheBloke/airoboros-l2-7B-2.2.1-GGUF | TheBloke | null | [
"transformers",
"gguf",
"llama",
"dataset:jondurbin/airoboros-2.2.1",
"base_model:jondurbin/airoboros-l2-7b-2.2.1",
"base_model:quantized:jondurbin/airoboros-l2-7b-2.2.1",
"license:llama2",
"region:us"
] | 1,695,503,041,000 | 2023-09-27T12:54:11 | 411 | 3 | ---
base_model: jondurbin/airoboros-l2-7b-2.2.1
datasets:
- jondurbin/airoboros-2.2.1
license: llama2
model_name: Airoboros L2 7B 2.2.1
inference: false
model_creator: Jon Durbin
model_type: llama
prompt_template: "A chat.\nUSER: {prompt}\nASSISTANT: \n"
quantized_by: TheBloke
---
<!-- header start -->
<!-- 200823 -->
<div style="width: auto; margin-left: auto; margin-right: auto">
<img src="https://i.imgur.com/EBdldam.jpg" alt="TheBlokeAI" style="width: 100%; min-width: 400px; display: block; margin: auto;">
</div>
<div style="display: flex; justify-content: space-between; width: 100%;">
<div style="display: flex; flex-direction: column; align-items: flex-start;">
<p style="margin-top: 0.5em; margin-bottom: 0em;"><a href="https://discord.gg/theblokeai">Chat & support: TheBloke's Discord server</a></p>
</div>
<div style="display: flex; flex-direction: column; align-items: flex-end;">
<p style="margin-top: 0.5em; margin-bottom: 0em;"><a href="https://www.patreon.com/TheBlokeAI">Want to contribute? TheBloke's Patreon page</a></p>
</div>
</div>
<div style="text-align:center; margin-top: 0em; margin-bottom: 0em"><p style="margin-top: 0.25em; margin-bottom: 0em;">TheBloke's LLM work is generously supported by a grant from <a href="https://a16z.com">andreessen horowitz (a16z)</a></p></div>
<hr style="margin-top: 1.0em; margin-bottom: 1.0em;">
<!-- header end -->
# Airoboros L2 7B 2.2.1 - GGUF
- Model creator: [Jon Durbin](https://huggingface.co/jondurbin)
- Original model: [Airoboros L2 7B 2.2.1](https://huggingface.co/jondurbin/airoboros-l2-7b-2.2.1)
<!-- description start -->
## Description
This repo contains GGUF format model files for [Jon Durbin's Airoboros L2 7B 2.2.1](https://huggingface.co/jondurbin/airoboros-l2-7b-2.2.1).
<!-- description end -->
<!-- README_GGUF.md-about-gguf start -->
### About GGUF
GGUF is a new format introduced by the llama.cpp team on August 21st 2023. It is a replacement for GGML, which is no longer supported by llama.cpp.
Here is an incomplate list of clients and libraries that are known to support GGUF:
* [llama.cpp](https://github.com/ggerganov/llama.cpp). The source project for GGUF. Offers a CLI and a server option.
* [text-generation-webui](https://github.com/oobabooga/text-generation-webui), the most widely used web UI, with many features and powerful extensions. Supports GPU acceleration.
* [KoboldCpp](https://github.com/LostRuins/koboldcpp), a fully featured web UI, with GPU accel across all platforms and GPU architectures. Especially good for story telling.
* [LM Studio](https://lmstudio.ai/), an easy-to-use and powerful local GUI for Windows and macOS (Silicon), with GPU acceleration.
* [LoLLMS Web UI](https://github.com/ParisNeo/lollms-webui), a great web UI with many interesting and unique features, including a full model library for easy model selection.
* [Faraday.dev](https://faraday.dev/), an attractive and easy to use character-based chat GUI for Windows and macOS (both Silicon and Intel), with GPU acceleration.
* [ctransformers](https://github.com/marella/ctransformers), a Python library with GPU accel, LangChain support, and OpenAI-compatible AI server.
* [llama-cpp-python](https://github.com/abetlen/llama-cpp-python), a Python library with GPU accel, LangChain support, and OpenAI-compatible API server.
* [candle](https://github.com/huggingface/candle), a Rust ML framework with a focus on performance, including GPU support, and ease of use.
<!-- README_GGUF.md-about-gguf end -->
<!-- repositories-available start -->
## Repositories available
* [AWQ model(s) for GPU inference.](https://huggingface.co/TheBloke/airoboros-l2-7B-2.2.1-AWQ)
* [GPTQ models for GPU inference, with multiple quantisation parameter options.](https://huggingface.co/TheBloke/airoboros-l2-7B-2.2.1-GPTQ)
* [2, 3, 4, 5, 6 and 8-bit GGUF models for CPU+GPU inference](https://huggingface.co/TheBloke/airoboros-l2-7B-2.2.1-GGUF)
* [Jon Durbin's original unquantised fp16 model in pytorch format, for GPU inference and for further conversions](https://huggingface.co/jondurbin/airoboros-l2-7b-2.2.1)
<!-- repositories-available end -->
<!-- prompt-template start -->
## Prompt template: Chat
```
A chat.
USER: {prompt}
ASSISTANT:
```
<!-- prompt-template end -->
<!-- compatibility_gguf start -->
## Compatibility
These quantised GGUFv2 files are compatible with llama.cpp from August 27th onwards, as of commit [d0cee0d](https://github.com/ggerganov/llama.cpp/commit/d0cee0d36d5be95a0d9088b674dbb27354107221)
They are also compatible with many third party UIs and libraries - please see the list at the top of this README.
## Explanation of quantisation methods
<details>
<summary>Click to see details</summary>
The new methods available are:
* GGML_TYPE_Q2_K - "type-1" 2-bit quantization in super-blocks containing 16 blocks, each block having 16 weight. Block scales and mins are quantized with 4 bits. This ends up effectively using 2.5625 bits per weight (bpw)
* GGML_TYPE_Q3_K - "type-0" 3-bit quantization in super-blocks containing 16 blocks, each block having 16 weights. Scales are quantized with 6 bits. This end up using 3.4375 bpw.
* GGML_TYPE_Q4_K - "type-1" 4-bit quantization in super-blocks containing 8 blocks, each block having 32 weights. Scales and mins are quantized with 6 bits. This ends up using 4.5 bpw.
* GGML_TYPE_Q5_K - "type-1" 5-bit quantization. Same super-block structure as GGML_TYPE_Q4_K resulting in 5.5 bpw
* GGML_TYPE_Q6_K - "type-0" 6-bit quantization. Super-blocks with 16 blocks, each block having 16 weights. Scales are quantized with 8 bits. This ends up using 6.5625 bpw
Refer to the Provided Files table below to see what files use which methods, and how.
