File size: 18,928 Bytes
4cba72f 508c460 |
1 2 3 4 5 6 7 8 9 10 11 12 13 14 15 16 17 18 19 20 21 22 23 24 25 26 27 28 29 30 31 32 33 34 35 36 37 38 39 40 41 42 43 44 45 46 47 48 49 50 51 52 53 54 55 56 57 58 59 60 61 62 63 64 65 66 67 68 69 70 71 72 73 74 75 76 77 78 79 80 81 82 83 84 85 86 87 88 89 90 91 92 93 94 95 96 97 98 99 100 101 102 103 104 105 106 107 108 109 110 111 112 113 114 115 116 117 118 119 120 121 122 123 124 125 126 127 128 129 130 131 132 133 134 135 136 137 138 139 140 141 142 143 144 145 146 147 148 149 150 151 152 153 154 155 156 157 158 159 160 161 162 163 164 165 166 167 168 169 170 171 172 173 174 175 176 177 178 179 180 181 182 183 184 185 186 187 188 189 190 191 192 193 194 195 196 197 198 199 200 201 202 203 204 205 206 207 208 209 210 211 212 213 214 215 216 217 218 219 220 221 222 223 224 225 226 227 228 229 230 231 232 233 234 235 236 237 238 239 240 241 242 243 244 245 246 247 248 249 250 251 252 253 254 255 256 257 258 259 260 261 262 263 264 265 266 267 268 269 270 271 272 273 274 275 276 277 278 279 280 281 282 283 284 285 286 287 288 289 290 291 292 293 294 295 296 297 298 299 300 301 302 303 304 305 306 307 308 309 310 311 312 313 314 315 316 317 318 319 320 321 322 323 324 325 326 327 328 329 330 331 332 333 334 335 336 337 338 339 340 341 342 343 344 345 346 347 348 349 350 351 352 353 354 355 356 357 358 359 360 361 362 363 364 365 366 367 368 369 370 371 372 373 374 375 376 377 378 379 380 381 382 383 384 385 386 387 388 389 390 391 392 393 394 395 396 397 398 399 400 401 402 403 404 405 406 407 408 409 410 411 412 413 414 415 416 |
---
license: mit
---
<h1 align='center'>Hallo2: Long-Duration and High-Resolution Audio-driven Portrait Image Animation</h1>
<div align='center'>
<a href='https://github.com/cuijh26' target='_blank'>Jiahao Cui</a><sup>1*</sup> 
<a href='https://github.com/crystallee-ai' target='_blank'>Hui Li</a><sup>1*</sup> 
<a href='https://yoyo000.github.io/' target='_blank'>Yao Yao</a><sup>3</sup> 
<a href='http://zhuhao.cc/home/' target='_blank'>Hao Zhu</a><sup>3</sup> 
<a href='https://github.com/NinoNeumann' target='_blank'>Hanlin Shang</a><sup>1</sup> 
<a href='https://github.com/Kaihui-Cheng' target='_blank'>Kaihui Cheng</a><sup>1</sup> 
<a href='' target='_blank'>Hang Zhou</a><sup>2</sup> 
</div>
<div align='center'>
<a href='https://sites.google.com/site/zhusiyucs/home' target='_blank'>Siyu Zhu</a><sup>1✉️</sup> 
