---
license: mit
library_name: transformers
pipeline_tag: image-text-to-text
---

π Paper β’ π Demo β’ π Github β’ π€ LongLLaVA-9B
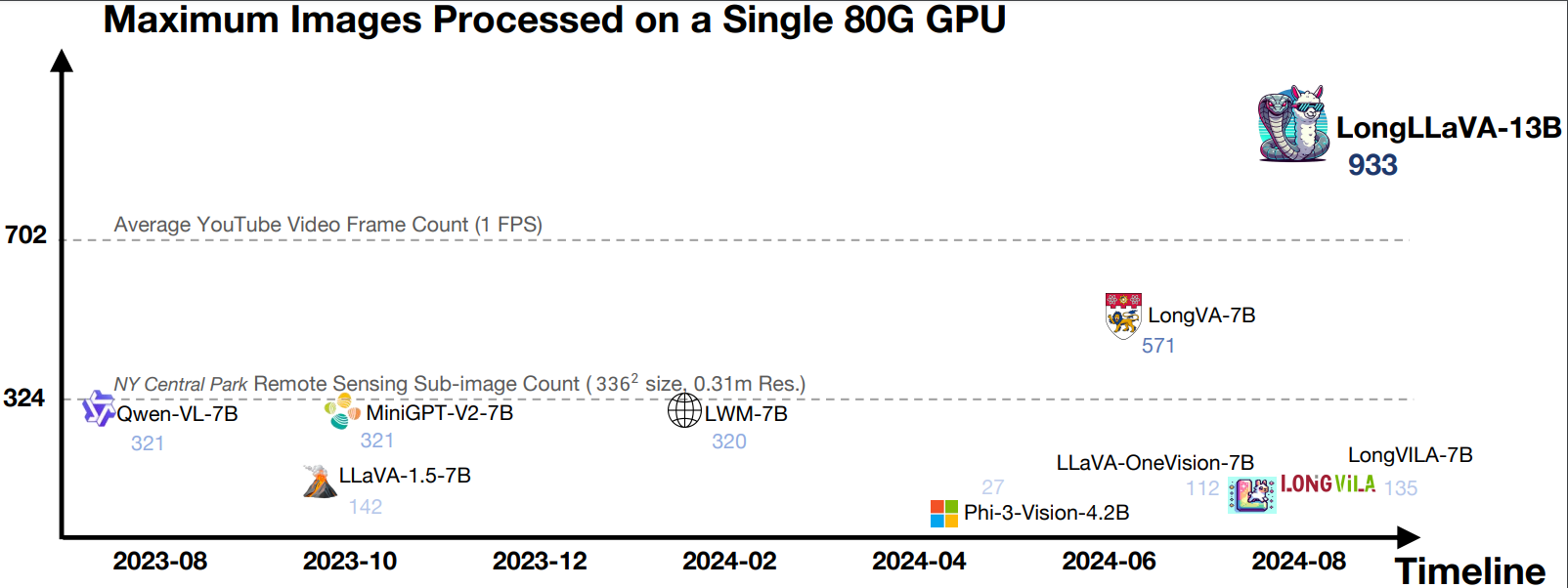
## π Update
* **[2024.09.05]** LongLLaVA repo is publishedοΌπ The Code will
## Architecture
Click to view the architecture image
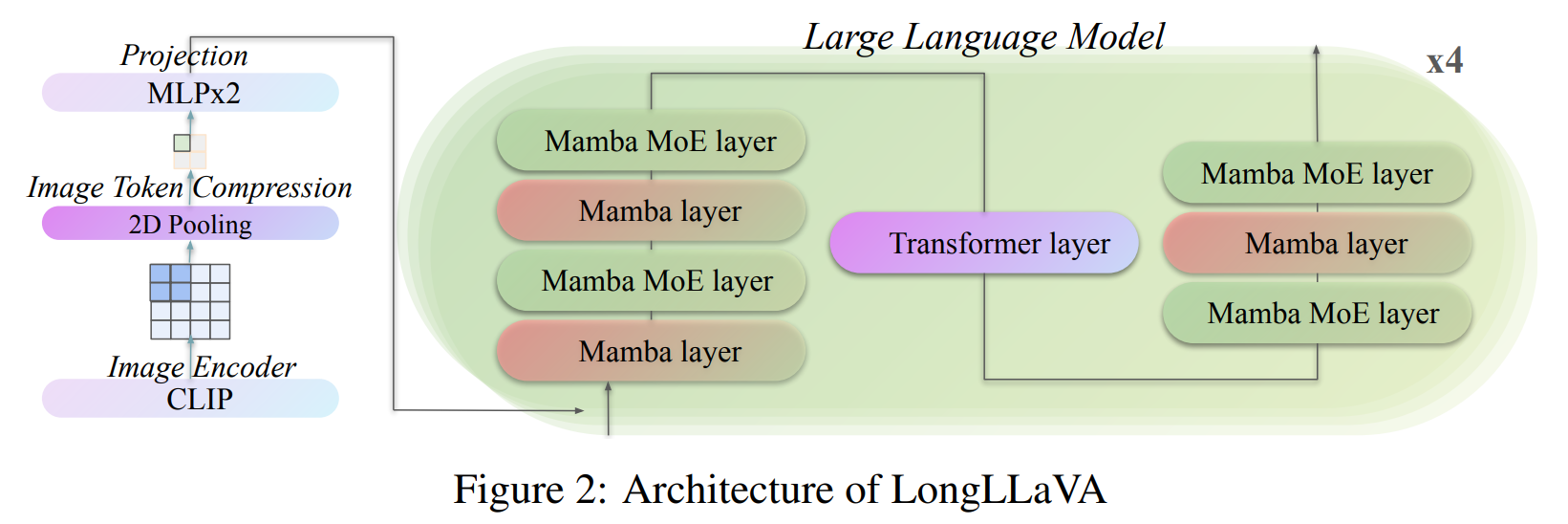
## Results
Click to view the Results
- Main Results
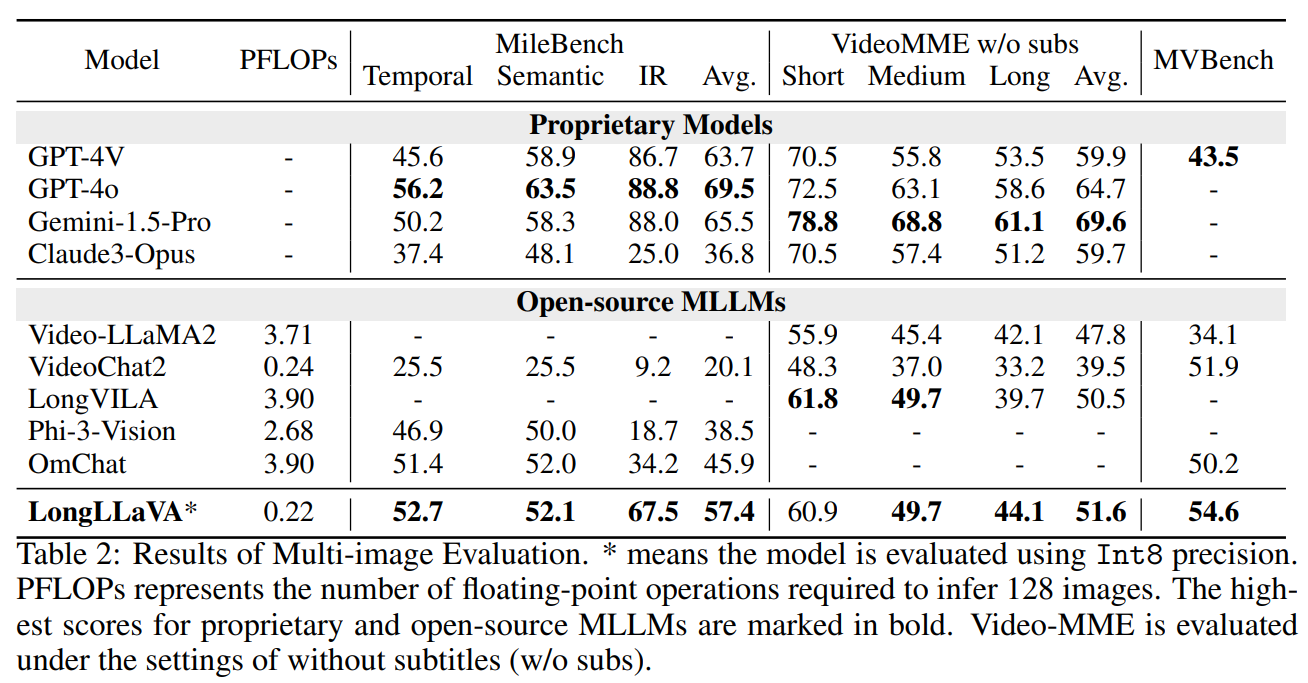