</details>
<!-- compatibility_gguf end -->
<!-- README_GGUF.md-provided-files start -->
## Provided files
| Name | Quant method | Bits | Size | Max RAM required | Use case |
| ---- | ---- | ---- | ---- | ---- | ----- |
| [airoboros-l2-7b-2.2.1.Q2_K.gguf](https://huggingface.co/TheBloke/airoboros-l2-7B-2.2.1-GGUF/blob/main/airoboros-l2-7b-2.2.1.Q2_K.gguf) | Q2_K | 2 | 2.83 GB| 5.33 GB | smallest, significant quality loss - not recommended for most purposes |
| [airoboros-l2-7b-2.2.1.Q3_K_S.gguf](https://huggingface.co/TheBloke/airoboros-l2-7B-2.2.1-GGUF/blob/main/airoboros-l2-7b-2.2.1.Q3_K_S.gguf) | Q3_K_S | 3 | 2.95 GB| 5.45 GB | very small, high quality loss |
| [airoboros-l2-7b-2.2.1.Q3_K_M.gguf](https://huggingface.co/TheBloke/airoboros-l2-7B-2.2.1-GGUF/blob/main/airoboros-l2-7b-2.2.1.Q3_K_M.gguf) | Q3_K_M | 3 | 3.30 GB| 5.80 GB | very small, high quality loss |
| [airoboros-l2-7b-2.2.1.Q3_K_L.gguf](https://huggingface.co/TheBloke/airoboros-l2-7B-2.2.1-GGUF/blob/main/airoboros-l2-7b-2.2.1.Q3_K_L.gguf) | Q3_K_L | 3 | 3.60 GB| 6.10 GB | small, substantial quality loss |
| [airoboros-l2-7b-2.2.1.Q4_0.gguf](https://huggingface.co/TheBloke/airoboros-l2-7B-2.2.1-GGUF/blob/main/airoboros-l2-7b-2.2.1.Q4_0.gguf) | Q4_0 | 4 | 3.83 GB| 6.33 GB | legacy; small, very high quality loss - prefer using Q3_K_M |
| [airoboros-l2-7b-2.2.1.Q4_K_S.gguf](https://huggingface.co/TheBloke/airoboros-l2-7B-2.2.1-GGUF/blob/main/airoboros-l2-7b-2.2.1.Q4_K_S.gguf) | Q4_K_S | 4 | 3.86 GB| 6.36 GB | small, greater quality loss |
| [airoboros-l2-7b-2.2.1.Q4_K_M.gguf](https://huggingface.co/TheBloke/airoboros-l2-7B-2.2.1-GGUF/blob/main/airoboros-l2-7b-2.2.1.Q4_K_M.gguf) | Q4_K_M | 4 | 4.08 GB| 6.58 GB | medium, balanced quality - recommended |
| [airoboros-l2-7b-2.2.1.Q5_0.gguf](https://huggingface.co/TheBloke/airoboros-l2-7B-2.2.1-GGUF/blob/main/airoboros-l2-7b-2.2.1.Q5_0.gguf) | Q5_0 | 5 | 4.65 GB| 7.15 GB | legacy; medium, balanced quality - prefer using Q4_K_M |
| [airoboros-l2-7b-2.2.1.Q5_K_S.gguf](https://huggingface.co/TheBloke/airoboros-l2-7B-2.2.1-GGUF/blob/main/airoboros-l2-7b-2.2.1.Q5_K_S.gguf) | Q5_K_S | 5 | 4.65 GB| 7.15 GB | large, low quality loss - recommended |
| [airoboros-l2-7b-2.2.1.Q5_K_M.gguf](https://huggingface.co/TheBloke/airoboros-l2-7B-2.2.1-GGUF/blob/main/airoboros-l2-7b-2.2.1.Q5_K_M.gguf) | Q5_K_M | 5 | 4.78 GB| 7.28 GB | large, very low quality loss - recommended |
| [airoboros-l2-7b-2.2.1.Q6_K.gguf](https://huggingface.co/TheBloke/airoboros-l2-7B-2.2.1-GGUF/blob/main/airoboros-l2-7b-2.2.1.Q6_K.gguf) | Q6_K | 6 | 5.53 GB| 8.03 GB | very large, extremely low quality loss |
| [airoboros-l2-7b-2.2.1.Q8_0.gguf](https://huggingface.co/TheBloke/airoboros-l2-7B-2.2.1-GGUF/blob/main/airoboros-l2-7b-2.2.1.Q8_0.gguf) | Q8_0 | 8 | 7.16 GB| 9.66 GB | very large, extremely low quality loss - not recommended |
**Note**: the above RAM figures assume no GPU offloading. If layers are offloaded to the GPU, this will reduce RAM usage and use VRAM instead.
<!-- README_GGUF.md-provided-files end -->
<!-- README_GGUF.md-how-to-download start -->
## How to download GGUF files
**Note for manual downloaders:** You almost never want to clone the entire repo! Multiple different quantisation formats are provided, and most users only want to pick and download a single file.
The following clients/libraries will automatically download models for you, providing a list of available models to choose from:
- LM Studio
- LoLLMS Web UI
- Faraday.dev
### In `text-generation-webui`
Under Download Model, you can enter the model repo: TheBloke/airoboros-l2-7B-2.2.1-GGUF and below it, a specific filename to download, such as: airoboros-l2-7b-2.2.1.Q4_K_M.gguf.
Then click Download.
### On the command line, including multiple files at once
I recommend using the `huggingface-hub` Python library:
```shell
pip3 install huggingface-hub
```
Then you can download any individual model file to the current directory, at high speed, with a command like this:
```shell
huggingface-cli download TheBloke/airoboros-l2-7B-2.2.1-GGUF airoboros-l2-7b-2.2.1.Q4_K_M.gguf --local-dir . --local-dir-use-symlinks False
```
<details>
<summary>More advanced huggingface-cli download usage</summary>
You can also download multiple files at once with a pattern:
```shell
huggingface-cli download TheBloke/airoboros-l2-7B-2.2.1-GGUF --local-dir . --local-dir-use-symlinks False --include='*Q4_K*gguf'
```
For more documentation on downloading with `huggingface-cli`, please see: [HF -> Hub Python Library -> Download files -> Download from the CLI](https://huggingface.co/docs/huggingface_hub/guides/download#download-from-the-cli).
To accelerate downloads on fast connections (1Gbit/s or higher), install `hf_transfer`:
```shell
pip3 install hf_transfer
```
And set environment variable `HF_HUB_ENABLE_HF_TRANSFER` to `1`:
```shell
HF_HUB_ENABLE_HF_TRANSFER=1 huggingface-cli download TheBloke/airoboros-l2-7B-2.2.1-GGUF airoboros-l2-7b-2.2.1.Q4_K_M.gguf --local-dir . --local-dir-use-symlinks False
```
Windows Command Line users: You can set the environment variable by running `set HF_HUB_ENABLE_HF_TRANSFER=1` before the download command.
</details>
<!-- README_GGUF.md-how-to-download end -->
<!-- README_GGUF.md-how-to-run start -->
## Example `llama.cpp` command
Make sure you are using `llama.cpp` from commit [d0cee0d](https://github.com/ggerganov/llama.cpp/commit/d0cee0d36d5be95a0d9088b674dbb27354107221) or later.
```shell
./main -ngl 32 -m airoboros-l2-7b-2.2.1.Q4_K_M.gguf --color -c 4096 --temp 0.7 --repeat_penalty 1.1 -n -1 -p "A chat.\nUSER: {prompt}\nASSISTANT:"
```
Change `-ngl 32` to the number of layers to offload to GPU. Remove it if you don't have GPU acceleration.
Change `-c 4096` to the desired sequence length. For extended sequence models - eg 8K, 16K, 32K - the necessary RoPE scaling parameters are read from the GGUF file and set by llama.cpp automatically.
If you want to have a chat-style conversation, replace the `-p <PROMPT>` argument with `-i -ins`
For other parameters and how to use them, please refer to [the llama.cpp documentation](https://github.com/ggerganov/llama.cpp/blob/master/examples/main/README.md)
## How to run in `text-generation-webui`
Further instructions here: [text-generation-webui/docs/llama.cpp.md](https://github.com/oobabooga/text-generation-webui/blob/main/docs/llama.cpp.md).
## How to run from Python code
You can use GGUF models from Python using the [llama-cpp-python](https://github.com/abetlen/llama-cpp-python) or [ctransformers](https://github.com/marella/ctransformers) libraries.
### How to load this model in Python code, using ctransformers
#### First install the package
Run one of the following commands, according to your system:
```shell
# Base ctransformers with no GPU acceleration
pip install ctransformers
# Or with CUDA GPU acceleration
pip install ctransformers[cuda]
# Or with AMD ROCm GPU acceleration (Linux only)
CT_HIPBLAS=1 pip install ctransformers --no-binary ctransformers
# Or with Metal GPU acceleration for macOS systems only
CT_METAL=1 pip install ctransformers --no-binary ctransformers
```
#### Simple ctransformers example code
```python
from ctransformers import AutoModelForCausalLM
# Set gpu_layers to the number of layers to offload to GPU. Set to 0 if no GPU acceleration is available on your system.
llm = AutoModelForCausalLM.from_pretrained("TheBloke/airoboros-l2-7B-2.2.1-GGUF", model_file="airoboros-l2-7b-2.2.1.Q4_K_M.gguf", model_type="llama", gpu_layers=50)
print(llm("AI is going to"))
```
## How to use with LangChain
Here are guides on using llama-cpp-python and ctransformers with LangChain:
* [LangChain + llama-cpp-python](https://python.langchain.com/docs/integrations/llms/llamacpp)
* [LangChain + ctransformers](https://python.langchain.com/docs/integrations/providers/ctransformers)
<!-- README_GGUF.md-how-to-run end -->
<!-- footer start -->
<!-- 200823 -->
## Discord
For further support, and discussions on these models and AI in general, join us at:
[TheBloke AI's Discord server](https://discord.gg/theblokeai)
## Thanks, and how to contribute
Thanks to the [chirper.ai](https://chirper.ai) team!
