Post
2090
🚀 We are delighted to announce MamayLM, a new state-of-the-art efficient Ukrainian LLM!
📈 MamayLM surpasses similar-sized models in both English and Ukrainian, while matching or overtaking up to 10x larger models.
📊 MamayLM is a 9B model that can run on a single GPU, enabling cost-efficient AI autonomy and adoption across sectors in Ukraine such as education, legal, healthcare, public services and others (e.g., by specializing it to particular use cases). MalayLM is also attractive for organizations wishing to preserve data privacy as it s efficiency allows it to run on a local machine.
🧠 MamayLM is trained on high-quality Ukrainian data and understands Ukrainian language, culture, and history. It is built on top of Google’s Gemma 2 9B model, but uses a number of new advances stemming from INSAIT’s experience in creating BgGPT, a Bulgarian LLM we released last year, now adopted nationwide and profiled several times by Google as a worldwide success case.
🤝 MamayLM is developed in a collaboration between researchers at INSAIT and ETH Zürich and is trained entirely via donations to INSAIT for AI compute resources.
📥 MamayLM is now freely available to download on INSAIT’s HuggingFace in both full and quantized versions. We also publicly release all Ukrainian benchmarks we evaluated on.
📝 Further, we release blog posts in both English and Ukrainian, sharing our approach to creating MamayLM, hoping to drive further improvements by the community.
🌎 The release of LLMs for various languages is part of INSAIT’s mission in ensuring countries can achieve AI autonomy in a cost-efficient, controlled, safe and predictable manner.
MamayLM model and benchmarks:
INSAIT-Institute
Blog (EN): https://huggingface.co/blog/INSAIT-Institute/mamaylm
Blog (UKR): https://huggingface.co/blog/INSAIT-Institute/mamaylm-ukr
📈 MamayLM surpasses similar-sized models in both English and Ukrainian, while matching or overtaking up to 10x larger models.
📊 MamayLM is a 9B model that can run on a single GPU, enabling cost-efficient AI autonomy and adoption across sectors in Ukraine such as education, legal, healthcare, public services and others (e.g., by specializing it to particular use cases). MalayLM is also attractive for organizations wishing to preserve data privacy as it s efficiency allows it to run on a local machine.
🧠 MamayLM is trained on high-quality Ukrainian data and understands Ukrainian language, culture, and history. It is built on top of Google’s Gemma 2 9B model, but uses a number of new advances stemming from INSAIT’s experience in creating BgGPT, a Bulgarian LLM we released last year, now adopted nationwide and profiled several times by Google as a worldwide success case.
🤝 MamayLM is developed in a collaboration between researchers at INSAIT and ETH Zürich and is trained entirely via donations to INSAIT for AI compute resources.
📥 MamayLM is now freely available to download on INSAIT’s HuggingFace in both full and quantized versions. We also publicly release all Ukrainian benchmarks we evaluated on.
📝 Further, we release blog posts in both English and Ukrainian, sharing our approach to creating MamayLM, hoping to drive further improvements by the community.
🌎 The release of LLMs for various languages is part of INSAIT’s mission in ensuring countries can achieve AI autonomy in a cost-efficient, controlled, safe and predictable manner.
MamayLM model and benchmarks:
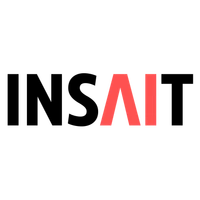
Blog (EN): https://huggingface.co/blog/INSAIT-Institute/mamaylm
Blog (UKR): https://huggingface.co/blog/INSAIT-Institute/mamaylm-ukr