<a href='https://jingdongwang2017.github.io/' target='_blank'>Jingdong Wang</a><sup>2</sup> 
</div>
<div align='center'>
<sup>1</sup>Fudan University  <sup>2</sup>Baidu Inc  <sup>3</sup>Nanjing University
</div>
<br>
<div align='center'>
<a href='https://github.com/fudan-generative-vision/hallo2'><img src='https://img.shields.io/github/stars/fudan-generative-vision/hallo2?style=social'></a>
<a href='https://fudan-generative-vision.github.io/hallo2/#/'><img src='https://img.shields.io/badge/Project-HomePage-Green'></a>
<a href='https://arxiv.org/abs/2410.07718'><img src='https://img.shields.io/badge/Paper-Arxiv-red'></a>
<a href='https://huggingface.co/fudan-generative-ai/hallo2'><img src='https://img.shields.io/badge/%F0%9F%A4%97%20HuggingFace-Model-yellow'></a>
<a href='assets/wechat.jpeg'><img src='https://badges.aleen42.com/src/wechat.svg'></a>
</div>
<br>
## 📸 Showcase
<table class="center">
<tr>
<td style="text-align: center"><b>Tailor Swift Speech @ NYU (4K, 23 minutes)</b></td>
<td style="text-align: center"><b>Johan Rockstrom Speech @ TED (4K, 18 minutes)</b></td>
</tr>
<tr>
<td style="text-align: center"><a target="_blank" href="https://cdn.aondata.work/hallo2/videos/showcases/TailorSpeech.mp4"><img src="https://cdn.aondata.work/hallo2/videos/showcases/gifs/TailorSpeechGIF.gif"></a></td>
<td style="text-align: center"><a target="_blank" href="https://cdn.aondata.work/hallo2/videos/showcases/TEDSpeech.mp4"><img src="https://cdn.aondata.work/hallo2/videos/showcases/gifs/TEDSpeechGIF.gif"></a></td>
</tr>
<tr>
<td style="text-align: center"><b>Churchill's Iron Curtain Speech (4K, 4 minutes)</b></td>
<td style="text-align: center"><b>An LLM Course from Stanford (4K, up to 1 hour)</b></td>
</tr>
<tr>
<td style="text-align: center"><a target="_blank" href="https://cdn.aondata.work/hallo2/videos/showcases/DarkestHour.mp4"><img src="https://cdn.aondata.work/hallo2/videos/showcases/gifs/DarkestHour.gif"></a></td>
<td style="text-align: center"><a target="_blank" href="https://cdn.aondata.work/hallo2/videos/showcases/LLMCourse.mp4"><img src="https://cdn.aondata.work/hallo2/videos/showcases/gifs/LLMCourseGIF.gif"></a></td>
</tr>
</table>
Visit our [project page](https://fudan-generative-vision.github.io/hallo2/#/) to view more cases.
## 🔧️ Framework
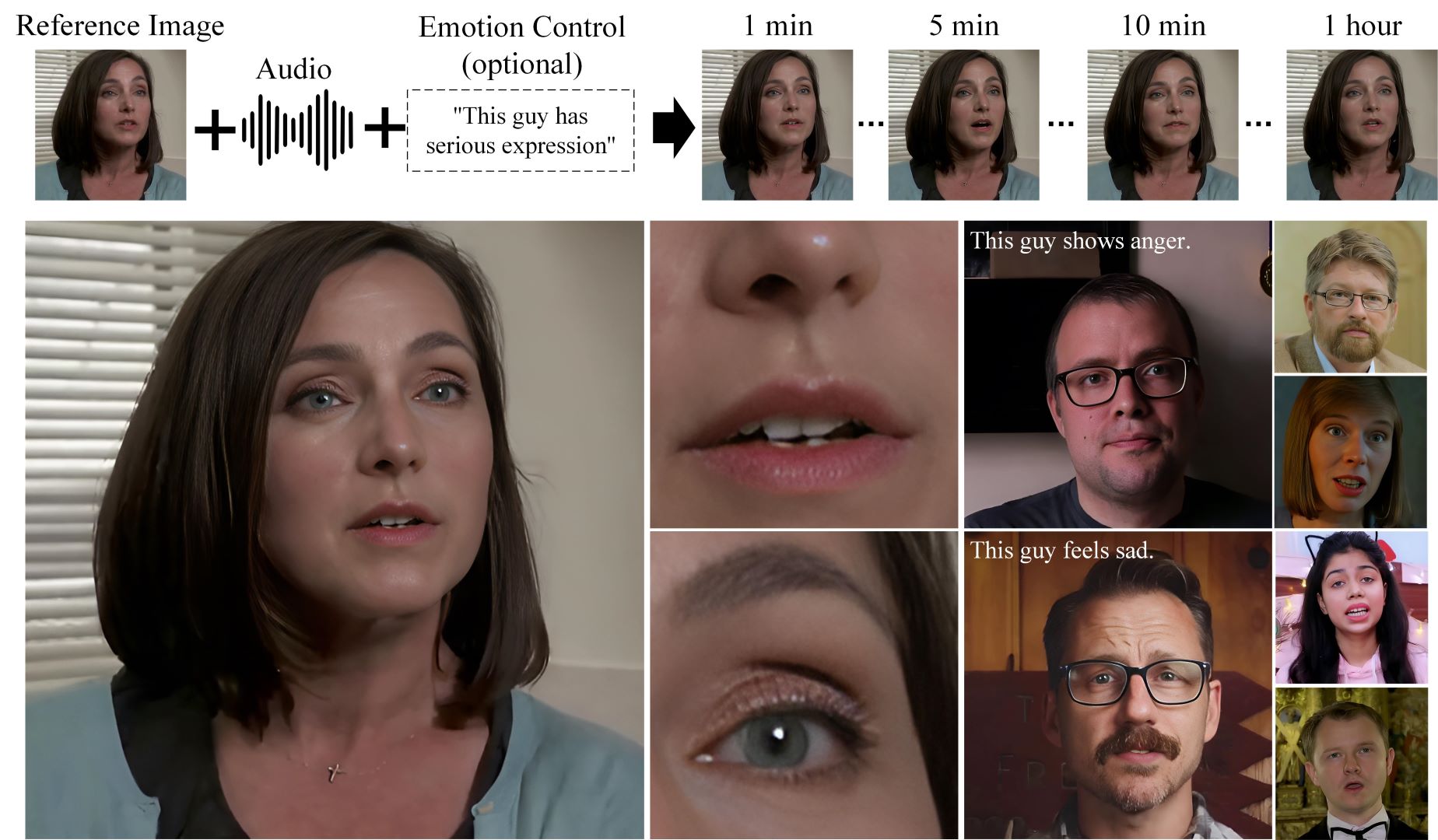
## ⚙️ Installation
- System requirement: Ubuntu 20.04/Ubuntu 22.04, Cuda 11.8