Thanks to Clay from [gpus.llm-utils.org](llm-utils)!
I've had a lot of people ask if they can contribute. I enjoy providing models and helping people, and would love to be able to spend even more time doing it, as well as expanding into new projects like fine tuning/training.
If you're able and willing to contribute it will be most gratefully received and will help me to keep providing more models, and to start work on new AI projects.
Donaters will get priority support on any and all AI/LLM/model questions and requests, access to a private Discord room, plus other benefits.
* Patreon: https://patreon.com/TheBlokeAI
* Ko-Fi: https://ko-fi.com/TheBlokeAI
**Special thanks to**: Aemon Algiz.
**Patreon special mentions**: Alicia Loh, Stephen Murray, K, Ajan Kanaga, RoA, Magnesian, Deo Leter, Olakabola, Eugene Pentland, zynix, Deep Realms, Raymond Fosdick, Elijah Stavena, Iucharbius, Erik Bjäreholt, Luis Javier Navarrete Lozano, Nicholas, theTransient, John Detwiler, alfie_i, knownsqashed, Mano Prime, Willem Michiel, Enrico Ros, LangChain4j, OG, Michael Dempsey, Pierre Kircher, Pedro Madruga, James Bentley, Thomas Belote, Luke @flexchar, Leonard Tan, Johann-Peter Hartmann, Illia Dulskyi, Fen Risland, Chadd, S_X, Jeff Scroggin, Ken Nordquist, Sean Connelly, Artur Olbinski, Swaroop Kallakuri, Jack West, Ai Maven, David Ziegler, Russ Johnson, transmissions 11, John Villwock, Alps Aficionado, Clay Pascal, Viktor Bowallius, Subspace Studios, Rainer Wilmers, Trenton Dambrowitz, vamX, Michael Levine, 준교 김, Brandon Frisco, Kalila, Trailburnt, Randy H, Talal Aujan, Nathan Dryer, Vadim, 阿明, ReadyPlayerEmma, Tiffany J. Kim, George Stoitzev, Spencer Kim, Jerry Meng, Gabriel Tamborski, Cory Kujawski, Jeffrey Morgan, Spiking Neurons AB, Edmond Seymore, Alexandros Triantafyllidis, Lone Striker, Cap'n Zoog, Nikolai Manek, danny, ya boyyy, Derek Yates, usrbinkat, Mandus, TL, Nathan LeClaire, subjectnull, Imad Khwaja, webtim, Raven Klaugh, Asp the Wyvern, Gabriel Puliatti, Caitlyn Gatomon, Joseph William Delisle, Jonathan Leane, Luke Pendergrass, SuperWojo, Sebastain Graf, Will Dee, Fred von Graf, Andrey, Dan Guido, Daniel P. Andersen, Nitin Borwankar, Elle, Vitor Caleffi, biorpg, jjj, NimbleBox.ai, Pieter, Matthew Berman, terasurfer, Michael Davis, Alex, Stanislav Ovsiannikov
Thank you to all my generous patrons and donaters!
And thank you again to a16z for their generous grant.
<!-- footer end -->
<!-- original-model-card start -->
# Original model card: Jon Durbin's Airoboros L2 7B 2.2.1
### Overview
Another experimental model, using mostly sythetic data generated by [airoboros](https://github.com/jondurbin/airoboros)
This is essentially a minor "fix" branch of [airoboros-l2-7b-2.2](https://hf.co/jondurbin/airoboros-l2-7b-2.2) with a updates, primarily:
- [re-generated writing responses](https://huggingface.co/datasets/jondurbin/airoboros-2.2.1#re-generated-writing-responses)
- [longer contextual blocks](https://huggingface.co/datasets/jondurbin/airoboros-2.2.1#longer-contextual-blocks)
- [removal of "rp" data](https://huggingface.co/datasets/jondurbin/airoboros-2.2.1#rp-category-removed)
This is a fairly general purpose model, but focuses heavily on instruction following, rather than casual chat/roleplay.
Huge thank you to the folks over at [a16z](https://a16z.com/) for sponsoring the costs associated with building models and associated tools!
### Prompt format
The prompt format:
```
A chat.
USER: {prompt}
ASSISTANT:
```
The default system prompt ("A chat.") was used for most of the prompts, however it also included a wide sampling of responses with other prompts, particularly in "stylized\_response", "rp", "gtkm", etc.
Here's another example:
```
A chat between Bob (aka USER) and Tom (aka ASSISTANT). Tom is an extremely intelligent 18th century bookkeeper, who speaks loquaciously.
USER: {prompt}
ASSISTANT:
```
And chat scenario that wouldn't require USER/ASSISTANT (but should use stopping criteria to prevent the model from speaking on your behalf).
```
A chat between old friends: Timmy and Tommy.
{description of characters}
{setting for the chat}
Timmy: *takes a big sip from his coffee* "Ah, sweet, delicious, magical coffee."
Tommy:
```
__*I strongly suggest adding stopping criteria/early inference stopping on "USER:", and/or whatever names you specify in the system prompt.*__
### Fine tuning info
https://wandb.ai/jondurbin/airoboros-l2-7b-2.2.1/runs/ka6jlcj7?workspace=user-jondurbin
### Helpful usage tips
*The prompts shown here are are just the text that would be included after USER: and before ASSISTANT: in the full prompt format above, the system prompt and USER:/ASSISTANT: have been omited for readability.*
#### Context obedient question answering
By obedient, I mean the model was trained to ignore what it thinks it knows, and uses the context to answer the question. The model was also tuned to limit the values to the provided context as much as possible to reduce hallucinations.
The format for a closed-context prompt is as follows:
```
BEGININPUT
BEGINCONTEXT
[key0: value0]
[key1: value1]
... other metdata ...
ENDCONTEXT
[insert your text blocks here]
ENDINPUT
[add as many other blocks, in the exact same format]
BEGININSTRUCTION
[insert your instruction(s). The model was tuned with single questions, paragraph format, lists, etc.]
ENDINSTRUCTION
```
It's also helpful to add "Don't make up answers if you don't know." to your instruction block to make sure if the context is completely unrelated it doesn't make something up.
*The __only__ prompts that need this closed context formating are closed-context instructions. Normal questions/instructions do not!*
I know it's a bit verbose and annoying, but after much trial and error, using these explicit delimiters helps the model understand where to find the responses and how to associate specific sources with it.
- `BEGININPUT` - denotes a new input block
- `BEGINCONTEXT` - denotes the block of context (metadata key/value pairs) to associate with the current input block
- `ENDCONTEXT` - denotes the end of the metadata block for the current input
- [text] - Insert whatever text you want for the input block, as many paragraphs as can fit in the context.
- `ENDINPUT` - denotes the end of the current input block
- [repeat as many input blocks in this format as you want]
- `BEGININSTRUCTION` - denotes the start of the list (or one) instruction(s) to respond to for all of the input blocks above.
- [instruction(s)]
- `ENDINSTRUCTION` - denotes the end of instruction set
It sometimes works without `ENDINSTRUCTION`, but by explicitly including that in the prompt, the model better understands that all of the instructions in the block should be responded to.
Here's a trivial, but important example to prove the point:
```
BEGININPUT
BEGINCONTEXT
date: 2021-01-01
url: https://web.site/123
ENDCONTEXT
In a shocking turn of events, blueberries are now green, but will be sticking with the same name.
ENDINPUT
BEGININSTRUCTION
What color are bluberries? Source?
ENDINSTRUCTION
```
And the response:
```
Blueberries are now green.
