Training-Free Efficient Video Generation via Dynamic Token Carving
Abstract
Jenga, a novel inference pipeline for video Diffusion Transformer models, combines dynamic attention carving and progressive resolution generation to significantly speed up video generation while maintaining high quality.
Despite the remarkable generation quality of video Diffusion Transformer (DiT) models, their practical deployment is severely hindered by extensive computational requirements. This inefficiency stems from two key challenges: the quadratic complexity of self-attention with respect to token length and the multi-step nature of diffusion models. To address these limitations, we present Jenga, a novel inference pipeline that combines dynamic attention carving with progressive resolution generation. Our approach leverages two key insights: (1) early denoising steps do not require high-resolution latents, and (2) later steps do not require dense attention. Jenga introduces a block-wise attention mechanism that dynamically selects relevant token interactions using 3D space-filling curves, alongside a progressive resolution strategy that gradually increases latent resolution during generation. Experimental results demonstrate that Jenga achieves substantial speedups across multiple state-of-the-art video diffusion models while maintaining comparable generation quality (8.83times speedup with 0.01\% performance drop on VBench). As a plug-and-play solution, Jenga enables practical, high-quality video generation on modern hardware by reducing inference time from minutes to seconds -- without requiring model retraining. Code: https://github.com/dvlab-research/Jenga
Community
Jenga can generate videos with 4.68-10.35 times faster on a single GPU.
Hope you enjoy this paper~
Code: https://github.com/dvlab-research/Jenga
Project Page: https://julianjuaner.github.io/projects/jenga/
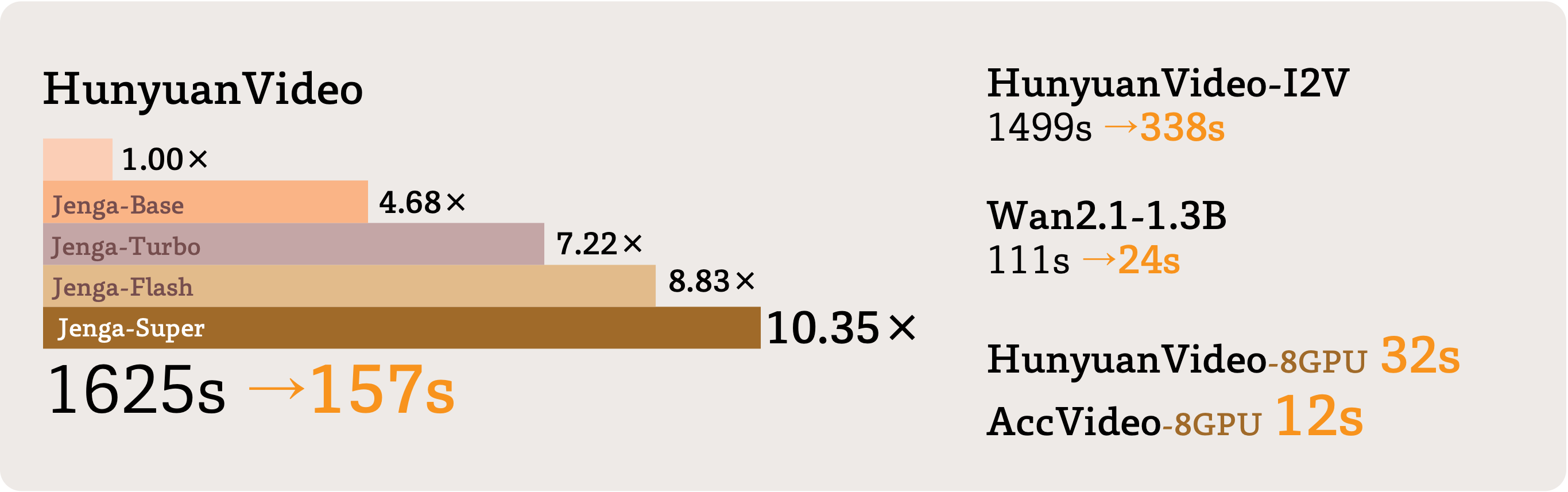

This is an automated message from the Librarian Bot. I found the following papers similar to this paper.
The following papers were recommended by the Semantic Scholar API
- Turbo2K: Towards Ultra-Efficient and High-Quality 2K Video Synthesis (2025)
- VGDFR: Diffusion-based Video Generation with Dynamic Latent Frame Rate (2025)
- DiTFastAttnV2: Head-wise Attention Compression for Multi-Modality Diffusion Transformers (2025)
- Model Reveals What to Cache: Profiling-Based Feature Reuse for Video Diffusion Models (2025)
- Mask2DiT: Dual Mask-based Diffusion Transformer for Multi-Scene Long Video Generation (2025)
- DiVE: Efficient Multi-View Driving Scenes Generation Based on Video Diffusion Transformer (2025)
- MAR-3D: Progressive Masked Auto-regressor for High-Resolution 3D Generation (2025)
Please give a thumbs up to this comment if you found it helpful!
If you want recommendations for any Paper on Hugging Face checkout this Space
You can directly ask Librarian Bot for paper recommendations by tagging it in a comment:
@librarian-bot
recommend
Models citing this paper 0
No model linking this paper
Datasets citing this paper 0
No dataset linking this paper
Spaces citing this paper 0
No Space linking this paper
Collections including this paper 0
No Collection including this paper