- Tested GPUs: A100
Create conda environment:
```bash
conda create -n hallo python=3.10
conda activate hallo
```
Install packages with `pip`
```bash
pip install torch==2.2.2 torchvision==0.17.2 torchaudio==2.2.2 --index-url https://download.pytorch.org/whl/cu118
pip install -r requirements.txt
```
Besides, ffmpeg is also needed:
```bash
apt-get install ffmpeg
```
### 📥 Download Pretrained Models
You can easily get all pretrained models required by inference from our [HuggingFace repo](https://huggingface.co/fudan-generative-ai/hallo2).
Clone the pretrained models into `${PROJECT_ROOT}/pretrained_models` directory by cmd below:
```shell
git lfs install
git clone https://huggingface.co/fudan-generative-ai/hallo2 pretrained_models
```
Or you can download them separately from their source repo:
- [hallo](https://huggingface.co/fudan-generative-ai/hallo2/tree/main/hallo2): Our checkpoints consist of denoising UNet, face locator, image & audio proj.
- [audio_separator](https://huggingface.co/huangjackson/Kim_Vocal_2): Kim*Vocal_2 MDX-Net vocal removal model. (\_Thanks to [KimberleyJensen](https://github.com/KimberleyJensen)*)
- [insightface](https://github.com/deepinsight/insightface/tree/master/python-package#model-zoo): 2D and 3D Face Analysis placed into `pretrained_models/face_analysis/models/`. (_Thanks to deepinsight_)
- [face landmarker](https://storage.googleapis.com/mediapipe-models/face_landmarker/face_landmarker/float16/1/face_landmarker.task): Face detection & mesh model from [mediapipe](https://ai.google.dev/edge/mediapipe/solutions/vision/face_landmarker#models) placed into `pretrained_models/face_analysis/models`.
- [motion module](https://github.com/guoyww/AnimateDiff/blob/main/README.md#202309-animatediff-v2): motion module from [AnimateDiff](https://github.com/guoyww/AnimateDiff). (_Thanks to [guoyww](https://github.com/guoyww)_).
- [sd-vae-ft-mse](https://huggingface.co/stabilityai/sd-vae-ft-mse): Weights are intended to be used with the diffusers library. (_Thanks to [stablilityai](https://huggingface.co/stabilityai)_)
- [StableDiffusion V1.5](https://huggingface.co/runwayml/stable-diffusion-v1-5): Initialized and fine-tuned from Stable-Diffusion-v1-2. (_Thanks to [runwayml](https://huggingface.co/runwayml)_)
- [wav2vec](https://huggingface.co/facebook/wav2vec2-base-960h): wav audio to vector model from [Facebook](https://huggingface.co/facebook/wav2vec2-base-960h).