Source:
date: 2021-01-01
url: https://web.site/123
```
#### Summarization
500 samples have been included from [this dataset](https://huggingface.co/datasets/mattpscott/airoboros-summarization), using the same format as contextual question answering, for example:
```
BEGININPUT
{text to summarize}
ENDINPUT
BEGININSTRUCTION
Summarize the input in around 130 words.
ENDINSTRUCTION
```
#### Getting longer responses
You can use a few techniques to get longer responses.
Detailed prompts, with explicit instruction for word count:
```
Please compose a narrative set in the heart of an ancient library, steeped in the scent of old parchment and ink. The protagonist should be a young scholar who is dedicated to studying the art of storytelling and its evolution throughout history. In her pursuit of knowledge, she stumbles upon a forgotten tome that seems to possess an unusual aura. This book has the ability to bring stories to life, literally manifesting characters and scenarios from within its pages into reality.
The main character must navigate through various epochs of storytelling - from oral traditions of tribal societies, through medieval minstrels' tales, to modern-day digital narratives - as they come alive around her. Each era presents its unique challenges and lessons about the power and impact of stories on human civilization.
One such character could be a sentient quill pen, who was once used by renowned authors of yesteryears and now holds their wisdom and experiences. It becomes her mentor, guiding her through this journey with witty remarks and insightful commentary.
Ensure that your tale encapsulates the thrill of adventure, the beauty of learning, and the profound connection between humans and their stories. All characters involved should be non-human entities. Feel free to explore creative liberties but maintain the mentioned elements.
Your response should be approximately 2300 words.
```
Or, a simpler example:
```
Please create a long, detailed story about a dragon in an old growth forest who, for some reason, begins speaking the words of the source code of linux.
```
#### Coding
You can ask for fairly complex coding instructions with multiple criteria, e.g.:
```
Create a python application with the following requirements:
- Asyncio FastAPI webserver
- ping endpoint that returns the current date in JSON format
- file upload endpoint, which calculates the file's sha256 checksum, and checks postgres to deduplicate
```
Or inline criteria:
```
Write a multi-threaded TCP server in C that accepts a "GET [key]" input and "SET [key] [value]" input, and uses a binary tree to get and store the input values.
```
You can also optionally add a single space and "PLAINFORMAT" at the end of your prompt to avoid backticks, explanations, etc. and just print the code, e.g.:
```
Write a websocket application in node.js. PLAINFORMAT
```
#### Agent/function calling
The dataset includes many examples of function/args generation based on input criteria. This is somewhat similar to the OpenAI function calling, but the output is either JSON or YAML.
Example prompt:
```
As an AI assistant, please select the most suitable function and parameters from the list of available functions below, based on the user's input. Provide your response in JSON format.
Input: I want to know how many times 'Python' is mentioned in my text file.
Available functions:
file_analytics:
description: This tool performs various operations on a text file.
params:
action: The operation we want to perform on the data, such as "count_occurrences", "find_line", etc.
filters:
keyword: The word or phrase we want to search for.
```
Response:
```json
{
"function": "file_analytics",
"params": {
"action": "count_occurrences",
"filters": {
"keyword": "Python"
}
}
}
```
#### Chain-of-thought
You can ask for several possible responses to a given problem, with a ranking and final answer selection.
Example prompt:
```
A group of people decide to play a round-robin tournament where every player plays every other player exactly once. If a total of 45 games were played, how many players participated in the tournament? Offer several potential answers, rank them according to mathematical logic, and pick the most feasible one.
```
Example response:
```
Solution 1:
In a round-robin tournament, each player plays every other player exactly once. This means that the total number of games played is equal to the combination of all players taken two at a time (since a game involves two players).
The formula for combinations is nC2 = n(n-1)/2, where n is the total number of players. We know that nC2 equals 45 (the total number of games), so we can set up the equation as follows:
n(n-1)/2 = 45
Solving this equation gives us n=10.
Final answer: There were 10 players in the tournament.
Solution 2:
Another way to approach this problem is by using the concept of triangular numbers. Triangular numbers are a sequence of numbers where each number can be represented as a triangle with dots. The nth triangular number is given by the sum of the first n natural numbers.
If we consider each game as a dot and each player as a side of the triangle, then the total number of games (45) would correspond to the 9th triangular number because 1+2+3+4+5+6+7+8+9=45. However, since each side of the triangle represents a player, and there's one more player than sides in our model (because the last player has no one left to play against), we need to add one more to get the total number of players.
Final answer: There were 10 players in the tournament.
Solution 3:
We could also use trial and error to solve this problem. Starting from a small number of players, we calculate how many games would be played in a round-robin tournament until we reach 45 games. For example, with 2 players, only 1 game is played; with 3 players, 3 games are played; with 4 players, 6 games are played, and so on. Continuing this process, we find that with 10 players, 45 games are played.
Final answer: There were 10 players in the tournament.
Ranking of solutions from best to worst:
1. Solution 1: This solution uses direct mathematical reasoning based on the properties of a round-robin tournament and solves an equation to find the answer.
2. Solution 2: This solution uses the concept of triangular numbers which requires additional steps and knowledge beyond the immediate problem context, but still arrives at the correct answer.
3. Solution 3: This solution relies on trial and error, which is less efficient and reliable than the other methods, but it eventually gets to the right answer.
Best and final answer: There were 10 players in the tournament.
```
#### reWOO style execution planning
The model now supports execution planning for complex instructions that would require making use of several tools. The output is just the plan, you must implement a mechanism to parse the output and actually call the functions!
Example prompt:
```
Please construct a systematic plan to generate an optimal response to the user instruction, utilizing a set of provided tools. Each plan will correspond to an evidence value, which will be the output of one of the available functions given an input string
that could be the user's question, one or more prior evidence values, or a combination of both.
Here are the tools available to be called:
DuckDuckGo[input]: Tool that enables the user to search for information using the DuckDuckGo search engine. This tool is helpful in locating a suitable list of sites that may contain the answer to the user's query. It does not directly answer the question but finds a list of sites that might have the answer.
TextScraper[input]: Load one or more websites from the input string containing newline delimited links, where input is one or more links, and produces plain text output containing the content of the links.
HyperlinkExtractor[input]: Extract links from plain text and produces a plain text, newline delimited response of links.
KnowledgeModel[input]: Question answering language model, particularly useful in answering questions based on an input passage of text. The input must be a text question that references an :evidence[n]: variable, e.g. What color is the cat, given :evidence1:?
The output should be in format:
Plan: [first action to take based on input question]
:evidence0: = [function to call with input parameter]
Plan: [next action to take, based on result of :evidence0:]
:evidence1: = [next function to call and input parameter, which may include reference to previous evidence, e.g. "Given context :evidence0"]
...
Answer: [:evidence[n]: containing the final answer.]
Question: Who won the Nobel Prize in Literature in 2020 and what is their most famous work?
```
Response:
```
Plan: Begin by conducting a web search to find out who won the Nobel Prize in Literature in 2020.
:evidence0: = DuckDuckGo[Nobel Prize in Literature 2020 winner]
Plan: Utilize the KnowledgeModel to interpret the search results and find the name of the laureate.
:evidence1: = KnowledgeModel[Who won the Nobel Prize in Literature in 2020, given :evidence0:?]
Plan: Conduct another web search to find the most famous work of the identified laureate.
:evidence2: = DuckDuckGo[Most famous work of :evidence1:]
Plan: Extract the relevant links from the DuckDuckGo search results for a more focused search.
:evidence3: = HyperlinkExtractor[:evidence2:]
Plan: Use the TextScraper tool to extract information from the relevant links.
:evidence4: = TextScraper[:evidence3:]
Plan: Finally, utilize the KnowledgeModel to identify and summarize the most famous work of the laureate from the extracted information.
:evidence5: = KnowledgeModel[What is the most famous work of :evidence1:, given :evidence4:?]
