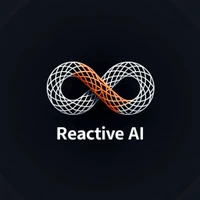
Reactive AI
AI & ML interests
AGI, Reactive Awareness Models, Memory Systems, Reactive Neural Networks
Reactive AI
We are working on our own ideas of Reactive Neural Networks (RxNN) and Event-Driven AI, advancing from language models to AGI awareness models.
Reactive Neural Networks and Event-Driven AI
Reactive Neural Networks (RxNN) are memory-augmented neural networks with higher levels of recurrence (inter-sequence vs. intra-sequence in RNNs), focused on processing single interactions with access to previous interactions via memory layers. We call this event-driven real-time processing to distinguish it from classical data-driven processing of the full conversation history in each interaction. This difference is crucial in case of AGI and awareness - the key feature of humans awareness, is that we remember what we were doing 10 mins ago, without recalling the whole-day history - we are working in real-time - just like event-driven Reactive Neural Networks.
In Event-Driven AI models are processing the data in reaction to environment or internal events, and are emitting other response events as a result. Processing of input and output events by the model is called the interaction. Event or an interaction could occur in any point in continous time. Models have to be stateful and remember the data between the interactions.
Strong Reactive Neural Networks like Reactor could emit and listen to its internal events, while the Weak Reactive Neural Networks are working only on environment events.
Reactor AGI
Our primary architecture - Reactor - is planned as the first awareness AGI model, that's modelling awareness as an Infinite Chain-of-Thoughts, connected to Short-Term and Long-Term Memory (Attention-based Memory System) and Receptors/Effectors systems for real-time reactive processing. It will be able to constantly and autonomously learn from interactions in Continouos Live Learning process.
Reactive Language Models (RxLM)
While the Reactor is the main goal, it's extremely hard to achieve, as it's definitely the most advanced neural network ensemble ever.
That's why we designed simplified architectures, for incremental transformation from language/reasoning models to awareness model:
- Reactive Transformer is introducing Attention-based Memory System and adding Short-Term Memory to Transformer language models
- Preactor is adding Long-Term Memory and ability to learn from interactions
RxT-Alpha Open Research
We are currently working on Reactive Transformer Proof-of-Concept - RxT-Alpha, especially on the new reinforcement learning stage - Memory Reinforcement Learning, that's required for our reactive models, between the Supervised Fine-Tuning and Reinforcement Learning from Human Feedback for reactive models (RxRLHF). The research is open, we are publishing the results of all separate steps, just after finishing them.
The Proof-of-Concept includes 3 small scale models based on Reactive Transformer architecture:
- RxT-Alpha-Micro (~11M params) - pre-training and fine-tuning finished, MRL in progress - training based on small synthetic datasets
- RxT-Alpha-Mini (~70M params) - pre-training in progress - training on real data
- RxT-Alpha (~530M/0.5B params) - pre-training in progress - training on real data
All the models have theoretically infinite context, limited only for single interaction (message + response), but in practice it's limited by short-term memory capacity (it will be improved in Preactor). Limits are:
- RxT-Alpha-Micro - 256 tokens for single interaction, 6 * 256 for STM size (768kb), expected length of a smooth conversation min. ~4k tokens
- RxT-Alpha-Mini - 1024 tokens for single interaction, 8 * 1024 for STM size (8mb), expected length of a smooth conversation min. ~16k tokens
- RxT-Alpha - 2048 tokens for single interaction, 12 * 2048 for STM size (50mb), expected length of a smooth conversation min. ~32k tokens
RxNN Platform
We are working on complete Reactive Neural Networks development framework - RxNN github
Additional Research
- Sparse Query Attention - the most cost-effective GQA variant, reducing training time/cost by ~3-10% with similar performance. Research in progress
Collections
2
models
16
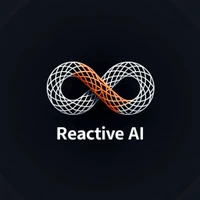
ReactiveAI/RxT-Alpha-Micro-Decoder-SFT
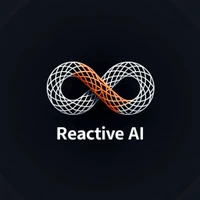
ReactiveAI/RxT-Alpha-Micro-MLM-SFT
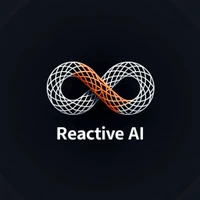
ReactiveAI/RxT-Alpha-Micro-Encoder-SFT
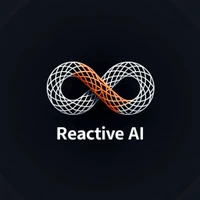
ReactiveAI/RxT-Alpha-Micro-Decoder-Plus
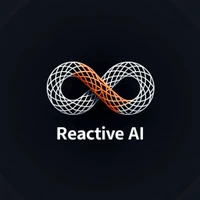
ReactiveAI/RxT-Alpha-Micro-Decoder
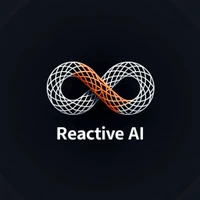
ReactiveAI/RxT-Alpha-Micro-MLM
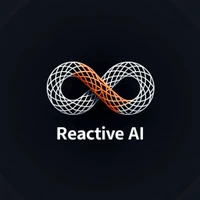
ReactiveAI/RxT-Alpha-Micro-Encoder
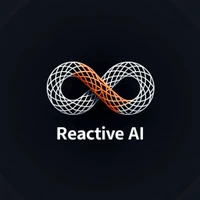
ReactiveAI/GQA-Ref-Micro
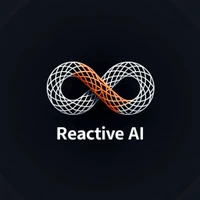
ReactiveAI/xSQAT-mm
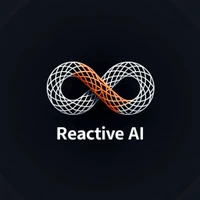