- [facelib](https://github.com/sczhou/CodeFormer/releases/tag/v0.1.0): pretrained face parse models
- [realesrgan](https://github.com/sczhou/CodeFormer/releases/download/v0.1.0/RealESRGAN_x2plus.pth): background upsample model
- [CodeFormer](https://github.com/sczhou/CodeFormer/releases/download/v0.1.0): pretrained [Codeformer](https://github.com/sczhou/CodeFormer) model, it's optional to download it, only if you want to train our video super-resolution model from scratch
Finally, these pretrained models should be organized as follows:
```text
./pretrained_models/
|-- audio_separator/
| |-- download_checks.json
| |-- mdx_model_data.json
| |-- vr_model_data.json
| `-- Kim_Vocal_2.onnx
|-- CodeFormer/
| |-- codeformer.pth
| `-- vqgan_code1024.pth
|-- face_analysis/
| `-- models/
| |-- face_landmarker_v2_with_blendshapes.task # face landmarker model from mediapipe
| |-- 1k3d68.onnx
| |-- 2d106det.onnx
| |-- genderage.onnx
| |-- glintr100.onnx
| `-- scrfd_10g_bnkps.onnx
|-- facelib
| |-- detection_mobilenet0.25_Final.pth
| |-- detection_Resnet50_Final.pth
| |-- parsing_parsenet.pth
| |-- yolov5l-face.pth
| `-- yolov5n-face.pth
|-- hallo2
| |-- net_g.pth
| `-- net.pth
|-- motion_module/
| `-- mm_sd_v15_v2.ckpt
|-- realesrgan
| `-- RealESRGAN_x2plus.pth
|-- sd-vae-ft-mse/
| |-- config.json
| `-- diffusion_pytorch_model.safetensors
|-- stable-diffusion-v1-5/
| `-- unet/
| |-- config.json
| `-- diffusion_pytorch_model.safetensors
`-- wav2vec/
`-- wav2vec2-base-960h/
|-- config.json
|-- feature_extractor_config.json
|-- model.safetensors
|-- preprocessor_config.json
|-- special_tokens_map.json
|-- tokenizer_config.json
`-- vocab.json
```
### 🛠️ Prepare Inference Data
Hallo has a few simple requirements for input data:
For the source image:
1. It should be cropped into squares.
2. The face should be the main focus, making up 50%-70% of the image.
3. The face should be facing forward, with a rotation angle of less than 30° (no side profiles).
For the driving audio:
1. It must be in WAV format.
2. It must be in English since our training datasets are only in this language.
3. Ensure the vocals are clear; background music is acceptable.
We have provided [some samples](examples/) for your reference.
### 🎮 Run Inference
#### Long-Duration animation
Simply to run the `scripts/inference_long.py` and change `source_image`, `driving_audio` and `save_path` in the config file:
```bash
python scripts/inference_long.py --config ./configs/inference/long.yaml
```
Animation results will be saved at `save_path`. You can find more examples for inference at [examples folder](https://github.com/fudan-generative-vision/hallo2/tree/main/examples).
For more options:
```shell
usage: inference_long.py [-h] [-c CONFIG] [--source_image SOURCE_IMAGE] [--driving_audio DRIVING_AUDIO] [--pose_weight POSE_WEIGHT]
[--face_weight FACE_WEIGHT] [--lip_weight LIP_WEIGHT] [--face_expand_ratio FACE_EXPAND_RATIO]
options:
-h, --help show this help message and exit
-c CONFIG, --config CONFIG
--source_image SOURCE_IMAGE
source image
--driving_audio DRIVING_AUDIO
driving audio
--pose_weight POSE_WEIGHT
weight of pose
--face_weight FACE_WEIGHT
weight of face
--lip_weight LIP_WEIGHT
weight of lip
--face_expand_ratio FACE_EXPAND_RATIO
face region
```
#### High-Resolution animation
Simply to run the `scripts/video_sr.py` and pass `input_video` and `output_path`:
```bash
python scripts/video_sr.py --input_path [input_video] --output_path [output_dir] --bg_upsampler realesrgan --face_upsample -w 1 -s 4
```
Animation results will be saved at `output_dir`.