Answer: :evidence5:
```
For this to be useful, you'd have to parse the output plan text, and implement/call each of the functions. This is just pseudo-code, completely untested off the top of my head, and obviously would requiring full implementation + hardening:
```python
import re
import requests
def inject_context(input_text, **context):
for ref in set(re.findall(r"(:evidence[0-9]+:)", input_text, re.I)):
input_text = input_text.replace(ref, context.get(ref, ""))
return input_text
def duckduckgo(input_text, **context):
search_string = inject_context(input_text, **context)
... search via duck duck go using search_string
... return text content
def link_extractor(input_text, **context):
input_text = inject_context(input_text, **context)
return "\n".join(list(set(re.findall(r"(https?://[^\s]+?\.?)", input_text, re.I))))
def scrape(input_text, **context):
input_text = inject_context(input_text, **context)
text = []
for link in input_text.splitlines():
text.append(requests.get(link).text)
return "\n".join(text)
def infer(input_text, **context)
prompt = inject_context(input_text, **context)
... call model with prompt, return output
def parse_plan(plan):
method_map = {
"DuckDuckGo": duckduckgo,
"HyperlinkExtractor": link_extractor,
"KnowledgeModel": infer,
"TextScraper": scrape,
}
context = {}
for line in plan.strip().splitlines():
if line.startswith("Plan:"):
print(line)
continue
parts = re.match("^(:evidence[0-9]+:)\s*=\s*([^\[]+])(\[.*\])\s$", line, re.I)
if not parts:
if line.startswith("Answer: "):
return context.get(line.split(" ")[-1].strip(), "Answer couldn't be generated...")
raise RuntimeError("bad format: " + line)
context[parts.group(1)] = method_map[parts.group(2)](parts.group(3), **context)
```
### Contribute
If you're interested in new functionality, particularly a new "instructor" type to generate a specific type of training data,
take a look at the dataset generation tool repo: https://github.com/jondurbin/airoboros and either make a PR or open an issue with details.
To help me with the OpenAI/compute costs:
- https://bmc.link/jondurbin
- ETH 0xce914eAFC2fe52FdceE59565Dd92c06f776fcb11
- BTC bc1qdwuth4vlg8x37ggntlxu5cjfwgmdy5zaa7pswf
### Licence and usage restrictions
The airoboros 2.2 models are built on top of llama-2/codellama.
The llama-2 base model has a custom Meta license:
- See the [meta-license/LICENSE.txt](meta-license/LICENSE.txt) file attached for the original license provided by Meta.
- See also [meta-license/USE_POLICY.md](meta-license/USE_POLICY.md) and [meta-license/Responsible-Use-Guide.pdf](meta-license/Responsible-Use-Guide.pdf), also provided by Meta.
The fine-tuning data was mostly generated by OpenAI API calls to gpt-4, via [airoboros](https://github.com/jondurbin/airoboros)
The ToS for OpenAI API usage has a clause preventing the output from being used to train a model that __competes__ with OpenAI
- what does *compete* actually mean here?
- these small open source models will not produce output anywhere near the quality of gpt-4, or even gpt-3.5, so I can't imagine this could credibly be considered competing in the first place
- if someone else uses the dataset to do the same, they wouldn't necessarily be violating the ToS because they didn't call the API, so I don't know how that works
- the training data used in essentially all large language models includes a significant amount of copyrighted or otherwise non-permissive licensing in the first place
- other work using the self-instruct method, e.g. the original here: https://github.com/yizhongw/self-instruct released the data and model as apache-2
I am purposingly leaving this license ambiguous (other than the fact you must comply with the Meta original license for llama-2) because I am not a lawyer and refuse to attempt to interpret all of the terms accordingly.
Your best bet is probably to avoid using this commercially due to the OpenAI API usage.
Either way, by using this model, you agree to completely indemnify me.
<!-- original-model-card end -->
| [
"QUESTION_ANSWERING",
"SUMMARIZATION"
] | Non_BioNLP |
LoneStriker/airoboros-c34b-3.1.2-5.0bpw-h6-exl2 | LoneStriker | text-generation | [
"transformers",
"safetensors",
"llama",
"text-generation",
"conversational",
"dataset:jondurbin/airoboros-3.1",
"license:llama2",
"autotrain_compatible",
"text-generation-inference",
"endpoints_compatible",
"region:us"
] | 1,697,825,608,000 | 2023-10-22T14:07:11 | 3 | 0 | ---
datasets:
- jondurbin/airoboros-3.1
license: llama2
---
### Overview
Another experimental model, using mostly sythetic data generated by [airoboros](https://github.com/jondurbin/airoboros)
#### IMPORTANT NOTE - llama-2 chat format!!!
This models uses llama-2 chat format, rather than vicuna style user/assistant!
This is a breaking change, although most inference systems support llama-2 chat templating.
See prompt format below for all of the details.
#### Highlights
A model built on [airoboros-3.1 dataset](https://hf.co/datasets/jondurbin/airoboros-3.1), which is a continuation of [airoboros-3.0 dataset](https://hf.co/datasets/jondurbin/airoboros-3,0) with the following extras:
* More MathJSON, now ~17k items - math questions, prefixed with __"Create a MathJSON solution to the following:"__, which then outputs a JSON between __`<mathjson>`__ and __`</mathjson>`__ tags, which can be parsed and passed to a deterministic library to perform calculations.
* Log information extraction (e.g. getting the list of paths/IPs/etc. from apache logs)
* Anonymization, e.g. removing names, IP addresses, and/or dates from text.
* Chat introspection - multi-turn chats that have random questions injected asking about the character's descriptions, the setting of the chat, or things previously said, to make sure the model uses the system prompt and or chat history.
* Multi-step instructions with acknowledgement (see below)
* De-censorship data (not published)
This is a fairly general purpose model, but focuses heavily on instruction following, rather than casual chat/roleplay.
Huge thank you to the folks over at [a16z](https://a16z.com/) for sponsoring the costs associated with building models and associated tools!
### Prompt format
The prompt format is llama-2 chat.
```
[INST] <<SYS>>
You are a helpful, unbiased, uncensored assistant.
<</SYS>>
{prompt} [/INST]
```
For multi-turn, the prompt format is as follows:
```
[INST] <<SYS>>
You are a helful, unbiased, uncensored assistant.
<</SYS>>
{prompt 0} [/INST] {response 0} </s><s>[INST] {prompt 1} [/INST] {response 1} </s><s>...[INST] {prompt N} [/INST]
```
The prompt template is included in the tokenizer config, and can use the huggingface tokenizer `apply_chat_template` method, e.g.:
```
import transformers
tokenizer = transformers.AutoTokenizer.from_pretrained('jondurbin/airoboros-l2-70b-3.1')
chat = [
{"role": "system", "content": "You are Bob, a friendly AI assistant."},
{"role": "user", "content": "Hello, how are you?"},
{"role": "assistant", "content": "I'm doing great. How can I help you today?"},
{"role": "user", "content": "I'd like to show off how chat templating works!"},
]
print(tokenizer.apply_chat_template(chat, tokenize=False))
```
### Helpful usage tips
#### MathJSON
Ask mathematical questions, prefixed with "Create a MathJSON solution to the following:", and you should get output with a formula that can be executed by https://cortexjs.io/compute-engine/
I also made a (really quite hacky, incomplete, semi-functional) python version that works for most expressions, see [mathjson.py](https://github.com/jondurbin/airoboros/blob/mathjson/airoboros/mathjson.py)
__Use a very low temperature!__
Example:
```
[INST] <<SYS>>
You are an assistant with vast knowledge in all things math.
<</SYS>>
Create a MathJSON solution to the following: Calculate the area of a circle with radius of 17.2456cm. Include your reasoning. [/INST]
```
Output:
```
The area (A) of a circle is given by the formula A = πr², where r is the radius of the circle. Since the radius is given in centimeters, we need to convert it to meters first. We know that 1 meter equals 100 centimeters. Therefore, we can represent the radius as: r = 17.2456 cm * 100/1.
Once we have the radius in meters, we can calculate the area of the circle using the formula A = πr².
Solution as MathJSON:
<mathjson>
[
"Multiply",
"Pi",
[
"Power",
17.2456,
2
]
]
</mathjson>
```
You can then validate the JSON between `<mathjson>` and `</mathjson>`, then pass the parsed JSON to compute-engine JS or the `evaluate` function in mathjson.py to calculate the response.