- Diagnostic Results
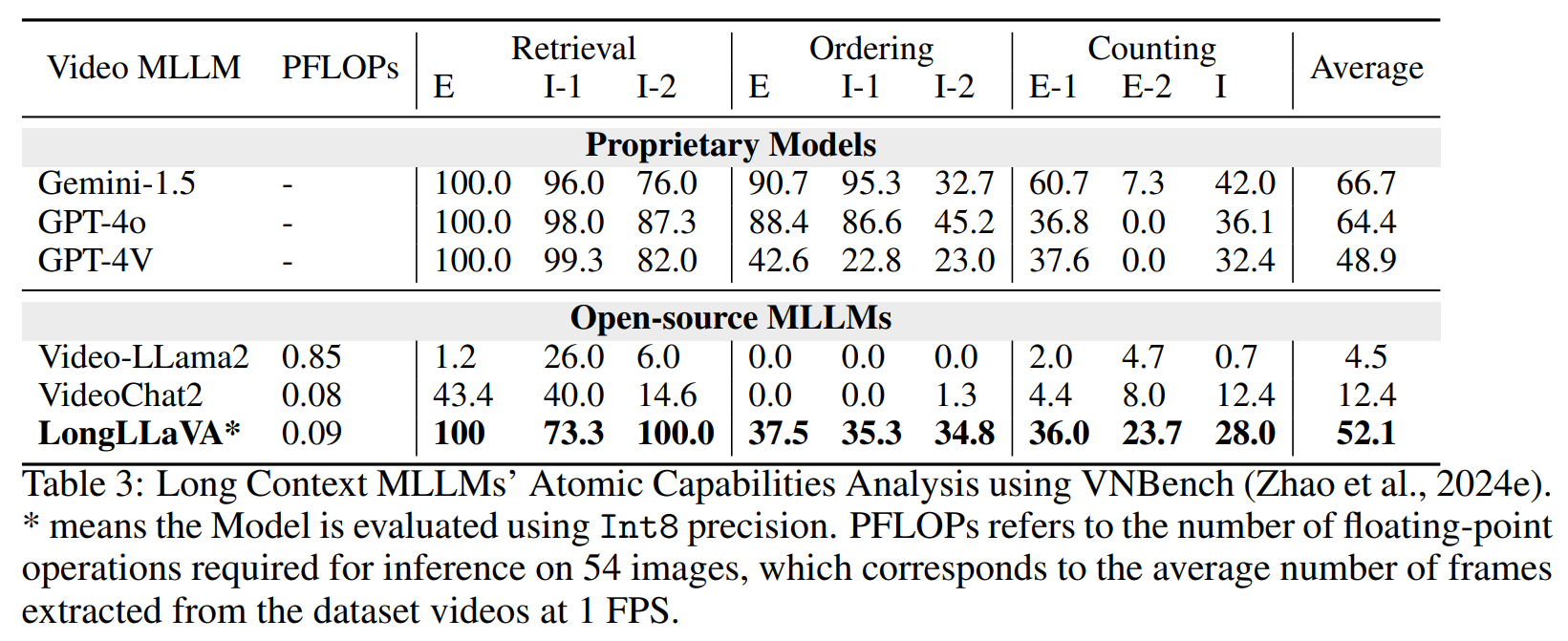
- Video-NIAH
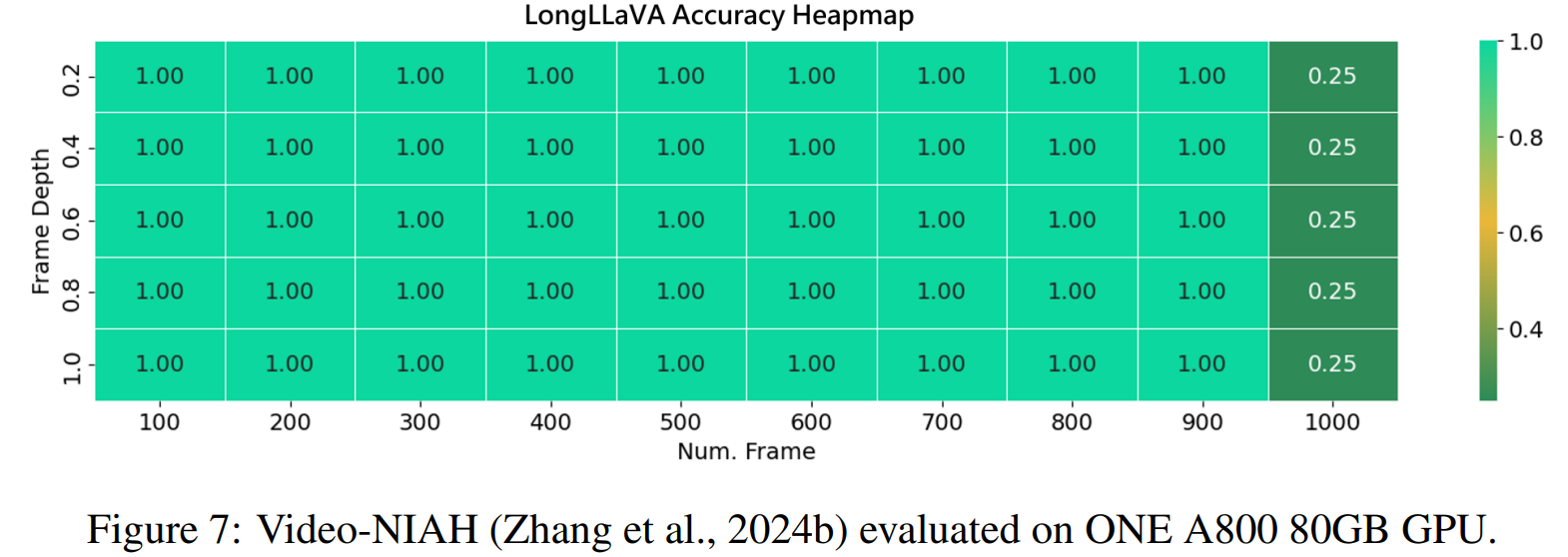
## Results reproduction
### Evaluation
- Preparation
Get the model inference code from [Github](https://github.com/FreedomIntelligence/LongLLaVA).
```bash
git clone https://github.com/FreedomIntelligence/LongLLaVA.git
```
- Environment Setup
```bash
pip install -r requirements.txt
```
- Command Line Interface
```bash
python cli.py --model_dir path-to-longllava
```
- Model Inference
```python
query = 'What does the picture show?'
image_paths = ['image_path1'] # image or video path
from cli import Chatbot
bot = Chatbot(path-to-longllava)
output = bot.chat(query, image_paths)
print(output) # Prints the output of the model
```
## Acknowledgement
- [LLaVA](https://github.com/haotian-liu/LLaVA): Visual Instruction Tuning (LLaVA) built towards GPT-4V level capabilities and beyond.
## Citation
```
@misc{wang2024longllavascalingmultimodalllms,
title={LongLLaVA: Scaling Multi-modal LLMs to 1000 Images Efficiently via Hybrid Architecture},
author={Xidong Wang and Dingjie Song and Shunian Chen and Chen Zhang and Benyou Wang},
year={2024},
eprint={2409.02889},
archivePrefix={arXiv},
primaryClass={cs.CL},
url={https://arxiv.org/abs/2409.02889},
}
```