For more options:
```shell
usage: video_sr.py [-h] [-i INPUT_PATH] [-o OUTPUT_PATH] [-w FIDELITY_WEIGHT] [-s UPSCALE] [--has_aligned] [--only_center_face] [--draw_box]
[--detection_model DETECTION_MODEL] [--bg_upsampler BG_UPSAMPLER] [--face_upsample] [--bg_tile BG_TILE] [--suffix SUFFIX]
options:
-h, --help show this help message and exit
-i INPUT_PATH, --input_path INPUT_PATH
Input video
-o OUTPUT_PATH, --output_path OUTPUT_PATH
Output folder.
-w FIDELITY_WEIGHT, --fidelity_weight FIDELITY_WEIGHT
Balance the quality and fidelity. Default: 0.5
-s UPSCALE, --upscale UPSCALE
The final upsampling scale of the image. Default: 2
--has_aligned Input are cropped and aligned faces. Default: False
--only_center_face Only restore the center face. Default: False
--draw_box Draw the bounding box for the detected faces. Default: False
--detection_model DETECTION_MODEL
Face detector. Optional: retinaface_resnet50, retinaface_mobile0.25, YOLOv5l, YOLOv5n. Default: retinaface_resnet50
--bg_upsampler BG_UPSAMPLER
Background upsampler. Optional: realesrgan
--face_upsample Face upsampler after enhancement. Default: False
--bg_tile BG_TILE Tile size for background sampler. Default: 400
--suffix SUFFIX Suffix of the restored faces. Default: None
```
> NOTICE: The High-Resolution animation feature is a modified version of [CodeFormer](https://github.com/sczhou/CodeFormer). When using or redistributing this feature, please comply with the [S-Lab License 1.0](https://github.com/sczhou/CodeFormer?tab=License-1-ov-file). We kindly request that you respect the terms of this license in any usage or redistribution of this component.
## Training
### Long-Duration animation
#### prepare data for training
The training data, which utilizes some talking-face videos similar to the source images used for inference, also needs to meet the following requirements:
1. It should be cropped into squares.
2. The face should be the main focus, making up 50%-70% of the image.
3. The face should be facing forward, with a rotation angle of less than 30° (no side profiles).
Organize your raw videos into the following directory structure:
```text
dataset_name/
|-- videos/
| |-- 0001.mp4
| |-- 0002.mp4
| |-- 0003.mp4
| `-- 0004.mp4
```
You can use any `dataset_name`, but ensure the `videos` directory is named as shown above.
Next, process the videos with the following commands:
```bash
python -m scripts.data_preprocess --input_dir dataset_name/videos --step 1
python -m scripts.data_preprocess --input_dir dataset_name/videos --step 2
```
**Note:** Execute steps 1 and 2 sequentially as they perform different tasks. Step 1 converts videos into frames, extracts audio from each video, and generates the necessary masks. Step 2 generates face embeddings using InsightFace and audio embeddings using Wav2Vec, and requires a GPU. For parallel processing, use the `-p` and `-r` arguments. The `-p` argument specifies the total number of instances to launch, dividing the data into `p` parts. The `-r` argument specifies which part the current process should handle. You need to manually launch multiple instances with different values for `-r`.
Generate the metadata JSON files with the following commands:
```bash
python scripts/extract_meta_info_stage1.py -r path/to/dataset -n dataset_name
python scripts/extract_meta_info_stage2.py -r path/to/dataset -n dataset_name
```
Replace `path/to/dataset` with the path to the parent directory of `videos`, such as `dataset_name` in the example above. This will generate `dataset_name_stage1.json` and `dataset_name_stage2.json` in the `./data` directory.