#### Context obedient question answering
By obedient, I mean the model was trained to ignore what it thinks it knows, and uses the context to answer the question. The model was also tuned to limit the values to the provided context as much as possible to reduce hallucinations.
The format for a closed-context prompt is as follows:
```
BEGININPUT
BEGINCONTEXT
[key0: value0]
[key1: value1]
... other metdata ...
ENDCONTEXT
[insert your text blocks here]
ENDINPUT
[add as many other blocks, in the exact same format]
BEGININSTRUCTION
[insert your instruction(s). The model was tuned with single questions, paragraph format, lists, etc.]
ENDINSTRUCTION
```
It's also helpful to add "Don't make up answers if you don't know." to your instruction block to make sure if the context is completely unrelated it doesn't make something up.
*The __only__ prompts that need this closed context formating are closed-context instructions. Normal questions/instructions do not!*
I know it's a bit verbose and annoying, but after much trial and error, using these explicit delimiters helps the model understand where to find the responses and how to associate specific sources with it.
- `BEGININPUT` - denotes a new input block
- `BEGINCONTEXT` - denotes the block of context (metadata key/value pairs) to associate with the current input block
- `ENDCONTEXT` - denotes the end of the metadata block for the current input
- [text] - Insert whatever text you want for the input block, as many paragraphs as can fit in the context.
- `ENDINPUT` - denotes the end of the current input block
- [repeat as many input blocks in this format as you want]
- `BEGININSTRUCTION` - denotes the start of the list (or one) instruction(s) to respond to for all of the input blocks above.
- [instruction(s)]
- `ENDINSTRUCTION` - denotes the end of instruction set
It sometimes works without `ENDINSTRUCTION`, but by explicitly including that in the prompt, the model better understands that all of the instructions in the block should be responded to.
__Use a very low temperature!__
Here's a trivial, but important example to prove the point:
```
BEGININPUT
BEGINCONTEXT
date: 2021-01-01
url: https://web.site/123
ENDCONTEXT
In a shocking turn of events, blueberries are now green, but will be sticking with the same name.
ENDINPUT
BEGININSTRUCTION
What color are bluberries? Source?
ENDINSTRUCTION
```
And the response:
```
Blueberries are now green.
Source:
date: 2021-01-01
url: https://web.site/123
```
#### Summarization
500 samples have been included from [this dataset](https://huggingface.co/datasets/mattpscott/airoboros-summarization), using the same format as contextual question answering, for example:
```
BEGININPUT
{text to summarize}
ENDINPUT
BEGININSTRUCTION
Summarize the input in around 130 words.
ENDINSTRUCTION
```
#### Getting longer responses
You can use a few techniques to get longer responses.
Detailed prompts, with explicit instruction for word count:
```
Please compose a narrative set in the heart of an ancient library, steeped in the scent of old parchment and ink. The protagonist should be a young scholar who is dedicated to studying the art of storytelling and its evolution throughout history. In her pursuit of knowledge, she stumbles upon a forgotten tome that seems to possess an unusual aura. This book has the ability to bring stories to life, literally manifesting characters and scenarios from within its pages into reality.
The main character must navigate through various epochs of storytelling - from oral traditions of tribal societies, through medieval minstrels' tales, to modern-day digital narratives - as they come alive around her. Each era presents its unique challenges and lessons about the power and impact of stories on human civilization.
One such character could be a sentient quill pen, who was once used by renowned authors of yesteryears and now holds their wisdom and experiences. It becomes her mentor, guiding her through this journey with witty remarks and insightful commentary.
Ensure that your tale encapsulates the thrill of adventure, the beauty of learning, and the profound connection between humans and their stories. All characters involved should be non-human entities. Feel free to explore creative liberties but maintain the mentioned elements.
Your response should be approximately 2300 words.
```
Or, a simpler example:
```
Please create a long, detailed story about a dragon in an old growth forest who, for some reason, begins speaking the words of the source code of linux.
```
There are a few examples of next chapter completion as well, e.g.:
```
Write the next chapter of a historical fiction novel set in Paris during the 20th century.
Here's a summary of the previous chapter:
In the vibrant city of Paris, amid the tumultuous changes of the 20th century, our protagonist Margot, an aspiring fashion designer, has just secured an apprenticeship at a prestigious couture house. She meets Lucien, a charming journalist who covers the fashion industry. Together they navigate the ever-changing world of fashion and society, uncovering secrets that reveal the intricate links between style, politics, and culture. As the chapter concludes, they decide to delve deeper into the hidden corners of the fashion world to unravel its mysteries.
Requirements for the next chapter:
1. Character Development of Margot and Lucien:
- Margot's Evolution: Unfold more about Margot's past, her dreams of revolutionizing fashion, and her struggle to establish herself in a male-dominated industry. Illustrate her growing expertise, innovative ideas, and increasing dependence on Lucien.
- Lucien's Complexity: Introduce uncertainties surrounding Lucien's background and real motives. Increase suspense by suggesting undisclosed information he possesses, while also highlighting his wit and perceptiveness.
2. Exploration of Paris and the Couture House:
- Paris: Elaborate their journey through the bustling streets of Paris, including encounters with iconic figures, social unrest, and relics from different eras of French history.
- The Couture House: Expand on the grandeur of the couture house they work in, filled with artistic masterpieces, intense competition, and cryptic notes hinting at a scandalous past.
3. Emergence of the Subplot: The Lost Collection:
- Discovery: Have Margot and Lucien stumble upon a secret vault containing a lost collection designed before World War II, raising new questions about the previous owner and the influence of war on fashion.
- Revelation: Capture their shock as they realize the designs were plagiarized, the potential repercussions, and the opportunities it presents for Margot's career.
- Twist: End with a twist that suggests there are other stolen collections across Paris, setting up their new mission.
Your response should be approximately 650 words.
```
#### Coding
You can ask for fairly complex coding instructions with multiple criteria, e.g.:
```
Create a python application with the following requirements:
- Asyncio FastAPI webserver
- ping endpoint that returns the current date in JSON format
- file upload endpoint, which calculates the file's sha256 checksum, and checks postgres to deduplicate
```
Or inline criteria:
```
Write a multi-threaded TCP server in C that accepts a "GET [key]" input and "SET [key] [value]" input, and uses a binary tree to get and store the input values.
```
You can also optionally add a single space and "PLAINFORMAT" at the end of your prompt to avoid backticks, explanations, etc. and just print the code, e.g.:
```
Write a websocket application in node.js. PLAINFORMAT
```
#### Agent/function calling
The dataset includes many examples of function/args generation based on input criteria. This is somewhat similar to the OpenAI function calling, but the output is either JSON or YAML.
Example prompt:
```
As an AI assistant, please select the most suitable function and parameters from the list of available functions below, based on the user's input. Provide your response in JSON format.
Input: I want to know how many times 'Python' is mentioned in my text file.
Available functions:
file_analytics:
description: This tool performs various operations on a text file.
params:
action: The operation we want to perform on the data, such as "count_occurrences", "find_line", etc.
filters:
keyword: The word or phrase we want to search for.
```
Response:
```json
{
"function": "file_analytics",
"params": {
"action": "count_occurrences",
"filters": {
"keyword": "Python"
}
}
}
```
#### Chain-of-thought
You can ask for several possible responses to a given problem, with a ranking and final answer selection.
Example prompt:
```
A group of people decide to play a round-robin tournament where every player plays every other player exactly once. If a total of 45 games were played, how many players participated in the tournament? Offer several potential answers, rank them according to mathematical logic, and pick the most feasible one.
```
Example response:
```
Solution 1:
In a round-robin tournament, each player plays every other player exactly once. This means that the total number of games played is equal to the combination of all players taken two at a time (since a game involves two players).
The formula for combinations is nC2 = n(n-1)/2, where n is the total number of players. We know that nC2 equals 45 (the total number of games), so we can set up the equation as follows:
n(n-1)/2 = 45
Solving this equation gives us n=10.
Final answer: There were 10 players in the tournament.