#### Training
Update the data meta path settings in the configuration YAML files, `configs/train/stage1.yaml` and `configs/train/stage2_long.yaml`:
```yaml
#stage1.yaml
data:
meta_paths:
- ./data/dataset_name_stage1.json
#stage2.yaml
data:
meta_paths:
- ./data/dataset_name_stage2.json
```
Start training with the following command:
```shell
accelerate launch -m \
--config_file accelerate_config.yaml \
--machine_rank 0 \
--main_process_ip 0.0.0.0 \
--main_process_port 20055 \
--num_machines 1 \
--num_processes 8 \
scripts.train_stage1 --config ./configs/train/stage1.yaml
```
##### Accelerate Usage Explanation
The `accelerate launch` command is used to start the training process with distributed settings.
```shell
accelerate launch [arguments] {training_script} --{training_script-argument-1} --{training_script-argument-2} ...
```
**Arguments for Accelerate:**
- `-m, --module`: Interpret the launch script as a Python module.
- `--config_file`: Configuration file for Hugging Face Accelerate.
- `--machine_rank`: Rank of the current machine in a multi-node setup.
- `--main_process_ip`: IP address of the master node.
- `--main_process_port`: Port of the master node.
- `--num_machines`: Total number of nodes participating in the training.
- `--num_processes`: Total number of processes for training, matching the total number of GPUs across all machines.
**Arguments for Training:**
- `{training_script}`: The training script, such as `scripts.train_stage1` or `scripts.train_stage2`.
- `--{training_script-argument-1}`: Arguments specific to the training script. Our training scripts accept one argument, `--config`, to specify the training configuration file.
For multi-node training, you need to manually run the command with different `machine_rank` on each node separately.
For more settings, refer to the [Accelerate documentation](https://huggingface.co/docs/accelerate/en/index).
### High-Resolution animation
#### Training
##### prepare data for training
We use the VFHQ dataset for training, you can download from its [homepage](https://liangbinxie.github.io/projects/vfhq/). Then updata `dataroot_gt` in `./configs/train/video_sr.yaml`.
#### training
Start training with the following command:
```shell
python -m torch.distributed.launch --nproc_per_node=8 --master_port=4322 \
basicsr/train.py -opt ./configs/train/video_sr.yaml \
--launcher pytorch
```
## 📝 Citation
If you find our work useful for your research, please consider citing the paper:
```
@misc{cui2024hallo2,
title={Hallo2: Long-Duration and High-Resolution Audio-driven Portrait Image Animation},
author={Jiahao Cui and Hui Li and Yao Yao and Hao Zhu and Hanlin Shang and Kaihui Cheng and Hang Zhou and Siyu Zhu and️ Jingdong Wang},
year={2024},
eprint={2410.07718},
archivePrefix={arXiv},
primaryClass={cs.CV}
}
```
## 🌟 Opportunities Available
Multiple research positions are open at the **Generative Vision Lab, Fudan University**! Include:
- Research assistant
- Postdoctoral researcher
- PhD candidate
- Master students
Interested individuals are encouraged to contact us at [[email protected]](mailto://[email protected]) for further information.
## ⚠️ Social Risks and Mitigations
The development of portrait image animation technologies driven by audio inputs poses social risks, such as the ethical implications of creating realistic portraits that could be misused for deepfakes. To mitigate these risks, it is crucial to establish ethical guidelines and responsible use practices. Privacy and consent concerns also arise from using individuals' images and voices. Addressing these involves transparent data usage policies, informed consent, and safeguarding privacy rights. By addressing these risks and implementing mitigations, the research aims to ensure the responsible and ethical development of this technology.
## 🤗 Acknowledgements
We would like to thank the contributors to the [magic-animate](https://github.com/magic-research/magic-animate), [AnimateDiff](https://github.com/guoyww/AnimateDiff), [ultimatevocalremovergui](https://github.com/Anjok07/ultimatevocalremovergui), [AniPortrait](https://github.com/Zejun-Yang/AniPortrait) and [Moore-AnimateAnyone](https://github.com/MooreThreads/Moore-AnimateAnyone) repositories, for their open research and exploration.
If we missed any open-source projects or related articles, we would like to complement the acknowledgement of this specific work immediately.
## 👏 Community Contributors
Thank you to all the contributors who have helped to make this project better!
<a href="https://github.com/fudan-generative-vision/hallo2/graphs/contributors">
<img src="https://contrib.rocks/image?repo=fudan-generative-vision/hallo2" />
</a>
|