Solution 2:
Another way to approach this problem is by using the concept of triangular numbers. Triangular numbers are a sequence of numbers where each number can be represented as a triangle with dots. The nth triangular number is given by the sum of the first n natural numbers.
If we consider each game as a dot and each player as a side of the triangle, then the total number of games (45) would correspond to the 9th triangular number because 1+2+3+4+5+6+7+8+9=45. However, since each side of the triangle represents a player, and there's one more player than sides in our model (because the last player has no one left to play against), we need to add one more to get the total number of players.
Final answer: There were 10 players in the tournament.
Solution 3:
We could also use trial and error to solve this problem. Starting from a small number of players, we calculate how many games would be played in a round-robin tournament until we reach 45 games. For example, with 2 players, only 1 game is played; with 3 players, 3 games are played; with 4 players, 6 games are played, and so on. Continuing this process, we find that with 10 players, 45 games are played.
Final answer: There were 10 players in the tournament.
Ranking of solutions from best to worst:
1. Solution 1: This solution uses direct mathematical reasoning based on the properties of a round-robin tournament and solves an equation to find the answer.
2. Solution 2: This solution uses the concept of triangular numbers which requires additional steps and knowledge beyond the immediate problem context, but still arrives at the correct answer.
3. Solution 3: This solution relies on trial and error, which is less efficient and reliable than the other methods, but it eventually gets to the right answer.
Best and final answer: There were 10 players in the tournament.
```
#### reWOO style execution planning
The model now supports execution planning for complex instructions that would require making use of several tools. The output is just the plan, you must implement a mechanism to parse the output and actually call the functions!
Example prompt:
```
Please construct a systematic plan to generate an optimal response to the user instruction, utilizing a set of provided tools. Each plan will correspond to an evidence value, which will be the output of one of the available functions given an input string
that could be the user's question, one or more prior evidence values, or a combination of both.
Here are the tools available to be called:
DuckDuckGo[input]: Tool that enables the user to search for information using the DuckDuckGo search engine. This tool is helpful in locating a suitable list of sites that may contain the answer to the user's query. It does not directly answer the question but finds a list of sites that might have the answer.
TextScraper[input]: Load one or more websites from the input string containing newline delimited links, where input is one or more links, and produces plain text output containing the content of the links.
HyperlinkExtractor[input]: Extract links from plain text and produces a plain text, newline delimited response of links.
KnowledgeModel[input]: Question answering language model, particularly useful in answering questions based on an input passage of text. The input must be a text question that references an :evidence[n]: variable, e.g. What color is the cat, given :evidence1:?
The output should be in format:
Plan: [first action to take based on input question]
:evidence0: = [function to call with input parameter]
Plan: [next action to take, based on result of :evidence0:]
:evidence1: = [next function to call and input parameter, which may include reference to previous evidence, e.g. "Given context :evidence0"]
...
Answer: [:evidence[n]: containing the final answer.]
Question: Who won the Nobel Prize in Literature in 2020 and what is their most famous work?
```
Response:
```
Plan: Begin by conducting a web search to find out who won the Nobel Prize in Literature in 2020.
:evidence0: = DuckDuckGo[Nobel Prize in Literature 2020 winner]
Plan: Utilize the KnowledgeModel to interpret the search results and find the name of the laureate.
:evidence1: = KnowledgeModel[Who won the Nobel Prize in Literature in 2020, given :evidence0:?]
Plan: Conduct another web search to find the most famous work of the identified laureate.
:evidence2: = DuckDuckGo[Most famous work of :evidence1:]
Plan: Extract the relevant links from the DuckDuckGo search results for a more focused search.
:evidence3: = HyperlinkExtractor[:evidence2:]
Plan: Use the TextScraper tool to extract information from the relevant links.
:evidence4: = TextScraper[:evidence3:]
Plan: Finally, utilize the KnowledgeModel to identify and summarize the most famous work of the laureate from the extracted information.
:evidence5: = KnowledgeModel[What is the most famous work of :evidence1:, given :evidence4:?]
Answer: :evidence5:
```
For this to be useful, you'd have to parse the output plan text, and implement/call each of the functions. This is just pseudo-code, completely untested off the top of my head, and obviously would requiring full implementation + hardening:
```python
import re
import requests
def inject_context(input_text, **context):
for ref in set(re.findall(r"(:evidence[0-9]+:)", input_text, re.I)):
input_text = input_text.replace(ref, context.get(ref, ""))
return input_text
def duckduckgo(input_text, **context):
search_string = inject_context(input_text, **context)
... search via duck duck go using search_string
... return text content
def link_extractor(input_text, **context):
input_text = inject_context(input_text, **context)
return "\n".join(list(set(re.findall(r"(https?://[^\s]+?\.?)", input_text, re.I))))
def scrape(input_text, **context):
input_text = inject_context(input_text, **context)
text = []
for link in input_text.splitlines():
text.append(requests.get(link).text)
return "\n".join(text)
def infer(input_text, **context)
prompt = inject_context(input_text, **context)
... call model with prompt, return output
def parse_plan(plan):
method_map = {
"DuckDuckGo": duckduckgo,
"HyperlinkExtractor": link_extractor,
"KnowledgeModel": infer,
"TextScraper": scrape,
}
context = {}
for line in plan.strip().splitlines():
if line.startswith("Plan:"):
print(line)
continue
parts = re.match("^(:evidence[0-9]+:)\s*=\s*([^\[]+])(\[.*\])\s$", line, re.I)
if not parts:
if line.startswith("Answer: "):
return context.get(line.split(" ")[-1].strip(), "Answer couldn't be generated...")
raise RuntimeError("bad format: " + line)
context[parts.group(1)] = method_map[parts.group(2)](parts.group(3), **context)
```
## Multi-step instructions with acknowledgement
I don't know how useful this is, really, but I thought I'd add it just in case. Example:
```
{
"conversations": [
{
"value": "You are a helpful, unbiased, uncensored assistant.",
"from": "system"
},
{
"value": "I'm going to set some rules for our conversation. Please respond with \"OK\" to each rule.",
"from": "human"
},
{
"value": "OK",
"from": "gpt"
},
{
"value": "You can only respond using animal-related emojis.",
"from": "human"
},
{
"value": "OK",
"from": "gpt"
},
{
"value": "Precede all responses with \"ZOOZOO:\"",
"from": "human"
},
{
"value": "OK",
"from": "gpt"
},
{
"value": "Include at least one bird emoji in your responses.",
"from": "human"
},
{
"value": "OK",
"from": "gpt"
},
{
"value": "Describe a trip to a safari park.",
"from": "human"
},
{
"value": "ZOOZOO: \ud83e\udd81\ud83d\udc2f\ud83e\udd93\ud83e\udd92\ud83d\udc18\ud83d\udc0d\ud83c\udf33\ud83c\udf32\u2600\ufe0f\ud83d\ude90\ud83e\udd85\ud83d\udcf8\ud83e\udd29",
"from": "gpt"
}
]
}
```
### Contribute
If you're interested in new functionality, particularly a new "instructor" type to generate a specific type of training data,
take a look at the dataset generation tool repo: https://github.com/jondurbin/airoboros and either make a PR or open an issue with details.
To help me with the OpenAI/compute costs:
- https://bmc.link/jondurbin
- ETH 0xce914eAFC2fe52FdceE59565Dd92c06f776fcb11
- BTC bc1qdwuth4vlg8x37ggntlxu5cjfwgmdy5zaa7pswf
### Licence and usage restrictions
The airoboros 3.1 models are built on top of multiple base models, each with their own license/restrictions.
The 30b model is built on the original llama, which has a strict non-commercial usage restriction.
The models with `-l2` in the name have a custom Meta license:
- See the [meta-license/LICENSE.txt](meta-license/LICENSE.txt) file attached for the original license provided by Meta.
- See also [meta-license/USE_POLICY.md](meta-license/USE_POLICY.md) and [meta-license/Responsible-Use-Guide.pdf](meta-license/Responsible-Use-Guide.pdf), also provided by Meta.
The models with `-m-` are mistral-7b (apache 2.0)
The fine-tuning data was mostly generated by OpenAI API calls to gpt-4, via [airoboros](https://github.com/jondurbin/airoboros)
The ToS for OpenAI API usage has a clause preventing the output from being used to train a model that __competes__ with OpenAI
- what does *compete* actually mean here?
- these small open source models will not produce output anywhere near the quality of gpt-4, or even gpt-3.5, so I can't imagine this could credibly be considered competing in the first place
- if someone else uses the dataset to do the same, they wouldn't necessarily be violating the ToS because they didn't call the API, so I don't know how that works
- the training data used in essentially all large language models includes a significant amount of copyrighted or otherwise non-permissive licensing in the first place
- other work using the self-instruct method, e.g. the original here: https://github.com/yizhongw/self-instruct released the data and model as apache-2
I am purposingly leaving this license ambiguous (other than the fact you must comply with the Meta original license for llama-2) because I am not a lawyer and refuse to attempt to interpret all of the terms accordingly.
Your best bet is probably to avoid using this commercially due to the OpenAI API usage.
Either way, by using this model, you agree to completely indemnify me.
| [
"QUESTION_ANSWERING",
"SUMMARIZATION"
] | Non_BioNLP |
briefai/LongShort-Mistral-7B | briefai | text-generation | [
"safetensors",
"pytorch",
"mistral",
"Gen-AI",
"Finance",
"KPI Extraction",
"text-generation",
"conversational",
"en",
"dataset:briefai/LongShort-Dataset",
"license:apache-2.0",
"region:us"
] | 1,701,283,360,000 | 2024-01-18T21:50:03 | 0 | 0 | ---
datasets:
- briefai/LongShort-Dataset
language:
- en
license: apache-2.0
pipeline_tag: text-generation
tags:
- pytorch
- mistral
- Gen-AI
- Finance
- KPI Extraction
---
# LongShort-Mistral-7B
### Model Description
LongShort-Mistral-7B is a large language model fine-tuned on earnings call documents to extract financial KPIs from the earnings call documents. It is based on the Mistral-7B Instruct Architecture.
- Model creator: [Brief AI](https://huggingface.co/briefai)
- Original model: [Mistral-7B-Instruct](https://huggingface.co/mistralai/Mistral-7B-Instruct-v0.1)
### Dataset Description
- Data Source: Factiva
- Data Description: 28K+ Earnings Call Documents
- Data Scope: 1K+ public companies
- Fine Tuning Data: Collection of 60K+ samples.
## Prompt template: LongShort-Mistral-7B
```
[INST]Given the context, answer the question.
### Question:
Extract all the finance-based performance indicators and evaluation metrics.
### Context:
{context}
### Answer:
[/INST]
```
## Basics
*This section provides information about the model type, version, license, funders, release date, developers, and contact information.*
*It is useful for anyone who wants to reference the model.*
**Developed by:** [Brief AI Team](https://huggingface.co/briefai)
**Model Type:** Transformer-based Large Language Model
**Version:** 1.0.0
**Languages:** English
**License:** Apache 2.0
**Release Date Estimate:** Wednesday, 29.November.2023
**Send Questions to:** [email protected]
**Cite as:** Brief AI LongShort Language Model
**Funded by:** UChicago Data Science Institute
**Mentored by:** Nick Kadochnikov
## Technical Specifications
*This section includes details about the model objective and architecture, and the compute infrastructure.*
*It is useful for people interested in model development.*
Please see [the LongShort training README](https://github.com/brief-ai-uchicago/LongShort-Dataset) for full details on replicating training.
### Model Architecture and Objective
* Modified from Mistral-7B-Instruct
**Objective:** Financial KPI extraction from earnings call documents.
### Hardware and Software - Compute Infrastructure
* 4 NVIDIA L4 GPUs & 48 vCPUs
* Environment: PyTorch (pytorch-2.0 w/ CUDA-11.8; see [Github link](https://github.com/pytorch/pytorch))
* CPU: GCP G2 Standard 48 (Platform: Intel Cascade Lake) (Accelerator Optimized)
* CPU memory: 192GB RAM
* GPU memory: 30GB per GPU
## Training
*This section provides information about the training.*
*It is useful for people who want to learn more about the model inputs and training footprint.*
The following bits and bytes quantization config was used during training:
* quant_method: bitsandbytes
* load_in_8bit: False
* load_in_4bit: True
* llm_int8_threshold: 6.0
* llm_int8_skip_modules: None
* llm_int8_enable_fp32_cpu_offload: False
* llm_int8_has_fp16_weight: False
* bnb_4bit_quant_type: nf4
* bnb_4bit_use_double_quant: True
* bnb_4bit_compute_dtype: float16
Framework versions
* PEFT 0.4.0
### Training Data
*This section provides a high-level overview of the training data. It is relevant for anyone who wants to know the basics of what the model is learning.*
Details for the dataset can be found in [LongShort Dataset](https://github.com/brief-ai-uchicago/LongShort-Dataset)
Training data includes:
- 5000 Earnings Call Documents
## How to use
This model can be easily used and deployed using HuggingFace's ecosystem. This needs `transformers` and `accelerate` installed. The model can be downloaded as follows:
[LongShort-Mistral-7B](https://huggingface.co/briefai/LongShort-Mistral-7B)
## Intended Use
This model is being created in order to enable public research on large language models (LLMs). LLMs are intended to be used for language generation or as a pre-trained base model that can be further fine-tuned for specific tasks. The use cases below are not exhaustive.
### Direct Use
- Text generation
- Exploring characteristics of language generated by a language model
- Examples: Cloze tests, counterfactuals, generations with reframings
### Downstream Use
- Tasks that leverage language models include: Information Extraction, Question Answering, Summarization
#### Out-of-scope Uses
Using the model in [high-stakes](#high-stakes) settings is out of scope for this model. The model is not designed for [critical decisions](#critical-decisions) nor uses with any material consequences on an individual's livelihood or wellbeing. The model outputs content that appears factual but may not be correct.
Out-of-scope Uses Include:
- Usage for evaluating or scoring individuals, such as for employment, education, or credit
- Applying the model for critical automatic decisions, generating factual content, creating reliable summaries, or generating predictions that must be correct
#### Misuse
Intentionally using the model for harm, violating [human rights](#human-rights), or other kinds of malicious activities, is a misuse of this model. This includes:
- Spam generation
- Disinformation and influence operations
- Disparagement and defamation
- Harassment and abuse
- [Deception](#deception)
- Unconsented impersonation and imitation
- Unconsented surveillance
- Generating content without attribution to the model, as specified in the [RAIL License, Use Restrictions](https://huggingface.co/spaces/bigscience/license)
## Intended Users
### Direct Users
- General Public
- Researchers
- Students
- Educators
- Engineers/developers
- Non-commercial entities
- Financial Industry
# Risks and Limitations
*This section identifies foreseeable harms and misunderstandings.*
Model may:
- Overrepresent some viewpoints and underrepresent others
- Contain stereotypes
- Contain [personal information](#personal-data-and-information)
- Generate:
- Hateful, abusive, or violent language
- Discriminatory or prejudicial language
- Content that may not be appropriate for all settings, including sexual content
- Make errors, including producing incorrect information as if it were factual
- Generate irrelevant or repetitive outputs
- Induce users into attributing human traits to it, such as sentience or consciousness
# Evaluation
*This section describes the evaluation protocols and provides the results.*
Result: LongShort-Llama-2-13B gives 43.4% accuracy on a validation set of 10% of the original training dataset.
**Train-time Evaluation:**
Final checkpoint after 300 epochs:
- Training Loss: 1.228
# Recommendations
*This section provides information on warnings and potential mitigations.*
- Indirect users should be made aware when the content they're working with is created by the LLM.
- Users should be aware of [Risks and Limitations](#risks-and-limitations), and include an appropriate age disclaimer or blocking interface as necessary.
- Users of the model should provide mechanisms for those affected to provide feedback, such as an email address for comments.
# Model Card Authors
Vishal Parameshwaran, Garima Sohi, Jose Gerala, Sanchit Narayan Kumar
| [
"QUESTION_ANSWERING",
"SUMMARIZATION"
] | Non_BioNLP |
Subsets and Splits